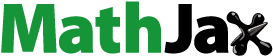
Abstract
The ongoing pandemic of COVID-19 has changed every aspect of life. Most of the people who become a victim of COVID-19 experience mild to moderate symptoms, but some people may become seriously ill. This illness, sometimes, may lead to a very painful death. The Fréchet distribution is one of the flexible distribution for survival time. Hence, in this article, the recovery time of COVID-19 patients is modeled by a new Fréchet-exponential (FE) distribution, and the parameters of the distribution are estimated in the classical and Bayesian paradigms. Since the Bayes estimators using informative priors are not in the closed form, the Lindley and Tierney–Kadane approximation methods are used for their evaluation. The results obtained through simulation studies and the COVID-19 data set assess the superiority of the Bayes estimators over the classical estimators in terms of minimum risks. Mathematically and graphically, it is shown that our proposed model appropriately fits the data set. The minimum values of Akaike information criterion, Bayesian information criterion, corrected Akaike information criterion, and Hannan-Quinn information criterion proves that the FE distribution better fit than the competitors’ distribution for the data set about the recovery time of COVID-19 patients.
1. Introduction
In 2019, as mentioned by Zhu et al. (Citation2020), a novel deadly virus originated in Wuhan, China, and started spreading initially in the state and then in the whole world. The virus was named as coronavirus, COVID-19 and SARC-CoV-2, i.e. severe acute respiratory syndrome coronavirus-2. In 2020, the virus got spread all over the world.
Lutchmansingh et al. (Citation2021) mentioned that COVID-19 has infected a huge population around the world. It has infected people of all ages, but elderly people or people with certain acute diseases are at higher risk of getting infected. The spread rate of COVID-19 is higher as it is transmitted from person to person by droplets released by the infected person’s cough or sneeze.
Medical reports have demonstrated that the initial symptoms of the virus include coughing, high fever, fatigue, sore throat, body aches, breathlessness, and severe weakness. If the patient is not treated on time, then the condition may get worsened to severe respiratory disorders, multi-organ dysfunctioning, and even death. Infected people are mostly found to be symptomatic, but certain asymptomatic patients have also been reported (WHO, Citation2020a). The asymptomatic COVID-19 patient acts as a carrier from infected to healthy humans (Rothe et al., Citation2020). The asymptomatic patients have a lower viral load in their nasal cavity than symptomatic patients (WHO, Citation2020b; Zou et al., Citation2020) has advised that the only way to stay safe from this deadly coronavirus is to keep a distance from everyone, wear surgical masks, sanitize your hands, and avoid touching public surfaces as the virus may remain viable for days, over any surface if environmental conditions are optimum for it. However, different disinfectants have been found effective against the virus, including hydrogen peroxide and hypochlorite as mentioned by Kampf, Todt, Pfaender, and Steinmann (Citation2020).
Studies like the one conducted by Jordan, Adab, and Cheng (Citation2020) to estimate the COVID-19 cases reported in the hospitals have shown that the death rate of COVID-19 patients is higher among the elderly population and those with weaker immune systems. As it is a new phenomenon, many studies have been carried out recently not only to study the properties of coronavirus but also to study the rate of transfer of virus, the survival time and length of stay of the covid patients, etc. Nemati, Ansary, and Nemati (Citation2020) studied the discharge time of COVID-19 patients, and using a survival model, predict the length of stay of patients in a hospital. Levy et al. (Citation2020) predicted the survival of hospitalized COVID-19 patients through clinical characteristics and mortality risk factors. (Car, Baressi Šegota, Anđelić, Lorencin, & Mrzljak, Citation2020) modeled the spread of COVID-19 disease using machine learning solutions that are multilayer perceptron and artificial neural network techniques. Niazkar, Eryılmaz Türkkan, Niazkar, and Türkkan (Citation2020) applied the three predictive models to forecast the outbreak of COVID-19 in Iran and Turkey. Zuo, Khosa, Ahmad, and Almaspoor (Citation2020) proposed a new flexible extended-X (NFE-X) family of distribution, and one of the members of this family, NFE-Weibull distribution, was used to model the total deaths of COVID-19 patients belonging to Asian countries. Tang and Wang (Citation2020) developed a mathematical modeling approach (5 days moving average) for a daily growth rate of COVID-19 cases in the United States. Nesteruk (Citation2020) predicted the number of Coronavirus victims using mathematical modeling. Din, Li, Khan, and Zaman (Citation2020) proposed a model that gives better constraints on understanding the climaxes of coronavirus spread. Almongy, Almetwally, Aljohani, Alghamdi, and Hafez (Citation2021) analyzed the mortality rate of COVID-19 patients using extended odd Weibull Rayleigh (EOWR) distribution. Haj Ismail, Dawi, Jwaid, Mahmoud, and AbdelKader (Citation2021) studied the spread of COVID-19 in the United Arabs Emirates. AlSayegh and Iqbal (Citation2021) investigated the effect of precautionary and preventive measures on the limited spread of coronavirus in Bahrain. Bakar et al. (Citation2021) developed the lock-down model in ASEAN countries by using hybrid ARIMA-SVR and hybrid SEIR-ANN.
The Fréchet distribution proposed by Fréchet (Citation1927) is one of the important extreme value distributions which has found wide applications in natural phenomena like earthquakes, floods, sea waves, rainfall, etc. However, for survival analysis, it is one of the flexible distributions. Krishna, Jose, Alice, and Ristić (Citation2013) introduced a new Marshall–Olkin Fréchet distribution and after studying its statistical properties, applied it to a real-life data set about the survival times of pigs. Shafiq et al. (Citation2021) purposed a modified Kies-Fréchet distribution to examine and model the COVID-19 mortality rates in Canada and Netherland. Abbas et al. (Citation2019) used the three-parameter Fréchet distribution for the analysis of survival times of a group of patients suffering from head and neck cancer. Kutal and Qian (Citation2018) studied a long-term survival non-mixture model of the Fréchet distribution and proved that it is the best for modeling the data on allogeneic marrow transplantation patients. Yousof, Altun, and Hamedani (Citation2018) derived the odd log-logistic Fréchet distribution and the heart transplant data set is used for its application. Korkmaz, Yousof, and Ali (Citation2017) proposed the odd Lindley-Fréchet distribution and used it to model the real-life data set of the survival time (in weeks) of acute Myelogenous Leukaemia patients.
The Bayesian statistics update the person’s belief in the evidence of new data. The Bayesian workflow is based on three steps; the first one is the prior distribution which provides the prior knowledge about parameters. The second is the likelihood function, which is the information about the parameters provided in the available data and the third one is the posterior distribution of the parameters, which is formulated via the Bayes theorem by combining the prior distribution with the likelihood function. In the last few years, the Bayesian statistics has found its applications in nearly every field of life because of its novel nature. Many statisticians like Bherwani et al. (Citation2021), De Oliveira et al. (Citation2020), and Manevski et al. (Citation2020), etc., have conducted the Bayesian study for the research and analysis of COVID-19 patients.
The main objective of this study is to model the recovery time of COVID-19 patients using a suitable statistical distribution and to propose an efficient estimation technique to attain estimators of the parameters of this distribution. The best estimators are selected so that worldwide health practitioners, researchers, and epidemiologists could use these estimators for the analysis and better prediction of the recovery time of COVID-19 patients.
As mentioned above, the Fréchet distribution has played an important role in survival analysis. This and many other life failure distributions have been successfully utilized to model and analyze the survival and reliability data. In this study, we have proposed a new Fréchet-exponential (FE) distribution using the Transformed-Transformation technique by Alzaatreh, Lee, and Famoye (Citation2013) and modeled the recovery time of a sample of COVID-19 patients. This model is analyzed and estimated using maximum likelihood and the Bayesian estimation techniques. The expressions of the Bayes estimators of the parameters of the FE distribution are not attained in closed-form. Hence, the Lindley and Tierney–Kadane (T-K) approximation methods are used. For illustrative purposes, the data set of 117 COVID-19 patients is taken from the Nishtar hospital Multan, Pakistan, and the recovery time (duration from the time of a patient’s admission in a hospital to the time of discharge) is recorded. It is shown mathematically and graphically that our proposed model adequately fits the data set. The performance of the Bayes estimators is observed to be better than the MLEs. The rest of the paper is organized as follows.
In Sec. 2, the FE distribution is derived. The survival, hazard rate, and cumulative hazard rate functions are presented here. In Sec. 3, the MLEs of the parameters of the distribution are estimated. In Sec. 4, the Lindley and T-K methods are utilized for the Bayes estimators using informative prior under five loss functions. Sections 5 and 6 are about the simulation study and real-life examples of the survival time of COVID-19 patients. Finally, the study is concluded in Sec. 7.
2. The Fréchet-exponential distribution
Because of the importance of Fréchet distribution in the survival and reliability analysis, a new and more flexible FE distribution has been suggested, in this study. This distribution is derived using one of the transformed-transformer techniques given by Alzaatreh et al. (Citation2013) and is used to model and analyze the data consisting of the recovery times of COVID-19 patients.
Alzaatreh et al. (Citation2013) proposed the transformed-transformer (T-X family of distribution) technique, in which the PDF ( of a continuous rv T is transformed using the transformer
which is a specific functional form of the CDF
of another rv X. This transformer is differentiable and monotonically non-decreasing function and its functional form depends upon the support of the rv T. Alzaatreh et al. (Citation2013) also defined the T-X family for various transformers under different supports of rv T.
In our study, the rvs T and X follow Fréchet and exponential distributions, respectively. One of the transformers given by Alzaatreh et al. (Citation2013) is defined as which is used to transform the Fréchet distribution. The support of the rv T is [
The PDF and CDF of the transformed FE distribution are:
(1)
(1)
(2)
(2)
where
and
are the shape and inverse shape parameters of the Fréchet and exponential distributions, respectively. The shape of the distribution depends upon the value of the parameters. shows the PDF and CDF plots of the distribution, and it shows that the distribution is unimodal and positively skewed.
2.1. Survival analysis
In survival analysis, we analyze the data that involves the time duration until an event occurs. The survival time is the duration of time from a predefined point to the occurrence of an event of interest. Similarly, the hazard function gives the rate of failure of a patient, component, or system given that before time t, the failure has not occurred. We have obtained the survival, hazard, and cumulative hazard functions of the FE distribution. In our case, the survival time will represent the recovery time (i.e. the duration of time between the COVID-19 patient’s admission in the hospital and his discharge after recovery). Let a non-negative rv T representing survival time follow the FE distribution. Then the survival function is the probability of surviving beyond the time t is given as:
Hazard function which is the ratio of the probability density function and the survival function is given as:
The expected failure time known as cumulative hazard function of FE distribution is:
shows the survival, hazard, and cumulative hazard function plots for different values of parameters. The chance of survival decreases by increasing the time. The hazard rate is high for time between 5 and 15, after that time it seems to be constant.
To estimate the parameters of the distribution in frequentist and Bayesian paradigms, the method of maximum likelihood and Bayesian estimation techniques are used which are elaborated in the further sections.
3. Maximum likelihood estimators
Let be a random sample from the FE distribution. Then the likelihood and log-likelihood function of the distribution are;
(3)
(3)
(4)
(4)
The maximum likelihood estimators (MLEs) and
of the parameters of FE distribution are obtained by differentiating the log likelihood function with respect to its parameters, the two normal equations obtained cann’t solve simultaneously. The iterative method is one of the solutions, hence Newton Raphson’s iterative procedure is used for the estimation of MLEs.
4. Bayes estimators using informative prior
In Bayesian statistics, the personal belief about the unknown parameters of the model of interest is quantified in the form of a prior distribution. Then the sample data and prior distribution are combined to form the posterior distribution. This posterior distribution contains all the probabilistic information about the parameters. The informative prior provide specific and valuable information about the parameters as compared to the deviance of data obtained from the experiment.
In this study, the appropriate prior distributions for the parameters and
are taken to be exponential and Weibull distributions, respectively (depending upon the support of the parameters). Considering the independence of prior, the joint prior distribution of
and
is:
(5)
(5)
where
are the hyperparameters. The joint posterior distribution of the parameters of FE distribution is obtained by combining the likelihood function and the joint prior distribution given in EquationEqs. (3)
(3)
(3) and Equation(5)
(5)
(5) , through the Bayes theorem, that is:
(6)
(6)
The inferential procedures of the parameters and
are based on the marginal distributions of the parameters. These marginal posterior distributions of the parameters are not in the closed-form expression.
The posterior risk is the expectation of loss function. The Bayes estimators are the minimizers of these risks. The Bayes estimators and associated posterior risks of the parameters and
are evaluated under the square error loss function (SELF), weighted loss function (WLF), quadratic loss function (QLF), precautionary loss function (PLF), and modified II loss function (MIILF). The Bayes estimators and associated posterior risks for the functional forms of the parameters
are defined as:
(7)
(7)
(8)
(8)
The expression of the Bayes estimators obtained from EquationEq. (8)(8)
(8) is not in a closed-form. Hence two approximation techniques, Lindley and T-K are used to derive the Bayes estimators of the parameters of the FE distribution under the loss functions mentioned above.
4.1. Bayes estimators and posterior risks using Lindley approximation
Lindley (Citation1980) provided an asymptotic solution of the ratio of two integrals which gives a single approximate result. This technique is widely used for estimation in Bayesian analysis. Lavanya and Alexander (Citation2016) used this technique to estimate the Constant Shape-Bi Weibull distribution based on failure time data. Sharma, Singh, and Singh (Citation2017) estimated all the parameters of the power Lindley distribution through this technique. Adnan (Citation2021) obtained the Bayes estimators of the scale and shape parameters of the Weibull-Lindley Rayleigh distribution using this method.
EquationEquation (7)(7)
(7) can be written as:
(9)
(9)
The functions are the log-likelihood function and log of a prior distribution, respectively. The expression Equation(9)
(9)
(9) using Lindley approximation is evaluated as:
(10)
(10)
where
is the ijth element of the var-covariance matrix of the parameters of FE distribution.
Also;
The Bayes estimators of under SELF, WLF, QLF, PLF, and MIILF, using expression Equation(10)
(10)
(10) are:
The corresponding posterior risks are obtained to be:
Similarly, the Bayes estimators and associated posterior risk of parameter are evaluated.
4.2. Bayes estimators and posterior risks using T-K approximation
Tierney and Kadane (Citation1986) applied the Laplace method for the approximate evaluation of the ratio of two integrals. This technique required the evaluation of the second derivative of the log-likelihood function, while the third derivative of the log-likelihood function is required for Lindley approximation. This technique is widely used in literature; Gencer and Gencer (Citation2019, Citation2020), Sana and Faizan (Citation2019), and Tanis and Saracoglu (Citation2019), etc., applied this technique in their studies.
For the FE distribution;
The expression for the Bayes estimators and associated posterior risk provided in EquationEq. (9)(9)
(9) can be expressed using T-K approximation, as:
(11)
(11)
where
and
maximize the
and
and
and
is the inverse of the Fisher information matrix of
and
at
and
respectively.
All the Bayes estimators and associated posterior risks of the parameters and
under SELF, WLF, QLF, PLF, and MIILF are evaluated using expression Equation(11)
(11)
(11) .
5. Simulation study
In this section, the Monte Carlo Simulation scheme is used to study the performance of the FE distribution both in the classical and Bayesian paradigms. These classical and Bayesian estimators under different loss functions are compared for different sample sizes. For this purpose, the random samples of 30, 50, 100, 200, 300, 500, 700, 1000, and 1500 observations are drawn from the FE distribution through the random number generator U is a uniform random variate over the interval (0,1). For the MLEs of the parameters of the distribution, the Newton-Raphson iterative process is used because the two normal equations obtained from the method of maximum likelihood cannot be solved simultaneous. The R package maxLik is used for this purpose. The Bayes estimators are evaluated using the theoretical results of the Lindley and T-K approximation methods given above. The informative priors (exponential prior for
and Weibull prior for
) under the SELF, WLF, QLF, PLF, and MIILF are used for the evaluation of Bayes estimators and associated posterior risks. The values of the parameters are taken as
and
The elicited values of the hyperparameters are
All the computation is done by making programming routines in the R language. The simulation size is taken to be 1000. The efficiency of the estimators is assessed on the basis of minimum values of the associated posterior risks. The results of the simulation study are shown in and . Here the bold font is used for the minimum risk values for each sample size.
Table 1. The MLEs and Bayes estimators of with values of risks under defined loss functions.
Table 2. The MLEs and Bayes estimators of with values of risks under defined loss functions.
From and , it is evident that the performance of the Bayes estimators, for all the sample sizes is better than the MLEs as can be seen from the values of the loss functions. When the sample size increases, the values of risks decrease and approach zero. For the parameter the estimators obtained through the T-K approximations method are better than the Lindley method with minimum risks and SELF proves to be better than the other loss functions. For parameter
the Bayes estimators under the Lindley method are better than the T-K method and WLF can be considered the best loss function as it gives the least posterior risks.
6. Analysis of recovery time for the COVID patients
In this section, the suitability of the FE model fitted to the data set of the recovery time of COVID-19 patients is studied. For this purpose, 117 patients are taken from the Nishtar hospital Multan, Pakistan. The data set consists of the recovery time of the COVID-19 patients admitted to the hospital and discharged after recovery. provides the summary statistics of the data set.
Table 3. Summary statistics of recovery time (days) of the COVID-19 patients.
For the 117 patients, the mean ( standard deviation) age of the patients is calculated to be 38 (
15.913 years), and the average duration of the patients’ stay from the time of admission in a hospital to their time of discharge after recovery is reported to be 15.1795 (
12.825 days).
The empirical and cumulative distribution of the data set and the goodness of fit curve of the FE distribution is shown in . It is evident that the data about the recovery time of COVID-19 patients adequately fit the FE distribution.
Figure 3. (a) Empirical and cumulative distribution of the data set. (b) The goodness of fit curve of the FE distribution with the data set.

In , the reliability plots of the FE distribution for the COVID-19 patient’s data set are displayed. The hazard plot provided in , shows that the hazard rate of COVID patients is very high in the early period after being admitted to the hospital, then level off and the hazard of death decreases over time. Hence, in the early period, the likelihood of failure is greater.
demonstrates the comparison of the flexibility of FE distribution using the data of COVID-19 patients with the following distributions:
Table 4. MLE, log-likelihood, AIC, BIC, CAIC, and HQIC of the distributions based on data set.
Exponential distribution with pdf,
Rayleigh distribution by Rayleigh (Citation1880) with pdf,
Inverse Rayleigh by Vodˇa (Citation1972) with pdf,
x,
Fréchet distribution by Fréchet (Citation1927), with pdf,
Gompertz Fréchet by Oguntunde, Khaleel, Ahmed, and Okagbue (Citation2019) with pdf,
Weibull Fréchet by Afify, Yousof, Cordeiro, Ortega, and Nofal (Citation2016) with pdf,
We have compared the distributions on the basis of maximized log-likelihood, Akaike information criterion (AIC), Bayesian information criterion (BIC), corrected Akaike information criterion (CAIC), and Hannan-Quinn information criterion (HQIC). The distribution having smaller values of these criteria for the data set is considered to be better.
is about the goodness of the curve of the FE distribution with the competitive distributions for the data set. It is depicted that the FE model fits the data appropriately and turns out to be more flexible than its competitors.
For the data set of the survival times of the COVID-19 patients, the MLEs of the parameters and
of the distribution are not in the closed-form, hence are evaluated using the Newton-Raphson method. On the other hand, the Bayes estimates of the parameters using informative prior (exponential prior for
and the Weibull prior for
are evaluated using the Lindley and T-K approximation methods. These MLEs and Bayes estimates are compared on the basis of minimum values of associated risks. The results are shown in .
Table 5. The MLEs and Bayes estimators with associated risks of and
for COVID-19 data.
The results of show that the Bayes estimators of the parameters of the FE distribution perform better than the MLEs, as they have minimum values of posterior risks. For parameter the estimators under the T-K method using SELF prove to be better than other Bayes estimators. The estimators of
under the Lindley method using SELF are better with minimum risks for the given data set.
shows the mean and standard deviation (sd) of the data set and those obtained from FE distribution via MLEs and the Bayes estimators using Lindley and T-K methods. The mean and sd obtained from data set and from the FE distribution are approximately closer to each other, which is evident that the FE distribution is suitable for the data set.
Table 6. The mean and standard deviation of the data and MLEs and Bayes estimates.
7. Concluding remarks
In this study, a univariate statistical distribution is proposed to model the time to recover of a sample of 117 COVID-19 patients. The data set is taken from the Nishtar hospital Multan, Pakistan. The parameters are estimated in the classical and Bayesian paradigms. The average time duration of the patients from the time of their admission in the hospital to discharge after recovery is obtained to be 14 to 15 days. The Bayes estimators are evaluated with informative prior using the Lindley and T-K approximation methods. The results of the simulation study and real-life data set show that the Bayes estimators prove to be more efficient than the MLEs with minimum values of risks. The FE distribution turns out to be a better fit than the standard distribution for the given data set. It is hoped that health practitioners and medical researchers may use the proposed probability distribution to model different medical and health phenomena. In addition to this, Bayesian estimation has proved to be better for parameter estimation than classical methods, especially in medical and health sciences. This study can also be extended by using the censored data as well.
Abbreviations | ||
rv(s) | = | Random variable(s) |
AIC | = | Akaike information criterion |
BIC | = | Bayesian information criterion |
CAIC | = | CorrectedAkaike information criterion |
FE | = | Fréchet-exponential |
T-K | = | Tierney–Kadane |
T-X Method | = | transformed-transformer Method |
S(t) | = | Survival Function |
h(t) | = | Hazard function |
H(t) | = | Cumulative Hazard Function |
= | Probability Density Function | |
CDF | = | Cumulated distribution Function |
MLE | = | Maxium Likelihood estimators |
SELF | = | Square error Loss Function |
WLF | = | Weighted loss Function |
MIILF | = | Modified loss Function |
Disclosure statement
No potential conflict of interest was reported by the authors.
References
- Abbas, K., Abbasi, N. Y., Ali, A., Khan, S. A., Manzoor, S., Khalil, A., … Altaf, M. (2019). Bayesian analysis of three-parameter Frechet distribution with medical applications. Computational and Mathematical Methods in Medicine, 2019, 9089856. doi:10.1155/2019/9089856
- Adnan, H. (2021). Bayes estimators of Weibull-Lindley Rayleigh distribution parameters using Lindley’s approximation. Turkish Journal of Computer and Mathematics Education (TURCOMAT), 12(12), 508–517.
- Afify, A. Z., Yousof, H. M., Cordeiro, G. M., Ortega, E. M., & Nofal, Z. M. (2016). The Weibull Fréchet distribution and its applications. Journal of Applied Statistics, 43(14), 2608–2626. doi:10.1080/02664763.2016.1142945
- Almongy, H. M., Almetwally, E. M., Aljohani, H. M., Alghamdi, A. S., & Hafez, E. (2021). A new extended Rayleigh distribution with applications of COVID-19 data. Results in Physics, 23, 104012. doi:10.1016/j.rinp.2021.104012
- AlSayegh, M. A. K., & Iqbal, A. (2021). The impact of the precautionary measures taken in the kingdom of Bahrain to contain the outbreak of COVID-19. Arab Journal of Basic and Applied Sciences, 28(1), 195–203. doi:10.1080/25765299.2021.1903143
- Alzaatreh, A., Lee, C., & Famoye, F. (2013). A new method for generating families of continuous distributions. METRON, 71(1), 63–79. doi:10.1007/s40300-013-0007-y
- Bakar, M. A., Mohamed, N., Pratama, D. A., Yusran, M. F. A., Aleng, N. A., Yanuar, Z., & Niken, L. (2021). Modelling lock-down strictness for COVID-19 pandemic in ASEAN countries by using hybrid ARIMA-SVR and hybrid SEIR-ANN. Arab Journal of Basic and Applied Sciences, 28(1), 204–224. doi:10.1080/25765299.2021.1902606
- Bherwani, H., Anjum, S., Kumar, S., Gautam, S., Gupta, A., Kumbhare, H., … Kumar, R. (2021). Understanding COVID-19 transmission through Bayesian probabilistic modeling and GIS-based Voronoi approach: A policy perspective. Environment, Development and Sustainability, 23(4), 5846–5864. doi:10.1007/s10668-020-00849-0
- Car, Z., Baressi Šegota, S., Anđelić, N., Lorencin, I., & Mrzljak, V. (2020). Modeling the spread of COVID-19 infection using a multilayer perceptron. Computational and Mathematical Methods in Medicine, 2020, 5714714. doi:10.1155/2020/5714714
- De Oliveira, A. C. S., Morita, L. H. M., Da Silva, E. B., Zardo, L. A. R., Fontes, C. J. F., & Granzotto, D. C. T. (2020). Bayesian modeling of COVID-19 cases with a correction to account for under-reported cases. Infectious Disease Modelling, 5, 699–713. doi:10.1016/j.idm.2020.09.005
- Din, A., Li, Y., Khan, T., & Zaman, G. (2020). Mathematical analysis of spread and control of the novel corona virus (COVID-19) in China. Chaos, Solitons, and Fractals, 141, 110286. doi:10.1016/j.chaos.2020.110286
- Fréchet, M. (1927). Sur la loi de probabilité de l‘écart maximum. Annales Societatis Mathematicae Polonae, 6, 93–116. doi:10.11429/sugaku1947.46.39
- Gencer, G., & Gencer, K. (2019). Comparison of maximum likelihood and Bayes estimators under symmetric and asymmetric loss functions by means of Tierney Kadane’s approximation for Weibull distribution. Turkish Journal of Science and Technology, 14(2), 69–78.
- Gencer, G., & Gencer, K. (2020). Estimations for the odd Weibull distribution under progressive type-II right censored samples. Sakarya Üniversitesi Fen Bilimleri Enstitüsü Dergisi, 24(1), 29–36.
- Haj Ismail, A., Dawi, E., Jwaid, T., Mahmoud, S. T., & AbdelKader, A. (2021). Simulation of the evolution of the Covid-19 pandemic in the United Arab Emirates using the sir epidemical model. Arab Journal of Basic and Applied Sciences, 28(1), 128–134. doi:10.1080/25765299.2021.1890900
- Jordan, R. E., Adab, P., & Cheng, K. (2020). Covid-19: Risk factors for severe disease and death. British Medical Journal, 368, m1198. doi:10.1136/bmj.m1198
- Kampf, G., Todt, D., Pfaender, S., & Steinmann, E. (2020). Persistence of coronaviruses on inanimate surfaces and their inactivation with biocidal agents. The Journal of Hospital Infection, 104(3), 246–251. doi:10.1016/j.jhin.2020.01.022
- Korkmaz, M. Ç., Yousof, H. M., & Ali, M. M. (2017). Some theoretical and computational aspects of the odd Lindley Fréchet distribution. İstatistikçiler Dergisi: İstatistik ve Aktüerya, 10(2), 129–140.
- Krishna, E., Jose, K., Alice, T., & Ristić, M. M. (2013). The Marshall-Olkin Fréchet distribution. Communications in Statistics - Theory and Methods, 42(22), 4091–4107. doi:10.1080/03610926.2011.648785
- Kutal, D. H., & Qian, L. (2018). A non-mixture cure model for right-censored data with Fréchet distribution. Stats, 1(1), 176–188. 10.3390/stats1010013.
- Lavanya, A., & Alexander, T. L. (2016). Estimation of parameters using Lindley’s method. International Journal of Advanced Research, 4(12), 1767–1778. doi:10.21474/IJAR01/2579
- Levy, T. J., Richardson, S., Coppa, K., Barnaby, D. P., McGinn, T., … Becker, L. B, the Northwell COVID-19 Research Consortium. (2020). Estimating survival of hospitalized COVID-19 patients from admission information. medRxiv, 1–29.
- Lindley, D. V. (1980). Approximate Bayesian methods. Trabajos de Estadistica Y de Investigacion Operativa, 31(1), 223–245. doi:10.1007/BF02888353
- Lutchmansingh, D. D., Knauert, M. P., Antin-Ozerkis, D. E., Chupp, G., Cohn, L., Dela Cruz, C. S., … Possick, J. D. (2021). A clinic blueprint for post-COVID-19 RECOVERY: Learning from the past, looking to the future. Chest, 159(3), 949–958. doi:10.1016/j.chest.2020.10.067
- Manevski, D., Ružić Gorenjec, N., Kejžar, N., & Blagus, R. (2020). Modeling COVID-19 pandemic using Bayesian analysis with application to Slovene data. Mathematical Biosciences, 329, 108466. doi:10.1016/j.mbs.2020.108466
- Nemati, M., Ansary, J., & Nemati, N. (2020). Machine-learning approaches in COVID-19 survival analysis and discharge-time likelihood prediction using clinical data. Patterns (New York, N.Y.), 1(5), 100074. doi:10.1016/j.patter.2020.100074
- Nesteruk, I. (2020). Statistics-based predictions of coronavirus epidemic spreading in mainland China. doi:10.1101/2020.02.12.20021931
- Niazkar, M., Eryılmaz Türkkan, G., Niazkar, H. R., & Türkkan, Y. A. (2020). Assessment of three mathematical prediction models for forecasting the COVID-19 outbreak in Iran and Turkey. Computational and Mathematical Methods in Medicine, 2020, 7056285. doi:10.1155/2020/7056285
- Oguntunde, P. E., Khaleel, M. A., Ahmed, M. T., & Okagbue, H. I. (2019). The Gompertz Fréchet distribution: Properties and applications. Cogent Mathematics & Statistics, 6(1), 1568662. doi:10.1080/25742558.2019.1568662
- Rayleigh, L. (1880). XII. On the resultant of a large number of vibrations of the same pitch and of arbitrary phase. The London, Edinburgh, and Dublin Philosophical Magazine and Journal of Science, 10(60), 73–78. doi:10.1080/14786448008626893
- Rothe, C., Schunk, M., Sothmann, P., Bretzel, G., Froeschl, G., Wallrauch, C., … Hoelscher, M. (2020). Transmission of 2019-nCoV infection from an asymptomatic contact in Germany. The New England Journal of Medicine, 382(10), 970–971. doi:10.1056/NEJMc2001468
- Sana, M., & Faizan, M. (2019). Bayesian estimation for Nadarajah-Haghighi distribution based on upper record values. Pakistan Journal of Statistics and Operation Research, 15(1), 217–230. doi:10.18187/pjsor.v15i1.2569
- Shafiq, A., Lone, S., Sindhu, T. N., El Khatib, Y., Al-Mdallal, Q. M., & Muhammad, T. (2021). A new modified Kies Fréchet distribution: Applications of mortality rate of Covid-19. Results in Physics, 28, 104638. doi:10.1016/j.rinp.2021.104638
- Sharma, V. K., Singh, S. K., & Singh, U. (2017). Classical and Bayesian methods of estimation for power Lindley distribution with application to waiting time data. Communications for Statistical Applications and Methods, 24(3), 193–209. doi:10.5351/CSAM.2017.24.3.193
- Tang, Y., & Wang, S. (2020). Mathematic modeling of COVID-19 in the United States. Emerging Microbes & Infections, 9(1), 827–829. doi:10.1080/22221751.2020.1760146
- Tanis, C., & Saracoglu, B. (2019). Comparisons of six different estimation methods for log-Kumaraswamy distribution. Thermal Science, 23(Suppl. 6), 1839–1847. doi:10.2298/TSCI190411344T
- Tierney, L., & Kadane, J. B. (1986). Accurate approximations for posterior moments and marginal densities. Journal of the American Statistical Association, 81(393), 82–86. doi:10.1080/01621459.1986.10478240
- Vodˇa, V. G. (1972). On the inverse Rayleigh distributed random variable. Reports of Statistical Application Research JUSE, 19(4), 13–21.
- WHO. (2020a). Coronavirus disease 2019 (COVID-19), Situation Report – 51. WHO.
- WHO. (2020b). Coronavirus disease 2019 (COVID-19), Situation Report – 53. WHO
- Yousof, H. M., Altun, E., & Hamedani, G. (2018). A new extension of Frechet distribution with regression models, residual analysis and characterizations. Journal of Data Science, 16(4), 743–770. doi:10.6339/JDS.201810_16(4).00005
- Zhu, N., Zhang, D., Wang, W., Li, X., Yang, B., Song, J., … Tan, W. (2020). A novel coronavirus from patients with pneumonia in China, 2019. New England Journal of Medicine, 382(8), 727–733. doi:10.1056/NEJMoa2001017
- Zou, L., Ruan, F., Huang, M., Liang, L., Huang, H., Hong, Z., … Wu, J. (2020). SARS-CoV-2 viral load in upper respiratory specimens of infected patients. The New England Journal of Medicine, 382(12), 1177–1179. doi:10.1056/NEJMc2001737
- Zuo, M., Khosa, S. K., Ahmad, Z., & Almaspoor, Z. (2020). Comparison of COVID-19 pandemic dynamics in Asian countries with statistical modeling. Computational and Mathematical Methods in Medicine, 2020, 4296806. doi:10.1155/2020/4296806