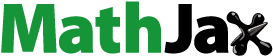
Abstract
Consistent estimation techniques need to be implemented to obtain robust empirical outcomes which help policymakers formulating public policies. Therefore, Mitscherlich Baule function was implemented using robust regression model based on a growth equation. In Mitscherlich Baule Function two methods were used one least square Method and Iteratively Reweighted Least Square Method. In Least Square method Gauss Newton Method, was used for the study, whereas in Iteratively Reweighted Least Square method Marquardt Method and Gradient Method was used. Secondary data has been used in the study. Classical and robust procedures were employed for the estimation of the parameters. Thus empirical results reveal that the overall fit of the model improves in case of Marquardt technique. Thus, empirical findings exhibit that the results, obtained through Marquardt method are better than LS techniques. The present study focus on when you are dealing with nonlinear production function Mitscherlich Baule function comes out to be best for handling outliers in data sets and also helpful for policy makers for formulating public policies.
1 Introduction
The Mitscherlich–Baule is an exponential function that allows for convex and plateau growth. This function is considered useful due to its flexibility regarding the degree of isoquant convexity and accommodating almost perfect factor substitution issues to zero factor substitution (Harrell et al. Citation1984). The Mitscherlich–Baule function is given by:
Where representing the growth, and c and e the natural input endowments that include x1 and
. The coefficients c and e describes the influence of the corresponding input factors on the study variable. Mitscherlich-Baule production function allows for a growth plateau, which follows from the Von Liebig approach to production functions. Moreover, this functional form is characterized by continuously positive marginal productivities of the input factors (Finger and Hediger Citation2008). It does not exhibit negative marginal productivities. It allows for a broad range of model evaluation in isoquant patterns and plateau vs. yield decline. Additionally, the function has sufficient flexibility to accommodate limited factor substitution and set plateau growth (Khan et al. Citation2021b). The classical Mitscherlich Baule function is based on Liebig’s Law of the Minimum and describes the response of a crop to an increase in the factor that is limiting growth, other factors being constant (Von Liebig Citation1855). As stated by Zaman et al. (Citation2001) “A review of the literature shows that robust regression techniques are not frequently employed.” As a result, the main goal of this study is to use robust regression approaches to analyze data. The primary purpose of this article is to provide other approaches that are robust and less susceptible toward outliers and include the weaknesses of the conventional estimation methods in order to capitalize on the drawbacks of the conventional estimation methods in the presence of outlying observations in the data that lead to ill-estimation. The Marquardt curve-fitting method is actually a combination of the two other minimization methods: the gradient descent method and the Gauss–Newton method. In the gradient descent method, the sum of the squared errors is reduced by updating the parameters in the steepest-descent direction. In the Gauss–Newton method, the sum of the squared errors is reduced by assuming the least squares function is locally quadratic, and finding the minimum of the quadratic (Khan et al. Citation2021a). The Levenberg-Marquardt method acts more like a gradient-descent method when the parameters are far from their optimal value, and acts more like the Gauss–Newton method when the parameters are close to their optimal value. The Marquardt method may be a suitable choice. On the other hand, if your production function is more flexible or doesn’t follow a known functional form, and you want to minimize the objective function without constraints, gradient-based methods offer greater flexibility and can be applied to a broader range of production functions. The choice depends on the specific characteristics of your production function and your optimization goals (Khan et al. Citation2021b).
2 Empirical methodology and data
This section includes the description of the data collected, the variables under consideration, the appropriate models used in this study, and statistical analysis for its estimation that have been carried out to meet the desired objectives.
2.1 Sources of data
As mentioned earlier, we have used the secondary data in this paper. It is the survey done by Division of Agricultural Economics and ABM during the year 2018 to study the yield of maize w.r.t to the labor cost and capital involves. The multi stage sampling procedure was adopted for the selection of unit block as the first, villages as second and farmers as third stage. The Chenani block of Udhampur district of Jammu was selected purposively.
2.2 Regression analysis
Regression is the most common and useful statistical tool which can be used to quantify the relationship between a response variable (y) and explanatory variables (x). If we have dependent variable Yand independent variables X1, X2,…Xn then the regression analysis is to estimate population regression function.The model generally can be expressed as:
Where regression parameters and error
is normally distributed with mean zero and equal variance discussed as (Montgomery et al. Citation2006). Problem that arises in regression analysis is to determine the best estimates for
. If assumptions are violated.
Simple linear regression allows the mean function E(y) to depend on more than one explanatory variable and to have shapes other than straight lines although does not allow for arbitrary shapes. To this end, the seminal works of Legendre (Citation1806) and Gauss (Citation1809) proposed the method of ordinary least squares (OLS) which has arguably become the most popular approach to conducting a regression analysis. This popularity is likely attributable to the fact that the OLS method can be expressed in closed form and achieve minimum variance among all unbiased estimators when the underlying error distribution follows normality. It has been observed that OLS may not be appropriate when the response variable differs from the regression function in an asymmetric manner which is commonly encountered usually in medical data, agricultural economic data and among other situations. Regression analysis is used to predict a continuous dependent variable from a number of independent variables. The independent variables used in regression can be either continuous or dichotomous. If the dependent variable is dichotomous, then logistic regression is advisable. Independent variables with more than two levels can also be used in regression analyses but they first must be converted into variables that have only two levels. This is called dummy coding. Usually, regression analysis is used with naturally occurring variables, as opposed to experimentally manipulated variables.
2.2.1 Outliers management
Outliers can be generated by from a simple operational mistake to including a small sample from a different population they result in serious effects on statistical inference. Even one outlying observation can destroy least squares estimation resulting in parameter estimates that do not provide useful information for the majority of the data. Robust regression analyses have been developed as an improvement to least squares estimation in the presence of outliers and to provide us information about what a valid observation is and whether this should be thrown out. The primary purpose of robust regression analysis is to fit a model which represents the information in the majority of the data. The properties of efficiency, breakdown point and bounded influence are used to define the measure of robust technique performance in a theoretical sense (Rousseeuw and Leroy Citation1987). Efficiency can tell us how well a robust technique performs relative to least squares on clean data (without outliers). Linear regression models are widely used in many fields of science such as engineering, economics, sociology, health, etc. Due to the simplicity of the idea behind the OLS method, the minimization of the sum of squared residuals, and the interpretability of the final model parameter estimates, the OLS method is very popular among practitioners. However it is also well known that outliers considered here are heterogeneous observations in comparison with the majority of the data and might strongly affect these estimators. Not all outliers are influential and not all influential points will hurt our modeling, but we still need robust methods to prevent possible influential outliers and bad leverage points from leading us to the wrong choice of model. Statisticians who neglect to consider robust methods are ignoring the fact that the methods they are using can perform very poorly when their data does not strictly satisfy the assumptions of their chosen method as per Weisberg (Citation2005) and Ronchetti et al. (Citation1997). There are several types of outliers in regression problems which include residual outliers, vertical outliers, and high leverage points. The term “residual outlier” refers to any observation with a big residual. Vertical outliers (VO), also known as y-outliers, are observations that are extreme or outlying in y-axis. High leverage points (HLPs) on the other hand are those observations which are extreme or outlying in X-Coordinate. HLP can be classified into good leverage points (GLP) and bad leverage points (BLP). GLP are outliers in the explanatory variables that follow the majority of the data’s pattern, whereas BLP are the absolute opposite. The computed values of various estimates are more affected by BLP.
2.2.2 Robust regression
Robust regression is a method of regression analysis considered to avoid some restrictions of traditional parametric and nonparametric methods. Certain widely used method of regression is ordinary least squares and has properties if their fundamental premises are true but can yield misleading results in case premises are not reliable. Robust regression methods are designed to be not overly affected by violations of assumptions.
Robust regression analysis provides an alternative to the least square regression model when fundamental assumptions are unfulfilled by the nature of the data (Marona et al. Citation2006). The analyst estimates his statistical regression models and tests his assumptions and frequently finds that the assumptions are substantially violated. Sometimes, the analyst can transform his variables to conform to those assumptions. However, a transformation will not eliminate the leverage of influential outliers that bias the prediction and distort the significance of parameter estimates. Under these circumstances, robust regression is resistant to the influence of the outliers and may be the only reasonable recourse (Yousuf et al. Citation2021b). Robust regression provides estimates of the relationships between variables that are more accurate and reliable. Robust Regression is important for policymakers to understand that the outcomes are less susceptible to extreme data points, so offering a more solid foundation for making decisions. Data of varying quality are frequently dealt by policymakers. Heteroscedasticity, non-constant variance, and other problems can be handled with robust regression. If the analysis relies on such data, policymakers should recognize that robust regression can provide more trustworthy results. Policymakers should be aware that robust regression can yield more reliable conclusions if the analysis depends on such data.
3 Empirical results and discussion
To apply the robust regression techniques on the secondary data least square Method and Iteratively Reweighted Least Square Method are being used. The Mitscherlich-Baule production functions estimates of real data are presented in . In Gauss Newton method coefficient estimate of c (0.01) and d (4.04) are nonsignificant while as the coefficient estimate of a (142.20), b (1.01) and e (44.00) are significant, R2 value in case of Gauss Newton method is 0.75 while as values of AIC and BIC are 381.39 and 389.10 units. In Marquardt method, the coefficient estimate of b (0.02) and d (0.04) are nonsignificant while as a (150.40), c (12.6) and e (7.64) is significant. The R2 value in this method is 0.94 which indicates that 94% variation of study variable is explained through this method and AIC and BIC have values are 362.96 and 370.67 unit respectively. In Gradient method the coefficient estimate of c (0.01) and d (0.04) are not significant while as a (99.99), b (0.05) and e (4.00) are significant. The coefficients and d describes the influence of the corresponding input factors on the study variable. The R2 value in this method is 0.77 with AIC and BIC are 374.6 and 382.31unit, respectively. The primary application of Marquardt algorithm is best among the others which is on the basis of IRLS.
Table 1 Estimates of Mitscherlich-Baule production function through robust methods for maize yield data w.r.t labor and capital and comparison with OLS thereof.
The marginal value product (MVP) is a measure of the additional profits raised during increasing the quantity of an input used by one unit while maintaining all other input quantities constant. The shows the MVP and elasticity of Mitscherlich-Baule production function of data. The MVP was calculated by using regression coefficients of each input of both the functions and geometric mean value of input values. In case of Mitscherlich-Baule production function c and e are the input variables that include x1 and . For the data the MVP for x1 and x2 were found to be 0.01 and 0.02. The elasticity for x1 (–5.58) was less than zero, signaling a negative decreasing function for the factors, indicating over-utilization of the input and implying that its allocation and use were in an irrational stage of the manufacturing process (stage III). The elasticity values for x2 (6.71) are greater than one, showing that production responds to increases in consumption significantly. The
and x2 describes the influence of the corresponding input factors on the study variable.
is labor and x2 is capital.These variables are explanatory variables.
Table 2 Estimated MVP and elasticity of Mitscherlich Baule production function.
represents the diagnostic panel in which we have Q–Q plot of these residuals, the observations are above the diagonal line at both ends of the pattern. That means that the distribution of residuals is positively skewed. This is confirmed by looking at the histogram of residuals. For this model, the residuals are normal. The graphs indicate whether the residuals for the model are normally distributed. Normally distributed residuals are one of the assumptions of regression that are used to derive inferential statistics (Yousuf et al. Citation2021c). The third plot is a normal quantile-quantile plot (Q–Q plot) of the residuals. The residuals are approximately normal; the observations are close to the diagonal line.
The partial results from this NLIN run are shown in ; the diagnostics plots corroborate the previously mentioned expectations: highly correlated raw residuals (with the predicted values), significant differences between tangential and Jacobian leverages and projected residuals which overcome some of the shortcomings of the raw residuals (Yousuf et al. Citation2021a).
The performance of production functions in data sets using cross validation techniques is shown in . Mitscherlich Baule function has RMSE value 6.32. The AIC, AICC, PRESS, SBC, and CVPRESS values for both the production functions are as 458.73 458.99, 3924.92, 364.88, and 4001.1, respectively. The lowest PRESS value indicates model has highest predictive power. The training error is not more than the validation error thus both models are operating normally.
Table 3 Performance criteria of Mitscherlich-Baule production function using cross-validation technique.
provides k-fold cross validation performance criteria for Mitscherlich-Baule production function. With a large number of folds (K), the real error rate is minimal, but the variation is substantial. The computational time may be very long depending on the complexity of the models under consideration. We replicated samples ten times in K-fold validation. For Mitscherlich-Baule production function, the K values used are 5 and 10, i.e. K 5 and K
10. The R2 value in the case of 10 folded cross-validation techniques is greater than the R2 value in the case of 5 folded cross-validation technique. Coefficient of variation in case of 5 fold was found be larger than 10 fold cross-validation.
Table 4 Performance criteria of Mitscherlich-Baule production function using k-fold cross validation technique.
4 Summary and conclusion
The MLE technique is used to calculate the parameters of the Mitscherlich Baule function regimes. If data or manufacturing processes follow von Liebig’s law of minimum, they have enough flexibility to support restricted factor substitution: it states that growth is determined not by the total number of resources available, but by the scarcest resource (limiting factor). The least square approach used the Gauss Newton Method, whereas the Iteratively Reweighted Least Square method used the Marquardt Method and Gradient Method. Any machine learning system will benefit from the Gauss-Newton approach, which is a simple optimization procedure (Wahid et al. Citation2017). There are interpolated between the gauss Newton and gradient methods. In the least quadruple curve fitting problem the Marquardt algorithm is most usually utilized (Hawkins and Khan Citation2009). The technique of gradients is an iterative way for resolving nonlinear minor square issues. The Marquardt approach in is better than other methods. The Marquardt method’s R2 value (0.94) is much higher than the Gauss Newton and Gradient’s R2 values. The AIC (362.96) and BIC (370.67) are also low in the case of the Marquardt Method. The primary application of Marquardt algorithm is best among the others which is on the basis of IRLS.
Disclosure statement
Authors declare that there is no conflict of interest.
References
- Finger R, Hediger W. 2008. An application of robust regression to a production function comparison. Open Agric J. 2:90–98.
- Gauss CF. 1809. Theoria Motus Corporum Coelestium. Hamburg: Perthes. Theory of the motions of the heavenly bodies moving about the Sun in Conic sections. New York: Dover. p. 1963.
- Harrell FE, Lee KL, Califf RM, Pryor DB, Rosati RA. 1984. Regression modelling strategies for improved prognostic prediction. Stat Med. 3:143–152.
- Hawkins DM, Khan DM. 2009. A procedure for robust fitting in nonlinear regression. Comput Stat Data Anal. 53:4500–4507.
- Khan DM, Ali M, Ahmad Z, Manzoor S, Hussain S. 2021a. A new efficient redescending M-estimator for robust fitting of linear regression models in the presence of outliers. Math Prob Eng. 2021:1–11.
- Khan DM, Yaqoob A, Zubair S, Khan MA, Ahmad Z, Alamri OA. 2021b. Applications of robust regression techniques: an econometric approach. Math Prob Eng. 2021:1–9.
- Legendre AM. 1806. Nouvelles méthodes pour la détermination des orbites des comètes; par AM Legendre… chez Firmin Didot, libraire pour lew mathematiques, la marine, l‘architecture, et les editions stereotypes, rue de Thionville.
- Marona RA, Martin DR, Yohai VJ. 2006. Robust statistics theory and methods. Chichester (UK): Wiley.
- Montgomery DC, Peck EA, Vining GG. 2006. Introduction to linear regression analysis. Hoboken (NJ): Wiley.
- Ronchetti E, Field C, Blanchard W. 1997. Robust linear model selection by cross-validation. J Am Stat Assoc. 92:1017–1023.
- Rousseeuw PJ, Leroy AM. 1987. Robust regression and outlier detection. New York (NY): Wiley.
- Von Liebig J. 1855. The basics of agricultural chemistry with reference to the experiments conducted in England (In German). Braunschweig, Germany: Vieweg und Sohn.
- Wahid A, Khan DM, Hussain I. 2017. Robust Adaptive Lasso method for parameter’s estimation and variable selection in high-dimensional sparse models. PLoS One. 12:e0183518.
- Weisberg S. 2005. Applied linear regression. Vol. 528. Hoboken (NJ): Wiley.
- Yousuf R, Sharma M, Bhat A. 2021a. Robust models and their validation for maize production in Jammu region of Jammu and Kashmir union territory. Int J Agric Stat Sci. 17:2065–2072.
- Yousuf R, Sharma M, Bhat MIJ, Rizvi SEH. 2021b. Robust model for the quadratic production function in presence of high leverage points. Int J Sci Res Math Stat Sci. 8:8–13.
- Yousuf R, Sharma M, Iqbal M, Bhat J, Gupta M. 2021c. Estimation and Validation of Robust Model for Productivity of Apple in UT of J&K using Cobb Douglas Method. Int J Stat Reliab Eng. 8:376–380.
- Zaman A, Rousseeuw PJ, Orhan M. 2001. Econometric applications of high-breakdown robust regression techniques. Econ Lett. 71:1–8.