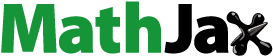
Abstract
In this study we have proposed the SIAQR model with time-dependent infection rate. The model’s well-posedness is demonstrated, and the proposed model is then extended to the fractional mathematical model by employing the Caputo-Fabrizio fractional derivative operator. We have also used the Lipschitz condition and the linear growth condition to determine the conditions under which the model has a unique solution. The numerical solutions are presented. Furthermore, using graphical representations we will see how the number of infected, recovered individuals vary as the order of fractional derivative varies.
2010 Mathematics Subject Classification:
1. Introduction
As we know that mathematical models are capable of decision-making, saving lives, assisting in policy, and many more. These are helpful in understanding the conditions needed to sustain lives and provide us with ways to study and predict the behavior of the spread. The concept of derivatives and integrals plays a lot in the formulation of these mathematical models. In this work, we will be concerned with the SIAQR mathematical model. A SIAQR model is an epidemiological model that determines the number of people infected with a transmissible infection in a closed population over a period of time. This class of model derives from the fact that they involve equations relating the number of susceptible people S(t), the number of people infected symptomatically I(t), the number of people who are asymptomatically infected A(t), the number of people who have been quarantined Q(t), and who have recovered R(t). Recently the concept of fractional derivatives (Kilbas et al., Citation2006; Miller and Ross, Citation1993; Oldham and Spanier, Citation1974) and integrals are proved to be very useful in making a better understanding of real-world problems which exhibit non-local behaviors. The first and most widely accepted definition of the singular kernel was proposed by Riemann-Liouville, later on, many new developments were made in the field of fractional calculus which involves the definition of the fractional derivative with the non-singular kernel, one of which was given by Caputo. This definition is based on the concept of the power law. The literature is full-fledged with many definitions of fractional derivatives and integrals. Applications can be seen in the literature (Ahmad et al., Citation2021; Ain & He, Citation2019; Ain et al., Citation2021, Citation2022a, Citation2022b, Citation2022c; Alkahtani & Alzaid, Citation2020, Citation2021; Alshomrani et al., Citation2021; Al-Smadi et al., Citation2021; Anjum et al., Citation2021a, Citation2021b; Atangana & Aguilar, Citation2018; Atangana & Araz, Citation2021; Atangana & Atangana, Citation2020; Djeddi et al., Citation2020; Hasan et al., Citation2022; Jarad et al., Citation2018; Karaji & Nyamoradi, Citation2020; Khan & Atangana, Citation2020; Modi et al., Citation2021; Momani et al., Citation2021; Naik et al., Citation2020, Citation2021, Citation2022; Ucar et al., Citation2019). In this article, we consider the SIAQR model with time-dependent infection rate. An analysis of this SIAQR model is presented in detail. Using the Caputo definition of fractional derivative, we will study the fractional SIAQR model. In Section 1, an introduction along with some definitions of the fractional derivatives are given. In Section 2, we have presented the SIAQR model with a time-dependent infection rate. In Section 3, we have presented the existence and the uniqueness of the system of solution of the SIAQR model. In Section 4, the SIAQR model with time-dependent infection rate in sense of the Caputo fractional derivative operator is given and numerical simulations are performed for a better understanding of the model.
Following are some definitions of fractional derivatives and integrals.
Definition 1.
For an integrable function f on the Riemann-Liouville fractional integral of order γ is given as
(1.1)
(1.1)
where
denotes the Riemann-Liouville fractional integral of order γ (Miller and Ross, Citation1993).
Definition 2.
For an integrable function f on the Riemann-Liouville fractional derivative of order γ is given as
(1.2)
(1.2)
where
denotes the Riemann-Liouville fractional derivative of order γ (Miller and Ross, Citation1993).
Definition 3.
For an integrable function f on the Caputo fractional derivative of order γ is given as
(1.3)
(1.3)
where
denotes the Caputo fractional derivative of order γ (Miller and Ross, Citation1993).
Theorem 1.
Assuming that there exists positive constants L and such that the following holds
Lipschitz condition:
and
(1.4)
(1.4)
Linear growth condition:
(1.5)
(1.5)
Then the Cauchy problem with Caputo derivative admits a unique solution (Atangana, Citation2020).
2. SIAQR model with time dependent infection rate
In the SIAQR model, we have considered the five classes of individuals as S: Susceptible group of individuals I: Infected symptomatic group of individuals A: Asymptomatically infected group of individuals, Q: Quarantined group of individuals and R: Recovered group of individuals. The following assumptions are made in this model:
We assume that the total population N(t) does not change much with time i.e.
There is the interaction among every person.
The infected symptomatically and asymptomatically infected group of individuals are transmitting the infection to a susceptible group of individuals.
It is also assumed that after recovery the infected individuals develop immunity and eventually the recovered individuals are prone to lose their immunity and return to the susceptible class of individuals after a period of time.
Further, a time dependence on the parameter β1 and β2, the transmission rate of infection, is introduced in this model, so the effect of transmission will be given as
and
This choice of decreasing exponential function is justified by step by step implementation of rules and by a rise in consciousness and awareness in individuals. Here, β1 and β2 is the infection rate at the beginning of the infection and z determines the change in the time of infection (Iannni & Rossi, Citation2020).
Keeping in mind the aforementioned assumptions the SIAQR model is formulated as:
where, δ defines the birth and natural death rate,
represents the recovery rates for infected symptomatically, asymptomatically infected, and quarantined groups of individuals respectively, q is the rate by which infected people are quarantined. β1 and β2 are the infection rate for the individuals infected symptomatic and asymptomatically. Further, a time dependence on the infection rate β1 and β2 is introduced with an exponential decay behaviour
where, z is the rate of decrease.
3. SIAQR model with time-dependent infection rate in sense of Caputo fractional derivative operator
We now extend the above formulated SIAQR model to the fractional SIAQR model in sense of Caputo fractional derivative, which is given as follows:
(3.1)
(3.1)
Here denotes the Caputo fractional derivative of order
Theorem 2.
Assuming that all the parameters defined in the above formulated model are positive, the solution of the model with non-negative initial conditions are positive
Proof.
We consider the first equation
(3.2)
(3.2)
On solving, we get where C1 is constant of integration.
Using the fact that we get
(3.3)
(3.3)
In a similar way, we consider the second equation
(3.4)
(3.4)
On solving, we get where C2 is constant of integration.
Using the fact that we get
(3.5)
(3.5)
On a similar account, we get
(3.6)
(3.6)
(3.7)
(3.7)
(3.8)
(3.8)
□
4. Existence and uniqueness of the fractional SIAQR model
In this section we will prove the existence and the uniqueness for the SIAQR model. For convenience, we write the SIAQR model as
(4.1)
(4.1)
where
(4.2)
(4.2)
To prove the existence and the uniqueness of the solution of the SIAQR model, we use the concept which was recently proposed by Atangana (Citation2020) and hence prove the following theorem.
Theorem 3.
Assuming that there exists positive constants and
such that the following holds
(4.3)
(4.3)
(4.4)
(4.4)
Proof.
Consider
(4.5)
(4.5)
Define the norm as we get
(4.6)
(4.6)
Taking we have
(4.7)
(4.7)
Next consider
(4.8)
(4.8)
Again defining the norm as we get
(4.9)
(4.9)
Take finally we get
(4.10)
(4.10)
Next consider,
(4.11)
(4.11)
On a similar account, we get
(4.12)
(4.12)
where
Similarly, we can show
(4.13)
(4.13)
and
(4.14)
(4.14)
We now prove the second part of the above stated theorem. We first show Consider
(4.15)
(4.15)
On taking and under the condition
we get
(4.16)
(4.16)
We now show
(4.17)
(4.17)
Consider
(4.18)
(4.18)
On taking and under the condition
We get
Next to show
Consider
(4.19)
(4.19)
On taking and under the condition
We get
Next to show
Consider
(4.20)
(4.20)
On taking and under the condition
We get
Lastly we show
Consider
(4.21)
(4.21)
On taking and under the condition
We get
Hence, by using Theorem 1, the existence and uniqueness of the SIAQR model is proved.
5. Derivation of numerical scheme for Caputo fractional SIAQR model using Atangana-Seda method
5.1. Atangana-Seda method with classical derivative
Consider the following Cauchy problem (Atangana & Araz, Citation2020).
(5.1)
(5.1)
where g is a non-linear function.
To provide a numerical scheme to solve the above mentioned equation, we convert the above equation as
(5.2)
(5.2)
At point we have
(5.3)
(5.3)
At point we have
(5.4)
(5.4)
On considering the difference of above two equations, we have
(5.5)
(5.5)
The approximation of as the Newton polynomial is given as
(5.6)
(5.6)
Substituting the approximated polynomial in Equationequation (5.5)(5.5)
(5.5) , we get
(5.7)
(5.7)
On solving, we get
(5.8)
(5.8)
The values of the integrals mentioned in above equation are as follows
(5.9)
(5.9)
Substituting values of integrals in Equationequation (5.8)(5.8)
(5.8)
(5.10)
(5.10)
5.2. Atangana-Seda method with Caputo derivative
Applying fractional integral on the above system using definition 1, we get
(5.12)
(5.12)
At point
(5.13)
(5.13)
The above can be written as
(5.14)
(5.14)
Using Newton’s polynomial approximation we write as
(5.15)
(5.15)
Substituting approximated through Newton’s polynomial in Equationequation (5.14)
(5.14)
(5.14) , we get
(5.16)
(5.16)
Lastly, on solving the integrals in the above expression, we finally get
(5.17)
(5.17)
To find the numerical solution, we make use of the Atangana-Seda (Atangana & Araz, 2018, Citation2020; Gnitchogna & Atangana, Citation2018; Toufik & Atangana, Citation2017).
Now, using the aforementioned numerical scheme, we finally get the numerical solution for the Caputo fractional SIAQR model as
(5.18)
(5.18)
where
(5.19)
(5.19)
where
(5.20)
(5.20)
where
(5.21)
(5.21)
where
(5.22)
(5.22)
where
5.3. Numerical simulations
Numerical simulations for SIAQR model with ,
,
,
,
,
,
,
,
,
.
In , we see the numerical simulations for z = 0.001 and in fractional order
We see the effect of adding the time-dependent parameter z. The figure shows that susceptible, infected symptomatically, and infected asymptomatically decrease, and recovered individuals increase.
In , we have presented the numerical simulation at
We see that as the order of fractional derivative increases from
to
the decrease in the number of individuals who are infected symptomatically decreases well and the number of quarantined individuals increases.
In , we have presented the numerical simulation at
and z = 0.001. We see that as the order of fractional derivative increases from
to
the decrease in the decrease in the number of individuals who are symptomatically infected is much greater compared to
and
Furthermore, we can see that the number of asymptomatically infected individuals is also decreased.
In , we have presented the numerical simulation at
and z = 0.01. We see that as the value of z increases from 0.001 to 0.01, the decrease in the decrease in the number of individuals who are infected symptomatically and asymptomatically is much greater. This shows the effect of time dependency on the infection rate due to the parameter z, which determines the change in the time of infection.
In , we have presented the numerical simulation at
and z = 0.01. We see that as the value of z increases from 0.001 to 0.01. The number of recovered individuals increases well and the number of asymptomatically infected individuals is quite small.
In , we have presented the numerical simulation at
and z = 0.01. We see that as the value of z increases from 0.001 to 0.01. The number of recovered individuals is much more than the number of individuals who are symptomatically and asymptomatically infected.
In , we have presented the numerical simulation at
and z = 0.1. We see that due to the effect of the parameter z (change in infection time), there is a sharp increase in the number of quarantined individuals, and hence the recovery rate is also much higher.
6. Conclusion
In this work, we have formulated the SIAQR model for Covid pestilence with a time-dependent infection rate. The SIAQR model is then extended to the fractional mathematical model within the framework of the Caputo derivative operator. The existence and uniqueness of the solution of the SIAQR system is carried out using the Lipschitz condition and the linear growth condition. The numerical scheme for the Caputo fractional SIAQR model is derived using the newly proposed Atangana-Seda method. Numerical simulations are performed for the SIAQR model with a time-dependent infection rate for different fractional order derivatives. Furthermore, we see that as the value of z increases, the number of infected individuals decreases. This is because the rate of infection is lower as the value of z increases. We also see that as the order of fractional derivatives increases, the number of infected populations decreases, and the number of recovered populations increases. Hence, the fractional derivatives provide us with a better understanding of the model.
Acknowledgements
Authors are thankful to reviewers for their constructive comments to improve this article. The researchers would like to acknowledge the Deanship of Scientific Research, Taif University for funding this work.
References
- Ahmad, A., Farman, M., Naik, P. A., Zafar, N., Akgul, A., & Saleem, M. U. (2021). Modeling and numerical investigation of fractional-order bovine babesiosis disease. Numerical Methods for Partial Differential Equations, 37(3), 1946–1964. doi:10.1002/num.22632
- Ain, Q. T., Anjum, N., Din, A., Zeb, A., Djilali, S., & Khan, Z. A. (2022a). On the analysis of Caputo fractional order dynamics of Middle East lungs coronavirus (MERS-Cov) model. Alexandria Engineering Journal, 61(7), 5123–5131. doi:10.1016/j.aej.2021.10.016
- Ain, Q. T., Anjum, N., & He, C. H. (2021). An Analysis of time-fractional heat transfer problem using two-scale approach. GEM: – International Journal on Geomathematics, 12(1), Article No. 18. doi:10.1007/s13137-021-00187-x
- Ain, Q. T., & He, J. H. (2019). On two-scale dimension and its applications. Thermal Science, 23(3 Part B), 1707–1712. doi:10.2298/TSC1190408138A
- Ain, Q. T., Khan, A., Ullah, M. I., Alqudah, M. A., & Abdeljawad, T. (2022b). On fractional impulsive system for methanol detoxification in human body. Chaos Solitons & Fractals, 160, 112235. doi:10.1016/j.chaos.2022.112235
- Ain, Q. T., Nadeem, M., Karim, S., Akgül, A., & Jarad, F. (2022c). Optimal variational iteration method for parametric boundary value problem. AIMS Mathematics, 7(9), 16649–16656. doi:10.3934/math.2022912
- Alkahtani, B. S. T., & Alzaid, S. S. (2020). Atangana-Seda numerical scheme apply to nonlinear circuit problems. Alexandria Engineering Journal, 59(4), 2027–2036. doi:10.1016/j.aej.2019.12.035
- Alkahtani, B. S. T., & Alzaid, S. S. (2021). A theoretical analysis of a SEAIJR model of Spanish flu with fractional derivative. Results in Physics, 26, 104236. doi:10.1016/j.rinp.2021.104236
- Alshomrani, A. S., Ullah, M. Z., & Baleanu, D. (2021). Caputo SIR model-19 under optimized fractional order. Advances in Difference Equations, 2021(1), 185. doi:10.1186/s13662-021-03345-5
- Al-Smadi, M., Djeddi, N., Momani, S., Al-Omari, S., & Araci, S. (2021). An attractive numerical algorithm for solving nonlinear Caputo-Fabrizio fractional Abel differential equation in a Hilbert Space. Advances in Difference Equations, 2021(1), Article ID 271. doi:10.1186/s13662-021-03428-3
- Anjum, N., Ain, Q. T., & Li, X. X. (2021a). Two-scale mathematical model for tsunami wave. GEM - International Journal on Geomathematics, 12(1), Article Number 10. doi:10.1007/s13137-021-00177-z
- Anjum, N., He, C. H., & He, J. H. (2021b). Two-scale fractal theory for the population dynamics. Fractals, 29(07), Article ID 2150182 . doi:10.1142/S0218348X21501826
- Atangana, A. (2020). Extension of rate of change concept: From local to nonlocal operators with applications. Results in Physics, 19, 103515. doi:10.1016/j.rinp.2020.103515
- Atangana, A., & Aguilar, J. F. G. (2018). Decolonisation of fractional calculus rules: Breaking commutativity and associativity to capture more natural phenomena. The European Physical Journal plus, 133(4), Article No. 166. doi:10.1140/epjp/i2018-12021-3
- Atangana, A., & Araz, S. I. (2020). Atangana-Seda numerical scheme for Labyrinth attractor with new differential and integral operators. Fractals, 28(08), 2040044. doi:10.1142/S0218348X20400447
- Atangana, A., & Araz, S. I. (2020). New numerical method for ordinary differential equations: Newton polynomial. Journal of Computational and Applied Mathematics, 372, 112622. doi:10.1016/j.cam.2019.112622
- Atangana, A., & Araz, S. I. (2021). Modeling and forecasting the spread of COVID-19 with stochastic and deterministic approaches: Africa and Europe. Advances in Difference Equations, 2021(1), 57. doi:10.1186/s13662-021-03213-2
- Atangana, E., & Atangana, A. (2020). Facemasks simple but powerful weapons to protect against COVID-19 spread: Can they have side effects? Results in Physics, 19, 103425. doi:10.1016/j.rinp.2020.103425
- Djeddi, N., Hasan, S., Al-Smadi, M., & Momani, S. (2020). Modified analytical approach for generalized quadratic and cubic logistic models with Caputo-Fabrizio fractional derivative. Alexandria Engineering Journal, 59(6), 5111–5122. doi:10.1016/j.aej.2020.09.041
- Gnitchogna, R., & Atangana, A. (2018). New two step Laplace Adam-Bashforth method for integer a noninteger order partial differential equations. Numerical Methods for Partial Differential Equations, 34(5), 1739–1758. doi:10.1002/num.22216
- Hasan, S., Al-Smadi, M., Dutta, H., Momani, S., & Hadid, S. (2022). Multi-step reproducing kernel algorithm for solving Caputo-Fabrizio fractional stiff models arising in electric circuits. Soft Computing, 26(8), 3713–3727. doi:10.1007/s00500-022-06885-4
- Iannni, A., & Rossi, N. (2020). Describing the COVID-19 outbreak during the lockdown: Fitting modified SIR models to data. The European Physical Journal plus. 135, Article No. 885. doi:10.1140/epjp/s.13360-020-00895-7
- Jarad, F., Abdeljawad, T., & Hammouch, Z. (2018). On a class of ordinary differential equations in the frame of Atangana–Baleanu fractional derivative. Chaos Solitons Fractals, 117, 16–20. doi:10.1016/j.chaos.2018.10.006
- Karaji, P. T., & Nyamoradi, N. (2020). Analysis of a fractional SIR model with general incidence function. Applied Mathematics Letters, 108, 106499. doi:10.1016/j.aml.2020.106499
- Khan, M. A., & Atangana, A. (2020). Modeling the dynamics of novel coronavirus (2019-nCov) with fractional derivative. Alexandria Engineering Journal, 59(4), 2379–2389. doi:10.1016/j.aej.2020.02.033
- Kilbas, A. A., Srivastava, H. M., & Trujillo, J. J. (2006). Theory and applications of fractional differential equations. In North-Holland mathematics studies (pp. xvi + 523). Elsevier.
- Miller, K. S., & Ross, B. (1993). An introduction to the fractional calculus and fractional differential equations. (pp. xvi + 366). New York: Willey.
- Modi, K., Umate, L., Makade, K., Dubey, R. S., & Agarwal, P. (2021). Simulation based study for estimation of COVID-19 spread in India using SEIR model. Journal of Interdisciplinary Mathematics, 24(2), 245–258. doi:10.1080/09720502.2020.1838059
- Momani, S., Djeddi, N., Al-Smadi, M., & Al-Omari, S. (2021). Numerical investigation for Caputo-Fabrizio fractional Riccati and Bernoulli equation using iterative reproducing kernel method. Applied Numerical Mathematics, 170, 418–434. doi:10.1016/j.apnum.2021.08.005
- Naik, P. A., Ghoreishi, M., & Zu, J. (2022). Approximate solution of a nonlinear fractional-order HIV model using homotopy analysis method. International Journal of Numerical Analysis and Modeling, 19(1), 52–84.
- Naik, P. A., Yavuz, M., Qureshi, S., Zu, J., & Townley, S. (2020). Modeling and analysis of COVID-19 epidemics with treatment in fractional derivatives using real data from Pakistan. The European Physical Journal plus, 135(10), Article No. 795. doi:10.1140/epjp/s13360-020-00819-5
- Naik, P. A., Zu, J., & Naik, M. U. (2021). Stability analysis of a fractional-order cancer model with chaotic dynamics. International Journal of Biomathematics, 14(06), 2150046. doi:10.1142/S1793524521500467
- Oldham, K. B., & Spanier, J. (1974). The fractional calculus. Theory and applications of differentiation and integration to arbitrary order. In Mathematics in science and engineering (pp. xiii + 234). New York: Academic Press.
- Toufik, M., & Atangana, A. (2017). New numerical approximation of fractional derivative with non-local and non-singular kernel. The European Physical Journal plus, 132(10), Article No. 444. doi:10.1140/epjp/i2017-11717-0
- Ucar, S., Ucar, E., Ozdemir, N., & Hammouch, Z. (2019). Mathematical analysis and numerical simulation for a smoking model with Atangana–Baleanu derivative. Chaos Solitons Fractals, 118, 300–306. doi:10.1016/j.chaos.2018.12.003