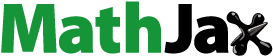
ABSTRACT
Crop residue burns typically result in particulate matter (PM2.5), methane (CH4), carbon monoxide (CO), nitrous oxide (N2O), nitrogen oxides (NOx), volatile organic carbon (VOC), and black carbon emissions, which affect air quality and can pose a risk to public health. Currently, Arkansas farmers self-regulate crop burning using voluntary smoke management guidelines to reduce community impacts from smoke by ensuring burns take place in optimal conditions. The aim of this study is to identify burned cropland areas and examine human-caused fire PM2.5 emissions and dispersion during optimal burn conditions, specifically within Mississippi County, Arkansas, USA, using two separate methods. During the 2019 harvest season, high-resolution satellite data was used to manually identify burned areas and crop types. The total cumulative cropland burned area in 2019 was estimated to be 7,137 acres (29.03 km2). Burning harvested rice fields accounted for approximately 35% of the total annual PM2.5 emissions from all annual agricultural burning as reported in the 2017 U.S. EPA National Emissions Inventory, while PM2.5 emissions from burning corn fields were only 8% of the total estimated annual PM2.5 emissions. Approximately 43% of annual agricultural burning PM2.5 emissions occurred between 15 August and 23 October in Mississippi County. These high-resolution burned areas were not captured in the standard coarse resolution active fire products. Secondly, during the 2020 fall harvest season, we measured PM2.5 emissions using a Purple Air sensor and modeled smoke dispersion from a planned burn of rice fields following state-level voluntary guidelines. Additionally, the smoke transport model HYSPLIT was deployed to model this planned burn. The HYSPLIT results suggest that smoke disperses into the atmosphere from burns following the guidelines, limiting ground-level human exposure under optimal burning conditions.
Implications: Fire has long been used as a cropland management tool to control weeds and invasive species and remove residues between crop rotations. Residue burns produce emissions that can present public health concerns. This study presents a novel attempt to quantify cropland burning with satellite imagery, estimate emission inventories by crop type, and simulate particulate matter dispersion from in-field burns. The results of this two-phase analysis show that our understanding and quantification of human-caused cropland burning and emissions can still be improved and integrated into management approaches as well as emission inventories.
1. Introduction
Though burning quickly removes leftover residues that can decrease nutrient uptake, it can damage organic matter in soils and release harmful pollutants into the atmosphere (Desrochers et al., Citation2020). In the southeastern United States, prescribed burns are frequently used for crop residue management. Prescribed burns allow farmers to quickly clear fields of unwanted residues after one harvest before planting for the next season. Double crop farming is a common practice in which more than one harvest is taken from the same field within 1 year. McCarty et al. (Citation2007) estimated that around one-third of all agricultural burns in the contiguous United States take place in the southeastern states. Of that one-third, more than three-quarters take place in Florida, Louisiana, and Arkansas. Farmers frequently burn the remaining residue of soybeans, rice, winter wheat, sugarcane, and corn (Haiyan et al., Citation2017; McCarty, Citation2011). Burning is dependent upon the crop rotation, harvesting schedule, and whether the use of double-cropping is implemented.
Satellite imagery and remote sensing techniques have been applied to quantify burned areas, specifically agricultural burning and emissions, in many regions of the world (Lasko et al., Citation2017; Shyamsundar et al., Citation2019; Vadrevu et al., Citation2011). However, such research on intentional agricultural burning for the contiguous U.S. has been limited to a few studies (McCarty, Citation2011; McCarty et al., Citation2007, Citation2009). Most remote sensing estimations of agricultural fire activity use active fires from the Moderate Resolution Imaging Spectroradiometer (MODIS) satellite constellation because of its revisit frequency (Huang et al., Citation2012; Korontzi et al., Citation2006; Li et al., Citation2016; Roy et al., Citation2008).
In 1997, the Environmental Protection Agency (EPA) determined that the fire particles contained in smoke (commonly called PM2.5) can have health effects when breathed at high concentrations and also contribute to haze that sometimes reduces visibility (US EPA, Citation1997). For these reasons, the EPA established the National Ambient Air Quality Standards (NAAQS) for PM2.5 and other pollutants. Health risks have been associated with exposure to emissions from fires (Cascio, Citation2018). In recent national surveys, 40% of the respondents have indicated that air quality and smoke are the main concerns relating to prescribed fires in the United States (Starns et al., Citation2020). Toxic emissions which can be released from agricultural burning include particulate matter (PM2.5), methane (CH4), carbon monoxide (CO), nitrous oxide (N2O), nitrogen oxides (NOx), volatile organic carbon (VOC), and black carbon (US EPA, Citation2021). Other emissions include organic aerosols and other toxins which result from photoreactions within the ozone (Cascio, Citation2018). Many of the toxins that have been mentioned here are pollutants that are monitored by the NAAQS and regulated by the U.S. Environmental Protection Agency (US EPA; Pouliot et al., Citation2016).
Based on the 2010 U.S. Census population estimates, approximately 21 million people in the United States live in areas where the air quality was worse than the NAAQS for PM2.5 under the 2012 update (US EPA, Citation2022). Rappold et al. (Citation2017) estimated that 29.7% of the population (91.1 million) live in areas with an annual average fire-PM2.5 between 0.75 and 1.5 µg/m3, while nearly 10% of the population (30.5 million) live in areas where the annual average ambient PM2.5 was above 1.5 µg/m3. Additionally, 82.4 and 10.3 million individuals experienced moderate air quality due to fire-PM2.5 (daily PM2.5 between 15 and 35 µg/m3) and 10.3 million individuals experienced unhealthy air quality levels (daily fire-PM2.5 >35 µg/m3) for more than 10 days between 2008 and 2012 (Rappold et al., Citation2017). Therefore, wildfire smoke impacts local and regional air quality and presents a challenge to protecting public health. Reviews have shown that there is a positive association between smoke exposure, often associated with PM2.5, and respiratory diseases, including but not limited to bronchitis, pneumonia, asthma, and Chronic Obstructive Pulmonary Disease (COPD), as well as pregnancy complications (Heft-Neal et al., Citation2021; Reid et al., Citation2016; Rutlen et al., Citation2021). However, more research is needed to consider how cardiovascular diseases such as stroke, rhythm disturbances, and heart failure can be related to emissions exposure as well (Cascio, Citation2018). Knowledge of the location of those communities at the highest risk of smoke exposure (i.e. most vulnerable) and subsequently smoke-related health effects can inform the development of smoke adaptation plans for these communities and increase their resilience to the effects of wildfire and prescribed burn smoke.
Currently, fire emissions are estimated to contribute to nearly 12% of the world’s air pollution, which results in thousands of deaths worldwide each year (Holder et al., Citation2016). Those most at risk of disease caused by particulate matter exposure include people with underlying respiratory issues, children, the elderly, pregnant women, African Americans, and members of lower socio-economic classes (Cascio, Citation2018; Heft-Neal et al., Citation2021). According to Rutlen et al. (Citation2021), treatment of respiratory diseases in Craighead County, Arkansas, increased during the fall months of 2014, 2015, and 2016. Uncoincidentally, this is the time of year when agricultural burns are utilized the most. During the same periods in all 3 years, PM2.5 levels were also found to be higher during the fall months (Rutlen et al., Citation2021).
As a first step toward mitigating smoke in vulnerable communities, stakeholders in the rice community developed the Voluntary Smoke Management Guidelines (VSMG). In the Arkansas Delta, crop residue is burned primarily in rice production systems (AVSMG, Citation2020), though burning in Arkansas’s cropland is commonly used as part of a crop management plan in other row crops, such as wheat, corn, cotton, and soybeans. The use of prescribed fire is mainly to remove stubble post-harvest for the next crop to be planted. When burning is used as a management tool, smoke can drift through populated communities, raising potential health concerns for residents. Management guidelines for forest landowners and prescribed fire technicians, and for row crop farmers, have been developed to provide guidance for safe, efficient prescribed burning that causes as little inconvenience and exposure to the public as possible.
Recommended weather conditions to keep smoke away from populated areas and general safety tips are provided for all Arkansans who use prescribed fire as a management tool. A farmers' checklist created by the Arkansas Department of Agriculture includes specific limitations: wind speed must be less than 15 mph, humidity less than 20%, wind direction is away from roadways or communities, and crop burns must be reported to the Arkansas Agriculture Dispatch Center to be sure the airshed is not overloaded. The aim of VSMG is to help ensure that air quality and human health are not compromised by smoke from crop burns (AVSMG, Citation2020). Air quality around Arkansas is monitored to ensure that the standard for PM2.5 is met and good air quality is maintained (AVSMG, Citation2020); however, there are substantial gaps in air quality sensor deployment for monitoring throughout the state. However, there remain opportunities to validate and improve the VSMG with prescribed burning through in-field testing and monitoring and satellite-based burned area estimation. There are also opportunities to improve on-farm adoption of VSMG through web application decision tools, but more research is needed to enable such improvements. In this study, we outline an initial approach to gathering in-field data during prescribed burns and linking it to smoke transport models and satellite imagery to improve quantification of emissions from agricultural burning.
2. Methods
2.1. Research area
The area of interest for this research was Mississippi County, Arkansas, USA (Figure ). Mississippi County is located within the northeastern portion of the state along the Mississippi River, just west across the border of Tennessee and immediately south of the Missouri border. It is less than a one-hour drive east of Jonesboro, Arkansas.
Figure 1. Mississippi County, Arkansas, USA, in this study is identified by the red boundary; background imagery from google earth (https://earth.google.com) with vector data from the database of Global Administrative areas (GADM; https://gadm.org/) and QGIS version 3.16.

2.2. Deriving cropland burned area from sentinel-2
High resolution 10 m Sentinel-2 satellite imagery was used to derive burned areas within croplands only. Our study, which was funded by the U.S. Department of Agriculture’s National Institute of Food and Agriculture, was restricted to license-free open-source satellite imagery in order to be accessible to federal, state, and local environmental and agricultural agencies, including potentially the rice producers themselves. To best represent the entirety of the agricultural burn season, dates were chosen to span across the harvest season from mid-August through the end of October. Due to atmospheric conditions, such as cloud cover, smoke, and haze, and the temporal resolution (i.e. repeat time) and orbit pattern of Sentinel-2, it was not possible to find an equal interval of days between imagery dates. The images with the least cloud coverage selected for this study were as follows: 19 August 2019, 3 September 2019, 18 September 2019, 3 October 2019, and 23 October 2019.
Sentinel Hub’s EO Browser (Hub, Citation2020; https://apps.sentinel-hub.com/eo-browser/), which allows users to download satellite images from multiple satellites for no cost, was used to create true color images (10 m band combinations of B4 [red spectrum at 665 nm], B3 [green spectrum at 560 nm], and B2 [blue spectrum at 490 nm]) and customized algorithms relying on 20 m thermal, 10 m near-infrared, and 10 m visible bands to highlight burn scars. Within the EO Browser, the five dates were selected to represent the least amount of cloud cover across the harvest season. The images were downloaded as geoTIFF (32-bit) files with the highest resolution (10 m) selected. The customized burn scar images were derived using JavaScript code (Markuse, Citation2018) and hand delineated within ESRI’s ArcGIS Pro. Additional geospatial data was obtained from the Database of Global Administrative Area (GADM; https://gadm.org/), a data layer of all counties within the United States was downloaded and saved.
To find the total area of burned fields between 19 August 2019 and 23 October 2019 polygons were digitized (delineated by hand) around each individual burned field. Five separate projects were created within ArcGIS Pro for each individual image for ease of loading and editing data. Using the create tool, each individual field was outlined, with polygon area automatically calculated. From the five Sentinel-2 images representing the span of the 2019 rice harvest, a cumulative burned area of 7,173.47 acres (i.e. 29.03 km2 or 29,030,000 m2) was derived for Mississippi County, Arkansas. The areas of individual burned areas ranged from approximately 22 acres (0.09 km2) of a single small field to 398 acres (1.61 km2) of several contiguous burned fields, with an average burned area of 98 acres (or 0.4 km2). Figure shows hand delineated burns for a single image in the fall 2019 harvest season.
Figure 2. Shown here are all of the hand delineated polygons representative of burned fields in Mississippi County, Arkansas between 19 August 2019 and 23 October 2019 (https://apps.sentinel-hub.com/eo-browser/).

2.3. Comparison of sentinel-2 data with VIIRS and MODIS active fire data
Coarse resolution active fire products from the Visible Infrared Imaging Radiometer Suite (VIIRS; Schroeder, Citation2020) and Moderate Resolution Imaging Spectroradiometer (MODIS; Giglio et al., Citation2018) were provided by NASA’s Fire Information for Resource Management System (FIRMS; https://firms.modaps.eosdis.nasa.gov/) and compared with the high-resolution Sentinel-2 burned area polygons. The spatial resolution of VIIRS and MODIS is 375 m and 1000 m, respectively, and both have daily revisit times. The MODIS instruments aboard the Terra and Aqua EOS satellites acquire data continuously, providing global coverage every 1–2 days. Terra (EOS AM) passes over the equator at approximately 10:30 a.m. and 10:30 p.m., Mean Local Time (MLT), each day; Aqua (EOS PM) passes over the equator at approximately 1:30 p.m. and 1:30 a.m., MLT. There are at least four daily MODIS observations for almost every area on the equator, with the number of observations increasing (due to overlapping orbits) closer to the poles. The VIIRS instruments aboard the Suomi NPP and NOAA-20 satellites also acquire data continuously. The 3,040 km VIIRS swath enables ≈ 15% image overlap between consecutive orbits at the equator, thereby providing full global coverage every 12 hr. Suomi NPP has a nominal (equator-crossing) observation times at 1:30 p.m., MLT, and 1:30 a.m., MLT. NOAA-20 operates about 50 min ahead of Suomi NPP. Thanks to its polar orbit, the mid-latitudes will experience 3–4 looks a day (FIRMS Active Fire Data: https://firms.modaps.eosdis.nasa.gov/active_fire).
The intention was to determine the sensitivity of a variety of satellites with different spatial, temporal, and spectral resolutions for mapping and quantifying cropland burning in an area where rice is commonly managed with fire. The date chosen for comparison was 18 September 2019, as this was the date with the most fire activity documented by 10 m Sentinel-2, 1 km MODIS, and 375 m VIIRS.
2.4. Assigning crop type to burned areas
To differentiate the total cropland burning emissions by crop type, the United States Department of Agriculture (USDA) Cropland Data Layer (CDL) was used (Figure ; Johnson & Mueller, Citation2010). The Citation2019 CDL for Mississippi County was downloaded from CropScape (https://nassgeodata.gmu.edu/CropScape/) and imported into the ArcGIS Pro project. The data layer was then overlaid with the Sentinel-2 hand delineated polygon layer of burned fields to assign cropland type for emission calculations, as fuel loading, emission factors, and combustion completeness are crop type-specific. It should be noted that rice and corn are the third and fourth most common crops in Mississippi County in 2019, with soybean and cotton representing the first and second most dominant crops, respectively (Figure ).
2.5. Calculation of PM2.5 fire emissions from the sentinel-2 burned area polygons
The equation used to calculate the total emissions of corn and rice fields that burned during the Fall 2019 harvest was derived from Seiler and Crutzen (Citation1980):
where ‘Burned Area’ is equal to the total calculated area from the delineated Sentinel-2 burned area polygons from August through October 2019. We used the PM2.5 emission factor for corn of 9.94 pounds per ton and 4.72 pounds per ton for rice (Pouliot et al., Citation2016). Fuel loading is representative of the quantity of combustible biomass, here for post-harvest field clearing. The fuel loading of corn is 4.20 tons per acre and 3.00 tons per acre for rice (Pouliot et al., Citation2016). Combustion completeness represents the percentage of biomass burned, typically ranging between 0.75 and 0.95 for croplands (McCarty, Citation2011). Because combustion completeness varies across different crop types, burn conditions, and ignition types, the total emissions were calculated three times for both rice and corn fields, respectively, using a low value of 0.75 combustion completeness, a medium value of 0.85, and a high value of 0.95. We report the range and average of these emission calculations.
2.6. Field-level observation of PM2.5 using purple air sensors following the Arkansas volunteer smoke management guidelines
A planned burn of a harvested rice field was completed in Fall 2020 using the AVSMG (Citation2020) by working with a local rice producer. VSMG recommends several steps to mitigate smoke impacts on local communities while still allowing farmers opportunities to use prescribed burning as a management practice. These steps include:
1. Planning: Estimating the tons of fuel that can be burned in a given air-shed (36 square miles) depends on knowing what the weather conditions will cause the smoke to be and the number of burned acres within the air-shed.
2. Fuel loading: total amount of fuel at the prescribed burn site (Table ). The emission data needed by the Arkansas Agriculture Dispatch Center are the consumption of ‘available’ fuels for the entire burn. (Available fuel loading per acre × total acres to be burned = total available fuel loading for the burn.)
Table 1. Common fuel load ranges for major crops grown in Arkansas; bu/a indicates bushels per acre, while tons/acre of post-harvest available fuel was used in the bottom-up emissions calculations
3. Identify the nearest smoke-sensitive area: Try to keep smoke away from smoke-sensitive areas. Examples include large urban centers, airports, highways, communities, recreation areas, schools, hospitals, nursing homes, and Class I areas (areas set aside under the Clean Air Act to receive the most stringent protection from air quality degradation. Designated Class I areas in Arkansas are Caney Creek and Upper Buffalo Wilderness). The distance to smoke-sensitive areas ranges from zero miles to more than 20 miles from the prescribed fire. Follow these five steps to identify smoke-sensitive areas:
Locate on a map the prescribed fire and all potential smoke-sensitive areas, plus areas known to already have air pollution problems. The distance to smoke-sensitive areas ranges from 0 miles to less than 20 miles from the prescribed fire.
Determine the wind direction for the burn that will have the least impact on smoke-sensitive areas.
Draw a line representing the centerline of the smoke plume path using the wind direction chosen.
Determine the distance from the edge of the prescribed fire to the nearest smoke-sensitive area.
To allow for horizontal dispersion of the smoke, as well as shifts in wind direction, draw two other lines from the burn at an angle of 30 degrees from the centerline(s).
Determine Category Day: A scale from 1 to 5 based on transport wind speed and mixing height. For smoke dispersal, 1 is poor and 5 is excellent.
Determine Tons of Fuel Allocated to an Airshed.
4. Determine Category Day: Category Day—a scale from 1 to 5 based on transport wind speed and mixing height. For smoke dispersal, 1 is poor and 5 is excellent. Inversion—increase in temperature with height in the atmosphere. This condition often exists in the morning and prevents smoke from rising into the atmosphere. The category day classification can be obtained from the Arkansas Agriculture Dispatch Center or National Weather Service at http://www.weather.gov/lzk/forest2.htm. Table provides burning guidelines according to the Category Day classification.
Table 2. Category day burning guidelines
5. Determine Tons of Fuel Allocated to an Airshed: Arkansas Department of Agriculture dispatchers will notify the farmer if the tons exceed the allotment for the airshed on the day, based on conditions, and others who have reported crop burns and other prescribed fire activities that day. The fuel tonnage is figured using Table .
Table 3. Maximum tons of fuel allocated to an airshed
The maximum tons of fuel that can be allocated to an airshed based upon the downwind distance to the nearest smoke-sensitive area and the category day are shown in Table . For example, if a smoke-sensitive area is located 1/4 (0.25) mile downwind, the maximum total available fuel loading allocated to the airshed for a Category 4 day would be 720 tons.
Following the VGSM guidelines, a rice field was burned in Mississippi County, Arkansas between 1:30 and 3:00 pm Central Standard Time on 2 October 2020. The field was 79 acres (0.32 km2), with a mean rice yield of 212 bushels per acre. The field was allowed to dry for several days and prepared with a finishing mower to ensure crop residue was finely chopped near the soil, which is the recommended preparation to ensure fields burn quickly and maximally (AVSMG, Citation2020). Weather conditions were within the VSMG, sunny with wind speeds of approximately 10 mph and humidity of 25–30%.
The in-situ burn lasted approximately 45 min with additional 45 min of monitoring pre- and post-burn. A Purple Air sensor (PA-II-SD) was mounted 2 m above ground on a tripod approximately 10 m from the field at a downwind location based on wind direction at burn commencement. The sensor collected PM2.5 data with single particle count response of ≤1 s and total particle count response time of 10 s. The sensor PM2.5 measurements were recorded at one-minute intervals for this analysis. The smoke plumes were vertical with winds coming primarily from the south-southwest.
2.7. Smoke transport models for in situ crop residue burning
This study exemplifies the use of the Hybrid Single-Particle Lagrangian Integrated Trajectory (HYSPLIT) model, developed more than 30 years ago by the National Oceanic and Atmospheric Administration (NOAA) Air Resources Laboratory (ARL), to estimate smoke transport from the observed in situ burning of two rice fields in Fall 2020 as described above. The HYSPLIT model simulates the dispersion, trajectory, and deposition of pollutants using a combined Lagrangian approach to project movement and transport of air parcels and an Eulerian approach to estimate air concentrations (Stein et al., Citation2015). HYSPLIT has previously been used to accurately model smoke transport from geostationary observations of fire for the contiguous U.S. (Brey et al., Citation2018). For this analysis, we used the HYSPLIT package in R Statistical Software, or SplitR, to produce smoke trajectories and dispersion models for the planned in-field crop residue burns (Iannone, Citation2016). Using historical meteorology data from NOAA for the specific burn date, HYSPLIT was allowed to simulate smoke transport and dispersion for approximately 1 hr from the start of the burn.
3. Results and discussion
3.1. Total PM2.5 emissions from cropland burning
A total of 529 acres (2.14 km2) of corn and 6,645 acres (26.89 km2) of rice were burned in Mississippi County in Fall 2019, for a total of 7,173 burned cropland acres (or 29.03 km2). Using county-level crop area estimates from the 2019 CDL, roughly 2.6% of all corn acreage and 12.3% of all rice acreage were burned post-harvest. Burning of harvested corn fields released 8.28 tons (or 7.51 metric tonnes) of PM2.5 when 0.75 combustion completeness was assumed, and 10.49 tons (~9.51 metric tonnes) of PM2.5 when 0.95 combustion completeness was assumed. The average PM2.5 emissions for all corn fields burned within Mississippi County for Fall 2019 was 9.38 tons (or 8.51 metric tonnes). Burning harvested rice fields released 35.28 tons (or ~32.01 metric tonnes) at 0.75 combustion completeness and 44.69 tons (~40.54 metric tonnes) at 0.95 combustion completeness. On average, the 6,645 burned rice acres averaged 39.99 tons (or ~36.28 metric tonnes) of PM2.5 emissions. It is important to note that although the total burn emissions of PM2.5 were much higher in rice compared to the emissions of corn, the total area of each crop type is responsible. If a comparison was to be made between one corn field and one rice field, the total burn emissions of PM2.5 would be higher in the individual corn field as the PM2.5 emissions factor of corn is nearly double the PM2.5 emission factor of rice and the total fuel loading of corn is almost one-third higher than rice. When comparing burned field by burned field, the total PM2.5 from open burning in corn fields is much higher than that of an individual rice field. It is also important to note that we did not find evidence of a soybean or cotton field burning in the Sentinel-2, MODIS, or VIIRS fire products even though these two crops combined represent nearly 60% of the total area of Mississippi County.
Table shows the data released by the U.S. EPA in the 2017 National Emission Inventory (NEI) for agricultural burning in Mississippi County, Arkansas (US EPA, Citation2021). The prescribed burning sector was not included in this comparison because it includes fires set in forests and/or grasslands for specific resource or landscape maintenance (US EPA, Citation2021). Agricultural burning, as defined by the NEI, is burning occurring in lands used to cultivate crops. Since the bottom-up approach (Seiler & Crutzen, Citation1980) to inform inventory-based assessments was used in the analysis, it is not able to speciate particulate matter, we report only the PM2.5 Primary and not the remaining PMFINE portion of PM2.5 from the NEI. According to the 2017 NEI, Arkansas is in the top 10 states for agricultural burning, finishing at number eight behind Texas but ahead of Louisiana (US EPA, Citation2021). Our results show that the burning of harvested rice fields between August and October 2019 accounted for approximately 35% of the total annual PM2.5 emissions from all annual agricultural burnings as reported in the 2017 NEI. PM2.5 emissions from burning corn fields were only 8% of the total estimated annual PM2.5 emissions. In total, approximately 43% of annual agricultural burning PM2.5 emissions occurred between 15 August and 23 October in Mississippi County.
Table 4. Total annual PM2.5 emissions estimated from the 2017 US EPA NEI for Mississippi County, Arkansas, USA, and total fall 2019 PM2.5 emissions for Mississippi County based on Sentinel-2 burned area data; all agricultural field burning for the entire year is included in the NEI, while only the mapped corn and rice fields are included from the three-month Fall 2019 Sentinel-2 data; units are in imperial tons and not metric tonnes
3.2. Sentinel-2 burned area captures different fires than VIIRS and MODIS active fire products
For the most part, the data collected between the three satellite images appear different, however a few similarities can be recognized. Figure shows Sentinel-2 captured burns in blue polygons, and VIIRS and MODIS captured burns are represented by red points. While there is some spatial matching between the two, it is weak. One explanation for the differences in detection is the spatial resolution of each satellite product, as well as limiting our data retrievals to cloud-free imagery only. VIIRS active fire data has a resolution of 375 m, while MODIS active fires have a resolution of 1 km, and the Sentinel-2 imagery has 10–20 m pixels depending on the bands used. Furthermore, the spatial mismatch is likely attributed to the timing of image acquisition. For example, using the Committee on Earth Observation Satellite (CEOS) Visualization Environment (COVE; Kessler et al., Citation2013; available at https://ceos-cove.org/) to determine acquisition times of Sentinel-2A and VIIRS on the NPP and NOAA-20 satellites for 19 August 2019, we find that Sentinel-2 imagery was collected at 16:54:30 UTC (10:54:30 CST), while NOAA-20 VIIRS was collected 90 min later at 18:31:30 UTC (12:31:30 CST) and NPP VIIRS was collected approximately 150 min later at 19:22:30 UTC (13:22:30 CST). In total, the fires in Figure represent the final overlap of the three unique satellite collections of fire activity which highlights the absence of MODIS detections based on its temporal and spatial resolution. These findings for a rice growing county in Arkansas are similar to previous results in rice growing areas of Punjab, India, which found that even ∼15-min differences between VIIRS and MODIS/Aqua afternoon overpasses caused large discrepancies in active fire detections (Li et al., Citation2018). For the air quality community, this is important to consider when calculating total PM2.5 emissions or modeling smoke transport. These results suggest that using all available satellite products, including commercial very high-resolution data like Planet or MAXAR’s WorldView, for burned area and active fire identification is important for improving emissions cropland and open burning emission estimates. Future studies should also compare Sentinel-2 with Planet and WorldView to capture high spatial and temporal resolution of rice residue burning in the Mississippi Delta.
Figure 4. For the Fall 2019 harvest period, the blue polygons illustrate burned fields delineated from 10 m Sentinel-2 data and red points illustrate active fires detected by VIIRS active fire product for both NOAA-20 and NPP satellites provided by the NASA fire information for Resource management System in Mississippi County, AR (Schroeder, Citation2020; NASA FIRMS; https://earthdata.nasa.gov/firms).

3.3. PM2.5 emissions from a planned rice field burn
Purple Air sensors were used to record air quality information before and during a planned rice field burn in Mississippi County, Arkansas. The sensor was placed 10 m outside of the field in the downwind direction from the planned burn. Figure illustrates the PM2.5 measurements taken each minute before and during the burn. Note the initial spike in PM2.5 at minute 37 and wavering but high PM2.5 for the approximate 30 min of the main burn event. The dip in the middle was primarily due to a brief shift in wind direction a few minutes into the burn. As the fire burned out and smoldered the final 15 min, the PM2.5 measurements decreased to pre-burn levels. Figure show field images pre- and peri-burn, respectively.
Figure 5. PM2.5 measurements from field-based Purple air sensors in Mississippi County, AR. [A]: the x-axis shows the minutes recorded by the sensor from 0 (start of data collection) through 70 (end of data collection) at 1-minute intervals and the y-axis shows the PM2.5 detected. The vertical red line illustrates the approximate start of the burn. [B] image of the pre-burn field. [C] image of the peri-burn field.
![Figure 5. PM2.5 measurements from field-based Purple air sensors in Mississippi County, AR. [A]: the x-axis shows the minutes recorded by the sensor from 0 (start of data collection) through 70 (end of data collection) at 1-minute intervals and the y-axis shows the PM2.5 detected. The vertical red line illustrates the approximate start of the burn. [B] image of the pre-burn field. [C] image of the peri-burn field.](/cms/asset/02784f2a-5ff1-4f2d-b5cc-bb79f37e566c/oaes_a_2274646_f0005_oc.jpg)
3.4. Emissions dispersion from crop residue burning in Mississippi, county Arkansas, USA
The results of the HYSPLIT model show that within 1 hour, smoke was able to transport approximately 4.6 miles (or 7.5 km) in a southeast direction (Figure ), ranging from ground-level to as high as nearly 400 m (or over 1,300 feet). In Figure , the HYSPLIT simulated smoke particle density is estimated for the horizontal and vertical distances (in kilometers) from the source location using a kernel density estimate to visualize the horizontal and vertical components of dispersion. Much of the smoke lofted at heights of 50 m (~164 feet) at approximately 0.6 miles (or 1 km) away from the planned burn. Within 3.1 miles/5 km, most of the smoke was lofted at or above 200 m or 656 feet.
4. Conclusion
To result in a definite causation and not an estimated correlation, additional information and research must be considered to provide a definitive answer to the overall effects of PM2.5 emissions on air quality and public health (Cascio, Citation2018). Although fires can occur in one centralized area, the effects do not always remain local nor does the smoke stay at ground level given ideal burning conditions like those documented in our planned burn in Fall 2020. Wind patterns can carry emissions a great distance affecting populations outside of the immediate area, while some of the smoke stays at ground-level. This means that although fires take place in Mississippi County, air pollutants can transport into other communities in surrounding counties. It is also important to note that emissions from agricultural burning from other counties can transport into Mississippi County, creating additional emissions that may not be accounted for.
Until additional research is conducted, it will be difficult to fully understand the harmful effects of cropland burn emissions on air quality and public health. Until further research is conducted it will be difficult to show the repercussions of the intensity of PM2.5 emissions, as it has been noted that various satellites provide varying data and results. Until then, correlations between increased burn emissions, decreased air quality, and increased reports of health implications will remain the explanation.
Current monitor placement by the U.S. EPA is not sufficient in East Arkansas to capture air quality information relevant for understanding the potential impacts of cropland burning (Figure ). Our analysis supports the need for strategic placement of new monitoring sites, especially in areas with higher population density such as Jonesboro and Osceola. Without better monitoring, it will not be possible to understand how smoke emissions from cropland burning affect local communities. Additional research is needed to fully understand the harmful effects of toxic burn emissions on air quality and public health as well as proving the intensity and dispersion of PM2.5 from cropland. Our study shows that various satellites provide varying data and results, so better in situ monitoring is of utmost importance for evaluating the linkages between cropland burning, PM2.5, and public health. Until such research is available, correlations between increased burn emissions, decreased air quality, and increased reports of health implications will remain the explanation.
Figure 7. U.S. EPA interactive map of air quality with green dots representing current PM2.5 monitoring sites. Source: U.S. EPA AirNow.

The results from this study can support the COVID-delayed 2020 and future NEI processes conducted by the U.S. EPA. This analysis shows that high resolution burned area data from Sentinel-2 imagery, here hand-delineated for one fall harvest period, add new and unique information on total acreage burned and an additional crop type of corn. These PM2.5 emission estimates based on high resolution burned area data are not currently included in the NEI process, which is dependent on MODIS and VIIR active fire data as well as state-level reports (Pouliot et al., Citation2017). Something as small as satellite overpass timing means that each new sensor flying overhead is collecting new fire activity data for the sector of agricultural burning, because the fires themselves burn so very quickly—which we also documented in our Fall 2020 planned burn. Active fire-based emission inventories are missing a lot of short-lived human-caused burning in agricultural landscapes (Ramo et al., Citation2021). Moving forward, fire emission inventories informed by Earth observations and remote sensing methodologies need to include high resolution burned area estimates.
In an attempt to reduce emissions released from human-caused fires, action should be taken by the government at the local, state, and federal levels (Cascio, Citation2018). Regulations should be instilled to determine what types of crop residues can or cannot be burned and under what conditions burns can be conducted. Rules should regulate when farmers can burn to ensure burns occur only on days that are favorable for fire and smoke conditions, when wind patterns will carry emissions away from densely populated areas. Smoke management guidelines, like Arkansas’ VSMG (AVSMG, Citation2020) can work if followed, which requires outreach, extension, implementation, and cooperation from agricultural producers. Continued refinement of transport models like HYSPLIT will aid in burn and smoke management for prescribed agricultural burning. Better management of emissions is an important issue which must be addressed in order to maintain the best interest of public health. If the issues related to agricultural burn emissions are not addressed, it is likely that health-care providers and health insurers will need to make adjustments to better meet the demands of patients and customers accordingly (Cascio, Citation2018).
Acknowledgements
The authors thank the students and research assistants who helped with field data collection. This work is supported by Capacity-Building Grants for Non-Land-Grant Colleges of Agriculture Program (NLGCA) grant no. 2020-70001-31289 from the USDA National Institute of Food and Agriculture. Any opinions, findings, conclusions, or recommendations expressed in this publication are those of the author(s) and do not necessarily reflect the view of the U.S. Department of Agriculture.
Disclosure statement
No potential conflict of interest was reported by the author(s).
Data availability statement
Data used in this study will be made upon reasonable request.
Additional information
Funding
References
- AVSMG. (2020). Arkansas voluntary smoke management guidelines. Arkansas Department of Agriculture, Little Rock.
- Brey, S. J., Ruminski, M., Atwood, S. A., & Fischer, E. V. (2018). Connecting smoke plumes to sources using hazard mapping system (HMS) smoke and fire location data over North America. Atmospheric Chemistry & Physics, 18(3), 1745–13. https://doi.org/10.5194/acp-18-1745-2018
- Brye, K. R., Longer, D. E., & Gbur, E. E. (2006). Impact of tillage and residue burning on carbon Dioxide Flux in a wheat–soybean production System. Soil Science Society of America Journal, 70(4), 1145–1154. https://doi.org/10.2136/sssaj2005.0151
- Cascio, W. E. (2018). Wildland fire smoke and human health. Science of the Total Environment, 624, 586–595. https://doi.org/10.1016/j.scitotenv.2017.12.086
- Chakrabarti, S., Khan, M. T., Kishore, A., Roy, D., & Scott, S. P. (2020). Corrigendum to: Risk of acute respiratory infection from crop burning in India: Estimating disease burden and economic welfare from satellite and national health survey data for 250 000 persons. International Journal of Epidemiology, 49(2), 710–711. https://doi.org/10.1093/ije/dyz279
- Desrochers, J., Brye, K. R., Gbur, E., Pollock, E. D., & Savin, M. C. (2020). Carbon and nitrogen properties of particulate organic matter fractions in an alfisol in the mid-Southern, USA. Geoderma Regional, 20, e00248. https://doi.org/10.1016/j.geodrs.2019.e00248
- Giglio, L., Boschetti, L., Roy, D. P., Humber, M. L., & Justice, C. O. (2018). The collection 6 MODIS burned area mapping algorithm and product. Remote Sensing of Environment, 217, 72–85. https://doi.org/10.1016/j.rse.2018.08.005
- Haiyan, N., Jie, T., Xiaoliang, W., Qiyuan, W., Yongming, H., Junji, C., Xin, L., Antony, L.-W., Judith, C. H., John, C., Ru-Jin, G. W., & Ulrike, H. (2017). PM2.5 emissions and source profiles from open burning of crop residues. Atmospheric Environment, 169, 229–23. https://doi.org/10.1016/j.atmosenv.2017.08.063
- Heft-Neal, S., Driscoll, A., Yang, W., Shaw, G., & Burke, M. (2021). Associations between wildfire smoke exposure during pregnancy and risk of preterm birth in California. Environmental Research, 203, 111872. https://doi.org/10.1016/j.envres.2021.111872
- Holder, A. L., Hagler, G. S. W., Aurell, J., Hays, M. D., & Gullett, B. K. (2016). Particulate matter and black carbon optical properties and emission factors from prescribed fires in the southeastern United States: Aerosol optical properties from fires. Journal of Geophysical Research: Atmospheres, 121(7), 3465–3483. https://doi.org/10.1002/2015JD024321
- Huang, X., Li, M., Li, J., & Song, Y. (2012). A high-resolution emission inventory of crop burning in fields in China based on MODIS thermal Anomalies/fire products. Atmospheric Environment, 50, 9–15. https://doi.org/10.1016/j.atmosenv.2012.01.017
- Hub, S. (2020). Modified Copernicus Sentinel data 2020/Sentinel hub (EO browser). Sinergise laboratory for geographical information systems. Ltd.
- Iannone, R., 2016. Splitr: V0.4. Zenodo. https://doi.org/10.5281/ZENODO.49106
- Johnson, D. M., & Mueller, R. (2010). The 2009 cropland data layer. Photogrammetric Engineering & Remote Sensing, 76, 1201–1205. https://www.nass.usda.gov/Research_and_Science/Cropland/docs/JohnsonPE&RS_Nov2010.pdf
- Kessler, P. D., Killough, B. D., Gowda, S., Williams, B. R., Chander, G., & Min, Q. (2013). CEOS visualization Environment (COVE) tool for intercalibration of satellite instruments. IEEE Transactions on Geoscience & Remote Sensing, 51(3), 1081–1087. https://doi.org/10.1109/TGRS.2012.2235841
- Korontzi, S., McCarty, J., Loboda, T., Kumar, S., & Justice, C. (2006). Global distribution of agricultural fires in croplands from 3 years of moderate resolution imaging spectroradiometer (MODIS) data: GLOBAL DISTRIBUTION of AGRICULTURAL FIRE. Global Biogeochemical Cycles, 20(2), n/a–n/a. https://doi.org/10.1029/2005GB002529
- Lasko, K., Vadrevu, K. P., Tran, V. T., Ellicott, E., Nguyen, T. T. N., Bui, H. Q., & Justice, C. (2017). Satellites may underestimate rice residue and associated burning emissions in Vietnam. Environmental Research Letters, 12(8), 085006. https://doi.org/10.1088/1748-9326/aa751d
- Li, J., Bo, Y., & Xie, S. (2016). Estimating emissions from crop residue open burning in China based on statistics and MODIS fire products. Journal of Environmental Sciences, 44, 158–170. https://doi.org/10.1016/j.jes.2015.08.024
- Li, F., Zhang, X., Kondragunta, S., & Csiszar, I. (2018). Comparison of fire radiative power estimates from VIIRS and MODIS observations. Journal of Geophysical Research: Atmospheres, 123(9), 4545–4563. https://doi.org/10.1029/2017JD027823
- Markuse, P. (2018). Visualizing wildfires and burn scars with the Sentinel hub EO browser V2. https://custom-scripts.sentinel-hub.com/custom-scripts/sentinel-2/markuse_fire/
- McCarty, J. L. (2011). Remote Sensing-based estimates of annual and seasonal emissions from crop residue burning in the contiguous United States. Journal of the Air & Waste Management Association, 61(1), 22–34. https://doi.org/10.3155/1047-3289.61.1.22
- McCarty, J., Justice, C., & Korontzi, S. (2007). Agricultural burning in the southeastern United States detected by MODIS. Remote Sensing of Environment, 108(2), 151–162. https://doi.org/10.1016/j.rse.2006.03.020
- McCarty, J. L., Korontzi, S., Justice, C. O., & Loboda, T. (2009). The spatial and temporal distribution of crop residue burning in the contiguous United States. Science of the Total Environment, 407(21), 5701–5712. https://doi.org/10.1016/j.scitotenv.2009.07.009
- Pouliot, G., Rao, V., McCarty, J. L., & Soja, A. (2016). Development of the crop residue and rangeland burning in the 2014 National Emissions Inventory using information from multiple sources. Journal of the Air & Waste Management Association, 67, 613–622. https://doi.org/10.1080/10962247.2016.1268982
- Pouliot, G., Rao, V., McCarty, J. L., & Soja, A. (2017). Development of the crop residue and rangeland burning in the 2014 National emissions inventory using information from multiple sources. Journal of the Air & Waste Management Association, 67(5), 613–622. https://doi.org/10.1080/10962247.2016.1268982
- Ramo, R., Roteta, E., Bistinas, I., van Wees, D., Bastarrika, A., Chuvieco, E., & van der Werf, G. R. (2021). African burned area and fire carbon emissions are strongly impacted by small fires undetected by coarse resolution satellite data. Proceedings of the National Academy of Sciences of the United States of America, 118(9), e2011160118. https://doi.org/10.1073/pnas.2011160118
- Rappold, A. G., Reyes, J., Pouliot, G., Cascio, W. E., & Diaz-Sanchez, D. (2017). Community vulnerability to health impacts of wildland fire smoke exposure. Environmental Science and Technology, 51(12), 6674–6682. https://doi.org/10.1021/acs.est.6b06200
- Reid, C. E., Brauer, M., Johnston, F. H., Jerrett, M., Balmes, J. R., & Elliott, C. T. (2016). Critical review of health impacts of wildfire smoke exposure. Environmental Health Perspectives, 124(9), 1334–1343. https://doi.org/10.1289/ehp.1409277
- Roy, D. P., Boschetti, L., Justice, C. O., & Ju, J. (2008). The collection 5 MODIS burned area product — Global evaluation by comparison with the MODIS active fire product. Remote Sensing of Environment, 112(9), 3690–3707. https://doi.org/10.1016/j.rse.2008.05.013
- Rutlen, C., Orloff, M., Bates, J., & Porter, A. (2021). Crop burning and the prevalence of asthma and COPD emergency department treatments in a rural Arkansas county. Journal of Asthma, 58(3), 293–298. https://doi.org/10.1080/02770903.2019.1708096
- Schroeder, W. (2020). VIIRS (NOAA-20/JPSS-1) I band 375 m active fire locations NRT (vector data). EarthData. https://doi.org/10.5067/FIRMS/VIIRS/VJ114IMGT_NRT.002
- Seiler, W., & Crutzen, P. J. (1980). Estimates of gross and net fluxes of carbon between the biosphere and the atmosphere from biomass burning. Climatic Change, 2(3), 207–247. https://doi.org/10.1007/BF00137988
- Shyamsundar, P., Springer, N. P., Tallis, H., Polasky, S., Jat, M. L., Sidhu, H. S., Krishnapriya, P. P., Skiba, N., Ginn, W., Ahuja, V., Cummins, J., Datta, I., Dholakia, H. H., Dixon, J., Gerard, B., Gupta, R., Hellmann, J., Jadhav, A. … Somanathan, R., 2019. Fields on fire: Alternatives to crop residue burning in India. Science.
- Starns, H. D., Tolleson, D. R., Agnew, R. J., Schnitzler, E. G., & Weir, J. R. (2020). Smoke in the Great Plains, USA: An increasing phenomenon with potential policy and health implications. Fire Ecology, 16(1), s42408-020-00073–1. https://doi.org/10.1186/s42408-020-00073-1
- Stein, A. F., Draxler, R. R., Rolph, G. D., Stunder, B. J. B., Cohen, M. D., & Ngan, F. (2015). NOAA’s HYSPLIT Atmospheric transport and dispersion modeling system. Bulletin of the American Meteorological Society, 96(12), 2059–2077. https://doi.org/10.1175/BAMS-D-14-00110.1
- USDA, N. A. S. S. (2019). USDA National agricultural Statistics Service cropland data layer. USDA National Agricultural Statistics Service.
- US EPA, 1997. Exposure factors handbook (1997, final report) (No. EPA/600/P-95/002F a-c). U.S. Environmental Protection Agency,
- US EPA. (2021). 2017 National emissions Inventory: January 2021 updated release, technical support document (No. EPA-454/R-21-001). U.S. Environmental Protection Agency.
- US EPA, 2022. Summary nonattainment area population exposure report. U.S. U.S. Environmental Protection Agency, https://www3.epa.gov/airquality/greenbook/popexp.html. Last updated: December 31, 2021. Last visited: January 26, 2022.
- Vadrevu, K. P., Ellicott, E., Badarinath, K. V. S., & Vermote, E. (2011). MODIS derived fire characteristics and aerosol optical depth variations during the agricultural residue burning season, north India. Environmental Pollution, 159(6), 1560–1569. https://doi.org/10.1016/j.envpol.2011.03.001