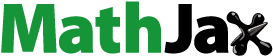
Abstract
Low production speed is a limiting factor for wider adoption of Powder Bed Fusion – Laser Beam (PBF-LB). The build rate can be increased by scaling up main process parameters, i.e., layer thickness, scan speed, and hatch distance. However, increased build speed leads to low quality in terms of density, surface finish, and mechanical properties. This study analyses size, orientation, and shape characteristics of 316 L with three- and four-factor increases in hatch distance and layer thickness beyond the state of the art to 270 and 80 µm. I-optimal designs were employed to create empirical models relating porosity level and processing parameters. The influence of overlapping melt pools on aspect ratio, size, and orientation of the pores were elucidated by analysis of optical micrographs of etched cross-sections. The lack-of-fusion pores generated by high layer thicknesses and hatch distances are preferentially orientated with respect to the build direction. With given layer thickness, the porosity level abruptly increases at a threshold of hatch distance, exceeding 1% areal fraction by 2D image analysis. Within the experimented region, the maximum allowable hatch distance for achieving <1% porosity decreased from 200 to 160 µm as the layer thickness increased from 20 to 80 µm.
1. Introduction
Powder bed fusion – laser beam (PBF-LB) is one of the most mature metal additive manufacturing (AM) processes. It utilizes a fine laser beam that selectively melts metal powder in a layer by layer fashion to manufacture 3D components based on computer aided designs (CAD) (DebRoy et al., Citation2018; Herzog, Seyda, Wycisk, & Emmelmann, Citation2016; Sun, Brandt, & Easton, Citation2017). The PBF-LB process allows manufacturing of complex structures that are otherwise not possible to make via conventional methods. The PBF-LB process has been successfully applied to process many types of metals and alloys such as steels (Haghdadi, Laleh, Moyle, & Primig, Citation2021), aluminum (Rometsch, Zhu, Wu, & Huang, Citation2022), titanium (Cao, Zou, Lim, & Wu, Citation2021; Tshephe, Akinwamide, Olevsky, & Olubambi, Citation2022), and nickel-based superalloys (Sanchez et al., Citation2021), achieving nearly full densification and good mechanical properties (Herzog et al., Citation2016). Currently, the PBF-LB process is mostly applied as niche technology in industries such as the aerospace, the energy, the medical, or the high-end automotive industry (Vafadar, Guzzomi, Rassau, & Hayward, Citation2021) where the benefits of geometrical freedom, weight reduction, and customization outweighs the high production costs.
For the PBF-LB technology to be adopted to a wider range of industries, the cost of production must be reduced by certain means. The cost can be reduced by boosting the production speed which depends on the three key process parameters in case of most commonly used single-laser systems, i.e., the layer thickness, scan speed, and hatch distance. By using larger layer thicknesses, the number of layers needed to manufacture a certain volume of material is reduced and hence increasing the build speed (Wang et al., Citation2017). Increasing the scan speed and hatch distance (distance between adjacent laser scan vectors) reduces the time needed to process each deposition layer, which also speeds up the process. In general, increasing the build speed by changing the process parameters comes at the sacrifice of build quality, such as density, surface finish, and mechanical performance (Kose, Jin, & Peng, Citation2020; Paradise et al., Citation2022).
The microstructure and state of defects in the PBF-LB processed 316 L both contribute to the mechanical behavior of the material. The PBF-LB processed 316 L typically feature columnar grains aligned in the build direction, fine grain size, and cellular subgrains, and usually shows excellent combination of strength and ductility in as-built condition (Leicht, Rashidi, Klement, & Hryha, Citation2020) due to the hierarchical strengthening by microstructural features at various scales. The large amount of high-angle grain boundaries, the cellular walls enriched with molybdenum and chromium and the high dislocation density hinder the movement of dislocations and thereby result in high strength. When the processing conditions of PBF-LB are fixed, microstructural differences upon different heat treatment governs the mechanical behavior of the material (Ronneberg, Davies, & Hooper, Citation2020). For instance, Ronneberg et al. (Citation2020), identified that the level of reduction in yield strength was connected to the removal of hierarchical microstructural features as defined by three temperature regions. Region of recovery (25–750 °C) removes dislocations, homogenization (750–1120 °C) dissolves cell walls and melt pool boundaries and finally the annealing region (>1120 °C) incurs grain growth which significantly reduces the yield strength. Conversely, pore characteristics, in particular size, distribution, and orientation, influence the ductility depending on the relation to the applied load (Choo et al., Citation2021; Ronneberg et al., Citation2020). For pores with sharp corners, when the longitudinal direction of the pore is aligned with the loading direction, the influence of pores on mechanical properties is minimal, but is significant when the loading direction is nearly perpendicular to the longitudinal direction of the pores as they induce premature crack initiation (Choo et al., Citation2021; Ronneberg et al., Citation2020).
Therefore, knowledge about the pore characteristics including size, shape, and orientations is necessary for prediction of part performance. Characterization of the pores is generally performed by analysis of 2D images of the sample cross-sections, and sometimes with the aid of 3D pore visualization via X-ray computed tomography (X-CT). Although 2D imaging of a statistically significant large area can be useful for characterizing the pore size and shape distribution, 3D pore characterization via X-CT is more accurate and thorough as many features can be missed by 2D observations (Choo et al., Citation2021; Du Plessis, Citation2019; Snell et al., Citation2020).
Previous studies have established correlation between the process parameters and the porosity present in the built part (Du Plessis, Citation2019; Kan et al., Citation2022; Oliveira, LaLonde, & Ma, Citation2020). To connect process parameters and quality, the combined process parameter, referred to as volumetric energy density (VED, J/mm3), that is the ratio of laser power over the product of layer thickness, scan speed and hatch distance, is commonly used to represent the amount of thermal energy imparted by the laser to unit volume of material, although it is not physically meaningful (Scipioni Bertoli, Wolfer, Matthews, Delplanque, & Schoenung, Citation2017). With excessive energy inputs, often created by low scan speed and large laser power, melt pools become deep and unstable. In such cases, evaporated metal at the bottom of the melt pool does not have sufficient time to escape from the top surface of the melt pool before solidification, leaving spherical pores with diameters of up to 100 µm which are referred to as keyhole pores (Kan et al., Citation2022). Conversely, low VED leads to lack of bonding of the melt pools to the material underneath or adjacent melt tracks, featuring sharp corners and irregular shape, and are referred to as lack-of-fusion (LOF) type of pores (Kan et al., Citation2022). While VED as a parameter for distinguishing the different regimes of process is simple and straightforward, it is not a physics-based quantity. As suggested by Scipioni Bertoli et al. (Citation2017), VED fails to capture the complex physics in the melt pool and should be used with caution. To add physical meaning and more universally applicability Thomas, Baxter, and Todd (Citation2016), introduced the normalized energy density where material unique thermal properties such as enthalpy, latent heat, and thermal conductivity was combined with process parameters which proved to be efficient in mapping historical data with new experiments. However, the highly complex and dynamic nature of PBF-LB leads to that material related thermal properties can only be estimated and not accurately measured which at current state can question its usefulness.
Trial-and-error experiments, sometimes with guidance from process simulations, are used to optimize the individual process parameters to achieve desirable quality of the built part. Statistical tools such as Design of Experiments (DOE) and regression analysis can be used to construct predictive models for the influence of processing parameters on the quality of the parts in the PBF-LB process (Paradise et al., Citation2022). Several studies used classical full factorial DOEs the effect of main PBF-LB process parameters on certain response variables (Delgado, Ciurana, & Rodríguez, Citation2012; Krishnan et al., Citation2014; Paradise et al., Citation2022), which require a large number of experiments (2k where k is the number of independent variables) to ensure orthogonality of the design space. In addition, priori knowledge exists in literature that certain combinations of process parameters are not feasible for given materials (Smucker, Krzywinski, & Altman, Citation2018), such knowledge should be used to better design the experiments to avoid unnecessary samples that yield unsatisfactory results. Hence, DOEs that allow the experimentalists to impose such constraints might be more efficient and cost-effective. The modern custom designs such as I-optimal and D-optimal design the experimental runs under user-specified constraints, while achieving most uniform or minimized prediction variance across the design space (Goos, Jones, & Syafitri, Citation2016; Smucker et al., Citation2018).
The current work aims to reveal how porosity and its characteristics change with processing parameters of higher productivity, namely higher layer thickness, hatch distance, and scan speed compared to the state-of-the-art. I-optimal design with linear constraints specified by upper and lower limits of VED values are employed to allow economic use of experimental resources, in other words, using less specimens compared to classical full factorial designs. Regression analysis was performed to build empirical models for predicting porosity level in the built part using a function of the process parameters. Furthermore, the change of pore size, shape, and spatial distribution are characterized in detail across a wide range of layer thicknesses (20, 40, 60, and 80 µm). Particularly, the process conditions that yield similar porosity levels and build speeds are compared in terms of the size, shape, and distribution of pores generated.
2. Methods
2.1. Powder feedstock
Gas atomized 316 L stainless steel powder was provided by Höganäs AB and the chemical composition is presented in . The powder particle size distribution (20–53 µm) was measured by laser diffraction using a Mastersizer 3000 from Malvern (Malvern, UK).
Table 1. Chemical composition, in wt.%
2.2. PBF-LB experiments
10 mm × 10 mm × 10 mm cubic samples were produced on an EOS M290 equipped with an Yb-fiber laser with a maximum power of 400 W and beam diameter of 80 µm. Twenty-four samples were produced per layer thicknesses (20, 40, 60, and 80 µm) in separate builds using a hatch rotation of 67˚ between layers. During the build process a constant supply of argon gas was used and oxygen levels were kept below 1000 ppm to prevent oxidation. The sample geometry and the distribution of the samples on the build plate is presented . Samples were sectioned along the build direction (BD) and recoater direction (X) for analysis.
2.3. Design of experiments
The process parameters of interest, namely the laser power p (W), scan speed v (mm/s), and hatch distance h (µm), were varied within selected ranges (see ), utilizing the Custom Design tool in JMP Pro 16 to generate the design matrix. The Custom Design feature offers flexibility where a combination of modern statistical design tools such as D-optimal and I-optimal designs can be combined with linear constraints (disallowed combinations). Linear constraints are useful to exclude parameter combinations in process space that are known to cause issues during the production process. During the PBF-LB process excessive heat input can result in swelling or spatter formation that can ultimately impact other components in the build. Furthermore, insufficient energy density can lead to lack of bonding between subsequent layers and cause lack of fusion defects. Therefore, parameter combinations resulting in too high or too low energy density can be excluded without jeopardizing the statistical validity of the design. Based on previous research lower and upper constraints were defined in terms of VED that were different at each layer thickness (see, ). Different constraints were utilized as process windows based on VED changes when altering layer thickness (Leicht, Fischer, Klement, Nyborg, & Hryha, Citation2021). The layer thickness enforces significant scaling of the VED meaning that VED as a parameter design criteria is not universally applicable across different layer thickness values.
Table 2. Process parameter ranges to be varied at each layer thickness 20, 40, 60, and 80 µm
Table 3. Upper and lower constraints utilized at each layer thickness in terms of VED (J/mm3)
Based on these constraints an I-optimal design space was constructed at 20, 40, 60, and 80 µm layer thicknesses, respectively. The I-optimal design distributes few data points at extremes and focuses on areas in between the center and the extremes of the selected parameter range. Therefore, I-optimal designs are typically used when acquiring a response surface (regression model) and is more cost-effective than full factorial designs as it requires less data points to evaluate the influence of investigated parameter combinations.
The I-optimal design matrix of each print is presented in . According to chosen constraints, combinations of p, v, and h that yield too high or too low VED values are excluded. It can be seen in that the VED constraints affect the DOE for 20 µm by only excluding the high VED regions (combinations of high p and low v, and low v and low h). Conversely, the VED constraints only affect the DOE for 40 µm, 60 µm, and 80 µm by excluding low VED regions (combinations of high v and high h, high h and low p, high v and low p). To capture uncertainty of measurements replicated conditions were added across layer thicknesses.
Figure 2. Graphical representation of the design space separated by layer thickness (20–80 µm). The upper (red) and lower (blue) constraints for VED defined in restrict the design space.

Regression analysis was performed by defining porosity (%) as a function of the main printing parameters; laser power p, hatch distance h, and the scan speed v (see EquationEq. (1)(1)
(1) ). Standard least square method was used to fit the regression functions with linear, quadratic, and second-order interaction terms of the three input variables.
(1)
(1)
The productivity of each process condition was evaluated by the build rate (BR) by multiplying scan speed v, hatch distance h and layer thickness t converted from mm3/s × 3.6 to cm3/h (see EquationEq. (2)(2)
(2) ). The BR only refers to the sequence when the laser is in operation and melting powder. It does not consider the number of layers nor the total time for recoating new powder but provides an estimation of the unit volume of material that can be manufactured per hour given certain combinations of scan speed, hatch distance and layer thickness.
(2)
(2)
To prepare for microstructural characterization, the produced samples were removed from the build plate by electric discharge machining (EDM). The individual samples were sectioned by a Buehler Isomet 2000 precision saw parallel to the BD-X plane, see . Samples were mounted in Polyfast and ground using SiC foil on a Struers TegraPol (grit size 320-2000). Fine polishing was carried out down to 1 µm diamond suspension to achieve mirror-finish.
To reveal the microstructure and more specifically melt pool boundaries, the samples underwent electrochemical etching in 10% oxalic acid with a constant potential of 3 V and a platinum cathode. The melt pool width (µm) and depth (µm) were estimated and averaged across 30 melt pools at the top surface of each sample.
2.4. Optical microscopy and image analysis
Light optical micrographs were captured by a Zeiss Axioscope 7 at × 50 magnification giving a pixel to distance ratio (pix/µm) of 1.14. The microscope was set by the proprietary software to take images in sequence, covering a large area. These images were montaged to form a large micrograph covering a sampled 5 × 5 mm area. The images were saved in 8-bit grayscale format (gray value ranging from zero to 255) and evaluated using the MATLAB image processing toolbox and the porosity level was calculated on binary images using a 170 threshold in gray value for consistency. Shape descriptors of interest, namely orientation (˚), major axis length (µm), minor axis length (µm), and aspect ratio of each pore, was calculated by the ‘regionprops’ command in MATLAB on features larger than 20 µm2. presents how these shape descriptors are correlated to each image and pore.
3. Results and discussion
3.1. Pore content
The area fraction of porosity (%) is presented in against the values of VED. On a global scale porosity decreases from approximately 16.5%, in the highlighted low VED region (<40 J/mm3), to porosity levels below 0.2% between 40 and 100 J/mm3 depending on the layer thickness. However, a wide spread in measured porosity is observed at the same VEDs. In the highlighted region, differences of up to approximately 7% porosity is seen at the same VED and layer thickness. At 20 J/mm3 the highest measured porosity of approximately 16% had a parameter combination of 270 µm hatch distance (largest in the DOE), scan speed of 600 mm/s (lowest in DOE) and the largest used laser power of 280 W. At the lowest porosity (∼11.2%) measured at the same VED and layer thickness a parameter combination of 280 W laser power, 960 mm/s scan speed, and 120 µm hatch distance was used. Thus, the reduced porosity is associated with smaller hatch distance rather than the impact of laser power and scan speed. Similarly, from 0.0% to 1.0% porosity large variations in porosity is measured at similar VEDs. The variations partly originate from the measurement uncertainties (the maximum difference between replicate samples is 1.32%), but also signifies that VED cannot be used as the sole criteria for evaluating the process parameters. The standard deviations of replicated conditions are presented in of the Appendix. Standard deviation increases with the level of porosity between replicates and the largest difference between replicated samples was found at 270 µm hatch distance and 40 µm layer thickness.
3.2. Regression analysis
As the experiments were not designed to change one factor at a time, there are no clear trends in which the porosity content changes with a single parameter. Predictive modeling through linear regression is useful for capturing the influence of input parameters and the interaction between parameters on the porosity content. The regression function can then be used to identify the appropriate combinations of process parameters for achieving desirable porosity.
Linear regression was performed at separate layer thicknesses to predict the porosity content as a function of laser power, scan speed, and hatch distance according to the expression defined in EquationEq. (1)(1)
(1) . The contour surfaces are plotted in corresponding to the regression functions to show the influence of process parameters on the predicted relative density (%) (1 - porosity level). For a given layer thickness, a higher laser power (280 W) expands the regions of high relative density in the contour surfaces compared to the case of 200 W, meaning one can choose to operate at higher productivity (high scan speed, and large layer thickness) while achieving desirable densification. For example, if ≥99.9% relative density is required and a 20 µm layer thickness chosen, one can operate at 210 µm hatch distance and 1300 mm/s speed using a laser power of 280 W (), instead of 180 µm hatch distance and 1100 mm/s scan speed using a laser power of 200 W (), which is significantly slower.
Figure 5. Contour surfaces of variation in relative density (%) as a function of laser power, scan speed, and hatch distance at 20, 40, 60, and 80 µm layer thickness separately.

Moreover, the process window with relative density ≥99.9% significantly shrinks as the layer thickness increases. With a laser power at 200 W, there is no possibility of achieving ≥99.9% relative density according to the contour surfaces for layer thickness of 60 µm and 80 µm (), and only limited to the corner of relatively low scan speed and small hatch distance when the laser power is 280 W (). This is consistent with previous work (Leicht et al., Citation2020) as the remelting of previous layers is reduced by a factor of 4 considering an increase from 20 µm to 80 µm layer thickness.
To check the validity of the regression models of , the predictions are compared to actual measurements. The predicted contour surface at 80 µm layer thickness and 280 W is presented in , where data representing actual density measurements with their respective micrographs and build rate. The experimental points in are mostly located at the boundaries of the contour surface, and one point located at the center where a 89% relative density is measured in the band between 90% and 80% predicted relative density. The curvature of the contour lines signifies that there are interaction effects between scan speed and hatch distance, meaning the setting of one parameter affects how relative density changes with the other. This interaction is also present in the regression results of a large range of laser powers (195–280 W). The actual measurements at 20, 40, and 60 µm layer thicknesses also show good agreement with their respective prediction model. Plots like can be found in the Appendix. It should be considered that the linear constraints set at 20 µm layer thickness in the DOE prevents data points with VED above 100 J/mm3. Hence, the lower regions (low scan speed, small hatch distance) at 280 W laser power are outside of the experimented region. It is expected that in this region keyhole type of porosity would appear due to excessive energy input, which reduces the relative density. The predictions are well aligned with actual densities within the region covered by experiments, suggesting model accuracy. With the small number of samples produced in this experiment (24 samples at each layer thickness), the methodology taken in the current work produced rich information about the process window. The contour surfaces in , as compared to the porosity versus VED plots in , is more informative for choosing appropriate parameters.
Figure 6. Comparison of predicted density at 80 µm layer thickness and micrographs of measured samples illustrating the accuracy of the regression model. Observations of how the porosity changes at different build rates cm3/h can be seen in each micrograph. Each micrograph represents a 5 mm × 5 mm area of sample cross-sections parallel with the build direction (BD).

3.3. Pore characteristics
not only shows the pore content of the bulk sample, but also shows the size, shape, and orientations of the pores. The build rate is simply defined as the product of the scan speed, hatch distance and layer thickness, also noted on the micrographs. One can compare the differences in pore characteristics where similar build rates are achieved. When a low scan speed of 600 mm/s and small hatch distance of 90 µm are used () the sample is almost free of pores, showing scarcely distributed spherical pores. The adjacent micrographs () show two conditions where a 28 cm3/h is achieved, an 80% improvement in build rate compared to the 15 cm3/h. More pores are found in the condition with large hatch distance and lower scan speed (), although the build rates are almost identical. As the process departs further (∼120% increase in build rate) from the fully dense condition in , the two conditions in ,e are showing porosity content and build rate of similar magnitude but are totally different in terms of pore characteristics. In , there are larger individual pores with major axes aligned horizontally, whereas in the pores are of smaller individual size and are aligned along the build direction. A detailed description of these differences is necessary as it may have an impact on mechanical properties (Choo et al., Citation2021; Ronneberg et al., Citation2020).
Image analysis was carried out to micrographs of 5 mm × 5 mm area as shown in to extract useful information to distinguish samples of different pore characteristics. The orientation of the pores was defined by the angle between the major axis of the pore and the horizontal axis (perpendicular to the build direction). Such angle provides indications of preferential alignment of pores that could yield anisotropic behavior (Ronneberg et al., Citation2020). In addition, the aspect ratio was defined as the ratio between the minor and major axis length of each pore. The sizes of pores were shown as the major axis length.
illustrates that there are clear differences in the pore characteristics between conditions of high build rate reached through increased hatch distance () and increased scan speed (). This is shown by the average orientation of pores larger than 100 µm being 70˚ meaning that they are close to alignment with the build direction (). In comparison, the orientation angle at increased scan speed is 25˚ which means a pore orientation perpendicular to the build direction of the sample (). The anisotropy of mechanical properties for PBF-LB 316 L is highly dependent on microstructural features, i.e., crystallographic texture, grain size, dislocation density, etc. (Leicht et al., Citation2020). In addition, Choo et al. (Citation2021), showed that pores oriented perpendicular to the load direction had inferior yield strength and ductility compared to parallelly oriented pores. Thus, presumably the manner of increasing the build rate affects the anisotropy of mechanical properties by altering the pore orientation. Consequently, the choice of parameter for increased build rate should take into consideration the load case the final application.
Figure 7. Difference in pore characteristics at conditions of high build rates (a) 33 cm3/h by increased hatch distance 190 µm and (b) 36 cm3/h by increased scan speed 1380 mm/s, as described by size, aspect ratio, and the orientation of each pore with respect to the build direction.

and present the micrographs of selected samples and the plots of pore characteristics. The DOE used in this work is not a typical one-factor-at-a-time design, i.e., it was not intended to vary layer thickness or hatch distance while keeping other parameters constant (see ). Therefore, the samples are selected to show the typical pore characteristics as hatch distance and layer thickness are increased, but not to strictly compare one sample to another.
Figure 8. (a–c) Micrographs of selected samples built with 40 µm layer thickness. The porosity contents and the build rates are indicated in the micrographs. (d–f) Corresponding scatter plots of pore size, aspect ratio, and pore orientation angles (shown by colormap).

Figure 9. (a–c) Micrographs of selected samples built with 80 µm layer thickness. The porosity contents and the build rates are indicated in the micrographs. (d–f) Corresponding scatter plots of pore size, aspect ratio, and pore orientation angles (shown by colormap).

When the hatch distance is 90 µm, there is no observable preference in pore orientations, as most of the pores are below 50 µm in size for both samples made with 40 µm and 80 µm layer thicknesses respectively ( and ). As the hatch distance is increased from 90 µm to 160 µm, the pores become more elongated as a larger population of the pores show aspect ratios deviating farther from one ( and ). There is also a shift in pore orientation, as most of the larger pores tend to have a larger orientation angle, indicating the larger pores are aligned with the build direction. This effect is most pronounced as the layer thickness and hatch distance are both increased as shown in , the larger pores (>,100 µm) of aspect ratio smaller than 0.5 are predominantly aligned along the build direction, with orientation angle close to 90 degrees. The preferential orientation of pores at increased hatch distance is less significant for the cases of 40 µm layer thickness (160 µm to 200 µm hatch distance, ) as compared to the cases of 80 µm layer thickness (160 µm to 190 µm hatch distance, ). The sample cross-section shown in with 80 µm layer thickness exhibits higher porosity content (%), individual pore size, and more pronounced preferential orientation of the pores along the build direction.
3.4. Melt pool characteristics
To understand how pores of various characteristics are formed in the built parts with increased layer thickness and hatch distance, selected samples are etched to reveal the morphologies of the melt pools. In the etched microstructure near the top surfaces (last processed layers) are shown. All samples shown in are processed with a laser power of 280 W and a scan speed of 600 mm/s. The morphologies of the melt pools appear similar across different layer thickness and hatch distance used. It is conceivable that as hatch distance increases the overlapping between neighboring scan tracks decreases, which can lead to un-melted gaps between adjacent tracks causing lack of fusion pores. In the case of 160 µm hatch distance and 80 µm layer thickness, small triangular shaped pores are identified near the waist of the melt pools (indicated in ). As the hatch distance further increases to 190 µm with the same layer thickness applied, the pores at the last processed layer become open to the top surface, separating individual melt pools (indicated in ). It is likely that this lack of fusion between the neighboring melt tracks are continuously formed and connected through deposition layers to form the large pores aligned in the build direction observed in . Meanwhile, with the same laser power and scan speed applied, when the layer thickness is reduced to 40 µm, such lack of bonding between adjacent scan tracks disappears. It is an interesting observation that on the last processed layer the melt pools are separate from one another, but no pores are present between these melt pools (), even though the hatch distances (230 µm and 250 µm) used is larger than those for the 80 µm layer thickness experiments. This agrees with the predictions from the regression analysis () that the use of smaller layer thickness allows the use of larger hatch distance given certain allowance for porosity contents. It seems that the layer thickness applied determines how the neighboring melt pools connect with each other. In the case of a high layer thickness at 80 µm the melt pools lose connections where the width of the melt pool is not sufficient, leaving pores beneath the topmost surface of the processed layer, as shown in . In the case of a relatively low layer thickness at 40 µm and a large hatch distance, 230 µm and 250 µm, although there is no lateral connection between the melt pools (), the gaps between the melt pools can be filled by materials deposited later.
Figure 10. Etched micrographs of selected sample cross-sections near the top surfaces, the hatch distance, and layer thickness are written alongside the micrographs. For all these samples, a laser power of 280 W and a scan speed of 600 mm/s are used.

To reveal the interplay between layer thickness and hatch distance in the formation of pores, the ratio of hatch distance over layer thickness (HD/LT) is introduced as a geometrical factor, and the ratio of laser power over scan speed (P/v) were calculated to represent the energy input in the line of scan by the laser. The porosity content is then plotted against HD/LT value in with the data grouped by layer thickness and P/v value (0.1–0.25 and ≥0.25 J/mm). The color bar and marker size represent measured melt pool depths (µm) and melt pool widths (µm), respectively.
Figure 11. Influence of laser power/scan speed (P/v) and hatch distance/layer thickness (HD/LT) on porosity (%).

By each factor of increase in the HD/LT ratio, an increase in porosity content is seen. This increase seems to depend on the P/v as the porosity is generally higher in the lower range of P/v (0.1–0.25 J/mm) as compared to the upper range (≥2.5 J/mm), since a higher P/v produces larger melt pool widths and depths (). The melt pool dimensions become more important at higher layer thickness and high hatch distance to maintain low porosity. In addition, at 40, 60, and 80 µm layer thickness clear thresholds of HD/LT are observed at which the porosity level increases rapidly. If a porosity of 1.0% is considered these thresholds would correspond to a factor of approximately 6.3, 3.5, and 2.0. Thus, within the investigated parameter range the ability to use large hatch distances while maintaining low porosity decreases with layer thickness, i.e., threshold hatch distances at 250 µm, 210 µm, 160 µm for 40 µm, 60 µm, and 80 µm layer thicknesses, respectively. It appears that at 20 µm layer thickness no clear threshold was observed. Nevertheless, the results show that a 200 µm hatch distance can be used to achieve less than 1% porosity when running the process at 20 µm layer thickness, with HD/LT at 10.
4. Conclusions
This study is motivated by the need to boost production speed in PBF-LB of 316 L by increasing the layer thickness and hatch distance. Efficient DOEs and regression analysis were employed to study how process level changes with a wide range of hatch distance at various layer thicknesses (20, 40, 60, and 80 µm). The empirical models acquired through regression analysis are presented as contour surfaces to illustrate how the main process parameters affect porosity and their interactive effects. Through image analysis, valuable information regarding the pore size, shape, distribution, and orientation relationship with the build direction are extracted from the micrographs. Based on this work the main findings can be summarized as follows:
The interplay between porosity and process parameters cannot be sufficiently described by the VED parameter. When varying multiple process parameters (layer thickness, scan speed, hatch distance, and laser power) in a wide range, up to 7.5% difference in porosity can be seen at the same VED value, this difference is mainly attributed to the wide range of hatch distance explored in this work.
The I-optimal design with linear constraints defined by upper and lower limits of VED proves to be an efficient way of acquiring experimental data for construction of empirical models for the PBF-LB process. The separate predictive models for different layer thicknesses at 20, 40, 60, and 80 µm showed good agreement with experimental results, which can be used as guidance for further optimization or flexible choice of process parameters according to the product requirements.
Approximately 120% build rate increase achieved through either increased scan speed or hatch distance led to pores perpendicular or parallel to the build direction. The choice of parameter increase should, therefore, depend on the load direction of the printed component.
The use of large hatch distance in PBF-LB processing of 316L resulted in preferential orientation of elongated pores (aspect ratio deviates far from 1) along the build direction when layer thickness is also large (80 µm). This phenomenon is not as prominent in samples built with 40 µm layer thickness. It is hypothesized that the use of smaller layer thickness incurs more remelting, which eliminates pores, or fills gaps between hatches.
Within the investigated parameter range, thresholds of sudden porosity increase (>1%) were identified at separate layer thicknesses as defined by the ratio of hatch distance over layer thickness HD/LT. As the layer thickness increases from 20 µm to 80 µm the threshold of HD/LT reduces from 10 to 2, corresponding to hatch distance reduction of 200 to 160 µm while maintaining approximately 1% porosity.
These conclusions can act as general guidelines for optimizing main process parameters for increased build speed utilizing layer thicknesses and hatch distances greater than state-of-the-art. These findings can be applied to commercially available PBF-LB systems utilizing powder of required quality and machine allowing processing using indicated parameters settings.
Disclosure statement
The authors declare that they have no known competing financial interests or personal relationships that could have appeared to influence the work reported in this paper.
Data availability
The data and image analysis are available from the authors upon request.
Additional information
Funding
References
- Cao, S., Zou, Y., Lim, C. V. S., & Wu, X. (2021). Review of laser powder bed fusion (LPBF) fabricated Ti-6Al-4V: Process, post-process treatment, microstructure, and property. Light: Advanced Manufacturing, 2(2), 1. doi:10.37188/lam.2021.020
- Choo, H., White, L. P., Xiao, X., Sluss, C. C., Morin, D., & Garlea, E. (2021). Deformation and fracture behavior of a laser powder bed fusion processed stainless steel: In situ synchrotron x-ray computed microtomography study. Additive Manufacturing, 40, 101914. doi:10.1016/j.addma.2021.101914
- DebRoy, T., Wei, H. L., Zuback, J. S., Mukherjee, T., Elmer, J. W., Milewski, J. O., … Zhang, W. (2018). Additive manufacturing of metallic components – Process, structure and properties. Progress in Materials Science, 92, 112–22. doi:10.1016/j.pmatsci.2017.10.001
- Delgado, J., Ciurana, J., & Rodríguez, C. A. (2012). Influence of process parameters on part quality and mechanical properties for DMLS and SLM with iron-based materials. The International Journal of Advanced Manufacturing Technology, 60(5–8), 601–610. doi:10.1007/s00170-011-3643-5
- Du Plessis, A. (2019). Effects of process parameters on porosity in laser powder bed fusion revealed by X-ray tomography. Additive Manufacturing, 30, 100871. doi:10.1016/j.addma.2019.100871
- Goos, P., Jones, B., & Syafitri, U. (2016). I-Optimal design of mixture experiments. Journal of the American Statistical Association, 111(514), 899–911. doi:10.1080/01621459.2015.1136632
- Haghdadi, N., Laleh, M., Moyle, M., & Primig, S. (2021). Additive manufacturing of steels: A review of achievements and challenges. Journal of Materials Science, 56(1), 64–107. doi:10.1007/s10853-020-05109-0
- Herzog, D., Seyda, V., Wycisk, E., & Emmelmann, C. (2016). Additive manufacturing of metals. Acta Materialia, 117, 371–392. doi:10.1016/j.actamat.2016.07.019
- Kan, W. H., Chiu, L. N. S., Lim, C. V. S., Zhu, Y., Tian, Y., Jiang, D., & Huang, A. (2022). A critical review on the effects of process-induced porosity on the mechanical properties of alloys fabricated by laser powder bed fusion. Journal of Materials Science, 57(21), 9818–9865. doi:10.1007/s10853-022-06990-7
- Kose, H., Jin, M., & Peng, T. (2020). Quality and productivity trade-off in powder-bed additive manufacturing. Progress in Additive Manufacturing, 5(2), 199–210. doi:10.1007/s40964-020-00122-w
- Krishnan, M., Atzeni, E., Canali, R., Calignano, F., Manfredi, D., Ambrosio, E. P., & Iuliano, L. (2014). On the effect of process parameters on properties of AlSi10Mg parts produced by DMLS. Rapid Prototyping Journal, 20(6), 449–458. doi:10.1108/RPJ-03-2013-0028
- Leicht, A., Fischer, M., Klement, U., Nyborg, L., & Hryha, E. (2021). Increasing the productivity of laser powder bed fusion for stainless steel 316L through increased layer thickness. Journal of Materials Engineering and Performance, 30(1), 575–584. doi:10.1007/s11665-020-05334-3
- Leicht, A., Rashidi, M., Klement, U., & Hryha, E. (2020). Effect of process parameters on the microstructure, tensile strength and productivity of 316L parts produced by laser powder bed fusion. Materials Characterization, 159, 110016. doi:10.1016/j.matchar.2019.110016
- Oliveira, J. P., LaLonde, A. D., & Ma, J. (2020). Processing parameters in laser powder bed fusion metal additive manufacturing. Materials and Design, 193, 108762. doi:10.1016/j.matdes.2020.108762
- Paradise, P., Patil, D., Van Handel, N., Temes, S., Saxena, A., & Bruce, D. (2022). Improving productivity in the laser powder bed fusion of inconel 718 by increasing layer thickness: Effects on mechanical behavior. Journal of Materials Engineering and Performance, 32(8), 6205–6220. doi:10.1007/s11665-022-06961-8
- Rometsch, P. A., Zhu, Y., Wu, X., & Huang, A. (2022). Review of high-strength aluminium alloys for additive manufacturing by laser powder bed fusion. Materials and Design., 219, 110779. doi:10.1016/j.matdes.2022.110779
- Ronneberg, T., Davies, C. M., & Hooper, P. A. (2020). Revealing relationships between porosity, microstructure and mechanical properties of laser powder bed fusion 316L stainless steel through heat treatment. Materials and Design, 189, 108481. doi:10.1016/j.matdes.2020.1084
- Sanchez, S., Smith, P., Xu, Z., Gaspard, G., Hyde, C. J., Wits, W. W. … Clare, A. T. (2021). Powder Bed Fusion of nickel-based superalloys: A review. International Journal of Machine Tools and Manufacture, 165, 103729. doi:10.1016/j.ijmachtools.2021.103729
- Scipioni Bertoli, U., Wolfer, A. J., Matthews, M. J., Delplanque, J. P. R., & Schoenung, J. M. (2017). On the limitations of volumetric energy density as a design parameter for selective laser melting. Materials and Design, 113, 331–340. doi:10.1016/j.matdes.2016.10.037
- Smucker, B., Krzywinski, M., & Altman, N. (2018). Optimal experimental design. Nature Methods, 15(8), 559–560. doi:10.1038/s41592-018-0083-2
- Snell, R., Tammas-Williams, S., Chechik, L., Lyle, A., Hernández-Nava, E., Boig, C. … Todd, I. (2020). Methods for rapid pore classification in metal additive manufacturing. Journal of the Minerals Metals and Materials Society, 72(1), 101–109. doi:10.1007/s11837-019-03761-9
- Sun, S., Brandt, M., & Easton, M. (2017). Powder bed fusion processes: An overview. In Laser Additive Manufacturing: Materials, Design, Technologies, and Applications. Elsevier Ltd. doi:10.1016/B978-0-08-100433-3.00002-6
- Thomas, M., Baxter, G. J., & Todd, I. (2016). Normalised model-based processing diagrams for additive layer manufacture of engineering alloys. Acta Materialia, 108, 26–35. doi:10.1016/j.actamat.2016.02.025
- Tshephe, T. S., Akinwamide, S. O., Olevsky, E., & Olubambi, P. A. (2022). Additive manufacturing of titanium-based alloys- A review of methods, properties, challenges, and prospects. Heliyon, 8(3), e09041. doi:10.1016/j.heliyon.2022.e09041
- Vafadar, A., Guzzomi, F., Rassau, A., & Hayward, K. (2021). Advances in metal additive manufacturing: A review of common processes, industrial applications, and current challenges. Applied Sciences (Sciences), 11(3), 1213. doi:10.3390/app11031213
- Wang, S., Liu, Y., Shi, W., Qi, B., Yang, J., Zhang, F. … Ma, Y. (2017). Research on high layer thickness fabricated of 316L by selective laser melting. Materials, 10(9), 1055. doi:10.3390/ma10091055
Appendix
Figure A1. Comparison of predicted density at 60 µm layer thickness and micrographs of measured samples illustrating the accuracy of the regression model. Observations of how the porosity changes at different build rates cm3/h can be seen in each micrograph. Each micrograph represents a 5 mm × 5 mm area of sample cross-sections parallel with the build direction (BD).

Figure A2. Comparison of predicted density at 40 µm layer thickness and micrographs of measured samples illustrating the accuracy of the regression model. Observations of how the porosity changes at different build rates cm3/h can be seen in each micrograph. Each micrograph represents a 5 mm × 5 mm area of sample cross-sections parallel with the build direction (BD).

Figure A3. Comparison of predicted density at 20 µm layer thickness and micrographs of measured samples illustrating the accuracy of the regression model. Observations of how the porosity changes at different build rates cm3/h can be seen in each micrograph. Each micrograph represents a 5 mm × 5 mm area of sample cross-sections parallel with the build direction (BD).

Table A1. Parameters with replicates based on three samples of each condition.