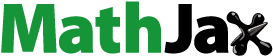
ABSTRACT
Contamination by hexavalent chromium [Cr (VI)] poses a threat to groundwater quality and its detection at point source is essential to provide early mitigating solutions. In this work, we report the fabrication of paper-based sensing system embedded with a novel nano-chromogenic complex having spherical gold nanoparticles modified with 1,5-diphenylcarbazide dye. This Au-DPC Functionalized paper strip develops pink color in <2 sec upon interaction with Cr (VI). With the developed optical fiber device a limit of detection of 0.02 ppm was achieved within a linear range of 0.01–0.4 ppm. RGB color analysis and data driven predictive modelling (KNN-model) demonstrated highest balanced accuracy score of 0.833 and cross validation accuracy of 0.714. Further, the portable optical fiber-based device offers advantages such as real-time monitoring, remote sensing capabilities, and the ability to integrate with existing optical systems for enhanced detection and analysis.
1. Introduction
Environmental pollution monitoring is a major contemporary challenge. Natural and anthropogenic activities caused massive leaching of heavy metals, toxins, organic dyes and various other harmful chemicals to water bodies, thereby contaminating them [Citation1]. Natural and anthropogenic activities and rampant industrialization cause massive leaching of poisonous gases, volatile organic compounds, persistent organic pollutants, radioactive contaminants, heavy metals, toxins, pesticides and dyes in the environment, causing long-lasting detrimental effects [Citation1–4]. Heavy metals in particular, pose a threat to all spheres of the environment (air, water, soil and biosphere) due to their ability to bioaccumulate and cause persisting toxic effects [Citation5]. Chromium is one of the most potent, toxic, teratogenic and carcinogenic heavy metal ions existing in nature [Citation6]. Chromium exists in various oxidation states, out of which trivalent [Cr (III)] and hexavalent [Cr (VI)] are the stable state oxyanionic forms found in the environment. Hexavalent chromium is extensively used as a component in pigments, paints and dyes, and in chrome plating industries and exposure to Cr (VI) can cause cancer and organ specific toxicity in humans [Citation7]. Owing to its carcinogenic and bio-hazardous nature, the governing bodies such as World Health Organization (WHO), various Environmental Protection Agencies (EPAs) and Pollution Control Boards (PCBs) globally have fixed their permissible limits in drinking water, beyond which the water is deemed unsafe for human consumption [Citation7]. The Bureau of Indian standards and the US EPA recommends the maximum permissible limit for Cr (VI) in drinking water to be 50 µg/L (50 ppb) and 10 µg/L (10 ppb), respectively [Citation8].
The conventional methods for detection and quantitative estimation of heavy metals includes UV-Visible spectroscopy, Atomic Absorption Spectroscopy (AAS), Inductively Coupled Plasma- Optical Emission Spectrometry (ICP-OES) & Mass Spectroscopy (ICP-MS) [Citation9]. Even though these methods are highly sensitive and accurate, they are costly, highly tedious, requiring elaborate lab set-up and skilled manpower [Citation10]. Alongside, sample pre-treatment and pre-concentration before testing make it expensive and unsuitable for field detection [Citation11]. Ease of detection and accuracy are the basis of field detection methods, hence advanced detection methods are the need of the hour. Nanomaterials such as nanoparticles, nanofibers, and nanocomposites can be engineered to have a high affinity for chromium ions. These nanomaterials provide a large surface area for adsorption, enabling efficient removal of chromium from contaminated water or soil. Functionalizing the nanomaterials with specific surface groups can enhance their selectivity towards chromium ions. Nanoparticles can be employed to facilitate the breakdown of toxic forms of chromium, such as hexavalent chromium (Cr(VI)), into less harmful forms like trivalent chromium (Cr(III)). Nanoparticles with catalytic properties, such as zero-valent iron nanoparticles, can be used to reduce Cr(VI) to Cr(III) through redox reactions [Citation12,Citation13]. Nanoparticles and advanced functional nanomaterials play a crucial role in the emerging trend for sensitive detection and remediation of environmental pollutants. Nanomaterials exhibit optical and electrochemical signal enhancing properties due to easy tunability, structural versatility, low toxicity, uniform size distribution, chirality, and stability. Gold [Citation14–16], silver [Citation17,Citation18], iron & iron oxide [Citation19], bi-metallic, fluorescent nanoparticles [Citation20,Citation21] and quantum dots [Citation22] are used increasingly for the rapid and trace level detection of heavy metals on site. Nanocomposites also exhibit intrinsic properties such as high surface area, abundant availability of functional moieties and enhanced sensing capability that make them ideal materials to be used in optical and electrochemical sensing of environmental pollutants and pharmaceutical waste [Citation23,Citation24]. Additionally, nanoadsorbents, and frameworks modified with different nanomaterials are increasingly being used for the removal and remediation of various heavy metals such as arsenic [As (V)], cadmium [Cd (II)], iron [Fe (II & III)] and chromium [Cr (VI)] from environmental matrices [Citation25–29].
Recently utilization of low-cost paper-based sensors as bioanalytical tool have increased in environmental studies, food safety and diagnostics [Citation30]. Compared to conventional sensors, paper-based and microfluidic sensors have the definite advantage of being cost-effective, user-friendly, simple fabrication, biocompatibility, and biodegradability [Citation31]. Paper-based analytical methods operate on various principles including colorimetric methods, electrochemical detection, chemiluminescence, fluorescence, surface-enhanced Raman spectroscopy (SERS) [Citation32,Citation33]. Among these, colorimetric detection is well established requires no complex reagents and instruments, is easy to perform and interpret and relatively inexpensive [Citation34]. Lab developed devices are also gaining popularity for the detection and estimation of heavy metals and other pollutants [Citation35,Citation36]. Paper-based substrates also facilitate portability and on-site, real time monitoring. Keeping all these factors in mind, researchers have developed various paper-based colorimetric and smartphone-based detection devices [Citation37]. Optical and smartphone-based devices can rapidly decode paper generated data into digital signals and images, providing real time analysis [Citation14,Citation38,Citation39].
Advanced analysis and prediction can help researchers to quickly detect elements. Over the decades, machine-learning models have played a major role in classification and prediction [Citation40]. The concentrations of toxic metal elements can be estimated by adapting machine learning models [Citation41]. The concentration of Cr (VI) was measured using a hybridized experimental-predictive model combined with a convolutional neural network and autoencoder. Significant accuracy between 75–85%was achieved using a nonlinear mixture of heavy metal concentration [Citation42]. The statistical exploration of spectral reflectance properties and correlation analysis indicates the importance and selection of the characteristic band for further machine learning modelling [Citation43].
In this paper, we have demonstrated the fabrication of a simple nano-chromogenic complex (AuNP-DPC Functionalized sensing strips: ADF sensing strips) POD for Cr (VI). The proposed sensor requires very low sample volume without pre-treatment. The POD measures Cr (VI) concentration based on the changes in the refractive index and the color gradient developed on the sensing zone of strips. The POD results were further supported by Image analysis of the ADF strips. Additionally, a hybrid colorimetric-optical sensing approach and predictive modelling based on optical variables was comparatively analyzed using Support Vector Machines (SVM), Logistic Regression Model (LRM), K-Nearest neighbor (KNN) and Random Forest (RF) algorithms [Citation44].
2. Experimental section
2.1. Reagents
High purity analytical grade reagents were procured and used throughout the experimental process without further purification unless specified. Chloroauric acid (HAuCl4), L-ascorbic acid (C6H8O6), polyvinylpyrrolidone (PVP), 1,5-diphenylcarbazide (DPC), hydrochloric acid (HCl) and potassium dichromate (K2Cr2O7) were procured from Loba Chemie Pvt. Ltd. Whatman Filter paper grade 1 was procured from MyLab Discovery Solutions and used as required. Throughout the experimental procedure, Milli-Q grade (18.2 MΩ.cm) water was used. Additionally, aqua regia solution (HCl: HNO3 = 3:1) was used to clean all the glasswares used during the experimental process, followed by repeated rinsing with Milli-Q (18.2 MΩ.cm) water.
2.2. Experimental
2.2.1. AuNP and AuNP-DPC complex synthesis
Sol-Gel method was utilized to synthesize PVP-stabilized gold nanoparticles (AuNPs) at room temperature [Citation45]. HAuCl4 (0.5 mM) was dissolved in 50 ml Milli-Q water (Solution A) and stirred constantly. In a separate beaker, 1 mM of L-Ascorbic acid was dissolved in 10 ml Milli-Q water (Solution B). Freshly prepared mixture B was added to mixture A dropwise at room temperature in dark. Then, an aqueous mixture of 500 µl PVP was rapidly added, and the reaction vessel was sealed and kept under constant stirring at 298 K for 2 hours. The change in color from yellow to pink after 2 hours indicates successful synthesis of AuNP’s. The resulting AuNPs were stored at 4°C for further characterization and application. To obtain AuNP-DPC complexes, the method reported by [Citation46]was modified. A 0.1 mM solution of DPC was prepared in a methanol: water system (1:9 ratio), and a small aliquot was added to the PVP-stabilized AuNP solution (50 nM) at 1:1 ratio with constant stirring at room temperature in an amber-colored bottle for 4 hours. The solution was periodically checked for aggregation or precipitation and stored at 4°C for further utilization.
2.2.2. Characterization
Spectroscopic measurements were conducted to confirm optical properties of synthesized nanoparticles and nanoparticle complex, using a Jasco V-750 UV-Visible Spectrophotometer. Particle size distribution and zeta potential analysis were performed on Anton Parr Litesizer 500 GmbH instrument. Nanoparticle shape, size, morphology, and distribution were studied by transmission electron microscopy (TEM) using a Tecnai 12 G2 TEM. Scanning electron microscopy (SEM) of ADF sensor strips was also performed using a Quant 200 ESEM with EDS detector at 20 kV. Chemical structure and functional groups in ADF sensing strips before and after interaction with Cr (VI) were analyzed by Fourier Transform Infrared Spectroscopy (FT-IR) using Shimadzu IRAffinity-1 spectrophotometer with a diamond ATR accessory at room temperature.
2.2.3. Fabrication of ADF sensing strips (Au-DPC Functionalized sensing strip)
Whatman filter paper #1 with 180 μm thickness and 11 μm pore size was used to immobilize the AuNP-DPC complex. Strips measuring 10 mm × 40 mm were cut and cleaned by briefly soaking in EtOH for 1 minute, rinsing with deionized water, and air-drying for 15 minutes [Citation14,Citation47]. Circular sensing zones with 6 mm diameter were created using stencil and permanent marker, and then backed by hydrophobic tape to prevent leaching. 5 µl of AuNP-DPC complex was added to each sensing zone and dried in a hot air oven at 40°C for 10 minutes. The process was repeated for better performance. These strips are referred to as ADF sensing strips.
2.2.4. Design of optical fiber detection setup
is a schematic representation of the no-contact POD set-up used to detect and estimate Cr (VI) from aqueous samples. A green LED of wavelength 535 nm is used as AuNP-DPC and Cr (VI) complex shows maximum absorbance at 540 nm [Citation48]. An optical fiber with a PMMA core of diameter 980 µm and fluorinated silica cladding of thickness 20 µm with refractive index 1.49 and 1.455 respectively is used. A bifurcated optical fiber captures the diffuse reflected signal from the sensing zone of the ADF strips. The green light source is directed to the sensing region of the ADF strips using the distal end of the optical fiber kept at 3 mm. The reflected signal is transmitted to the photodetector and converted to voltage in the microcontroller unit.
2.2.5. ADF sensing strip-based detection of Cr (VI)
A 100-ppm stock of K2Cr2O7 was prepared by dissolving 2.747 gm oven-dried K2Cr2O7 salt in 1000 ml Milli-Q. Eleven working solutions of known concentrations between 0.01 to 1.0 ppm were made by diluting the stock solution. To detect using the ADF strips, 2 µl of acidified sample was added to the detection zone and color was immediately observed. Voltage responses were recorded by the optical fiber within 5 seconds. Ten voltage responses were recorded for each concentration from different regions within the detection zone, and the average voltage was calculated. To reduce error, individual measurements were carried out in triplicate for each concentration of Cr (VI) (from 0.01 to 1.0 ppm).
2.2.6. Image analysis
Images of individual detection zones were analyzed using the ImageJ software version 1.46 r, to determine RGB values, while a Dino-Lite Edge 3.0 digital microscope was used to record colorimetric responses and images were saved in JPEG format at 300 dpi. Color development on individual sensing zones was compared to the blank or control zone, and grayscale distribution was determined as later described in section 3.5 [Citation34].
2.2.7. Analysis of environmental samples
The POD’s practical feasibility and accuracy was assessed using spiked sample recovery. Four water samples from different locations in Pune were collected, filtered (via 0.22 μm filter membranes), and acidified as described in section 2.2.5. Spiked samples were prepared by adding 0.1 ppm Cr (VI) standard to accidified real water samples. The original and spiked samples were analyzed and the percentage accuracy of the POD was calculated and compared against UV-Visible diffuse reflectance spectroscopy.
2.2.8. Computational analysis
We used data-driven predictive modelling algorithms to estimate Cr (VI) via detector voltage responses of the ADF strip. Four machine learning models, that is, KNN, SVM, LR, and RF, were compared to predict Cr (VI) concentrations using a Python module for data simulation and deep learning. The voltage values were stacked and fed into the models, and statistical parameters such as Root Mean Squared Error (RMSE), Mean Absolute Error (MAE), and Balanced Accuracy Score were evaluated using 75:25 Training: Testing paradigm for training and validating the models.
3. Results & discussion
3.1. Material characterization
3.1.1. UV-Visible spectroscopy
Conventional method for AuNP synthesis includes Turkevich method that involves the reduction of chloroauric acid by trisodium citrate dehydrate solution at 100°C [Citation49]. The formation of AuNPs and AuNP-DPC complex is preliminarily confirmed by the appearance of characteristic localized surface plasmon resonance (LSPR) peaks in UV-visible spectroscopy. The morphology and in situ self-assembly of AuNPs and AuNP-DPC complex provides the desired stability to the sensing system [Citation50]. exhibits the UV spectra of the synthesized AuNPs and AuNP-DPC complex. The PVP-capped AuNPs have absorbance peak at 538 nm and are wine red in color. However, after interaction with DPC, the surface plasmon resonance (SPR) peak shows a bathochromic shift and the λmax value shifts to 530 nm [Citation51]. However, the observed red shift in λmax from 538 to 530 nm is due to the covalent attachment of DPC on the surface of the AuNPs to form AuNP-DPC complex with higher size.
Figure 2. (a) UV-Visible absorbance spectra of the synthesized AuNP colloidal suspension and AuNP-DPC complex with λmax at 538 nm and 530 nm, respectively (Inset: Hydrodynamic diameter of the AuNPs and AuNP-DPC complex and table summarizing changes in Zeta potential values of AuNP and AuNP-DPC complex) (b) FTIR spectra of ADF sensing strip before and after interaction with Cr (VI).

3.1.2. DLS- Particle size and zeta potential
The hydrodynamic diameter (HD) and zeta potential (ZP) values of the samples are illustrated in the inset of . The HD is an indicative parameter of how a particle reacts with incident light and diffuses within a liquid [Citation52]. The size histogram obtained for AuNPs indicates a HD of 42.5 ± 5 nm for PVP capped AuNPs. In addition, polydispersity index percentage (PDI %) of 16.1% indicates that AuNPs are monodispersed with uniform size distribution [Citation53]. However, with DPC, the HD increases to 90.7 ± 5 nm and PDI % increases to 21.4%, indicating changes in the surface morphology and uniform size distribution in AuNP-DPC complex. Furthermore, on reaction with 0.5 ppm acidified Cr (VI), HD further increased to 109.6 ± 5 nm and PDI % increases to 25.66% indicating the chelation of Cr (VI) with the AuNP-DPC complex, that directs the aggregation of AuNPs. The ZP of the AuNPs was measured to be −29.7 mV showing high stability. On complexation with DPC, ZP showed a slight increase and was found to be −27.04 mV, which still indicated stability. The minor change in ZP value also validates the attachment of DPC with the PVP capped AuNPs through hydrogen binding. However, on reaction with 0.5 ppm Cr (VI), ZP value of the solutions rapidly increased to −15.4 mV, which clearly demonstrated a loss in stability of the particles, leading to aggregation [Citation46]. The in situ redox reaction occurring in reaction mixture, oxidizes DPC to 1,5-diphenylcarbazone (DPCO) and help to form co-ordinate bonds between DPCO and Cr (VI) that leads to AuNPs aggregation.
3.1.3. FTIR spectroscopy
Confirmation of functional groups present in individual reaction components as well as to determine the groups involved in the formation of complex structures were done by FTIR spectra of the ADF strips before and after Cr (VI) absorption within wavenumber range of 400–4000 cm−1 as shown in . Prominent spectral bands were observed at 3340 cm−1, 2985 cm−1, 1728 cm−1, 1448 cm−1, 1165 cm−1, 1026 cm−1 and 856 cm−1in the ADF strip. The broad peak observed at 3340 cm−1 indicates weak intermolecular bonding and stretching of the O-H group in the polysaccharide chain of the cellulose-based Whatman filter paper [Citation54]. The broad peak at 2985 cm−1indicates O-H stretching of the alcoholic fractions.
A weak shoulder peak at 2930 cm−1 suggests the presence of aldehydic C-H stretching. The other prominent sharp peak observed at 1728 cm−1 is attributed to the stretching vibration of the C=O of the carboxylic group in filter paper. This may indicate the binding between the amide group present in the DPC with the carboxylic acid of ADF strip. The peaks at 1442 cm−1 and 1311 cm−1 also indicate medium bending of the carboxylic O-H groups. The spectral bands observed at 1165 cm−1, 1026 cm−1 and 856 cm−1 indicate the stretching and bending vibrations of the C-H, O-H and C=O bonds in cellulose [Citation55].
The FTIR spectra of the filter paper after addition of Cr (VI) ions shows marked changes in the spectral bands in the IR spectrum. The major peaks of O-H, present at 3340 cm−1 and 2985 cm−1 are still prominently visible but there is change in the peak intensity that may be indicative of hydrogen binding between the Cr (VI) and some of the groups present in the ADF sensing strip. However, a remarkable change is observed in the intensity of the peaks at 1728 cm−1 and 1026 cm−1. The peak intensities have reduced considerably as the C=O and C-O in DPC change to 1,5-diphenylcarbazone (DPCO) on binding with Cr (VI) [Citation55]. Another key change observed at 856 cm−1 indicating the binding between DPC and Cr (VI). Cr (VI) acts as a strong base attacking oxygen containing species generating the change in intensity of the C=O group present in the DPC and PVP capped AuNPs [Citation56]. The change in the peak intensity at 694 cm−1 also indicates the complexing of the Cr (VI) with the N-H group in DPCO.
3.1.4. TEM, SEM and elemental mapping results
Details about the morphology and structure of nanoparticles were obtained via TEM as shown in . The TEM of bare AuNPs (data not shown here), indicates formation of spherical, crystalline microspheres with a size range of 42.5 ± 4 nm. However, upon interaction with DPC, the average diameter of AuNP-DPC increased to 93.3 ± 5 nm as represented in . The inset of represents the particle size distribution which clearly indicates that even after functionalization with DPC, the size of the AuNPs increased. This increase in size is attributed to the attachment and uniform distribution of the DPC molecules on the surface of the PVP capped AuNPs. PVP facilitates DPC attachment on the surface of the AuNPs by providing free functional groups. Furthermore, upon addition of 0.1 ppm Cr (VI) standard, the AuNP-DPC complex is seen to aggregate as shown in . The particle size of the aggregated particles was found to be 110 ± 5 nm. Cr (VI) forms coordinate bonds with the DPC and since DPC is attached to the surface of the AuNPs, aggregation is observed. This aggregation leads to enhanced SPR phenomena. DPC is extensively used as the standard chromogenic dye for chromium detection and speciation. However, it is used in higher quantities in the standard protocol. But, by functionalizing AuNPs with DPC we reported significant reduction in the concentration of DPC used with enhanced colorimetric sensing.
Figure 3. TEM Micrographs of (a) AuNP-DPC Complex showing surface functionalization of AuNPs with DPC (Inset: Particle size distribution histogram); (b) AuNP-DPC complex after interaction with 0.1 ppm Cr (VI) depicting aggregation in nano-chromogenic complex (Inset: Zoomed in image of aggregated nano-chromogenic complex); (c) ADF sensing strip after interaction with 0.1 ppm Cr (VI) (Inset: ADF sensing strip before interaction); (d) Elemental distribution profile for C, O, Au and Cr elements.

Figure 4. Colorimetric determination and Correlation with Optical device readings (a) Calibration curve indicating correlation between values of optical readings and varying Cr (VI) concentration (0.01 to 1 ppm). (Inset: Images taken after development of pink spot on the ADF sensing strip for Optical determination of Cr (VI)); (b) Selectivity of the ADF strip-based POD toward Cr (VI) in presence of 1 ppm of interfering ions (Inset: Probable reaction mechanism highlighting in situ redox between AuNP-DPC complex and Cr (VI)).

The ADF strips were also subjected to SEM with EDX and elemental mapping to confirm the embedment of the AuNP-DPC complex in the filter papers and interaction with Cr (VI). The AuNP-DPC complex is a small molecule that can easily accumulate in the pores of the filter paper. Elemental mapping reveals the presence of AuNPs in the fibres as shown in . After interaction with Cr (VI), the aggregated AuNP-DPC-Cr complex can be seen accumulated on the fibers of the ADF strips as seen from . As shown in , the elemental percentage values for AuNPs and Cr (VI) obtained from the ADF sensing strip after interaction were 0.8% and 0.1%, respectively.
3.2. Colorimetric assay and reaction mechanism
The probable reaction mechanism for colorimetric assay is illustrated in the inset of and discussed in this section. Interaction between the AuNP-DPC complex with Cr (VI) results in the in situ redox reaction in which DPC is oxidized to DPCO with simultaneous reduction of Cr (VI) to Cr (III). Further complexation of Cr (III) with DPCO results in AuNPs agglomeration, and the color changes from red to pink-purple. The redox reaction can be established as follows:
Figure 5. Image analysis and RGB correlation between different Cr (VI) concentrations and color gradient on the ADF sensing strips depicting variation in Cr (VI) concentration (Inset: Image Histogram obtained from spot images of varying Cr (VI) concentrations showing grayscale distribution with normalized intensity).

(1)
(2)
Colorimetric responses in the aqueous phase are illustrated in . With increase in Cr (VI) concentration, the absorbance at 530 nm decreases. The colorimetric reaction can be visually perceived on the paper within 2 seconds. demonstrates the concentration dependent changes in color gradient on interaction with Cr (VI). The colorimetric responses obtained on the ADF sensing strips are uniform and can be correlated linearly with the concentration of Cr (VI) in the sample.
3.3. Cr (VI) sensing
For accurate and sensitive analysis, a range of known concentrations of Cr (VI) ions was prepared and each set was performed in triplicate. The sensing zone of ADF strips was measured within 1 min after analyte addition and multiple measurements of the same sample by varying the region of interest were taken and means were taken for further calculations. This simple operation and instant response fulfilled the key criteria for portable and field deployable systems and the optical fiber device can be used for on-site detection of Cr (VI). The development of color and gradient development with increase in Cr (VI) concentration can be ascertained in in the range of 0.01–1.0 ppm. At highest concentration, that is, 1 ppm, a dark pink-purple spot within the hydrophobic sensing zone can be seen instantly within 1 sec. At concentrations ≤0.01 ppm, the color is almost undiscernible visually and the detector voltage do not show much deviations from that of the blank ADF strip.
Immediately after spot development on sensing zone, the ADF sensing strips were analyzed by the optical fiber device [Citation58]. The detector voltage readings vary inversely with increasing analyte concentration, that is, highest intensity was observed in the Blank ADF strip while the lowest at 1.0 ppm Cr (VI) exhibiting linear correlation with the Cr (VI) concentration in the range of 0.01 to 0.4 ppm. The device was calibrated with a range of Cr (VI) concentrations on ADF strip. The voltage values of the optical fiber are shown to exhibit good linear correlation with the Cr (VI) concentration values in the range of 0.01 to 0.4 ppm. The correlation equation was found to be ΔV = 0.31428 + 0.01563 C; where, ΔV value is calculated by subtracting the voltage of sample from the voltage of blank zone and C is the concentration of Cr (VI). It was evident from the measurements that the POD could selectively detect and estimate Cr (VI) concentration at a lower limit of 0.02 ppm. This lower limit suggests that the POD can be suitably used for water monitoring in real environment. The reproducibility and stability of the POD was satisfactory.
3.4. Selectivity
Selectivity of the sensing agent was evaluated towards Cr (VI) by measuring responses against other environmentally hazardous metal ions such as Al3+, Ba2+, Cd2+, Co2+, Cu2+, Mg2+, Pb2+, Fe3+ and Ni2+ under same experimental conditions. The final concentration of the metal ions was adjusted to 1ppm and each individual metal ion solution was added to the ADF sensing strips and optical responses measured within 1 min and no visible change was observed. On measuring response on POD, it was observed that the other ions give very weak responses as elucidated from . However, on introduction of 0.1 ppm Cr (VI), we could clearly observe color development and voltage jump on detector voltage. This validates the selectivity of the sensing system for Cr (VI).
3.5. Image analysis
The sensing zones of the fabricated ADF strips changed to pink on Cr (VI) addition while no color change is observed with other metal ions and was recorded by a Dino-Lite Edge 3.0 digital microscope. While color intensity was gauged using RGB detector. The results of colorimetric assay and image analysis are presented in . Image analysis was performed through RGB profiling, and the RGB values of the image were measured. The slope of the calibration curve as shown in , was utilized to calculate the error and Cr (VI) concentration [Citation59]. We can observe that with increasing Cr (VI) concentration, the intensity of pink spot increases. Color intensity increased in an exponential manner with increasing Cr (VI) concentration. To further minimize calculation error, the images were converted to 8-bit grayscale using ImageJ software and grayscale distribution was scrutinized and graphically plotted, as represented in the inset of . These histograms depict the ranges of color distribution with respect to different Cr (VI) concentrations.
3.6. Analysis of environmental samples
The feasibility of ADF sensing strip-based POD with real environmental samples were analysed, including river and tap water. The results were compared with diffuse reflectance UV-Visible spectrophotometry analysis as shown in where environmental samples tested negative for Cr (VI) concentration. However, on spiking the samples with 0.1 ppm Cr (VI), the relative accuracy percentages of 99.4, 103.7, 98.9 and 104% were obtained by our method as compared to 127, 141, 97.3 and 138%, respectively, by UV-Vis spectroscopy. These results indicate good conformity and comparability with the standard method and our device can be further exploited for detection and estimation of Cr (VI).
Table 1. Comparative results of environmental sample analysis by UV-Visible spectroscopy and ADF sensing strip-based POD.
3.7. Computational analysis
In this study, the observed data was collected at a 2.5 detector voltage (2.5 V) threshold. For each concentration (in triplicates), 10 voltage readings were recorded. This research was further extended by computational modelling. shows the framework for predicting Cr (VI) concentrations based on detector voltage readings. There are three different stages in the above framework: (i) Data Pre-processing, (ii) Model Prediction and (iii) Evaluation of the model’s performance using metrics. The first stage of this framework is to engineer the data where Concentration and Detector Voltage are two different units that are normalized by scaling using a linear transformation function, namely min-max, as shown in EquationEquations (1)(1)
(1) and (Equation2
(2)
(2) ).
Figure 6. Data driven Predictive Modeling (a) Schematic for data preprocessing and predictive modeling (b) & (c) Scatter plots of predicted vs observed values for evaluation of KNN model in standards and Real Environmental Samples.

Considation C, which contains the values of C1, C2, C3 …… Cn, where the new values are mapped as per EquationEquation (1)(1)
(1) , as ∆Ci is the new normalized concentration attribute. Similarly, the detector voltage attribute V contains the values of V1, V2, V3 …… Vn, where the new values are mapped as per EquationEquation (2)
(2)
(2) , as ∆Vi is the new normalized concentration attribute [Citation60].
Once the data were feature engineered, they were divided into training and testing datasets of 75% and 25%, respectively. This observatory data contained 12 classes, and each class had three different voltage values, and 10 readings were recorded at different points. Supervised learning models provide promising results, where the models are trained and validated to improve the performance of the prediction model. For this study, four machine learning models were utilized as previously mentioned. After training, the model was tested by passing the test data.
From experimental analysis, the RF-model scored balanced and CV model accuracies of 68% and 0.679 ± 0.036, respectively. The comparative data for voltage predictions for diferent Cr (VI) concentrations using the four predictive models is lited in . The RMSE and MAE of the RF-model are 0.8017 and 0.4285, respectively. The SVM-model achieved balanced and CV model accuracies of 62.85% and 0.515 ± 0.015, respectively. The LR-model scored balanced and CV model accuracies of 75% and 0.357 ± 0.071, respectively. The RMSE and MAE of SVM were 0.586 and 0.2857, respectively. The KNN-model achieved highest balanced and CV model accuracies of 83.33% and 0.714, respectively. The RMSE and MAE values of the KNN model were 0.586 and 0.36, respectively. It was observed that the KNN-model performed significantly better than the other ML-models in terms of accuracy, balanced accuracy, MAE, and RMSE.
Table 2. Comparative analysis of the voltage prediction models for different Cr (VI) concentrations.
4. Conclusion
The results reported in this study offer the possibility of assembling a low-cost portable POD for the detection and quantitative estimation of Cr (VI). The facile synthesized nano-chromogenic AuNP-DPC complex is a stable complex that acts towards selective colorimetric detection of Cr (VI). The ADF strip-based POD exhibits good detection limit within a wide concentration range of 0.01–1 ppm. Additionally, the POD has been successfully implemented for quantitative determination with a lower detection limit of 0.02 ppm within a linear range of 0.01–0.4 ppm in less than 5 seconds. The POD makes it a promising prospect for real time monitoring and estimation of Cr (VI). Analysis of spiked environmental samples also exhibit good conformity with standard analytical techniques. Additionally with computational predictive approach, the experimental model has been effectively validated. In future, spectral reflectance parameters and RGB values for detection of chromium will be considered by hybridizing optimized algorithms to improve the prediction of Cr (VI) concentration.
Disclosure statement
No potential conflict of interest was reported by the authors.
References
- Banerjee S, Kamila B, Barman S, et al. Interlining Cr(VI) remediation mechanism by a novel bacterium Pseudomonas brenneri isolated from coalmine wastewater. J Environ Manage. 2019;233:271–12.
- Deng Z, Deng Q, Wang L, et al. Modifying coconut shell activated carbon for improved purification of benzene from volatile organic waste gas. Adv Compos Hybrid Mater. 2021;4(3):751–760.
- Jansone-Popova S, Moinel A, Schott JA, et al. Guanidinium-based ionic covalent organic framework for rapid and selective removal of toxic Cr(VI) oxoanions from water. Environ Sci Technol. 2019;53(2):878–883.
- Si Y, Li J, Cui B, et al. Janus phenol–formaldehyde resin and periodic mesoporous organic silica nanoadsorbent for the removal of heavy metal ions and organic dyes from polluted water. Adv Compos Hybrid Mater. 2022;5(2):1180–1195.
- Briffa J, Sinagra E, Blundell R. Heavy metal pollution in the environment and their toxicological effects on humans. Heliyon. 2020;6(9):e04691.
- Pushkar B, Sevak P, Parab S, et al. Chromium pollution and its bioremediation mechanisms in bacteria: a review. J Environ Manage. 2021;287:112279.
- Prasad S, Yadav KK, Kumar S, et al. Chromium contamination and effect on environmental health and its remediation: a sustainable approaches. J Environ Manage. 2021;285:112174.
- Norton SB, Cormier SM, Suter GW, et al. CADDIS: the causal analysis/diagnosis decision information system. In: Marcomini A, Suter II G, Critto A, editors. Decision support systems for risk-based management of contaminated sites. Boston, MA: Springer US; 2008. pp. 1–24.
- Ilieva D, Surleva A, Murariu M, et al. Evaluation of ICP-OES method for heavy metal and metalloids determination in sterile dump material. Solid State Phenom. 2018;273:159–166.
- Aragay G, Pons J, Merkoci A. Recent trends in macro-, micro-, and nanomaterials-based tools and strategies for heavy-metal detection. Chem Rev. 2011;111(5):3433–3458.
- Gallardo-Gonzalez J, Baraket A, Boudjaoui S, et al. A fully integrated passive microfluidic Lab-on-a-Chip for real-time electrochemical detection of ammonium: sewage applications. Sci Total Environ. 2019;653:1223–1230.
- Manjari G, Saran S, Radhakrishanan S, et al. Facile green synthesis of Ag-Cu decorated ZnO nanocomposite for effective removal of toxic organic compounds and an efficient detection of nitrite ions. J Environ Manage. 2020;262:110282.
- Yu Q, Guo J, Muhammad Y, et al. Mechanisms of enhanced hexavalent chromium removal from groundwater by sodium carboxymethyl cellulose stabilized zerovalent iron nanoparticles. J Environ Manage. 2020;276:111245.
- Guo JF, Huo DQ, Yang M, et al. Colorimetric detection of Cr (VI) based on the leaching of gold nanoparticles using a paper-based sensor. Talanta. 2016;161:819–825.
- Wu B, Wang M, Sun Y, et al. Near-infrared chirality of plasmonic metasurfaces with gold rectangular holes. Adv Compos Hybrid Mater. 2022;5(3):2527–2535.
- Yuan M, Feng X, Yan TH, et al. Superparamagnetic iron oxide-enclosed hollow gold nanostructure with tunable surface plasmon resonances to promote near-infrared photothermal conversion. Adv Compos Hybrid Mater. 2022;5(3):2387–2398.
- Kahandal AW, Sharma L, Sirdeshmukh V, et al. A sensitive image-based optical detection of heavy metal ions using green synthesized silver nanoparticles. Int J Environ Sci Technol. 2022;1–12. DOI:10.1007/s13762-022-04539-4
- Shrivas K, Kant T, Patel S, et al. Inkjet-printed paper-based colorimetric sensor coupled with smartphone for determination of mercury (Hg2+). J Hazard Mater. 2021;414:125440.
- Swain KK, Balasubramaniam R, Bhand S. A portable microfluidic device-based Fe3O4-urease nanoprobe-enhanced colorimetric sensor for the detection of heavy metals in fish tissue. Prep Biochem Biotechnol. 2020;50(10):1000–1013.
- Chen W, Lin H, Wu Y, et al. Fluorescent probe of nitrogen-doped carbon dots derived from biomass for the sensing of MnO4− in polluted water based on inner filter effect. Adv Compos Hybrid Mater. 2022;5(3):2378–2386.
- Yuan H, Peng J, Ren T, et al. Novel fluorescent lignin-based hydrogel with cellulose nanofibers and carbon dots for highly efficient adsorption and detection of Cr(VI). Sci Total Environ. 2021;760:143395.
- Song Y, Qi N, Li K, et al. Green fluorescent nanomaterials for rapid detection of chromium and iron ions: wool keratin-based carbon quantum dots. RSC Adv. 2022;12(13):8108–8118.
- Kumar R, Umar A, Kumar R, et al. Spindle-like Co3O4-ZnO nanocomposites scaffold for hydrazine sensing and photocatalytic degradation of rhodamine B dye. Eng Sci. 2021;16:288–300.
- Wu M, Jing T, Tian J, et al. Synergistic effect of silver plasmon resonance and pn heterojunction enhanced photoelectrochemical aptasensing platform for detecting chloramphenicol. Adv Compos Hybrid Mater. 2022;5(3):2247–2259.
- Guo L, Zhang Y, Zheng J, et al. Synthesis and characterization of ZnNiCr-layered double hydroxides with high adsorption activities for Cr(VI). Adv Compos Hybrid Mater. 2021;4(3):819–829.
- Wu Q, Gao L, Huang M, et al. Aminated lignin by ultrasonic method with enhanced arsenic (V) adsorption from polluted water. Adv Compos Hybrid Mater. 2022;5(2):1044–1053.
- Xie X, Gao H, Luo X, et al. Polyethyleneimine-modified magnetic starch microspheres for Cd (II) adsorption in aqueous solutions. Adv Compos Hybrid Mater. 2022;5(4):2772–2786.
- Yin C, Wang C, Hu Q. Selective removal of as (V) from wastewater with high efficiency by glycine-modified Fe/Zn-layered double hydroxides. Adv Compos Hybrid Mater. 2021;4(2):360–370.
- Yin H, Zhong W, Yin M, et al. Carboxyl-functionalized poly (arylene ether nitrile)-based rare earth coordination polymer nanofibrous membrane for highly sensitive and selective sensing of Fe3+ ions. Adv Compos Hybrid Mater. 2022;5(3):2031–2041.
- Ding R, Cheong YH, Ahamed A, et al. Heavy metals detection with paper-based electrochemical sensors. Anal Chem. 2021;93(4):1880–1888.
- Kuswandi B, Hidayat MA, Noviana E. Paper-based sensors for rapid important biomarkers detection. Biosens Bioelectron: X. 2022;12:100246.
- Peixoto S, Machado A, Oliveira HP, et al. Paper-based biosensors for analysis of water. IntechOpen. 2019. DOI:10.5772/intechopen.84131
- Yao Z, Coatsworth P, Shi X, et al. Based sensors for diagnostics, human activity monitoring, food safety and environmental detection. Sens Diagn. 2022;1(3):312–342.
- Devadhasan JP, Kim J. A chemically functionalized paper-based microfluidic platform for multiplex heavy metal detection. Sensors And Actuat B Chem. 2018;273:18–24.
- Deshpande P, Alset U, Mehta H, et al. 2021July. Detection of hexavalent chromium concentration in aqueous solution using lab developed colorimeter. International journal of physics: conference series, (Vol. 19643)p. 032005. IOP Publishing. DOI:10.1088/1742-6596/1964/3/032005
- Ferreira F, Luxardi G, Reid B, et al. Real-time physiological measurements of oxygen using a non-invasive self-referencing optical fiber microsensor. Nat Protoc. 2020;15(2):207.
- Lewińska I, Speichert M, Granica M, et al. Colorimetric point-of-care paper-based sensors for urinary creatinine with smartphone readout. Sensors And Actuat B Chem. 2021;340:129915.
- Gong X, Liu Y, Yang Z, et al. An “on-off-on” fluorescent nanoprobe for recognition of chromium(VI) and ascorbic acid based on phosphorus/nitrogen dual-doped carbon quantum dot. Anal Chim Acta. 2017;968:85–96.
- Salimi F, Kiani M, Karami C, et al. Colorimetric sensor of detection of Cr (III) and Fe (II) ions in aqueous solutions using gold nanoparticles modified with methylene blue. Optik. 2018;158:813–825.
- Venketeswaran A, Lalam N, Wuenschell J, et al. Recent advances in machine learning for fiber optic sensor applications. Adv Intell Syst. 2022;4(1):2100067.
- Aliyana AK, Naveen Kumar SK, Marimuthu P, et al. Machine learning-assisted ammonium detection using zinc oxide/multi-walled carbon nanotube composite based impedance sensors. Sci Rep. 2021;11(1):24321.
- Pyo J, Hong SM, Kwon YS, et al. Estimation of heavy metals using deep neural network with visible and infrared spectroscopy of soil. Sci Total Environ. 2020;741:140162.
- Sang L, Wang Y, Zong C, et al. Machine learning for evaluating the cytotoxicity of mixtures of nano-TiO2 and heavy metals: qSAR model apply random forest algorithm after clustering analysis. Molecules. 2022;27(18):6125. DOI:10.3390/molecules27186125
- Zhou W, Yang H, Xie L, et al. Hyperspectral inversion of soil heavy metals in three-river source region based on random forest model. Catena. 2021;202:105222.
- Sunatkari AL, Talwatkar SS, Tamgadge YS, et al. Surfactant-dependent thermally induced nonlinear optical properties of l-ascorbic acid-stabilized colloidal GNPs and GNP–PVP thin films. RSC Adv. 2019;9(27):15502–15512.
- Liu L, Leng Y, Lin H. Photometric and visual detection of Cr(VI) using gold nanoparticles modified with 1,5-diphenylcarbazide. Mikrochim Acta. 2016;183(4):1367–1373.
- Guo X, Huang J, Wei Y, et al. Fast and selective detection of mercury ions in environmental water by paper-based fluorescent sensor using boronic acid functionalized MoS2 quantum dots. J Hazard Mater. 2020;381:120969.
- Mukherjee S, Bhattacharyya S, Ghosh K, et al. Sensory development for heavy metal detection: a review on translation from conventional analysis to field-portable sensor. Trends Food Sci Technol. 2021;109:674–689.
- Dong J, Carpinone PL, Pyrgiotakis G, et al. Synthesis of precision gold nanoparticles using Turkevich method. Kona: Powder Sci Technol In Japan. 2020;37(0):224.
- Chen R, Shi J, Liu C, et al. In situ self-assembly of gold nanorods with thermal-responsive microgel for multi-synergistic remote drug delivery. Adv Compos Hybrid Mater. 2021;5(3):2223–2234.
- Ali Dheyab M, Abdul Aziz A, Jameel MS, et al. Rapid sonochemically-assisted synthesis of highly stable gold nanoparticles as computed tomography contrast agents. Appl Sci. 2020;10(20):7020.
- Botteon CEA, Silva LB, Ccana-Ccapatinta GV, et al. Biosynthesis and characterization of gold nanoparticles using Brazilian red propolis and evaluation of its antimicrobial and anticancer activities. Sci Rep. 2021;11(1):1974.
- Clayton KN, Salameh JW, Wereley ST, et al. Physical characterization of nanoparticle size and surface modification using particle scattering diffusometry. Biomicrofluidics. 2016;10(5):054107.
- Hospodárova V, Singovszka E, Stevulova N. Characterization of cellulosic fibers by FTIR spectroscopy for their further implementation to building materials. American J Anal Chem. 2018;09(06):303–310.
- Panggabean AS, Yusuf B. Determination of Chromium (VI) by using chitosan-1, 5-diphenyl carbazide resin modified at the preconcentration system with column method. Int J Pharm Biol Sci. 2015;6(3):101–111.
- Faham S, Khayatian G, Golmohammadi H, et al. A paper-based optical probe for chromium by using gold nanoparticles modified with 2,2’-thiodiacetic acid and smartphone camera readout. Mikrochim Acta. 2018;185(8):374.
- Ricardo S, Castro Carneiroa M, Inês Couto Monteiro M, et al. Simultaneous speciation of chromium by spectrophotometry and multicomponent analysis. Chem Speciat Bioavailab. 2009;21(3):153–160.
- Apostol I, Krull I, Kelner D. Analytical method validation for biopharmaceuticals. Anal Chem. 2012. DOI:10.5772/52561. IntechOpen.
- Chen W, Fang X, Li H, et al. A simple paper-based colorimetric device for rapid mercury (II) assay. Sci Rep. 2016;6(1):1–7.
- Han J, Kamber M, Pei J. Chapter 3-data preprocessing. Data Mining Third Edition ed. United States: Morgan Kaufmann, Elsevier; 2012. pp. 83–124.