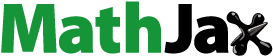
ABSTRACT
Land degradation because of the overutilization of natural resources is a suitable strategy for many countries of the world. This crucial strategy hasn’t been introduced or implemented widely enough. The present study intended to examine the status of Land Degradation Neutrality (LDN) for the study Rib Watershed, which covers an area of 1585 km2 and is situated in the Upper Blue Nile Basin, Ethiopia. For the past two periods between 2000 and 2020, three indicators, such as Land Cover/Use Changes (LUC), Soil Organic Carbon (SOC), and Net Primary Productivity (NPP) metrics were primarily used to assess the LDN status of the study site. A total of 80 soil samples were then collected from the top 15 cm for six different types of LULC, including cultivated land, forest land, shrub lands, grassland, settlement, and water body. Consequently, land uses land cover matrices, NPP metric, and SOC metrics were also obtained using Land sat images, soil samples, and MODIS satellite images, respectively, for three periods of 2000, 2010, and 2020. Lastly, by integrating the three indicators and using one out all out framework, the status of LDN in the study area was evaluated. The combined findings of the study measurements showed that there was a net loss in cultivated land (123,977 ha), forest land (5623 ha), shrub lands (13984 ha), grassland (11,999 ha), water body (1056 ha) and settlement (1993 ha) for the past two decades (2000 to 2020). For the past two decades, LDN status of the Rib Watershed was generally in a net loss condition. The information delivered by the three sub-indicators is important for experts for the good recognition of their spatial distribution and types of land degradation to attain the LDN targets.
1. Introduction
Land degradation (LD) is a serious environmental problematic issue that harmfully affects ecosystem services, biodiversity, and the lives of millions of people in the world by promoting poverty and migration (Gashu & Muchie, Citation2018; Meseret, Citation2016; Susilowati & Syekhfani, Citation2015). LD can indicate the decline of biological or economic productivity in rain-fed farmland (Reith et al., Citation2021; Susilowati & Syekhfani, Citation2015). However, due to the complexity and variety of the biophysical and socioeconomic factors that affect land resources, it is challenging to identify this phenomenon (Lu et al., Citation2022; Meseret, Citation2016). By integrating information about ecosystems and the mechanisms that produce degradation, numerous research has evaluated the phenomenon of LD at the international as well as national levels. Due to the complexity, variety, and quantity of indicators employed in the research carried out during the past 30 years, the evidence is not similar throughout regions (Gashu & Muchie, Citation2018; Lu et al., Citation2022). However, data was scarce, and the cost of repeatability and implementation of techniques makes them rarely practical at more local and regional scales (Adnan, Citation2020; Del Barrio et al., Citation2021; Malav et al., Citation2022).
The notion of ‘Land Degradation Neutrality’ (LDN), which is a component of one of the Sustainable Development Goals (SDG), target 15 articles 3 (15.3), was recently introduced by the United Nations General Assembly as a novel approach to tackling land degradation (LD). A state in which the quantity and quality of land resources required to maintain ecosystem functions and services and enhance food security within particular temporal and spatial scales and ecosystems is referred to as LDN”. and is served as a measurement of SDG 15.3 (Cowie et al., Citation2018). To reach a healthy, productive state for land resources with no net loss, land degradation must therefore be avoided, reduced, and reversed.
The LDN can be assessed by using a methodological and conceptual scientific framework accompanied by the Convention to Combat Desertification (UNCCD) (Gonzalez-Roglich et al., Citation2019; Liniger et al., Citation2019). In this framework, using the ‘one out of all out (1OAO)’ approach, the three indicators, such as Land Cover (LC), Land Productivity Dynamics (LPD), and Soil Organic Carbon (SOC) are combined in the assessment of LD. However, the decline of any indicator corresponds to the degradation of LDN. The choice of indicators provides a foundation for assessing the current state of the land, understanding changes and trends through time, monitoring the rate of degradation, and calculating the proportion of degraded land (Cowie et al., Citation2018; Erşahin, Citation2020; Quatrini & Crossman, Citation2018). In addition, UNCCD offers a global dataset to identify patterns of land degradation neutrality in a particular geographic area (Gonzalez-Roglich et al., Citation2019).
Some studies on LD and inducing factors have been performed in various areas (Del Barrio et al., Citation2021; Gashu & Muchie, Citation2018; Malav et al., Citation2022; Susilowati & Syekhfani, Citation2015). Yet, past research suggested that the LDN concept had only lately been introduced and taken into account. LDN was initially studied in dry areas by (Grainger, Citation2015). They emphasized the significance of researching LD in dry regions and achieving the objectives for sustainable development goals. (Minelli et al., Citation2018) offered a basic outline of important worldwide decisions that contributed to the formation of the LDN conception in addition to explaining how to measure, monitor, and assess LDN. (Safriel, Citation2017) studied on LDN has been designed with a focus on semi-arid areas, to neutralize LD and restore degraded lands following the UNCCD to accomplish the SDGs. (Dengiz, Citation2018) explored possible impacts of land use changes on the dynamics of land productivity in semi-humid parts of the Gediz Watershed over 14 years (2001–2014) with a focus on the LDN concept. He used data from Landsat satellite images to detect changes in land use and cover. He collected 319 soil samples from all around the study catchment to evaluate SOC. The results showed that the watershed’s total land productivity has dropped by 23%. (Kiani-Harchegani & Hamidreza Sadeghi, Citation2020) looked into the LDN status of the Shazand watershed, in Iran. They uses satellite images, MODIS NPP, and Soil samples for the assessment of the three sub-indicators of LDN from 2000 to 2016. The result of the combination of the study indicators showed that the status of LDN in the watershed was in a net loss situation.
In Ethiopia’s context, the idea of LDN is not researched by scholars. For instance, in the study Rib watershed, no one is doing research on the assessment of LDN. Therefore, this research intends to fill the knowledge gap by taking the Rib watershed of the Upper Blue Nile basin as a case site for the past two decades. Hence, this study was targeted at examining the LDN status in the study watershed using three determinant variables (land use/land cover change, net primary productivity, and soil carbon content) from 2000 up to 2020. The objective of the present study was to (1) Examine the land use land cover change for the past two decades (2000 up to 2020), (2) Detect the trend of net primary productivity in the study area from the time series of the (MODIS) NPP data (2000–2020). (3) Assess soil organic carbon change in the study Rib watershed, (4) determine the status of LDN in the Rib watershed of Upper Blue Nile Basin, Ethiopia from the period 2000 to 2020.
2. Material and method
2.1 Description of the study area
The research was done in the Rib watershed of Upper Blue Nile Basin, Ethiopia, which extends from Mountain Guna to Lake Tana in the eastern direction and is considered the principal source of water for the Blue Nile. It is situated under the Lake Tana basin of northwestern Ethiopia and has an absolute location between 10°43’ − 11° 53’ N and 35° − 37° 47’ E. The elevation of the study watershed ranges from 1785 – 4049 above sea level and has a catchment area of 1583 km2 (Figure ). The watershed’s terrain is extremely hilly, with a steep mountain range on its southern border and sparsely spaced hills and their escarpments on its center and northern sides (Sinshaw et al., Citation2021). The watershed is the main source of water for Lake Tana. The major river in the watershed is the Rib River. Woredas, such as Ebnat, Farta, Libo Kemkem, and Fogera are all intersected by the river. Rib Dam is under construction since 2008 and cost $ 40 million. It has a reservoir storage capacity of 234 million m3. The dam is targeted to use for irrigation purposes at Fogera plain of Amhara regional state and is proposed to supply 14,000 ha of land to serve more than 28,000 households (Annys et al., Citation2020). The rice granary and demonstration location for national rice research are both located in Fogera Plain, which is part of the Fogera woreda.
According to the projected Central Statistical Authority (Central Statistical Agency, Citation2014), 878,261 people are living within the watershed, with 89.7% of them living in rural regions and relying primarily on agriculture. According to (Anley et al., Citation2022), the land use land cover of the watershed consists of Agriculture (123,977 ha), Forest land (5623 ha), Shrub land (13984), Grassland (11,999 ha), and water body (1056 ha). Among those land use, agriculture is the primary economic activity in the study area and is used as the source of livelihood for the rural community. On one side, a variety of agricultural products are grown, and on the other, animals are reared for both market and domestic consumption in the study watershed. Rainfall is a major factor in crop productivity; it mostly falls between June and September. As a result, farming can only be done during the remaining months of dry weather, which is uncommon in the watershed. However, only a small portion of the farming community employs irrigation to harvest maize and onions. The community in the study watershed depends largely on the rearing of cattle for their survival. Four types of soils derived from weathering of basaltic rocks were known in the study area, including Luvisols (34%), Leptosols (28%), Vertisols (23%), and Regosols (15%) (Ayalew & Bharti, Citation2022).
The climatic zone of the Rib watershed is highly dominated by subtropical (traditionally, woina dega), statistically 2% (alpine), 64.4% (sub-tropical), and 33.6% (temperate). Weather record data for the past two decades indicated an annual average rainfall (1550.5 mm) and temperature (15.4 °C) of the study watershed. Over 70% of the rainfall occurs during the summer season. Little or no rainfall data was recorded in the remaining months.
2.2 Measurement of LDN metrics
The LDN status in the study Rib Watershed was examined using the Scientific Conceptual Framework (SCF) developed by (Cowie et al., Citation2018). Accordingly, the LUC, NPP, and SOC indicators for the study area throughout the periods of 2000–2010, 2010–2020, and 2000–2020 were used to examine the LDN status (Figure ). The existing approach for evaluating and assessing LDN consists of the use of site‐based data to measure the quantitative value of the sub-indicators resulting from Earth observation and geospatial information. For those indicators, the baseline data was determined. This would involve the preparation of baseline data on the land cover that builds on existing land cover ontologies, as well as establishing baselines for soil organic carbon and land productivity in the research area.
After the baseline data was prepared, change detection for each sub-indicator was done to identify the area subject to change and mostly where the change in two or three indicators overlap spatially. Lastly, the methodological and conceptual scientific framework conducted by (Cowie et al., Citation2018) was applied to evaluate the status of LDN in the study watershed. In this framework, the evaluation of LD uses the ‘one out all out (1OAO)’ approach to integrate the three indicators, where the degradation of any indication results in the degradation of LDN (Figure ). According to this framework, if any of the three indicators displays considerable negative change, it is considered as degradation. Conversely, it is deemed an improvement of the land condition if at least one indicator shows a significant positive change and none shows a significant negative change. The change of each sub-indicator of LDN between years was calculated using analysis of variance in SPSS software version 23. The preparation and measurement of the indicators are as follows:
2.1.1 Land use/land cover
The term ‘land cover’ describes the (bio) physical cover that can be seen on the earth’s surface (Karki et al., Citation2018; Tolessa et al., Citation2017; Vigl et al., Citation2017; Willemen et al., Citation2018). One of the most frequently used markers for influences on ecosystems caused by humans or by the environment is land cover (Arunyawat & Shrestha, Citation2016; Meshesha et al., Citation2014). This indicator of LDN can detect the change in time series and may show degradation or the improvement (Recovery) of the land condition in a certain geographical territory.
Time-series multispectral Landsat imagery taken in three different years (2000, 2010, and 2020) were utilized in the study area to set the baseline land cover and identify land cover changes (Table ). The images were kindly downloaded from the United States Geological Survey (USGS) (https://www.usgs.gov). The raw images were free from clouds because they were taken during the drier season. Therefore, all satellite images were taken in February with less cloud cover.
Table 1. The accuracy assessment report (in percent) of the classified LULC maps
By utilizing the fundamental image preparation techniques, satellite image data were prepared and processed using the ERDAS IMAGINE 14 software. Pre-processing methods for the satellite images included color composite, layer stacking, and sub-setting were done. To increase image quality, Landsat images were then rectified geometrically and radiometrically. Finally, a projection to the Universal Transverse Mercator (UTM) was applied. The satellite image from the years 2000, 2010, and 2020 were classified using the supervised image classification method with a maximum likelihood classification algorithm. Finally, Six LULC classes such as cultivated land, grazing land, forest, shrub land, waterbody, and settlement were identified in the research area.
Google Earth, interviews with elder people, and expert knowledge were utilized to classify images for 2000 and 2010. Field observation was carried out, and ground control points (GCPs) were acquired using a Garmin GPS device to perform accuracy assessments for each land-use class to gather the training sample for 2020. According to (ERDAS Inc., Citation2005), it is recommended that at least 50 GPS points for each land-use type must be taken for image classification. To improve accuracy, 100 GPS points were typically taken at each land use in this study watershed. As a result, the entire sets of LULC classes were used to collect 600 GCP training samples for supervised image classification.
The number of GCPs at each class was determined using the areal extent of each land use. By contrasting the sample LULC class of the classified layer to the reference layer, the classification accuracy assessment of the LULC layers of satellite images was explored. To determine the degree of accuracy error, the overall accuracy and Kappa statistics were analyzed. Lastly, the area in hectares was calculated. LULC is one of the indicators of LDN. The change of each LULC has its contribution to determining the status of LDN in the study Rib watershed. According to (Cowie, Citation2018), the expansion of agricultural land and settlement, along with the loss of natural and semi-natural land cover types (forest, shrubs, grasslands, and waterbodies), indicated that the degradation (loss) of the land condition, and the inverse is true for the improvement (gain) of the land. Hence, this standard was applied to examine the impact of LULC on the status of LDN in the study area.
2.2.2. Net Primary Productivity (NPP)
The difference between total photosynthesis and total plant respiration in an ecosystem is also known as land productivity. It is a measure of above-ground net primary productivity (NPP) and is considered as the total amount of new organic matter produced over a certain period (de la Fuente et al., Citation2020). Tones of dry matter per hectare per year are used to measure NPP (t DM/ha/year). Primary productivity of plants indicates the distinct change over varied temporal scales; daily variability because of the position of the sun, intra-annual variability because of seasonal effects, and inter-annual variability because of dynamics in land use (Liu et al., Citation2022; Xuan & Rao, Citation2023). Therefore, the land productivity of the study watershed was disaggregated by type of land cover. The spatial and temporal resolution of remote sensing data may give ongoing and synoptic information to determine the influence of natural and human stresses on the status and trends of the land’s productive potential (Cui & Li, Citation2022; de la Fuente et al., Citation2020).
In the Rib Watershed, Upper Blue Nile Basin, the NPP distribution was examined in six LULC classes, including cultivated land, grazing land, forest, shrub land, water bodies, and settlement, within the node years of 2000, 2010, and 2020. The NPP estimation image at the watershed level was downloaded from the NASA Moderate Resolution Imaging Spectroradiometer (MODIS) MOD17A3 NPP product (http://lpdaac.usgs.gov) with 500 m spatial resolution. Finally, Arc GIS 10.3 software was used to extract the NPP of each land cover type, and SPSS 23 software was used to check whether there is a significant difference or not between the years of each LULC class.
2.2.3. Soil organic carbon
The residual plant and animal matter that bacteria produce and decomposes in response to environmental factors including temperature, moisture, and soil conditions is what makes up soil organic carbon (Amanuel et al., Citation2018a). Depending on natural factors (temperature, soil parent material, land cover and/or vegetation, and topography), as well as human-induced factors (land use, management, and degradation), the annual rate of loss of organic matter can vary significantly. It is one of the sub-indicator of LDN. The study area was divided into Land Unit Tracts (land use classes) to measure the soil’s organic carbon content. The soil sampling was collected in late January and February 2020. Accordingly, eighty soil samples were ultimately taken from the entire watershed while considering the proportion of six different land cover types. (i.e. grazing land, cultivated land, shrub land, forest land, water body, and settlement). The sampling sites were properly located using a global positioning system (GPS). Each quadrate’s center was sampled for soil by inserting a core sampler up to a depth of 15 cm.
Two types of soil samples were collected in the field. From the first groups, twenty soil samples for bulk density and another twenty soil samples for organic carbon were collected from the types of land cover, which was unchanged for the past two decades. These types of sample soil represent the baseline carbon stock data for the year 2000. From the second group, twenty soil samples for bulk density and twenty soil samples for organic carbon were collected from the types of land cover which were changed to other land use for the last two decades. This soil sample represents the soil carbon stock of the target year (2020). The soils were collected using an auger, immediately placed in polythene bags to maintain their field moisture, and then taken to the Amhara agriculture center soil laboratory. The samples were dry in an oven to calculate the bulk density (BD) using the cold method. The Walkley and Black method was finally used to compute SOC.
Towards this, SOC maps for the years 2000 and 2020 were developed for the Rib watershed using the Inverse distance weight method of interpolation in ArcGIS 10.3 software. Information on the grids was individually extracted to form a SOC map in the years 2000 and 2020 for each land cover type in the study site. In the next step, SOC amounts for the years 2000 and 2020 were computed using EquationEquation (1)(1)
(1) and (Equation2
(2)
(2) ). Lastly, a proper database was ready for SOC in Excel, and the crucial statistical analyses were made in SPSS software packages. Using the following equation, the SOC density for each sampling site (SOCD in t C ha1) was calculated (Husein et al., Citation2019).
Where SOC refers to the SOC content in %, BD refers to the bulk density in g cm−3, and D indicates the depth of the soil surface layer at 15 cm. The total SOC storage in the study region, TSOC (t C), was expressed in this regard as follows.
Where ASOCD refers to the average SOC density (t C ha−1), S indicates the area of different land covers (ha).
2.3 Statistical analysis
SPSS 23.0 statistical software was used to conduct all statistical analyses. To compare the means of measurements for LUC, NPP, and SOC, a one-way analysis of variance (one-way ANOVA) was employed during the periods of 2000–2010, 2010–2020, and 2000–2020. Finally, using the three metric values, LDN statuses were investigated throughout periods at a statistically significant level of P < 0.05. One-way analysis of variance was important to check whether there is a significant difference between the baseline years (2000) and targeted years (2020). According to (Cowie et al., Citation2018), a significant increment and stability of the indicator shows the improvement of the land condition (positive impact for LDN) and the significant reduction of those indicators shows the reduction of the land condition (negative LDN).
3. Results
3.1. Analysis of indicators of land degradation neutrality
3.1.1 Land use land cover change
One of the most crucial indicators to evaluate the status of LDN in the study watershed was land use and land cover. Six LULC classes, including cultivated land, forestland, shrub land, grassland, water body, and settlement, were found in the study area. According to our earlier analysis (Anley et al., Citation2022), the overall accuracy assessment for the years 2000, 2010, and 2020 was 80.11%, 82.7%, and 84.8%, respectively (Table ). This confirms the suggested and recommended value by (Olofsson et al., Citation2014). Thus, the data was used for the assessment of LDN in the study Rib watershed.
Statistically, cultivated land has increased by 23% in the last two decades. Whereas forest decreased by 8.9% from (2000 to 2010), 41% (2010 up to 2020), and 46.5% (2000 to 2020). Shrub land decreased by 27.1% from 2000 to 2010 and 24% from 2010 to 2020. The total reduction of shrubs was 44.5% from 2000 to 2020. Grassland decreased by 20%, 27%, and 41.5% from 2000 to 2010, 2010 to 2020, and 2000 to 2020, respectively. Water body decreases considerably between 2000 to 2010 but showed an incredible expansion between 2010 and 2020 (Table , Figure ). The expansion in the water body is probably caused by the building of the Rib irrigation dam. According to (Cowie et al., Citation2018), natural and semi-natural land cover types (forest, shrubs, grasslands, water body) change to agricultural land and settlement indicate the degradation of the land condition, and the inverse is true for the improvement (Recovery) of the land. Hence, this standard was applied to this study.
Table 2. The LULC map of Rib watershed for the year 2000, 2010 and 2020 periods
As depicted above in Table , there was a significant change in cultivated and settlement areas between 2000 and 2020. Therefore, the expansion of cultivated land and settlement has a negative effect on the status of LDN (Cowie et al., Citation2018). Additionally, there was a significant reduction in forest, shrubs, and grassland. This significant reduction has also a negative impact on the status of LDN. Whereas water body showed a significant increment over the past two decades. This shows that the increment of the water body has a positive impact on the status of LDN.
3.1.2 Land productivity change
The intricate processing of space-based data provides an evaluation of the dynamics of land production, which is a proxy indicator of the status of sustained land quality. An excellent indicator of Earth’s potential to continue providing ecosystem services is the dynamics of its biomass. An approximation of a metric for the general productivity levels of the land environment system is to evaluate variations in NPP dynamics. Land users take advantage of this land productivity to harvest biological goods with a profit (Cui & Li, Citation2022; Sciortino et al., Citation2020). A decline in total land productivity might be expected to show degradation (loss) of the land quality, whereas an increment of NPP shows improvement (gain) of the land condition in a certain geographical territory. According to (Cowie et al., Citation2018), a significant negative trend of NPP over the analysis period shows productivity decline (loss), whereas a significant positive trend leads to an increase in NPP, hence improvement (gain) of the land condition.
The land productivity change for each land use cover was extracted using Arc Map 10.3 software. The gain or loss of biomass, which reflects the LPD, is significantly influenced by the land use/land cover and average NPP. Table indicated that there was a significant positive change in NPP between 2000 and 2020 for forest land, shrub land, and grassland. These increments have a positive impact on the status of LDN in the study watershed. Whereas the Average NPP of water body indicated significant negative change, this situation has a negative impact on the status of LDN. Furthermore, the NPP value of cultivated land and settlement was stable for the past two decades in the study watershed.
Table 3. NPP of the year 2000, 2020 and implication for LDN
The Terra Moderate Resolution Imaging Spectroradiometer (MODIS) NPP time series was used to calculate the trend of net primary productivity for the years 2000 to 2020. These data were produced at a spatial resolution of 500 m by the United States National Aeronautics and Space Administration’s Land Processes Distributed Active Archive Center (NASA LP DAAC). The MODIS NPP product is estimated from atmospherically corrected bi-directional surface reflectance that has been masked for water, clouds, heavy aerosols, and cloud shadows (Liu et al., Citation2022; Quatrini & Crossman, Citation2018; Sciortino et al., Citation2020; Yu & Song, Citation2023). The average annual NPP was calculated for every pixel using MODIS NPP data from 2000 to 2020. The average annual was calculated as the arithmetic mean of the NPP values within each year.
The change in NPP value of cultivated land, forest land, shrub land, settlement, and Grassland indicated a positive change for the year 2000 to 2020. But the NPP value of water body indicated a negative change. Statistically, Table, 3 indicated that cultivated land and settlement increased by 259.61 t/DM/ha and 343.52 t/DM/ha, respectively, for the past two decades. These changes are stable with no significant change at p < 0.05. Whereas forest land increased by 914.47 t/DM/ha, Shrub land by 487.10 t/DM/ha, Grassland by 555.65 t/DM/ha and their change was a significant positive change from 2000 to 2020.
3.1.3 Soil organic carbon
The largest concentration of carbon is found in soils, which are also used as a proxy indicator for LD assessments in particular geographic regions (Amanuel et al., Citation2018b; Husein et al., Citation2019), but there is a shortage of quality data about carbon stocks in different land use land cover types (Sims et al., Citation2019). The SOC concentration indicated the major difference with land cover types (p < 0.05; Table , Figure ). The overall average SOC concentration was higher under forest land and lower under cultivated land compared with other land uses in 2000 as well as 2020. In contrast, for the year 2020, the lowest average SOC stock of cultivated land was 76.98 t/c/ha, and the highest was for forest land at 125.27 t/c/ha.
Table 4. Mean soil organic carbon data of each land use and the status of metrics
In this study site, average SOC stock of the agricultural field was reduced by − 2.58 t/c/ha from 2000 to 2020. This showed that there is no significant difference between the changes in carbon stock for the past two decades in the study area in the surface layer (0–15 cm). The same is true for other land cover types, such as forest land reduced by (−3.33), Shrub land by (− 2.27), and grassland by (− 4.15) from 2000 to 2020. The change of SOC for the above-mentioned land use was non-significant at p < 0.05. In the study, Rib watershed compared different land use showed that SOC declined by 3.32% in cultivated land, 2.58% in forest land, 0.02% in shrub land, and 3.69% in grassland land compared between 2000 and 2020. Whereas the average SOC of settlement and water body increased by 6.08t/ha (5.64%) and 8.31(7.79%) t/ha, respectively, for the past two decades. Those land covers showed a significant difference in the change of SOC between 2000 and 2020 (Table , Figure ). This positive change of SOC in settlement and waters body has a positive impact on the status of LDN in the study watershed.
3.2. Analysis of the LDN status
The years between 2000 and 2020 were then examined for the three primary LDN metrics of LC, NPP, and SOC. For this aim, the maps in the changes of metrics were prepared in the pixel size as shown in (Figures ) and précised (Table ). Then, one-way analysis of variance (ANOVA) was used to compare measures’ mean values for the period 2000–2020. The metrics of each data of different years of 2000, 2010, and 2020 were obtained from Land sat image, MODIS NPP, and soil samples. Lastly, the value of each metrics data of the watershed as well as each land cover type was then extracted from Arc GIS 10.3 using a spatial analysis tool and depicted in (supplementary material). Finally, databases of each metric (LC, NPP, and SOC) were prepared in Excel 2016 for SPSS software to check whether there is a significant difference between metrics or not.
Table 5. LDN status of the Rib watershed
The indicator was quantified by estimating changes in the sub-indicators over time, to determine the spatial extent of land that is degraded over total land area. To determine whether this area was improving or degraded over time, the extent of degradation assessed in each sub-indicator for the targeted periods (2020) was compared to the extent of degradation measured in the baseline period (2000). The baseline determines the benchmark extent of degradation against which changes towards achieving SDG Target 15.3 and LDN status was evaluated in the study watershed. The 1OAO concept was therefore used in the calculating procedure, and changes in the sub-indicators are shown as Positive (improving), Negative (declining), and Stable. (Unchanging). If one of the sub-indicators is significantly declining then LDN status degradation will occur for a particular land unit.
According to Table , there was a positive significant change in cultivated land between 2000 and 2020 at P < 0.05. The expansion of cultivated land is considered the cause of degradation (Cowie et al., Citation2018). The remaining metrics, NPP and SOC observed that there was no significant change (Stable) for the past two decades. As can be seen, using the 1OAO rule, the calculated LDN metrics show a degradation trend of cultivated land in the study Rib watershed.
As can be seen from Table , the three metrics of forest land and shrub land showed the Sig—ve change, Sig + ve change, and Stable (no sign change), respectively, for the past two decades. This indicates that the LDN status of both forest land and shrub land was under degradation (loss) in the study watershed. The status of metrics for settlement was Sig—ve change for LUC, Stable, no significant change for NPP and Sign + ve change for SOC from the year 2000 to 2020. The change of metrics for settlement for the past two decades showed a degradation in the status of LDN. In the same vein, grassland showed Sig—ve change, Sig + ve change, and Stable, with no significant change for LUC, NPP, and SOC, respectively. This situation implies the degradation (loss) of grassland. The last type of land cover was water body. The change of tree metrics for water body was Sig +ve change for LUC and again Sig +ve change for SOC at P < 0.05. However, NPP showed the Sig—ve change for the past two decades. In general, the output of the above-mentioned land cover type indicated that a net loss (degradation) occurred from 2000 to 2020 at the Rib watershed of the Upper Blue Nile Basin, Ethiopia.
4. Discussion
4.1 Trends of LULC, NPP, and soil organic carbon changes
The decline of the forest, shrub land, grassland, and the increase of cultivated and settlement in the Rib watershed during the study period (2000–2020) is consistence with the findings of several studies in Ethiopia (Meshesha et al., Citation2014; Minta et al., Citation2018; Tolessa et al., Citation2017). The expansion of cultivated land in Ethiopia is mostly credited to the country’s policy. For instance, in the existing Ethiopian prosperity government, the land ownership policy is specified in the constitution, with land as the belongings of the state (Mengesha et al., Citation2022). This allowable them to use the land but not to sell it. Due to the state’s ownership of resources related to land, rural residents were easily able to transform grazing, shrub, and forest land into cultivated land. In the 1990s, the government also implemented the Agricultural Development Led Industrialization (ADLI) policy, to ensure that agriculture is a key driver of economic growth in Ethiopia (Welteji, Citation2018). From this perspective, a rise in the amount of cultivated land is anticipated in the country.
An evaluation of the dynamics of land productivity, which is a proxy statement of the status of sustained land quality, is produced through the complex processing of routine space-based observation. The change in the Earth’s biomass cover is an important expression of its capacity to supply ecosystem services. Assessing the dynamics in vegetative cover change is an approximation of a measure for general productivity levels of the land. Land users take advantage of this land productivity to produce biological products of economic value. The change in NPP value of cultivated land, forest land, shrub land, settlement, and Grassland indicated a significant positive change in land productivity for the year 2000 to 2020. The finding of our result was which is in line with the conclusion of other papers (Lu et al., Citation2022; Wang et al., Citation2015). Whereas the change in settlement and waterbody showed stable and negative changes in land productivity in the study watershed, respectively.
The SOC concentration indicated that there is a significant difference within land use types (p < 0.05; Table ). The total mean SOC concentration was higher under forest and lower under cultivated land compared with other land uses which is similar with other studies such as (Amanuel et al., Citation2018b; Soleimani et al., Citation2019). The cause for more soil carbon stock in the soil of forest is due to the minimum disturbance in soil and a slow amount of SOC decomposition which result in higher carbon accumulation, whereas low SOC stock in agricultural land is due to intensive cultivation practices which results in larger soil disturbance, and increase rate of SOC decomposition.
This study showed that the change in land cover could have an effect on how much carbon is stored in the soil. To increase the SOC content, efforts should be made to use appropriate land use management practices, such as applying organic manure. In addition to helping to increase soil carbon, land use management is a crucial step in protecting the soils organic carbon. Based on the type of land use or cover, the study found considerable variations in the amount of organic carbon in the top 15 cm of soil. The results showed the average reduction of organic carbon from cultivated land (2.58t/c/ha), forest land (3.33), shrub land (2.27), and grassland (4.15) for the past two decades at Rib watershed. These results highlight the important role that land use plays in influencing soil carbon levels and the need for prudent land-use procedures to maintain soil health and maintain soil carbon levels. This decline is a result of the removal of above-ground biomass from cultivated and grassland areas for livestock feed. In addition, tillage and site preparation activities disrupt the soil, which exposes organic matter to breakdown and causes quick losses of soil organic carbon. The decomposition, leaching, and soil erosion can contribute to the decreased soil organic carbon storage. Poor farm management and post-harvest grazing may also be to impact soil organic carbon storage (Yu & Song, Citation2023). After harvest, there may be a large loss of topsoil and organic matter, especially in regions with high levels of precipitation and rapid rates of erosion, like the Rib watershed.
4.2 The status of land degradation neutrality
The result of the tree indicator indicated that a net loss (degradation) occurred from 2000 to 2020 at the Rib watershed of Upper Blue Nile Basin, Ethiopia. In this regard, the results are consistence with (Akhtar-Schuster et al., Citation2017), who highlighted the change in land use change, continuous expansion of degraded land, and avoiding the prospect of ever achieving a stable state of LDN. To combat LD and ecologically degraded lands in the research area, sound planning and management are then necessary for any land use. The LDN status was under degradation state in the Rib Watershed of Upper Blue Nile Basin for the year 2020. This study provides decision-makers with key information on the LDN status in different LULCs of the Rib Watershed. The output of the study is vital to apprise policy development on land management mostly, specifically on how to design for the implementation and monitoring of LDN by 2030. In this concern (Akhtar-Schuster et al., Citation2017; Grainger, Citation2015), and (Gichenje et al., Citation2019) stressed the evaluation of LDN and restoring degraded lands, improving national land use planning systems, and establishing national monitoring capacities. The outcomes for the LDN were also compared with those for the LDN approach, as published by (Bobushev & Sultanaliev, Citation2020; Gichenje & Godinho, Citation2019; Gichenje et al., Citation2019; Kust et al., Citation2020; Sims et al., Citation2019; Teich et al., Citation2019; Von Maltitz et al., Citation2019; Zolotov et al., Citation2020) dealing with LDN approach.
5. Conclusions
The goal of the current study was to evaluate the current LDN status by using the LDN approach in the Rib Watershed of the Upper Blue Nile Basin. To plan and manage the many compartments in a watershed ecosystem adequately, managers and planners require information on the LDN status for at least 10 years. To assess the LDN status in the Rib Watershed, the metrics provided by UNCCD, including changes in land cover, NPP, and SOC, were employed. Different land uses were classified as being in a state of degradation (sign—ve change), improvement (sign +ve change), or stability (no change) based on the analysis of the trend in each metric. In order to prevent, decrease, and stop degradation in the settlement, water body, and cultivated land in the study watershed, appropriate managerial actions might be planned using the research’s findings.
As one of the determinant factors LDN of in the research area, various conservation agricultural land management strategies can also be recommended to increase the organic matter contents of the soil. Therefore, managers and planners must take into account the capability and potential of the lands in diverse ecosystems given the LDN status in various types of land use in the Rib Watershed. Meanwhile, it is essential to put suitable managerial and developmental plans into place to prevent interventions or changes in land use that would worsen land degradation in the research area. In conclusion, experts are suggested to take managerial actions to avoid the degradation of different types of land use.
Authorship contribution
MA carried out, data collection in the field, data analysis, and writing of the manuscript. AS contributed to the overall supervision of the work and commented and edited the manuscript. All writers read and approved the final manuscript.
Availability of data and materials
The datasets used during the current study are available from the corresponding author on reasonable request.
Acknowledgements
The authors thank the development of agricultural experts of Yefag, Guna begemeder, Ebnat, and Addis zemen woreda for their genuine contribution during ground control point data collection.
Disclosure statement
The writers attest that they have no known financial conflicts of interest that could have seemed to influence the work reported in this paper.
Additional information
Funding
Notes on contributors

Melkamu Alebachew Anley
Melkamu Alebachew Anley is a lecturer at Debre Tabor University in the Faculty of Social Science and the Department of Geography and Environment. He have published an article entiled on ”Assessing the impacts of land use/cover changes on ecosystem service values in Rib watershed, Upper Blue Nile Basin, Ethiopia” at journal of Trees, Forests and People, Elsevier. His research area focuses on Ecosystem service value, soil loss, sediment export and Land degradation related issues. This manuscript is the result of one of the objectives of his dissertation.
Amare Sewnet Minale
Amare Sewnet (Proffessor) is my principal advisor; he is the first professor in the history of geography department at Bahir Dar University. He is a senior lecturer, researcher, and advisor at Bahir Dar University, Department of Geography and Environmental Studies. He published many articles related with soil loss, Ecosystem service,urban and climate related issues.
References
- Adnan, M. (2020). Land degradation and its management: A review. International Journal of Environmental Sciences & Natural Resources, 25(1), 63–14. https://doi.org/10.19080/ijesnr.2020.25.556157
- Akhtar-Schuster, M., Stringer, L. C., Erlewein, A., Metternicht, G., Minelli, S., Safriel, U., & Sommer, S. (2017). Unpacking the concept of land degradation neutrality and addressing its operation through the rio conventions. Journal of Environmental Management, 195, 4–15. https://doi.org/10.1016/j.jenvman.2016.09.044
- Amanuel, W., Yimer, F., & Karltun, E. (2018a). Soil organic carbon variation in relation to land use changes: The case of birr watershed, Upper Blue Nile river Basin, Ethiopia. Journal of Ecology and Environment, 42(1), 1–11. https://doi.org/10.1186/s41610-018-0076-1
- Amanuel, W., Yimer, F., & Karltun, E. (2018b). Soil organic carbon variation in relation to land use changes: The case of birr watershed, Upper Blue Nile river Basin, Ethiopia. Journal of Ecology and Environment, 42(1), 1–11. https://doi.org/10.1186/s41610-018-0076-1
- Anley, M. A., Minale, A. S., Ayehu, N. H., & Gashaw, T. (2022). Assessing the impacts of land use/cover changes on ecosystem service values in Rib watershed, Upper Blue Nile Basin, Ethiopia. Trees, Forests and People, 7(3), 100212. https://doi.org/10.1016/j.tfp.2022.100212
- Annys, S., Van Passel, S., Dessein, J., Adgo, E., & Nyssen, J. (2020). From fast-track implementation to livelihood deterioration: The dam-based Ribb irrigation and drainage project in Northwest Ethiopia. Agricultural Systems, 184(July), 102909. https://doi.org/10.1016/j.agsy.2020.102909
- Arunyawat, S., & Shrestha, R. P. (2016). Assessing land use change and its impact on ecosystem services in Northern Thailand. Sustainability (Switzerland), 8(8), 768. https://doi.org/10.3390/su8080768
- Ayalew, L. T., & Bharti, R. (2022). Modeling sediment yield of Rib watershed, Northwest Ethiopia. ISH Journal of Hydraulic Engineering, 28(S1), 491–502. https://doi.org/10.1080/09715010.2020.1797544
- Bobushev, T. S., & Sultanaliev, K. E. (2020). Evaluation and adaptation of the land degradtion neutrality approach to land classification Resources in the Kyrgyz Republic. Arid Ecosystems, 10(2), 123–126. https://doi.org/10.1134/S2079096120020031
- Central statistical Agency. (2014). Federal democratic republic of Ethiopia Central statistical Agency population projection of Ethiopia for all regions at Wereda level from 2014 – 2017. The Central Statistics Agency.
- Cowie, A. L., Orr, B. J., Castillo Sanchez, V. M., Chasek, P., Crossman, N. D., Erlewein, A., Louwagie, G., Maron, M., Metternicht, G. I., Minelli, S., Tengberg, A. E., Walter, S., & Welton, S. (2018). Land in balance: The scientific conceptual framework for land degradation neutrality. Environmental Science and Policy, 79(October), 25–35. https://doi.org/10.1016/j.envsci.2017.10.011
- Cui, Y., & Li, X. (2022). A New global land productivity dynamic product based on the consistency of various vegetation biophysical indicators. Big Earth Data, 6(1), 36–53. https://doi.org/10.1080/20964471.2021.2018789
- de la Fuente, B., Weynants, M., Bertzky, B., Delli, G., Mandrici, A., Garcia Bendito, E., Dubois, G., & Martínez-Paz, J. M. (2020, August). Land productivity dynamics in and around protected areas globally from 1999 to 2013. PLoS ONE, 15(8), 1–13. https://doi.org/10.1371/journal.pone.0224958
- Del Barrio, G., Sanjuán, M. E., Martínez-Valderrama, J., Ruiz, A., & Puigdefábregas, J. (2021). Land degradation means a loss of management options. Journal of Arid Environments, 189(2021), 104502. https://doi.org/10.1016/j.jaridenv.2021.104502
- Dengiz, O. (2018). Potential impact of land use change on land productivity dynamics with focus on land degradation in a sub-humid terrestrial ecosystem. Theoretical and Applied Climatology, 133(1–2), 73–88. https://doi.org/10.1007/s00704-017-2162-1
- ERDAS Inc. (2005). ERDAS field guide (5th ed.). Leica Geosystems Geospatial Imaging, LLC.
- Erşahin, S. (2020). A method for calculating land degradation neutrality. MethodsX, 7(April), 100969. https://doi.org/10.1016/j.mex.2020.100969
- Gashu, K., & Muchie, Y. (2018). Rethink the interlink between land degradation and livelihood of rural communities in Chilga District, Northwest Ethiopia. Journal of Ecology and Environment, 42(1), 1–11. https://doi.org/10.1186/s41610-018-0077-0
- Gichenje, H., & Godinho, S. (2019). A climate-smart approach to the implementation of land degradation neutrality within awater catchment area in Kenya. Climate, 7(12), 136. https://doi.org/10.3390/cli7120136
- Gichenje, H., Muñoz-Rojas, J., & Pinto-Correia, T. (2019). Opportunities and limitations for achieving land degradation-neutrality through the Current land-use Policy framework in Kenya. Land, 8(8), 115. https://doi.org/10.3390/land8080115
- Gonzalez-Roglich, M., Zvoleff, A., Noon, M., Liniger, H., Fleiner, R., Harari, N., & Garcia, C. (2019). Synergizing global tools to monitor progress towards land degradation neutrality: Trends. Earth and the world overview of conservation approaches and technologies sustainable land management database. Environmental Science and Policy, 93(December), 34–42. https://doi.org/10.1016/j.envsci.2018.12.019
- Grainger, A. (2015). Is land degradation neutrality feasible in dry areas? Journal of Arid Environments, 112(PA), 14–24. https://doi.org/10.1016/j.jaridenv.2014.05.014
- Husein, H. H., Mousa, M., Sahwan, W., Bäumler, R., & Lucke, B. (2019). Spatial distribution of soil organic matter and soil organic carbon stocks in semi-Arid area of Northeastern Syria. Natural Resources, 10(12), 415–432. https://doi.org/10.4236/nr.2019.1012028
- Karki, S., Thandar, A. M., Uddin, K., Tun, S., Aye, W. M., Aryal, K., Kandel, P., & Chettri, N. (2018). Impact of land use land cover change on ecosystem services: A comparative analysis on observed data and people’s Perception in Inle Lake, Myanmar. Environmental Systems Research, 7(1). https://doi.org/10.1186/s40068-018-0128-7
- Kiani-Harchegani, M., & Hamidreza Sadeghi, S. (2020). Practicing land degradation neutrality (LDN) approach in the shazand watershed, Iran. Science of the Total Environment, 698, 134319. https://doi.org/10.1016/j.scitotenv.2019.134319
- Kust, G. S., Andreeva, O. V., & Lobkovskiy, V. A. (2020). Land degradation neutrality: The Modern approach to research on Arid regions at the national level. Arid Ecosystems, 10(2), 87–92. https://doi.org/10.1134/S2079096120020092
- Liniger, H., Harari, N., van Lynden, G., Fleiner, R., de Leeuw, J., Bai, Z., & Critchley, W. (2019). Achieving land degradation neutrality: The role of SLM knowledge in evidence-based decision-making. Environmental Science and Policy, 94(June), 123–134. https://doi.org/10.1016/j.envsci.2019.01.001
- Liu, Y., Wang, X., Xin, L., & Lu, Y. (2022). Impact of farmland change on vegetation NPP in the one river and two streams region of Tibet. Land, 11(12), 2223. https://doi.org/10.3390/land11122223
- Lu, A., Tian, P., Mu, X., Zhao, G., Feng, Q., Guo, J., & Xu, W. (2022). Fuzzy logic modeling of land degradation in a Loess Plateau watershed, China. Remote Sensing, 14(19), 4779. https://doi.org/10.3390/rs14194779
- Malav, L. C., Yadav, B., Tailor, B. L., Pattanayak, S., Singh, S. V., Kumar, N., Reddy, G. P. O., Mina, B. L., Dwivedi, B. S., & Kumar Jha, P. (2022). Mapping of land degradation vulnerability in the semi-Arid watershed of Rajasthan, India. Sustainability (Switzerland), 14(16), 1–16. https://doi.org/10.3390/su141610198
- Mengesha, A. K., Mansberger, R., Damyanovic, D., Agegnehu, S. K., & Stoeglehner, G. (2022). The contribution of land registration and certification program to implement SDGs: The case of the Amhara region, Ethiopia. Land, 12(1), 93. https://doi.org/10.3390/land12010093
- Meseret, D. (2016). Land degradation in Amhara region of Ethiopia: Review on extent, impacts and rehabilitation practices. Journal of Environment and Earth Science, 6(1), 120–130.
- Meshesha, D. T., Tsunekawa, A., Tsubo, M., Ali, S. A., & Haregeweyn, N. (2014). Land-use change and its socio-Environmental impact in Eastern Ethiopia’s Highland. Regional Environmental Change, 14(2), 757–768. https://doi.org/10.1007/s10113-013-0535-2
- Minelli, C., van der Plaat, D. A., Leynaert, B., Granell, R., Amaral, A. F. S., Pereira, M., Mahmoud, O., Potts, J., Sheehan, N. A., Bowden, J., Thompson, J., Jarvis, D., Davey Smith, G., Henderson, J., & Lewis, C. (2018). Age at puberty and risk of asthma: A mendelian randomisation study. PLoS Medicine, 15(8), 1–14. https://doi.org/10.1371/journal.pmed.1002634
- Minta, M., Kibret, K., Thorne, P., Nigussie, T., & Nigatu, L. (2018). Land use and land cover dynamics in Dendi-Jeldu Hilly-Mountainous areas in the Central Ethiopian Highlands. Geoderma, 314(May), 27–36. https://doi.org/10.1016/j.geoderma.2017.10.035
- Olofsson, P., Foody, G. M., Herold, M., Stehman, S. V., Woodcock, C. E., & Wulder, M. A. (2014). Good practices for estimating area and assessing accuracy of land change. Remote Sensing of Environment, 148, 42–57. https://doi.org/10.1016/j.rse.2014.02.015
- Quatrini, S., & Crossman, N. D. (2018). Most finance to halt desertification also benefits multiple ecosystem services: A key to unlock investments in land degradation neutrality? Ecosystem Services, 31, 265–277. https://doi.org/10.1016/j.ecoser.2018.04.003
- Reith, J., Ghazaryan, G., Muthoni, F., & Dubovyk, O. (2021). Assessment of land degradation in semiarid tanzania-using multiscale Remote Sensing datasets to support sustainable development goal 15.3. Remote Sensing, 13(9), 1–21. https://doi.org/10.3390/rs13091754
- Safriel, U. (2017). Land degradation neutrality (LDN) in Drylands and beyond – where has it come from and where does it go. Silva Fennica, 51(1), 1–19. https://doi.org/10.14214/sf.1650
- Sciortino, M., De Felice, M., De Cecco, L., & Borfecchia, F. (2020). Remote Sensing for monitoring and mapping land productivity in Italy: A rapid assessment methodology. Catena, 188(May), 104375. https://doi.org/10.1016/j.catena.2019.104375
- Sims, N. C., England, J. R., Newnham, G. J., Alexander, S., Green, C., Minelli, S., & Held, A. (2019). Developing good practice guidance for estimating land degradation in the context of the United Nations sustainable development goals. Environmental Science and Policy, 92(October), 349–355. https://doi.org/10.1016/j.envsci.2018.10.014
- Sinshaw, B. G., Belete, A. M., Mekonen, B. M., Wubetu, T. G., Anley, T. L., Alamneh, W. D., Atinkut, H. B., Gelaye, A. A., Bilkew, T., Tefera, A. K., Birara Dessie, A., Fenta, H. M., Beyene, A. M., Bizuneh, B. B., Alem, H. T., Eshete, D. G., Atanaw, S. B., Tebkew, M. A., & Mossie Birhanu, M. (2021). Watershed-based soil erosion and sediment yield modeling in the Rib watershed of the Upper Blue Nile Basin, Ethiopia. Energy Nexus, 3(September), 100023. https://doi.org/10.1016/j.nexus.2021.100023
- Soleimani, A., Hosseini, S. M., Bavani, A. R. M., Jafari, M., & Francaviglia, R. (2019). Influence of land use and land cover change on soil organic carbon and microbial activity in the Forests of Northern Iran. Catena, 177(July), 227–237. https://doi.org/10.1016/j.catena.2019.02.018
- Susilowati, L. E., & Syekhfani. (2015). Journal of degraded and mining lands management. Degraded and Mining Lands Management, 2(3), 361–367. https://doi.org/10.15243/jdmlm.2014.021.235
- Teich, I., Roglich, M. G., Corso, M. L., & García, C. L. (2019). Combining Earth observations, cloud computing, and expert knowledge to inform national level degradation assessments in support of the 2030 development agenda. Remote Sensing, 11(24), 2918. https://doi.org/10.3390/rs11242918
- Tolessa, T., Senbeta, F., & Abebe, T. (2017). Land use/land cover analysis and ecosystem services valuation in the central highlands of Ethiopia. Forests, Trees and Livelihoods, 26(2), 111–123. https://doi.org/10.1080/14728028.2016.1221780
- Vigl, E., Lukas, E. T., Schirpke, U., & Tappeiner, U. (2017). Using land use/land cover trajectories to uncover ecosystem service patterns across the Alps. Regional Environmental Change, 17(8), 2237–2250. https://doi.org/10.1007/s10113-017-1132-6
- Von Maltitz, G. P., Gambiza, J., Kellner, K., Rambau, T., Lindeque, L., & Kgope, B. (2019). Experiences from the South African land degradation neutrality target setting process. Environmental Science & Policy, 101(May), 54–62. https://doi.org/10.1016/j.envsci.2019.07.003
- Wang, Z., Wang, Z., Zhang, B., Lu, C. & Ren, C. (2015). Impact of land use/land cover changes on ecosystem services in the Nenjiang River Basin, Northeast China. Ecological Processes, 4(1). https://doi.org/10.1186/s13717-015-0036-y
- Welteji, D. (2018). A critical review of rural development Policy of Ethiopia: Access, utilization and coverage. Agriculture & Food Security, 7(1), 1–6. https://doi.org/10.1186/s40066-018-0208-y
- Willemen, L., Crossman, N. D., Quatrini, S., Egoh, B., Kalaba, F. K., Mbilinyi, B., & de Groot, R. (2018). Identifying ecosystem service hotspots for targeting land degradation neutrality investments in South-Eastern Africa. Journal of Arid Environments, 159, 75–86. https://doi.org/10.1016/j.jaridenv.2017.05.009
- Xuan, W., & Rao, L. (2023). Spatiotemporal dynamics of net primary productivity and its influencing factors in the middle reaches of the Yellow river from 2000 to 2020. Frontiers in Plant Science, 14(January), 1–16. https://doi.org/10.3389/fpls.2023.1043807
- Yu, H., & Song, W. (2023). Research progress on the impact of land use change on soil carbon Sequestration. Land, 12(1), 213. https://doi.org/10.3390/land12010213
- Zolotov, D. V., Chernykh, D. V., Yu Biryukov, R., Pershin, D. K., Malygina, N. S., & Gribkov, A. V. (2020). Change of land use in Altai Krai: Problems and prospects for the achievement of land degradation neutrality. Arid Ecosystems, 10(2), 106–113. https://doi.org/10.1134/S2079096120020134