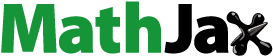
ABSTRACT
In this study, geostatistical techniques were used to investigate spatial variability of physico-chemical properties of soil samples from farming- and artisanal gold mining-affected areas. Based on coefficients of variation, chemical properties, except pH, had high variability compared to physical properties. This could be attributed to the local lithology, vegetation cover and land-use. Spatial distribution patterns of silt, clay, total nitrogen, pH and C/N could be controlled by extrinsic factors such as biological activity and land-use patterns, whereas those of electrical conductivity, organic matter (SOM) and sand were governed by both intrinsic and extrinsic factors. Because of the dominance of clay type, the distribution of cation exchange capacity (CEC) was spatially correlated with clay. About 80% of the area had acidic to circumneutral pH, which may increase mobility and toxicity of most cationic heavy metals. Distribution maps showed that microbial activities, expressed as C/N ratios, tend to be weak around farmlands, reflecting the impacts of soil management practices. In contrast, abundance of clay around the river bank is an indication of soil erosion and its redistribution from upland to depositional areas. Mean error and root mean square error of kriging were higher than those of cokriging for SOM, suggesting that cokriging was more reliable for spatial interpolation of this variable. The work reported in this study indicated that that 29%, 77% and 75% of the area had high probabilities to have pH, CEC and SOM contents below which most cationic heavy metals would be mobile and bioavailable in the soils.
1. Introduction
In recent years, misuse of fertilizers and pesticides in farming, high population growth and artisanal mining have been releasing huge quantities of heavy metals into soils worldwide (Bamba et al., Citation2013; Marrugo-Negrete et al., Citation2017; Sako et al., Citation2018). Once in the soil environment, these metals can be immobilized or be available to plants and animals and end up in the food web (Ashraf et al., Citation2017; Kumar et al., Citation2019). The bioavailability and geochemical behavior of heavy metals in soils are directly and indirectly influenced by soil physico-chemical properties such as soil organic matter (SOM) content, particle size distribution, pH, electrical conductivity (EC) and cation exchange capacity (CEC). Contrary to temperate soils, semi-arid tropical soils are byproducts of intensive chemical weathering over a pedological time scale through which primary minerals (e.g. felsic and ferromagnesian minerals) are converted into clay and residual minerals with near complete removal of base cations (da Silva et al., Citation2022; Hampl et al., Citation2023). Not only these cations are essential macronutrients for plant growth and development, their presence also increases soil CEC, which determines its buffering capacity to maintain metallic pollutants against leaching and subsequent migration to the groundwater systems (Aharonov-Nadborny et al., Citation2017; B. Huang et al., Citation2015; Puga et al., Citation2016; Suzuki et al., Citation2020). Accordingly, several studies have indicated that CEC is one of the key soil quality indicators that are sensitive to anthropogenic activities (e.g. Graber et al., Citation2017; Khaledian et al., Citation2017).
Given their large specific surface area, negative charges, ability to hold and exchange positively charged ions, clay minerals are major carriers of heavy metals in soils (Uddin, Citation2017). Unlike the soils with dominant coarse particles, clayey soils tend to retain heavy metals, and thus reduce their mobility and bioavailability (Zhang et al., Citation2020). Similarly, soils with high clay and SOM contents tend to have high CEC (Moral & Rebollo, Citation2017; Rahal & Alhumairi, Citation2019). Under high SOM contents, dissolved organic matter can be complexed with heavy metals, which, in turn, will improve soil structure and its adsorptive capacity (Jiang et al., Citation2021). Loss of SOM, due to farming, may destroy soil structure and imped water flow and atmospheric O2 and soil CO2 exchanges (Helliwell et al., Citation2014; Siedt et al., Citation2020). The subsequent buildup of CO2 decreases soil pH, increases heavy metal concentrations and exacerbates their migration and toxicity (Al-Wabel et al., Citation2019; Ouyang et al., Citation2020). Moreover, the low pH and O2 contents are likely to inhibit the growth and activity of soil microbial community, leading to a low humus production and formation of unstable soil aggregates (Obalum et al., Citation2017; Oldfield et al., Citation2018). Ultimately, this will affect soil structure and its ability to immobilize anthropogenically derived heavy metals. Another factor that may cause soil acidity and heavy metal content is the application of acidifying fertilizers with trace concentrations of heavy metals (Wei et al., Citation2020). Such agricultural practices are likely to increase heavy metal background levels and reduce soil pH (Ning et al., Citation2017). In the resulting acidified soils, nutrient loss and heavy metal concentrations will be increased (Anza et al., Citation2021; Kicińska et al., Citation2021). Therefore, among soil physico-chemical properties, pH is considered as one of the most important environmental indicators that can be used to estimate heavy metal mobility and toxicity in the soil environment (Maurya et al., Citation2020; Sungur et al., Citation2016).
Artisanal gold mining and its associated activities also have profound effects on the environmental quality of the surrounding soils (Compaore et al., Citation2019; Eludoyin et al., Citation2017; Tankari Dan-Badjo et al., Citation2019). That is, the compounded impacts of climate change on agricultural yields and high gold price, have led to an upsurge of artisanal gold mining sites across Burkina Faso in recent years. Notwithstanding artisanal gold mining contributes to poverty alleviation among rural communities through income generation, it causes a number of environmental concerns. These unmonitored activities profoundly affect land-use patterns, aggravate soil degradation and soil and water pollution (Essandoh et al., Citation2021; Torrance et al., Citation2021). Due to pedogenic processes, topography, bioclimatic conditions, underlying geology and land-use changes, soil environmental quality indicators usually show a strong spatial dependence (Babur et al., Citation2021). Therefore, a thorough evaluation of the spatial variability of the indicators at macro- and micro-scales and identification of their potential sources by using adequate modeling tools is crucial for setting up site-specific pollution monitoring strategies. However, conventional statistical techniques such univariate (e.g. coefficient of variation) and multivariate analyses (e.g. correlation analysis and factor analysis), which have been used to model spatial variability, are inadequate because they assume that the measured variables are independent of their spatial distribution (Hengl et al., Citation2015).
Alternatively, researchers have applied geostatistical techniques to interpolate the spatial variability of soil physico-chemical properties and to predict the values at unsampled locations (e.g. Bhunia et al., Citation2018; Ghong et al., Citation2023). The techniques can also be used to quantify spatial characteristics of agricultural lands so that the different landscape services (e.g. food supply, clean water and beautiful sceneries) can be mapped and sustainably managed (Aredehey et al., Citation2018; Hilal et al., Citation2024). The description of how spatial relationships change with the distance of separation between sampling points (lag distances) are accomplished through variogram analysis, which decomposes the spatial components of the variables into structural components that are used for interpolation. Ordinary kriging (OK) and cokriging (CK) are the interpolation techniques widely used to estimate spatial variability of soil physico-chemical properties (e.g. Delbari et al., Citation2019; Mousavi et al., Citation2017; Pirestani et al., Citation2024). Ordinary kriging is a geostatistical interpolation technique that uses statistical properties of sampled points (Isaaks & Srivastava, Citation1989), whereas CK uses one or more secondary variables to account for spatial cross-correlation between the primary and secondary variables (Goovaerts, Citation1998). As a result, CK can produce much more accurate and reliable estimation results than OK (Belkhiri et al., Citation2020; Khosravi & Balyani, Citation2019).
Soil physico-chemical properties like most environmental data often contain highly skewed values. In which case, applying indicator kriging (IK), a nonparametric type of conditional kriging and OK, may be a more appropriate geostatistical technique (Antunes & Albuquerque, Citation2013; Bradaï et al., Citation2016). As a result, IK model shows the quantified probability (0–1) of a variable that may exceed or not exceed a specified threshold value. That is, the expected value at an unsampled location derived from indicator data is equivalent to the cumulative distribution of the variable. Consequently, IK has been used to produce the probability maps of estimate values that exceed given limits such as regulatory threshold values (Belkhiri et al., Citation2017; Liang et al., Citation2018).
The main objectives of the work reported here were as follows: 1) to evaluate spatial variability of selected physico-chemical properties of surface soil samples in an area largely affected by cultivation and artisanal gold mining, 2) to determine the plausible sources of the spatial variability and 3) to identify areas with high environmental risks. The study is based on the hypothesis that land-use patterns such as farming and artisanal gold mining are the major driving factors of spatial variability of soil environmental quality. We have thought that a combination of geostatistical techniques (i.e. OK, CK and IK) will be appropriate for assessing spatial variability of the soil physico-chemical properties. The findings of the present study can help environmental geochemists in predicting behavior and fate of inorganic pollutants in highly anthropized semi-arid tropical soils at a relatively low cost.
2. Materials and methods
2.1. Study site and fieldwork
The study area (~42 km2) is located in southwestern Burkina Faso within the Bougouriba River drainage basin (Figure ). With a slop of 0.2 m/km and total length of 406 km, the Bougouriba River is the main watercourse in the area (Moniod et al., Citation1977). The area belongs to the South Sudanian climatic zone with predominant savannah vegetation. The regional annual rainfall varies between 900 and 2300 mm, whereas the average annual temperature is between 20.8 and 34.7°C (Forkuor et al., Citation2017). As far as the geology is concerned, the area is underlain by volcano-sedimentary rocks composed of andesite, tonalite and basalt with granitoid intrusion (Figure ). Under warm and humid climatic conditions, these geological formations are highly weathered, producing acidic soils with kaolinite as the dominant clay type. Hence, about 62% of the soil in the study area is classified as leached tropical ferruginous, 28% as latosols and 10% as hydromorphic soils with low SOM content (Hounkpatin et al., Citation2018). Artisanal gold mining is the main economic activity in the area. This unregulated activity includes explosive excavation and ore crushing, panning and mercury amalgamation, which have dramatic impacts on environmental soil quality. Subsistence (maize, sorghum, and yam) and cash crop (cotton) agricultures are the second largest land-use practice (Figure ).
Figure 1. Maps showing the study area (a), land occupation (b) and lithology with 132 topsoil sampling points from southwestern Burkina Faso (c).

In the present study, a total of 132 surface soil (0–30 cm) samples including 17 termite mounds were randomly collected using a stainless-steel scoop and stored in clean unused polyethylene bags. Back in the laboratory, the samples were dried at room temperature and sieved to 2 mm.
2.2. Soil physico-chemical property analyses
Both pHH2O and EC of the samples were measured in 1 g soil to 2.5 mL distilled water using a calibrated electronic multimeter, whereas CEC was determined by the neutral ammonium acetate method (Bain & Smith, Citation1987). Particle size distribution of the samples was characterized through sieving for silt and sand, and through hydrometric method for clay content. Soil organic carbon content was determined using the Walkley and Black dichromate oxidation method (Nelson & Sommers, Citation1982). The method consisted of adding concentrated H2SO4 to a mixture of soil and aqueous K2Cr2O7. After heating and oxidizing, the residual dichromate is back-titrated using ferrous sulfate (FeSO). The difference in added FeSO4 is compared with a blank titration and determines the amount of easily oxidizable OC. The percentage of OC was calculated as follows (Eq. 1):
Where M is the molarity of FeSO4 solution of blank, V1 is the volume (mL) of FeSO4 required in blank titration, V2 is the volume (mL) of FeSO4 required in sample titration, ms (g) is the mass of the soil samples and CF is the correction factor used to account for carbon that does not oxidize in the procedure. The soil organic matter content was then obtained by multiplying the percentage of OC by the Bemmelen factor of 1.724 according to the following equation (Eq 2; Jackson, Citation1958):
The total nitrogen content (TN) was determined using the Kjeldahl method (Bremner & Sparks, Citation1996). A sub-sample of each sample was digested with concentrated H2SO4 by using CuSO4 as a catalyst through which any nitrogen in the soil sample was converted into ammonia borate. Total nitrogen is then estimated by titrating ammonia borate with hydrochloric acid. Internal soil samples with known OC and TN content were analyzed along with the samples, and the recovery was better than 90%. Furthermore, three replicates were carried out for each analysis, and the relative standard deviations were less than 10%.
2.3. Exploratory data analysis
Soil physico-chemical property data were explored through a series of exploratory data analysis techniques. Thus, arithmetic mean and median were used to express the central tendency, whereas the spread of the data was evaluated through standard deviation (SD), maximum and minimum values. Traditionally, coefficient of variation (CV) has been used to evaluate the overall variability of soil physico-chemical properties (Castrignanò et al., Citation2000). The CV of a soil property is considered low if it is less than 15%, moderate if it is between 15 and 30% and high if it is greater than 35% (Wilding & Drees, Citation1978). Likewise, skewness and kurtosis were used to evaluate the data normality and asymmetry. Outliers and high skewness can affect negatively the spatial continuity of the variogram function. In order to provide stable variograms and kriging results, a transformation is considered as essential. The Pearson’s correlation analysis was used to investigate the relationship among the soil physico-chemical properties. The quantile-quantile (Q-Q) plots were used to test the normality of soil physico-chemical properties. In this study, pH, EC, TN and C/N did not show normal distribution, and they were log-transformed. All statistical analyses were performed using SPSS 20.0, PAST3 and Origin 2000 Pro.
2.4. Geostatistical analysis
2.4.1. Ordinary kriging and cokriging
Geostatistical techniques have been widely used to predict the spatial distribution of soil physico-chemical variables across unsampled areas based on the assumption that concentrations of a variable in closer samples are more spatially correlated than in those further away (Matheron, Citation1963). Kriging, in honor of Danie Krige, who first formulated this form of interpolation in 1951, has been extensively used as an important interpolation method in environmental studies. In the present study, OK was used to produce predictive maps of soil physico-chemical properties for unsampled locations. Some authorized theoretical models such as linear, exponential, spherical and Gaussian are usually used to fit the experimental semivariograms (Webster & Oliver, Citation2007). The semivariogram is expressed by using the Matheron’s classical estimator (Eq. 3).
Where Z(x) is the value of Z at location xi and Z(xi + h) is the value of Z at a location apart from xi by distance h and N(h) is the number of pairs of separated by h. Based on the exploratory data analysis, non-skewed data (less than 0.5), do not require transformation, whereas those with skewness value greater than 0.5 must be transformed before variogram analysis. There was no obviously anisotropy in semivariograms of soil physico-chemical properties in four different direction classes of 45°each. As a consequence, isotropic models were adjusted to fit to the data set of each individual parameter and to auto-fit one of the models (i.e. spherical, exponential, Gaussian or linear), and then select the best matching one.
The spatial structure of each model can be assessed using three variograms parameters: 1) nugget effect (C0), which is the value of the semivariogram when the distance is 0. The nugget describes the spatial variation caused by either natural or anthropogenic factors. 2) Sill (C0 + C), which is the value approaching the data variance, and it reflects the intensity of the total variation of regionalized variables, and higher values indicate higher degrees of variation. 3) The range (a) reflects the spatial autocorrelation of a regionalized variable and larger values indicate weaker spatial variability of the regionalized variable and stronger spatial correlation (Kashem & Singh, Citation2001; Matheron, Citation1963). The nugget to sill ratio also known as spatial dependence ratio (SDR) that is expressed as a percentage ((C0/C0 + C) ×100) was calculated for individual soil physico-chemical properties (Rosemary et al., Citation2017). A SDR value less than 25% indicates a strong spatial dependence, whereas values between 25% and 75% and higher than 75% indicate moderate and weak spatial dependences, respectively (Cambardella et al., Citation1994). Ordinary kriging was extended to cokriging when a strong correlation existed between a primary variable and a secondary variable.
2.4.2. Indicator kriging
Indicator kriging estimates the probability of exceeding specific threshold values, zk, at a given location (Goovaerts, Citation1997). In the geostatistical techniques, the probability of attribute value is not greater than a specific critical limit (Zk) was estimated using IK (Goovaerts, Citation1997). The threshold value or critical limit is selected arbitrary based on the case study at hand. In indicator kriging, the data, z(x), are transformed into an indicator variable as follows (Eq. 4):
At an unsampled location, the probability that z(x) ≤zk can be estimated using a linear combination of neighboring indicator variables. In IK, data values are transformed into indicator values. The transformation of the original data on values of probabilities was carried out as follows: the values lower than the median value were assigned 0 values and values equal or greater than the median value was transformed into 1. That is, the original values which exceed the selected threshold value are coded 1, and those below the threshold value are coded 0.
Then, the semivariogram γi (h) is used to quantify the spatial correlation of the indicator codes, i (xi, zk), and it is written as follows (Eq. 5):
Where h is the distance between both locations, xi and xi + h, and N (h) is the number of pairs for xi and xi+h. The indicator kriging estimator, i (xi; zk) at the location x0 can be calculated by (Eq. 6):
And the indicator kriging system is given the sum of λi as follows (Eq. 7)
Where λi is the weight coefficient, γi is the semi-variance of the indicator codes at the respective lag distance, and µ is the Lagrange multiplier.
The recommended international references for SOM and CEC contents (ITA, Citation1977; LCA, Citation2008) above which soils are likely to retain cationic nutrients and heavy metals (2% and 25 cmol/kg for SOM and CEC, respectively) were used to indicator variograms and probability maps of sensitive areas. That threshold values of pH (pH < 6.5) at which most cationic heavy metals (e.g. Pb, Cr and Cd) and oxyanion-forming elements such as Se, As and Sb (pH > 7.4) are mobile in soils are also used to delineate area at risk of heavy metal pollution.
2.4.3. Cross-validation
Accuracy of the kriging was evaluated through the leave-one-out cross-validation technique (Kerry et al., Citation2012). This technique is primarily used in scenarios where the objective is to predict accurately how a model will perform in practice. The cross-validation was conducted by visual inspection and optimization semivariogram parameters such as lag size (400) and lag number (12). The mean error (ME), mean standardized error (MSE), root mean square error (RMSE), root mean square standardized error (RMSSE) and average standard error (ASE) were the five validation parameters used to determine the best fitting models. ME values close to zero indicates unbiased predictions of soil physico-chemical properties, whereas any deviation of prediction values from measured values is evaluated by RMSE. An RMSE value close to that of ASE indicates that the assessment of prediction errors has been properly performed (Erdogan Erten et al., Citation2022; Nikroo et al., Citation2010). However, if RMSE is less than ASE, the variability of prediction errors is overestimated and the reverse case indicates an underestimation of error variability. The value of RMSSE should be close to 1. In the case where RMSE is greater than 1, the variability of prediction errors is underestimated, whereas a RMSSE value less than 1, then the variability of errors is overestimated (Ghazi et al., Citation2014; Nas & Berktay, Citation2010). When more than one model could be chosen for a variable, the choice was based the Akaike Information Criterion (Akaike, Citation1973). Geostatistical Analyst Extension within ArcMap 10.8 software was used to interpolate the spatial variability of soil physico-chemical properties and to produce the maps. For a given variable, all kriged maps were kept on the same scale in order to allow easier comparison.
3. Results and discussion
3.1. General soil physico-chemical properties
The spatial variability of soil physico-chemical properties is the result of intrinsic and extrinsic factors such as geological and pedogenic processes, fertilizer application, livestock grazing and mining (Bitencourt et al., Citation2016; Eljebri et al., Citation2018). Among the 10 soil physico-chemical properties used in this study, OC, SOM, TN, CEC, clay and silt data had skewness values less than 0.5 (; ), whereas EC, pH, C/N and sand were positively skewed (0.60–3). On the normal Q-Q plots, skewed variables deviated considerably from the normal distribution pattern (Figure ). After log-transformation, EC, pH, C/N and sand were normally distributed. In overall, soil physico-chemical properties exhibited high variabilities as highlighted by CV values (11–123%). However, there was a remarkable difference between physical properties and chemical properties. That is, the soil chemical properties, except pH (CV = 11%), exhibited relatively higher variabilities compared to physical properties (i.e. sand, silt and clay content). These high variabilities could be attributed to the local geology, residual fertilizers and vegetation cover (Ayele et al., Citation2019; Bogunovic et al., Citation2017). Nevertheless, the CV values of sand, silt and clay were greater than 30%, suggesting their high variabilities. This could be an indication of degradation of soil structure through tillage, deforestation and widespread artisanal gold mining in the study area (Bouasria et al., Citation2021; Eludoyin et al., Citation2017). With the application of acidifying fertilizers, intensive agriculture is also likely to impact other soil properties such as soil pH, EC and SOM content.
Figure 2. Normal Q-Q plots of soil physico-chemical properties collected in southwestern Burkina Faso: a) pH, b) EC, c) OC, d) SOM, e) N, f) C/N, g) CEC, h) clay, i) silt and j) sand.

Table 1. Univariate statistical summary of physico-chemical properties of topsoil samples from southwestern Burkina Faso
The soil pH in the present study varied from 5.1 to 9.7 (6.7 ± 0.7) with the majority of the samples (up to 75%) having pH less than 7, indicating that the soils were mostly acidic to circumneutral (Penn & Camberato, Citation2019). The low soil pH values are generally inherent to tropical soils that are the byproducts of intensive chemical weathering, depletion of alkali and alkaline earth elements (Latifah et al., Citation2017; Russell et al., Citation2017). The absence of these elements in the soil is corroborated by the relatively low soil EC (Rangel-Peraza et al., Citation2017). Thus, the recorded soil EC ranged from 9 to 625 µS/cm with an average value of 85.0 ± 102.5 µS/cm. According to Richards’s salinity classification (Richards, Citation1954), the measured EC values were low with a large difference between the median and average values (85.02 µS/cm versus 48.60 µS/cm). However, a moderate positive correlation (r = 0.53; p < 0.05) between pH and EC () suggests that soil pH controls ion abundance in soil solution (Penn & Camberato, Citation2019). The low pH values may also affect the rates of biological activities, and thereby soil C/N ratios.
Overall, the soil C/N ratios ranged from 5.9 to 17.98 with 75% of the samples having C/N ratios less than 12. According to the LCA guidelines (LCA, Citation2008), C/N ratios comprised between 8 to 12 indicate good organic matter decomposition, with ratios greater than 12 imply that activities of soil microbial community is reduced and the organic matter decomposition rate is slow. The areas with high C/N ratios (~25%) greater than 12 are characterized by low biological activities, which could be attributed to low dissolved oxygen content and pH (Z. Xu et al., Citation2020; Zhao et al., Citation2018). The SOM content of the present study ranged from 0.33 to 3.3% (1.7 ± 0.6%) with 50% of soil samples having moderate SOM content (~2%; ITA, Citation1977). This is an indication of good biological activities and organic matter decomposition rates. Soil organic matter plays a prominent role in mobility and biogeochemical cycling of heavy metals in the soil environment (Kwiatkowska-Malina, Citation2018; Zhu et al., Citation2018). Thus, in the presence of high SOM content in the upper horizon, certain heavy metals, particularly Pb, tend to be accumulated in the soil (Y. Li et al., Citation2017). Consequently, SOM could be a limiting factor in immobilizing heavy metals in the present soils. As expected, the soil OC contents were relatively lower than that of SOM, and it ranged from 0.19 to 1.93% (0.99 ± 0.3%). About 75% of the soil samples had low TN content (<0.11%; Batjes, Citation2014). The highly positive correlations (), observed in the present work, between SOM and OC contents and between OC and TN content suggests that OC and TN contents are largely controlled by the supply of readily decomposable organic materials (Bongiorno et al., Citation2019). Furthermore, the significant correlation between OC and TN contents is an indication of soil C/N stabilization in the plow layer (Kopittke et al., Citation2020; Y. Wang et al., Citation2018). Similar close relationships have been observed in terrestrial ecosystems with different land-use or management practices (Ferreira et al., Citation2016; T. Wang et al., Citation2016).
On the textural triangle, the majority of the soil samples was projected in the clayey and silty-clayey textural classes (). Under intense and long-term chemical weathering of crystalline bedrocks, one could expect a predominance of sand and residual Fe and Al oxide minerals (Souza et al., Citation2018). However, the observed textural classes could be a result of tillage, water and erosional redistribution of fine-grained particles from nearby upland areas to deposition areas () in the study area (Berhe & Torn, Citation2016; Nie et al., Citation2016). The soil CEC, which varied broadly across the study area (4.9–38.6 cmol/kg), showed a strong positive correlation with clay (r = 0.94, p < 0.05). This indicates that CEC is solely related to the local clay type (Pincus et al., Citation2017; Ramos et al., Citation2018). The recorded CEC values fall within the range of kaolinite (3–57 cmol/kg; Kabata-Pendias, Citation2011).
3.2. Structural characteristics of soil physico-chemical properties
Isotropic semivariogram models were fitted to the raw and log-transformed soil physico-chemical property data (). That is, the Gaussian model was best-fitted for pH, OC, C/N, CEC and sand, whereas exponential model was more adequate for EC, SOM and clay, and the spherical model for TN and silt. For all samples, RMSE values were close to 1 (1.00–0.96) and MSE values were low (−0.009742–0.0174), which indicated that the fitted variograms and subsequent kriging standard errors were satisfactory (). Thus, OK could successfully interpolate pH, OC, SOM, C/N, CEC and silt with minimum RMSE (0.04–9.3) and ASE (0.04–9.3) values, whereas EC, clay and sand showed larger uncertainty with maximum RMSE (98, 11 and 15) and ASE (139, 11 and 17) values. Furthermore, the low ME values, except for EC, indicated a good match between observed and predicted soil physico-chemical properties.
Figure 5. Experimental semivariograms for a) log-transformed pH, b) log-transformed EC, c) OC, d) SOM, e) TN, f) log-transformed C/N, g) CEC, h) clay, i) silt and j) log-transformed sand based on cross validation analysis.

Table 2. Best-fitted variogram parameters and cross-validation prediction errors for ordinary kriging (OK) of soil physico-chemical properties
Five variables (pH, TN, C/N, OC and sand) showed very low nugget effects (0.007–0.105). In contrast, clay, silt and CEC (96, 75 and 14.3) showed the highest nugget effects, indicating a random spatial distribution behavior of the variables at short range (Song et al., Citation2018). The large nugget effect suggests that the lag distance did not characterize the spatial variations, and thus an additional sampling of the variable at smaller lag distances and in larger number is required. Another factor that may explain the large nugget effect is the subscale variability of the variable (Guedes et al., Citation2020). In other words, the random distribution may be attributed to the presence of extremely high or extremely low values that are outliers or to sampling design.
The range was used to reflect the distance of spatial correlation for the soil physico-chemical properties (Bitencourt et al., Citation2016). Electrical conductivity, TN and clay were autocorrelated in longer ranges than pH, OC, SOM and silt. This is consistent with the high CV values of EC, TN and clay. The SDR of the individual soil properties were in the following decreasing order (Table ): The SDR of the individual soil properties were in the following decreasing order (Table ): silt (96%)> TN=C/N(88%)>OC=clay (81%)>SOM (75%)>sand (65%)> pH (63%)>EC(55%)> CEC (24). All the ratios, except that of CEC, were greater than 25%, implying that the spatial dependence was weak to moderate. With SDR values greater than 75%, silt, TN, C/N, clay and OC had weak spatial dependence, implying that the spatial distribution of these soil properties was only controlled by extrinsic factors such as biological activity and land-use pattern (Eljebri et al., Citation2018; Phefadu & Kutu, Citation2016). Conversely, SDR values of EC, SOM, sand and pH comprised between 25 and 75%, and were therefore considered to be moderately dependent. The spatial distribution of these chemical properties can be attributed to both intrinsic and extrinsic factors. Thus, EC, pH and sand had SDR slightly over 50%, which suggests that the extrinsic factors, such as tillage, vegetation cover and biological activities were the most controlling factors in the spatial distribution of these variables. Soil CEC was the only soil property with strong spatial dependency in the study area. This implies that the soil CEC might have been controlled by intrinsic factors such as the dominant clay type (i.e. kaolinite).
3.3. Spatial distribution of soil physico-chemical properties
Semivariogram parameters were used to produce spatial distribution maps of the individual soil physico-chemical properties through OK (). In the study area, about 87% of the total surface area is covered by slightly acidic to circumneutral soils (pH = 6.1–7.3; ) in farming dominant northern and eastern parts, whereas neutral to alkaline soils (pH = 7.4–8.7) occupied about 7% of the area n northwest, and acidic soils (~6%) were found mainly in the southeast. In addition to anthropogenic activities, the spatial distribution patterns of pH could be partially attributed to the local lithology, low inputs of fresh organic material, removal of basic nutrients and continuous use of ammonium fertilizers (Khadka et al., Citation2018; Khan et al., Citation2019).
Spatial distribution of EC varied widely across the study area with the high values (69–167 µS/cm) confined in northwest, and mainly occupied by farmlands, whereas the rest of the study area was characterized by low EC values (17–69 µS/cm; ). The pedogeochemical signature of these soils could be directly related to the prevailing agricultural practices in the area. Excessive application of inorganic fertilizers could considerably increase soil background concentrations in certain ions. Thus, the underlying causes of the spatial distribution of EC are extrinsic (e.g. land-use) factors.
The soil samples with moderate SOM content were collected in the central and southeastern parts of the study area (), whereas the soil samples with low SOM content were mainly collected in farmland. Clearly, the spatial distribution pattern of soil SOM was primarily controlled by farming. It is noteworthy that soils samples with moderate TN content were collected in areas characterized by natural vegetation cover, whereas those with low TN content were located in farmlands (), indicating the influence of tillage on the soil organic matter content. Moreover, soil samples with moderate to high C/N ratios (<12; LCA, Citation2008) were spread around artisanal gold mining sites and farmland (), whereas those with very high ratios (>12) were mainly collected around human settlements and the textile factory. Therefore, it can be concluded that biological activities and organic matter decomposition rates were directly associated with land-use patterns. Again, this indicates that particular attention should be paid to farmland and artisanal gold mining sites as far as inorganic pollutant mobility is concerned.
Patches of soils with high CEC content (25–37.4 cmol/kg) were scattered throughout the study area (). Moreover, the clay distribution showed a significant spatial correlation with CEC (), which is consistent with previous studies that indicated that soil CEC is directly dependent on clay types. Soils with a high clay content (35–43%) were collected around the artisanal gold mining sites and along the river bank (). Based on the significant positive correlations between CEC and clay and between SOM, TN and OC, the spatial distribution of CEC and SOM was mapped using CK. Soil CEC and SOM were used as primary variables, whereas clay, OC and TN as secondary variables. Nonetheless, the ME and RMSE values of OK for SOM were lower than those of CK, suggesting that CK was more accurate and reliable in predicting SOM. In contrast, ME and RMSE values of OK for CEC were lower than those of CK. Hence, in the present study CK was less accurate in estimating CEC and clay (; ). Therefore, there is no need to determine TN and OC in future monitoring programs as SOM can be used instead.
Table 3. Best-fitted variogram parameters of ordinary kriging (OK) and cokriging (CK) of CEC and SOM as primary variables and clay. TN and OC as secondary variables
3.4. Delineation of environmentally high risk areas
Identification of areas at high risk of heavy metal pollution is urgently required for a thorough assessment of their behavior and fate in highly impacted rural soils. Soil physico-chemical properties (e.g. pH, CEC and SOM) play a critical role in mobility and bioavailability of heavy metals in the soil environment (Antoniadis et al., Citation2017; Gao et al., Citation2016). Several studies have reported that pH contributes to metal mobility from adsorption sites to soil solution and their subsequent uptake by organisms (Jović et al., Citation2017; Pikuła & Stępień, Citation2021; D. M. Xu et al., Citation2021). This is likely due to the twofold effects of pH on mobility and speciation of most heavy metals (Jarvela-Reijonen et al., Citation2016; Korfali & Karaki, Citation2018). Estimated probability maps of soils physico-chemical properties were produced by IK using published threshold values (LCA, Citation2008). A Map of land occupation was used to highlight spatial distribution the different soil physico-chemical properties at risk of heavy metal mobility and toxicity (Figure ).
Figure 8. Estimated probability maps of soil physico-chemical properties indicating areas at risk of exceeding or not exceeding environmental threshold values with relation to farmlands. a) land occupation, b) pH < 6.5, c) pH > 7.4, d) CEC < 25 cmol/kg and e) SOM < 2 %.

About 29% of the sampled area, particularly, in northern and eastern parts had high probability (50–100%) of not exceeding the pH value (<6.5) at which most heavy metals are not mobile and toxic (Figure ; Table ; Sintorini et al., Citation2021). By contrast, about 2% of the soils in the northwestern part (Figure ) of the study had high probability (50–75%) of exceeding the threshold value (pH = 7.4) above which the mobility of most oxyanion-forming elements such as Cr, Se and As can be enhanced (Kodirov et al., Citation2018; Verbeeck et al., Citation2019). This suggests that about 31% of the total areas was at risk of heavy metal mobility (metallic and metalloid elements).
Table 4. Percentage areas at risk of not exceeding (SOM. CEC and pH < 6.5) or exceeding (pH > 7.4) threshold values with respect to the total surface of the study area
As far as CEC is concerned, 77% of the total area was unlikely (50–100%) to have CEC values above 25 cmol/kg (Figure ), suggesting that soils in these areas are characterized by low cation retention capacity. The adsorption capacity of heavy metals onto soils is a function of surface charges, ionic strength and clay mineralogy (Anjolaiya, Citation2015; Sarkar et al., Citation2017; Uddin, Citation2017). In contrast to temperate soils with dominant illite (2:1 clay types) and permanent structural charges, highly weathered tropical soils with dominant kaolinite (1:1 clay types) are characterized by pH-variable surface charges (Chen et al., Citation2017; Colzato et al., Citation2018; Singh et al., Citation2016). That is, the adsorptive capacity of soils in areas with relatively high CEC (>25 cmol/kg) may be reduced at small declines in pH.
Similarly, 75.61% of the study area had high probability (50–100%) of not exceeding the SOM threshold (<2%; Figure ). These soils are therefore considered to be relatively poor in SOM and would not be capable of retaining cationic elements including potentially toxic heavy metals (M. Huang et al., Citation2016; Lwin et al., Citation2018). In that sense, protonation (high H+) and deprotonation (high OH−) of soil surfaces are greatly influenced by SOM content as organic matter balances both acid and base addition to the soil solution. Less than 1% of the total surface area had low probability (0–25%) to be below the threshold value of 2% that will allow heavy metal retention. Soil organic matter is deprotonated at high pH and therefore can adsorb cationic heavy metals, whereas at low pH, SOM is protonated and previously adsorbed cations will be released into soil solution. This protonation will pose serious bioenvironmental concerns as previously retained heavy metals may be released through desorption into the soil solution (M. Huang et al., Citation2019). Based on low pH, low CEC low and SOM content of large portion of the study area, it can be deduced that the studied soils would be vulnerable to anthropogenically derived heavy metals.
Since agricultural soils are characterized by high physical and chemical properties, homogenous management of agricultural fields may lead to overapplication of fertilizers in high-yielding areas and underapplication of fertilizers in low-yielding areas (Bhatt et al., Citation2018; Q. Li et al., Citation2021; Nadarajan & Sukumaran, Citation2021). After establishing spatial dependence through geostatistical modeling, isarithmic maps for spatial distribution of CEC, pH, SOM and clay can be generated. These maps can be used to categorize areas with low, medium and high physico-chemical properties for site-specific soil fertility management (Silva et al., Citation2023). Not only quantifying spatial variability of physico-chemical properties will increase agricultural yield, but it will also allow better management of natural resources and environmental pollution control. That is, the site-specific field management minimize landscape degradation, eutrophication, soil and water resource contamination through excess inorganic fertilizers.
4. Conclusions
Exploratory data analysis showed that soil chemical properties, except pH, were more variable compared to physical properties. This is due to both intrinsic and extrinsic factors such lithology, chemical weathering, vegetation cover, biological activities and land-use pattern. The pronounced low pH across the study area is likely associated with lithology and pedogenic process and agricultural practices. Due to the crystalline nature of the local lithology, one could expect dominant sandy soil rather than the observed clayey and silty soils. This discrepancy reflects transport and deposition of fine-grained particle from human-impacted agricultural and mining areas to lowlands along the river bank. Cokriging was the best method for modeling spatial variability of SOM, OC and TN. Indicator kriging showed that most of the soil samples had probability of exceeding threshold values for pH, CEC and SOM at which most cationic heavy metals will be mobile and bioavailable. The study also showed that through a combination of geostatistical approaches, the routine soil physico-chemical properties can be used to predict areas at risk of heavy metal pollution so that robust pollution control strategies can be set up.
Availability of data
The data generated and/or analyzed to support the findings of the study is available from the corresponding author, Sako, A., up on reasonable request.
Disclosure statement
No potential conflict of interest was reported by the author(s).
Additional information
Funding
References
- Aharonov-Nadborny, R., Tsechansky, L., Raviv, M., & Graber, E. R. (2017). Impact of spreading olive mill waste water on agricultural soils for leaching of metal micronutrients and cations. Chemosphere, 179, 213–22. https://doi.org/10.1016/j.chemosphere.2017.03.093
- Akaike, H. (1973). Information theory as an extention of the maximum likelihood principle. In B. Petrov & F. Csaki (Eds.), Int symp on information theory (2nd ed., pp. 267–281). Akademiai Kiadi.
- Al-Wabel, M. I., Usman, A. R. A., Al-Farraj, A. S., Ok, Y. S., Abduljabbar, A., Al-Faraj, A. I., & Sallam, A. S. (2019). Date palm waste biochars alter a soil respiration, microbial biomass carbon, and heavy metal mobility in contaminated mined soil. Environmental Geochemistry and Health, 41(4), 1705–1722. https://doi.org/10.1007/s10653-017-9955-0
- Anjolaiya, O. (2015). Sorption Behaviour of Metal Contaminants in Clay Minerals, Soils and Matrices: Understanding the Influence of Organic Matter, pH, Ionic Strength and Mineralogy [ Doctoral dissertation], Loughborough University.
- Antoniadis, V., Levizou, E., Shaheen, S. M., Ok, Y. S., Sebastian, A., Baum, C., Prasad, M. N. V., Wenzel, W. W., & Rinklebe, J. (2017). Trace elements in the soil-plant interface: Phytoavailability, translocation, and phytoremediation–A review. Earth-Science Reviews, 171, 621–645. https://doi.org/10.1016/j.earscirev.2017.06.005
- Antunes, I. M. H. R., & Albuquerque, M. T. D. (2013). Using indicator kriging for the evaluation of arsenic potential contamination in an abandoned mining area (Portugal). Science of the Total Environment, 442, 545–552. https://doi.org/10.1016/j.scitotenv.2012.10.010
- Anza, M., Garbisu, C., Salazar, O., Epelde, L., Alkorta, I., & Martínez-Santos, M. (2021). Acidification alters the functionality of metal polluted soils. Applied Soil Ecology, 163, 1–9. https://doi.org/10.1016/j.apsoil.2021.103920
- Aredehey, G., Libsekal, H., Brhane, M., Welde, K., Giday, A., & Tejada Moral, M. (2018). Top-soil salinity mapping using geostatistical approach in the agricultural landscape of Timuga irrigation scheme, South Tigray, Ethiopia. Cogent Food & Agriculture, 4(1), 1–13. https://doi.org/10.1080/23311932.2018.1514959
- Ashraf, M. A., Hussain, I., Rasheed, R., Iqbal, M., Riaz, M., & Arif, M. S. (2017). Advances in microbe-assisted reclamation of heavy metal contaminated soils over the last decade: A review. Journal of Environmental Economics and Management, 198, 132–143. https://doi.org/10.1016/j.jenvman.2017.04.060
- Ayele, G. T., Demissie, S. S., Jemberrie, M. A., Jeong, J., & Hamilton, D. P. (2019). Terrain effects on the spatial variability of soil physical and chemical properties. Soil Systems, 4(1), 1–21. https://doi.org/10.3390/soilsystems4010001
- Babur, E., Süha Uslu, Ö., Leonardo Battaglia, M., Diatta, A., Fahad, S., Datta, R., Zafar-Ul-Hye, M., Hussein, G. S., & Danish, S. (2021). Studying soil erosion by evaluating changes in physico-chemical properties of soils under different land-use types. Journal of the Saudi Society of Agricultural Sciences, 20(3), 190–197. https://doi.org/10.1016/j.jssas.2021.01.005
- Bain, D. C., & Smith, B. E. L. (1987). Chemical analysis. In M. J. Wilson, G. Blackie (Eds.), A handbook of determinative methods in clay mineralogy (pp. 248–274).
- Bamba, O., Pélédé, S., Sako, A., Kagambega, A., & Miningou, M. (2013). Impact de l’artisanat minier sur les sols d’un environnement agricole aménagé au Burkina Faso. Journal de sciences, 13, 1–11.
- Batjes, N. H. (2014). Total carbon and nitrogen in the soils of the world. The European Journal of Soil Science, 65(1), 10–21. https://doi.org/10.1111/ejss.12114_2
- Belkhiri, L., Mouni, L., Narany, T. S., & Tiri, A. (2017). Evaluation of potential health risk of heavy metals in groundwater using the integration of indicator kriging and multivariate statistical methods. Groundwater for Sustainable Development, 4, 12–22. https://doi.org/10.1016/j.gsd.2016.10.003
- Belkhiri, L., Tiri, A., & Mouni, L. (2020). Study of the spatial distribution of groundwater quality index using geostatistical models. Groundwater for Sustainable Development, 11, 1–9. https://doi.org/10.1016/j.gsd.2020.100473
- Berhe, A. A., & Torn, M. S. (2016). Erosional redistribution of topsoil controls soil nitrogen dynamics. Biogeochemistry, 132(1–2), 37–54. https://doi.org/10.1007/s10533-016-0286-5
- Bhatt, M. K., Raverkar, K. P., Labanya, R., & Bhatt, C. K. (2018). Effects of long-term balanced and imbalanced use of inorganic fertilizers and organic manure (FYM) on soil chemical properties and yield of rice under rice-wheat cropping system. Journal of Pharmacognosy and Phytochemistry, 7(3), 703–708.
- Bhunia, G. S., Shit, P. K., & Chattopadhyay, R. (2018). Assessment of spatial variability of soil properties using geostatistical approach of lateritic soil (West Bengal, India). Annals of Agrarian Science, 16(4), 436–443. https://doi.org/10.1016/j.aasci.2018.06.003
- Bitencourt, D. G. B., Barros, W. S., Timm, L. C., She, D., Penning, L. H., Parfitt, J. M. B., & Reichardt, K. (2016). Multivariate and geostatistical analyses to evaluate lowland soil levelling effects on physico-chemical properties. Soil and Tillage Research, 156, 63–73. https://doi.org/10.1016/j.still.2015.10.004
- Bogunovic, I., Trevisani, S., Seput, M., Juzbasic, D., & Durdevic, B. (2017). Short-range and regional spatial variability of soil chemical properties in an agro-ecosystem in eastern Croatia. Catena, 154, 50–62. https://doi.org/10.1016/j.catena.2017.02.018
- Bongiorno, G., Bünemann, E. K., Oguejiofor, C. U., Meier, J., Gort, G., Comans, R., Mäder, P., Brissard, L., & de Goede, R. (2019). Sensitivity of labile carbon fractions to tillage and organic matter management and their potential as comprehensive soil quality indicators across pedoclimatic conditions in Europe. Ecological Indicators, 99, 38–50. https://doi.org/10.1016/j.ecolind.2018.12.008
- Bouasria, A., Namr, K. I., Rahimi, A., & Ettachfini, E. M. (2021). Geospatial assessment of soil organic matter variability at Sidi Bennour District in Doukkala Plain in Morocco. Journal of Ecological Engineering, 22(11), 120–130. https://doi.org/10.12911/22998993/142935
- Bradaï, A., Douaoui, A., Bettahar, N., & Yahiaoui, I. (2016). Improving the prediction accuracy of groundwater salinity mapping using indicator kriging method. Journal of Irrigation & Drainage Engineering, 142(7), 1–11. https://doi.org/10.1061/(ASCE)IR.1943-4774.0001019
- Bremner, J. M., & Sparks, D. (1996). Methods of soil analyses part 3: Chemical analyses.
- Cambardella, C. A., Moorman, T. B., Novak, J. M., Parkin, T. B., Karlen, D. L., Turco, R. F., & Konopka, A. E. (1994). Field-scale variability of soil properties in central Iowa soils. Soil Science Society of America Journal, 58(5), 1501–151. https://doi.org/10.2136/sssaj1994.03615995005800050033x
- Castrignanò, A., Giugliarini, L., Risaliti, R., & Martinelli, N. (2000). Study of spatial relationships among some soil physico-chemical properties of a field in central Italy using multivariate geostatistics. Geoderma, 97(1–2), 39–60. https://doi.org/10.1016/S0016-7061(00)00025-2
- Chen, H., Koopal, L. K., Xiong, J., Avena, M., & Tan, W. (2017). Mechanisms of soil humic acid adsorption onto montmorillonite and kaolinite. Journal of Colloid and Interface Science, 504, 457–467. https://doi.org/10.1016/j.jcis.2017.05.078
- Colzato, M., Alleoni, L. R. F., & Kamogawa, M. Y. (2018). Cadmium sorption and extractability in tropical soils with variable charge. Environmental Monitoring and Assessment, 190(6), 1–10. https://doi.org/10.1007/s10661-018-6666-7
- Compaore, W. F., Dumoulin, A., & Rousseau, D. P. L. (2019). Gold mine impact on soil quality, Youga, Southern Burkina Faso, West Africa. Water, Air, & Soil Pollution, 230(8), 1–14. https://doi.org/10.1007/s11270-019-4257-z
- da Silva, R. J. A. B., da Silva, Y. J. A. B., van Straaten, P., Araújo Do Nascimento, C. W., Biondi, C. M., da Silva, Y. J. A. B., & de Araújo Filho, J. C. (2022). Influence of parent material on soil chemical characteristics in a semi-arid tropical region of Northeast Brazil. Environmental Monitoring and Assessment, 194(5), 331. https://doi.org/10.1007/s10661-022-09914-9
- Delbari, M., Afrasiab, P., Gharabaghi, B., Amiri, M., & Salehian, A. (2019). Spatial variability analysis and mapping of soil physical and chemical attributes in a salt-affected soil. Arabian Journal of Geosciences, 12(3), 1–18. https://doi.org/10.1007/s12517-018-4207-x
- Eljebri, S., Mounir, M., Faroukh, A. T., Zouahri, A., & Tellal, R. (2018). Application of geostatistical methods for the spatial distribution of soils in the irrigated plain of Doukkala, Morocco. Modeling Earth Systems and Environment, 5(2), 669–687. https://doi.org/10.1007/s40808-018-0558-2
- Eludoyin, A. O., Ojo, A. T., Ojo, T. O., Awotoye, O. O., Nzeadibe, T., & Nzeadibe, T. (2017). Effects of artisanal gold mining activities on soil properties in a part of southwestern Nigeria. Cogent Environmental Science, 3(1), 1–11. https://doi.org/10.1080/23311843.2017.1305650
- Erdogan Erten, G., Yavuz, M., & Deutsch, C. V. (2022). Combination of machine learning and kriging for spatial estimation of geological attributes. Natural Resources Research, 31(1), 191–213. https://doi.org/10.1007/s11053-021-10003-w
- Essandoh, P. K., Takase, M., Bryant, I. M., & Cameselle, C. (2021). Impact of small-scale mining activities on physicochemical properties of soils in Dunkwa East Municipality of Ghana. The Scientific World Journal, 2021, 1–13. https://doi.org/10.1155/2021/9915117
- Ferreira, A. C. C., Leite, L. F. C., de Araújo, A. S. F., & Eisenhauer, N. (2016). Land-use type effects on soil organic carbon and microbial properties in a semi-arid region of Northeast Brazil. Land Degradation and Development, 27(2), 171–178. https://doi.org/10.1002/ldr.2282
- Forkuor, G., Hounkpatin, O. K. L., Welp, G., Thiel, M., & Hui, D. (2017). High resolution mapping of soil properties using remote sensing variables in south-western Burkina Faso: A comparison of machine learning and multiple linear regression models. Public Library of Science ONE, 12(1), 1–21. https://doi.org/10.1371/journal.pone.0170478
- Gao, J., Mahapatra, C. T., Mapes, C. D., Khlebnikova, M., Wei, A., & Sepúlveda, M. S. (2016). Vascular toxicity of silver nanoparticles to developing zebrafish (danio rerio). Nanotoxicology, 10(9), 1363–1372. https://doi.org/10.1080/17435390.2016.1214763
- Ghazi, A., Moghadas, N., Sadeghi, H., Ghafoori, M., & Lashkaripour, G. (2014). Spatial variability of shear wave velocity using geostatistical analysis in Mashhad city, NE Iran. Open Journal of Geology, 4(8), 354–363. https://doi.org/10.4236/ojg.2014.48027
- Ghong, N. P., Ngwabie, N. M., Asongwe, G. A., Kedia, A. C., & Suh, C. E. (2023). An assessment and geostatistics of land-use and selected physico-chemical properties of soils in the Mount Cameroon Area. Journal of Geographic Information System, 15(2), 244–266. https://doi.org/10.4236/jgis.2023.152013
- Goovaerts, P. (1997). Kriging vs. stochastic simulation for risk analysis in soil contamination. In A. Soares, J. Gomez-Hernandez, & R. Froidevaux (Eds.), geoENV I — geostatistics for environmental applications (pp. 247–258). Kluwer Academic Publishers.
- Goovaerts, P. (1998). Geostatistical tools for characterizing the spatial variability of microbiological and physico-chemical soil properties. Biology and Fertility of Soils, 27(4), 315–334. https://doi.org/10.1007/s003740050439
- Graber, E. R., Singh, B., Hanley, K., & Lehmann, J. (2017). Determination of cation exchange capacity in biochar. In B. Singh, M.-C. Arbestain, & J. Lehmann (Eds.), Biochar: A Guide to analytical methods (pp. 74–84). CRC Press LLC.
- Guedes, L. P. C., Bach, R. T., & Uribe-Opazo, M. A. (2020). Nugget effect influence on spatial variability of agricultural data. Engenharia Agricola, 40(1), 96–104. https://doi.org/10.1590/1809-4430-eng.agric.v40n1p96-104/2020
- Hampl, F. J., Schiperski, F., Schwerdhelm, C., Stroncik, N., Bryce, C., Friedhelm von Blanckenburg, F., & Neumann, T. (2023). Feedbacks between the formation of secondary minerals and the infiltration of fluids into the regolith of granitic rocks in different climatic zones (Chilean coastal Cordillera). Earth Surface Dynamics, 11(3), 511–528. https://doi.org/10.5194/esurf-11-511-2023
- Helliwell, J. R., Miller, A. J., Whalley, W. R., Mooney, S. J., & Sturrock, C. J. (2014). Quantifying the impact of microbes on soil structural development and behaviour in wet soils. Soil Biology and Biochemistry, 74, 138–147. https://doi.org/10.1016/j.soilbio.2014.03.009
- Hengl, T., Heuvelink, G. B. M., Kempen, B., Leenaars, J. G. B., Walsh, M. G., Shepherd, K. D., Sila, A., MacMillan, R. A., Mendes de Jesus, J., & Tondoh, J. E. (2015). Mapping soil properties of Africa at 250 m resolution: Random forests significantly improve Current predictions. Public Library of Science ONE, 10(6), 1–26. https://doi.org/10.1371/journal.pone.0125814
- Hilal, A., Bangroo, S. A., Kirmani, N. A., Wani, J. A., Biswas, A., Bhat, M. I., Farooq, K., Bashir, O., & Shah, T. I. (2024). Chapter 19 - geostatistical modeling—a tool for predictive soil mapping. Remote Sensing in Precision Agriculture, 389–418.
- Hounkpatin, O. K. L., Op de Hipt, F., Bossa, A. Y., Welp, G., & Amelung, W. (2018). Soil organic carbon stocks and their determining factors in the dano catchment (Southwest Burkina Faso). Catena, 166, 298–309. https://doi.org/10.1016/j.catena.2018.04.013
- Huang, B., Li, Z., Huang, J., Chen, G., Nie, X., Ma, W., Yao, H., Zhen, J., & Zeng, G. (2015). Aging effect on the leaching behavior of heavy metals (cu, zn, and cd) in red paddy soil. Environmental Science and Pollution Research, 22(15), 11467–11477. https://doi.org/10.1007/s11356-015-4386-x
- Huang, M., Li, Z., Chen, M., Wen, J., Xu, W., Ding, X., Yang, R., Luo, N., & Xing, W. (2019). In situ investigation of intrinsic relationship between protonation behavior and HA characteristics in sediments. Science of the Total Environment, 683, 258–266. https://doi.org/10.1016/j.scitotenv.2019.05.235
- Huang, M., Zhu, Y., Li, Z., Huang, B., Luo, N., Liu, C., & Zeng, G. (2016). Compost as a soil amendment to remediate heavy metal-contaminated agricultural soil: Mechanisms, efficacy, problems, and strategies. Water, Air, & Soil Pollution, 227(10), 1–18. https://doi.org/10.1007/s11270-016-3068-8
- Isaaks, E. H., & Srivastava, R. M. (1989). An introduction to applied Geostatistics. Oxford University Press.
- ITA. (1977). Laboratoire du sol : méthode d’analyses physiques et chimiques du sol (3ème ed.). Mostaganem.
- Jackson, M. L. (1958). Soil chemical analysis. Prentice Hall., Inc.
- Jarvela-Reijonen, E., Karhunen, L., Sairanen, E., Rantala, S., Laitinen, J., Puttonen, S., Peuhkuri, K., Hallikainen, M., Juvonen, K., Myllymaki, T., Fohr, T., Pihlajamaki, J., Korpela, R., Ermes, M., Lappalainen, R., & Kolehmainen, M. (2016). High perceived stress is associated with unfavorable eating behavior in overweight and obese Finns of working age. Appetite, 105, 249–258. https://doi.org/10.1016/j.appet.2016.04.023
- Jiang, Y., Ma, X. L., Sun, F. Y., Guo, Y. X., Ren, L. J., Wang, Y. J., & Xie, Z. L. (2021). A comparative study on the adsorption properties of heavy metal cr in lake sediment and soil. Applied Ecology and Environmental Research, 19(2), 901–914. https://doi.org/10.15666/aeer/1902_901914
- Jović, M., Šljivić-Ivanović, M., Dimović, S., Marković, J., & Smičiklas, I. (2017). Sorption and mobility of Co(II) in relation to soil properties. Geoderma, 297, 38–47. https://doi.org/10.1016/j.geoderma.2017.03.006
- Kabata-Pendias, A. (2011). Trace elements in soils and plants (4th ed.). Taylor and Francis Group.
- Kashem, M. A., & Singh, B. R. (2001). Metal availability in contaminated soils: I. Effects of flooding and organic matter on changes in eh, pH and solubility of cd, ni and Zn. Nutrient Cycling in Agroecosystems, 61(3), 247–255. https://doi.org/10.1023/A:1013762204510
- Kerry, R., Goovaerts, P., Rawlins, B. G., & Marchant, B. P. (2012). Disaggregation of legacy soil data using area to point kriging for mapping soil organic carbon at the regional scale. Geoderma, 170, 347–358. https://doi.org/10.1016/j.geoderma.2011.10.007
- Khadka, D., Lamichhane, S., Bhantana, P., Ansari, A. R., Joshi, S., & Barwal, P. (2018). Soil fertility assessment and mapping of chungbang farm, pakhribas, dhankuta, Nepal. Advances in Plants & Agriculture Research, 8(3), 219–227. https://doi.org/10.15406/apar.2018.08.00317
- Khaledian, Y., Brevik, E. C., Pereira, P., Cerda, A., Fattah, M. A., & Tazikeh, H. (2017). Modeling soil cation exchange capacity in multiple countries. Catena, 158, 194–200. https://doi.org/10.1016/j.catena.2017.07.002
- Khan, M. Z., Islam, M. A., Sadiqul Amin, M., & Bhuiyan, M. M. R. (2019). Spatial variability and geostatistical analysis of selected soil. Bangladesh Journal of Scientific and Industrial Research, 54(1), 55–66. https://doi.org/10.3329/bjsir.v54i1.40731
- Khosravi, Y., & Balyani, S. (2019). Spatial modeling of mean annual temperature in Iran: Comparing cokriging and Geographically Weighted Regression. Environmental Modeling & Assessment, 24(3), 341–354. https://doi.org/10.1007/s10666-018-9623-5
- Kicińska, A., Pomykała, R., & Izquierdo-Diaz, M. (2021). Changes in soil pH and mobility of heavy metals in contaminated soils. European Journal of Soil Science, 73(1), 1–14. https://doi.org/10.1111/ejss.13203
- Kodirov, O., Kersten, M., Shukurov, N., & Martín Peinado, F. J. (2018). Trace metal(loid) mobility in waste deposits and soils around Chadak mining area, Uzbekistan. Science of the Total Environment, 622-623, 1658–1667. https://doi.org/10.1016/j.scitotenv.2017.10.049
- Kopittke, P. M., Dalal, R. C., Hoeschen, C., Li, C., Menzies, N. W., & Mueller, C. W. (2020). Soil organic matter is stabilized by organo-mineral associations through two key processes: The role of the carbon to nitrogen ratio. Geoderma, 357, 1–9. https://doi.org/10.1016/j.geoderma.2019.113974
- Korfali, S. I., & Karaki, H. (2018). Speciation of metals in soils and water: Risk assessment. Environmental Processes, 5(Suppl 1), 101–125. https://doi.org/10.1007/s40710-018-0328-1
- Kumar, S., Prasad, S., Yadav, K. K., Shrivastava, M., Gupta, N., Nagar, S., Bach, Q.-V., Kamyab, H., Khan, S. A., Yadav, S., & Malav, L. C. (2019). Hazardous heavy metals contamination of vegetables and food chain: Role of sustainable remediation approaches - a review. Environmental Research, 179, 108792. https://doi.org/10.1016/j.envres.2019.108792
- Kwiatkowska-Malina, J. (2018). Functions of organic matter in polluted soils: The effect of organic amendments on phytoavailability of heavy metals. Applied Soil Ecology, 123, 542–545. https://doi.org/10.1016/j.apsoil.2017.06.021
- Latifah, O., Ahmed, O. H., & Majid, N. M. A. (2017). Soil pH buffering capacity and nitrogen availability following compost application in a Tropical Acid soil. Compost Science & Utilization, 26(1), 1–15. https://doi.org/10.1080/1065657X.2017.1329039
- LCA. (2008). Practical guide: Understanding and using agri-environmental analyses. 82.
- Li, Q., Gu, F., Zhou, Y., Xu, T., Wang, L., Zuo, Q., Xio, L., Liu, J., & Tian, Y. (2021). Changes in the Impacts of Topographic Factors, Soil Texture, and cropping systems on Topsoil Chemical Properties in the mountainous areas of the of the subtropical monsoon region from 2007 to 2017: A case study in Hefeng, China. International Journal of Environmental Research and Public Health, 18(2), 1–22. https://doi.org/10.3390/ijerph18020832
- Li, Y., Zhang, H., Tu, C., Song, F., & Luo, Y. (2017). Occurrence of red clay horizon in soil profiles of the Yellow River Delta: Implications for accumulation of heavy metals. Journal of Geochemical Exploration, 176, 120–127. https://doi.org/10.1016/j.gexplo.2015.11.006
- Liang, C.-P., Chen, J.-S., Chien, Y.-C., & Chen, C.-F. (2018). Spatial analysis of the risk to human health from exposure to arsenic contaminated groundwater: A kriging approach. Science of the Total Environment, 627, 1048–1057. https://doi.org/10.1016/j.scitotenv.2018.01.294
- Lwin, C. S., Seo, B.-H., Kim, H.-U., Owens, G., & Kim, K.-R. (2018). Application of soil amendments to contaminated soils for heavy metal immobilization and improved soil quality—a critical review. Soil Science and Plant Nutrition, 64(2), 156–167. https://doi.org/10.1080/00380768.2018.1440938
- Marrugo-Negrete, J., Pinedo-Hernández, J., & Díez, S. (2017). Assessment of heavy metal pollution, spatial distribution and origin in agricultural soils along the Sinú River Basin, Colombia. Environmental Research, 154, 380–388. https://doi.org/10.1016/j.envres.2017.01.021
- Matheron, G. (1963). Principles of geostatistics. Economic Geology, 58(8), 1246–1266. https://doi.org/10.2113/gsecongeo.58.8.1246
- Maurya, S., Abraham, J. S., Somasundaram, S., Toteja, R., Gupta, R., & Makhija, S. (2020). Indicators for assessment of soil quality: A mini-review. Environmental Monitoring and Assessment, 192(9), 1–22. https://doi.org/10.1007/s10661-020-08556-z
- Moniod, F., Pouyaud, B., & Séchet, P. (1977). The Volta River basin. In Monographie Hydrologique 5. ORSTOM.
- Moral, F. J., & Rebollo, F. J. (2017). Characterization of soil fertility using the Rasch model. Soil Science and Plant Nutrition, 17, 486–498. https://doi.org/10.4067/S0718-95162017005000035
- Mousavi, S. R., Sarmadian, F., Dehghani, S., Sadikhani, M. R., & Taati, A. (2017). Evaluating inverse distance weighting and kriging methods in estimation of some physical and chemical properties of soil in Qazvin Plain. Eurasian Journal of Soil Science (EJSS), 6(4), 327–336. https://doi.org/10.18393/ejss.311210
- Nadarajan, S., & Sukumaran, S. (2021). Chemistry and toxicology behind chemical fertilizers. Controlled Release Fertilizers for Sustainable Agriculture, 195–229. https://doi.org/10.1016/b978-0-12-819555-0.00012-1
- Nas, B., & Berktay, A. (2010). Groundwater quality mapping in urban groundwater using GIS. Environmental Monitoring and Assessment, 160(1–4), 215–227. https://doi.org/10.1007/s10661-008-0689-4
- Nelson, D. W., & Sommers, L. E. (1982). Total carbon, organic carbon and organic matter. In A. L. Page (Ed.), Methods of soil analysis, part 2: Chemical and microbiology properties. Agronomy monograph, no. 9 (pp. 539–579).
- Nie, X. J., Zhang, J. H., Cheng, J. X., Gao, H., & Guan, Z. M. (2016). Effect of soil redistribution on various organic carbons in a water- and tillage-eroded soil. Soil and Tillage Research, 155, 1–8. https://doi.org/10.1016/j.still.2015.07.003
- Nikroo, L., Kompani-Zare, M., Sepaskhah, A. R., & Shamsi, S. R. F. (2010). Groundwater depth and elevation interpolation by kriging methods in Mohr Basin of Fars province in Iran. Environmental Monitoring and Assessment, 166(1–4), 387–407. https://doi.org/10.1007/s10661-009-1010-x
- Ning, C., Gao, P., Wang, B., Lin, W., Jiang, N., & Cai, K. (2017). Impacts of chemical fertilizer reduction and organic amendments supplementation on soil nutrient, enzyme activity and heavy metal content. Journal of Integrative Agriculture, 16(8), 1819–1831. https://doi.org/10.1016/S2095-3119(16)61476-4
- Obalum, S. E., Chibuike, G. U., Peth, S., & Ouyang, Y. (2017). Soil organic matter as sole indicator of soil degradation. Environmental Monitoring and Assessment, 189(4), 1–19. https://doi.org/10.1007/s10661-017-5881-y
- Oldfield, E. E., Wood, S. A., & Bradford, M. A. (2018). Direct effects of soil organic matter on productivity mirror those observed with organic amendments. Plant and Soil, 423(1–2), 363–373. https://doi.org/10.1007/s11104-017-3513-5
- Ouyang, J., Liu, Z., Zhang, L., Wang, Y., & Zhou, L. (2020). Analysis of influencing factors of heavy metals pollution in farmland-rice system around a uranium tailings dam. Process Safety and Environmental Protection, 139, 124–132. https://doi.org/10.1016/j.psep.2020.04.003
- Penn, C. J., & Camberato, J. J. (2019). A Critical Review on Soil Chemical Processes that control how Soil pH affects phosphorus availability to plants. Agriculture, 9(6), 1–18. 120. https://doi.org/10.3390/agriculture9060120
- Phefadu, K. C., & Kutu, F. R. (2016). Evaluation of spatial variability of soil physico-chemical characteristics on Rhodic Ferralsol at the Syferkuil Experimental Farm of University of Limpopo, South Africa. The Journal of Agricultural Science, 8(10), 92–106. https://doi.org/10.5539/jas.v8n10p92
- Pikuła, D., & Stępień, W. (2021). Effect of the degree of soil contamination with heavy metals on their mobility in the soil profile in a microplot experiment. Agronomy, 11(5), 1–11. https://doi.org/10.3390/agronomy11050878
- Pincus, L. N., Ryan, P. C., Huertas, F. J., & Alvarado, G. E. (2017). The influence of soil age and regional climate on clay mineralogy and cation exchange capacity of moist tropical soils: A case study from late quaternary chronosequences in Costa Rica. Geoderma, 308, 130–148. https://doi.org/10.1016/j.geoderma.2017.08.033
- Pirestani, N., Ahmadi Nadoushan, M., Abolhasani, M. H., & Ahamadmahmoudi, R. Z. (2024). Mapping soil characteristics: Spatio-temporal comparison of land use regression and ordinary kriging in an Arid Environment. Journal of the Indian Society of Remote Sensing, 52(1), 79–93. https://doi.org/10.1007/s12524-023-01804-y
- Puga, A. P., Melo, L. C. A., de Abreu, C. A., Coscione, A. R., & Paz-Ferreiro, J. (2016). Leaching and fractionation of heavy metals in mining soils amended with biochar. Soil and Tillage Research, 164, 25–33. https://doi.org/10.1016/j.still.2016.01.008
- Rahal, N. S., & Alhumairi, B. A. J. (2019). Modelling of soil cation exchange capacity for some soils of east gharaf lands from mid-Mesopotamian plain (wasit province/Iraq). International Journal of Environmental Science and Technology, 16(7), 3183–3192. https://doi.org/10.1007/s13762-018-1913-6
- Ramos, F. T., de Carvalho Dores, E. F. G., dos Santos Weber, O. L., Beber, D. C., Campelo, J. H., & de Souza Maia, J. C. (2018). Soil organic matter doubles the cation exchange capacity of tropical soil under no-till farming in Brazil. Journal of the Science of Food and Agriculture, 98(9), 3595–3602. https://doi.org/10.1002/jsfa.8881
- Rangel-Peraza, J. G., Padilla-Gasca, E., López-Corrales, R., Rochín Medina, J., Bustos-Terrones, Y., Amabilis-Sosa, L. E., Rodríguez-Mata, A. E., & Osuna-Enciso, T. (2017). Robust soil quality index for tropical soils influenced by agricultural activities. Journal of Agricultural Chemistry and Environment, 6(4), 199–221. https://doi.org/10.4236/jacen.2017.64014
- Richards, L. C. (1954). Diagnosis and improvement of saline and saline and alkali soils (agricultural handbook No (60)). US Department of Agriculture, US.
- Rosemary, F., Vitharana, U. W. A., Indraratne, S. P., Weerasooriya, R., & Mishra, U. (2017). Exploring the spatial variability of soil properties in an Alfisol soil catena. Catena, 150, 53–61. https://doi.org/10.1016/j.catena.2016.10.017
- Russell, A. E., Hall, S. J., & Raich, J. W. (2017). Tropical tree species traits drive soil cation dynamics via effects on pH: A proposed conceptual framework. Ecological Monographs, 87(4), 685–701. https://doi.org/10.1002/ecm.1274
- Sako, A., Nimi, M., & Rein, A. (2018). Environmental geochemistry and ecological risk assessment of potentially harmful elements in tropical semi-arid soils around the Bagassi South artisanal gold mining site, Burkina Faso. Cogent of Environmental Sciences, 4(1), 1543–1565. https://doi.org/10.1080/23311843.2018.1543565
- Sarkar, S., Sarkar, B., Basak, B. B., Mandal, S., Biswas, B., & Srivastava, P. (2017). Soil mineralogical perspective on Immobilization/Mobilization of heavy metals. In A. Rakshit, P. Abhilash, H. Singh, & S. Ghosh (Eds.), Adaptive soil management : From theory to practices. Springer. https://doi.org/10.1007/978-981-10-3638-5_4
- Siedt, M., Schäffer, A., Smith, K. E. C., Nabel, M., Roß-Nickoll, M., & van Dongen, J. T. (2020). Comparing straw, compost, and biochar regarding their suitability as agricultural soil amendments to affect soil structure, nutrient leaching, microbial communities, and the fate of pesticides. Science of the Total Environment, 751, 1–19. https://doi.org/10.1016/j.scitotenv.2020.141607
- Silva, C. O., Manzione, R. L., & Oliveira, S. R. M. (2023). Exploring 20-year applications of geostatistics in precision agriculture in Brazil: What’s next? Precision Agriculture, 24(6), 2293–2326. https://doi.org/10.1007/s11119-023-10041-9
- Singh, M., Sarkar, B., Biswas, B., Churchman, J., & Bolan, N. S. (2016). Adsorption-desorption behavior of dissolved organic carbon by soil clay fractions of varying mineralogy. Geoderma, 280, 47–56. https://doi.org/10.1016/j.geoderma.2016.06.005
- Sintorini, M. M., Widyatmoko, H., Sinaga, E., & Aliyah, N. (2021). Effect of pH on metal mobility in the soil. Earth and Environmental Science, 737(1), 012071–6. https://doi.org/10.1088/1755-1315/737/1/012071
- Song, W., Jia, H., Li, Z., & Tang, D. (2018). Using geographical semi-variogram method to quantify the difference between NO2 and PM2.5 spatial distribution characteristics in urban areas. Science of the Total Environment, 631–632, 688–694. https://doi.org/10.1016/j.scitotenv.2018.03.040
- Souza, E. S. D., Fernandes, A. R., De Souza Braz, A. M., Oliveira, F. J. D., Alleoni, L. R. F., & Campos, M. C. C. (2018). Physical, chemical, and mineralogical attributes of a representative group of soils from the eastern Amazon region in Brazil. SOIL, 4(3), 195–212. https://doi.org/10.5194/soil-4-195-2018
- Sungur, A., Soylak, M., & Özcan, H. (2016). Chemical fractionation, mobility and environmental impacts of heavy metals in greenhouse soils from Çanakkale, Turkey. Environmental Earth Sciences, 75(4), 1–11. https://doi.org/10.1007/s12665-016-5268-3
- Suzuki, T., Nakase, K., Tamenishi, T., & Niinae, M. (2020). Influence of pH and cations contained in rainwater on leaching of Cd(II) from artificially contaminated montmorillonite. Journal of Environmental Chemical Engineering, 8(5), 1–8. https://doi.org/10.1016/j.jece.2020.104080
- Tankari Dan-Badjo, A., Ibrahim, O. Z., Guéro, Y., Morel, J. L., Feidt, C., & Echevarria, G. (2019). Impacts of artisanal gold mining on soil, water and plant contamination by trace elements at Komabangou, Western Niger. Journal of Geochemical Exploration, 205, 1–10. https://doi.org/10.1016/j.gexplo.2019.06.010
- Torrance, K. W., Redwood, S. D., & Cecchi, A. (2021). The impact of artisanal gold mining, ore processing and mineralization on water quality in Marmato, Colombia. Environmental Geochemistry and Health, 43(10), 4265–4282. https://doi.org/10.1007/s10653-021-00898-y
- Uddin, M. K. (2017). A review on the adsorption of heavy metals by clay minerals, with special focus on the past decade. Chemical Engineering Journal, 308, 438–462. https://doi.org/10.1016/j.cej.2016.09.029
- Verbeeck, M., Thiry, Y., & Smolders, E. (2019). Antimonate sorption in soils increases with ageing. European Journal of Soil Science, 71(1), 55–59. https://doi.org/10.1111/ejss.12845
- Wang, T., Kang, F., Cheng, X., Han, H., & Ji, W. (2016). Soil organic carbon and total nitrogen stocks under different land uses in a hilly ecological restoration area of North China. Soil and Tillage Research, 163, 176–184. https://doi.org/10.1016/j.still.2016.05.015
- Wang, Y., Ran, L., Fang, N., & Shi, Z. (2018). Aggregate stability and associated organic carbon and nitrogen as affected by soil erosion and vegetation rehabilitation on the Loess Plateau. Catena, 167, 257–265. https://doi.org/10.1016/j.catena.2018.05.005
- Webster, R., & Oliver, M. A. (2007). Geostatistics for environmental scientists. Wiley.
- Wei, B., Yu, J., Cao, Z., Meng, M., Yang, L., & Chen, Q. (2020). The availability and accumulation of heavy metals in greenhouse soils associated with intensive fertilizer application. International Journal of Environmental Research and Public Health, 17(15), 1–13. https://doi.org/10.3390/ijerph17155359
- Wilding, L. P., & Drees, L. R. (1978). Spatial variability; a pedologist’s viewpoint. In diversity of soils in the tropics. Soil Science Society of America Journal, 34, 1–12.
- Xu, D. M., Fu, R. B., Wang, J. X., Shi, Y. X., & Guo, X. P. (2021). Chemical stabilization remediation for heavy metals in contaminated soils on the latest decade: Available stabilizing materials and associated evaluation methods–A critical review. Journal of Cleaner Production, 321, 1–22. https://doi.org/10.1016/j.jclepro.2021.128730
- Xu, Z., Zhang, T., Wang, S., & Wang, Z. (2020). Soil pH and C/N ratio determines spatial variations in soil microbial communities and enzymatic activities of the agricultural ecosystems in Northeast China: Jilin province case. Applied Soil Ecology, 155, 1–12. https://doi.org/10.1016/j.apsoil.2020.103629
- Zhang, Z., Wu, X., Wu, Q., Huang, X., Zhang, J., & Fang, H. (2020). Speciation and accumulation pattern of heavy metals from soil to rice at different growth stages in farmland of southwestern China. Environmental Science and Pollution Research, 27(28), 35675–35691. https://doi.org/10.1007/s11356-020-09711-2
- Zhao, F. Z., Ren, C. J., Han, X. H., Yang, G. H., Wang, J., & Doughty, R. (2018). Changes of soil microbial and enzyme activities are linked to soil C, N and P stoichiometry in afforested ecosystems. Forest Ecology and Management, 427, 289–295. https://doi.org/10.1016/j.foreco.2018.06.011
- Zhu, X., Beiyuan, J., Lau, A. Y. T., Chen, S. S., Tsang, D. C. W., Graham, N. J. D., Lin, D., Sun, J., Pan, Y., Yang, X., & Li, X.-D. (2018). Sorption, mobility, and bioavailability of PBDEs in the agricultural soils: Roles of co-existing metals, dissolved organic matter, and fertilizers. Science of the Total Environment, 619-620, 1153–1162. https://doi.org/10.1016/j.scitotenv.2017.11.159