Abstract
Among the wide range of proteomic technologies, targeted mass spectrometry (MS) has shown great potential for biomarker studies. To extend the degree of multiplexing achieved by selected reaction monitoring (SRM), we recently developed SWATH MS. SWATH MS is a variant of the emerging class of data-independent acquisition (DIA) methods and essentially converts the molecules in a physical sample into perpetually re-usable digital maps. The thus generated SWATH maps are then mined using a targeted data extraction strategy, allowing us to profile disease-related proteomes at a high degree of reproducibility. The successful application of both SRM and SWATH MS requires the a priori generation of reference spectral maps that provide coordinates for quantification. Herein, we demonstrate that the application of the mass spectrometric reference maps and the acquisition of personalized SWATH maps hold a particular promise for accelerating the current process of biomarker discovery.
For many diseases, early and accurate diagnosis are beneficial for the selection of optimized, specific and timely management decisions Citation[1]. The diagnostic process requires certain measurables indicating a specific biological or clinical state, the so-called biomarkers. Particularly, important biomarkers are those that can indicate the risk for a particular condition, the presence or the stage of disease, or the response of a patient to therapy Citation[2]. For example, cancer biomarkers can be used to detect early-stage carcinogenesis, predict tumor progression, drug response and clinical outcomes of therapies Citation[3].
The clinical needs of personalized medicine require multiple biomarkers for tailoring medical decisions and practices to the individual patients. Despite the standardized tumor, node, metastasis system Citation[4] as well as the recent progress in medical imaging technology, molecular biomarkers provide additional personalized information and are thus promising for the customization of cancer prediction and monitoring Citation[5]. With the advances in high-throughput technologies, such as genomics, transcriptomics and proteomics, biomarker candidate lists containing hundreds of analytes are being generated at increasing throughput. Furthermore, computational network inference and extension approaches have shown great potential to integrate results from diverse large-scale studies for predicting new biomarker candidates in silico. Different types of biomarkers are being tested including RNA, DNA, metabolites and proteins. Among these, proteins are more dynamic, diverse and more directly reflective of cellular physiology than nucleic acid-based markers, offering high potential to serve as biomarkers for routine application Citation[6].
Proteomics is the field of research that is concerned with the analysis of proteins usually at a large scale. Current proteomic techniques and strategies can be broadly grouped into discovery and targeting methods Citation[7]. Targeted proteomics Citation[8–10] exemplified by selected reaction monitoring (SRM, also referred as multiple reaction monitoring) attempts to reproducibly and accurately quantify sets of predetermined proteins across multiple samples. The technique has been used for the fast evaluation of potential protein biomarker candidates (typically <100 proteins per analysis) in large patient cohorts to assess their clinical value Citation[11,12]. Another recently developed targeting mass spectrometry (MS) approach is based on a data-independent acquisition (DIA) mass spectrometric method – SWATH MS. In contrast to SRM, in SWATH MS feature sets for all analytes detectable in a biological sample are recorded Citation[13], which are then used to identify and quantify the respective analyte using a targeted data analysis strategy Citation[13,14].
The successful applications of targeted proteomic methods such as SRM and SWATH MS require the one time generation of mass spectrometric reference maps for each component of whole canonical proteomes or specific subsets of proteomes (subproteomes), for example, those of particular clinical interest. Here, we describe the recent advances in the generation of mass spectrometric reference maps and their applications. We also discuss the impact of these advances on the field of biomarker discovery and clinical research.
MS-based proteomics in clinical research
Clinical need in diagnostics: personalized medicine and unbiased measurements
The identification of specific biomarkers is essential for the realization of personalized medicine, in terms of better estimated disease risk, more adapted therapies and improved disease outcome. Quantitative proteome patterns of a person’s tissue or body fluid may reveal information about the health state that is not apparent from, for example, genomic or transcriptomic information. This is partially due to the fact that the translation of genomic patterns into proteomic phenotypes is complex and poorly understood and partially due to the heterogeneity of many diseases, for instance in the case of cancer, where cancer cells harboring a broad spectrum of genetic alternations are contained in a specific tumor. It is therefore not surprising that enormous efforts have been expended to detect new protein biomarkers for many diseases. These efforts have resulted in a large number of publications that provide evidence for biomarkers, yet very few of such proteins have made it into routine clinical practice Citation[15]. The limited success is a result of different reasons including limitations of the current protein quantitative methods (discussed below) and the lack of more active collaborations between proteomic and clinical committees Citation[2,5]. Nevertheless, there is a great need to validate proposed biomarker candidates in well-designed, quality controlled studies, which require suitable and sufficient clinical specimens and highly reproducible and quantitatively accurate analytical techniques Citation[16]. For the validation, the acquisition of personalized proteomic expression maps containing multiple, ideally all detectable biomarker candidates would be highly desirable, because it would allow the nonbiased measurement of all possible biomarkers across sample cohorts, to maximize the predictive power and to select the biomarker or biomarker pattern with the best discriminant power.
Antibody-based methods: strengths & weaknesses
Traditionally, the measurement of protein biomarkers in clinical samples (e.g., Prostate-specific antigen for prostate cancer Citation[17], CA125 for ovarian cancer Citation[18] and hEGF receptor-2 for breast cancer Citation[19]) has primarily relied on antibody-based immunoassays, such as immunohistochemistry (IHC) staining technique or enzyme-linked immunosorbent assay (ELISA). ELISA is generally used to determine protein abundances in bodily fluids, whereas IHC allows for determining the antigen abundances and localizations in cells of fresh tissue sections or immobilized biological biopsies. Antibody-based approaches are routinely employed in the clinics because they provide convenient, rapid, sensitive and high-throughput solutions for the application of biomarkers. High-throughput assays using automated analyzers (such as Beckman Coulter Access and Roche Elecsys Systmes, etc.) are now standard in clinical chemistry laboratory offering high reproducibility (coefficients of variation [CVs] for immunoassays are typically <5%).
However, antibody-based methods are accompanied with several limitations. First, high-quality tests based on automated analyzers are available for only a few well-established biomarkers. Second, ELISA measurements rely on two highly specific (preferably mono-clonal) antibodies and are thus critically dependent on the availability and quality of the antibody, which is the reason for their costly and lengthy developmental process. Third, it is difficult to multiplex ELISA assays for measuring a large number of targets, due to the likely cross-reactivity between antibodies. Fourth, the costs of applying an ELISA kit increase linearly with the number of samples to be tested. And the costs can be prohibitive if >5–10 proteins need to be measured. Also IHC has its own limitations. The fixation and tissue preservation are obligatory sample processing steps in IHC to make proteins/epitopes available for proper antibody staining. These steps can possibly affect the preservation and detection of different proteins. Moreover, IHC usually only offers a semi-quantitative measurement for protein abundances that are scored by expert opinion across multiple tissue sections. In light of the above, alternative protein measurement approaches such as MS-based technologies are being developed.
Evolution of MS-based proteomic methods & their promises for clinical research
The last decade has witnessed significant progress in protein identification and quantification using MS-based methods Citation[20,21]. In particular, new instrument configurations, new quantitative approaches and optimized software tools to analyze MS-derived proteomic data have largely improved the analytical performance of MS-based proteomics, in terms of reproducibility, dynamic range, limit of detection (LOD), comprehensiveness and quality of the results Citation[7,9,22–24].
Shotgun proteomics, also known as discovery proteomics, is a universally and successfully used proteomic method for identifying proteins in complex mixtures Citation[7]. In this method, the enzymatically digested protein sample is analyzed by liquid chromatography tandem MS (LC–MS/MS) operated in data-dependent acquisition (DDA) mode. In this mode, peptide fragmentation in the mass spectrometers is guided by the abundance of peptide ions or so-called precursor ions detected in a survey scan. Detected peptide ions (or a subset of them) are then selected for collision-induced dissociation (CID) and fragment ion (MS2) spectra are recoded . The MS2 spectra along with the mass of the precursor ion are then searched against protein database to infer the peptide sequence and protein identity. Dynamic exclusion filtering in DDA mode excludes the re-sampling of the same precursor ion, thus maximizing the number of different precursors that are selected over time. This optimization, however, comes at the price that precursor ions eluting at similar times from the columns are excluded from the analysis if their precursor mass is within the excluded mass range. Furthermore, the precursor ion selection is largely stochastic following simple intensity-dependent heuristics, which leads to an irreproducible selection of precursor ions and therefore irreproducible protein identification and quantification results across samples Citation[25].
Figure 1. The MS instrumental principles of shotgun proteomics, selected reaction monitoring and SWATH MS analysis. (A) The purpose of shotgun proteomics is the generation of fragment ion spectra for the identification of the amino acid sequence of peptides. The first analyzer (MS1) is set to scan all the precursor ions at a time and then to select one specific precursor (based on the intensity) for fragmentation. The selected ion undergoes collision-induced dissociation in the collision cell, and the resulting fragments are analyzed by the second analyzer (MS2). The process is repeated for different precursors, yielding the MS2 spectra (shown on the far right). (B) In SRM, precursor ions of a specific peptide are selected in MS1. After fragmentation step, a specific fragment ion from the target peptide (transition) is selected in Q3 and guided to the detector. Finally, an SRM trace corresponding to the specific fragment(s) of the peptide monitored over time is generated. (C) SWATH-MS is a data-independent acquisition method in which the MS2 spectra generation is not guided by the real-time intensity of precursor ions. Here, the MS2 data are acquired by repeatedly cycling through 32 consecutive 25 Da precursor isolation windows (swaths) and monitoring all fragment ions. The high resolution of MS2 spectra (10 p.p.m) ensures the specificity of peptide identification.
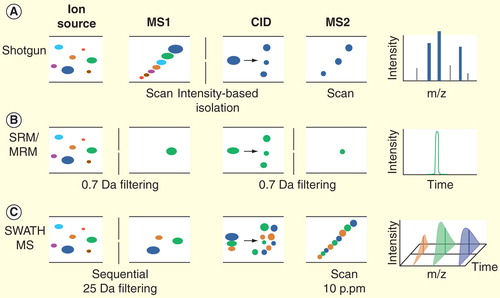
New MS-based analytical strategies with increased selectivity such as accurate mass and time tag strategy Citation[26], directed MS Citation[7,27] (also termed as AIMS Citation[28]), parallel reaction monitoring Citation[29] and SRM Citation[8,30] have emerged in recent years. These methods attempt to increase data reproducibility by eliminating or constraining the stochastic nature of precursor ion selection. Among them, SRM is currently most widely used for clinical proteomics. SRM is usually implemented on triple quadrupole (QQQ) instruments where the first and third quadrupoles of the instrument act as mass filters and selectively monitor a specific precursor ion and one or several fragment ions unique to this precursor ion, whereas the CID fragmentation step is conducted in the second quadrupole. The number of fragment ions of each type that reach the detector is counted over time, resulting in a chromatographic traces indicating intensity profiles of the fragment ion signals (also referred to as SRM transitions) over time that can be used for precise quantification of the peptide Citation[8] . Compared to the discovery approach, SRM is well suited for highly reproducible quantification across many samples and in fact across different laboratories Citation[16]. Therefore, this method is important for biomarker validation and could serve as an alternative to ELISA or IHC for assaying disease biomarkers Citation[7,16]. In fact, SRM is routinely used in the clinics for monitoring small molecules Citation[7].
Whereas a discovery LC–MS/MS run can identify hundreds or thousands of proteins, SRM is currently limited to the targeted measurement of up to 100 proteins per run. To alleviate this limitation, we recently developed a new DIA mass spectrometric technology – SWATH MS Citation[13], which in contrast to SRM is not based on targeted MS acquisition, but on targeted data extraction. The method is implemented in a fast-scanning, high-resolution quadrupole time-of-flight mass spectrometer (TripleTof 5600TM, AB Sciex). SWATH acquisition consists of the sequential selection of sequential precursor ion mass windows (normally 32 windows of 25 amu width covering 400–1200 m/z range), fragmentation of all precursor ions selected in each window and recording of the resulting composite fragment ion spectra. This acquisition mode essentially converts the peptides in a physical sample into a high-resolution digital map consisting of MS2 ions derived from the fragmentation of all precursor ions present in the sample in a predetermined mass to charge window and at a certain time . For the targeted data analysis, several fragment ion chromatograms for each peptide of interest are extracted from the digital maps, whereby mass and chromatography features provide information to identify the peptides. Because of the highly accurate fragment mass (10 parts per million [p.p.m.]) and the predicted retention time, the relative specificity offered by SWATH MS compared with SRM remains qualitatively the same Citation[13].
The need for mass spectrometric reference maps
As reviewed above, mass spectrometric methods have evolved and matured to a level where it is possible to assess the complexity of the human proteome Citation[31], to uncover disease-related subproteomes, and therefore to facilitate biomarker discovery and their validation. The MS-based protein expression data sets, no matter whether they are acquired by DDA or DIA mode, contain a high number of ion traces and signals that can be used for qualitative and quantitative analysis. Therefore, the annotation of MS ion signals to the corresponding peptides or proteins is essential and should be significantly facilitated by mass spectrometric reference maps, in which spectral libraries are meticulously compiled from a large collection of previously observed and identified peptide MS2 spectra Citation[32]. The matching of acquired data sets to such reference maps supports unbiased protein measurements and offers benefits in speed gain and increase in sensitivity and selectivity, compared to sequence database searching using in silico generated fragment ion spectra Citation[32–34]. Whereas the use of proteome-wide spectral reference maps is a convenience for discovery-type proteomic experiments, it is essential prior information for targeted and SWATH MS-type measurements.
Reference maps supporting mass spectrometric navigation of proteomes
Definition of a mass spectrometric reference map
Mass spectrometric reference maps are defined as the collection of fragment ion spectra of peptides corresponding to predicted protein sequences based on the genome Citation[35]. These reference maps constitute highly specific protein assays including all essential coordinates of informative peptides (such as the mass, charge state distribution and chromatographic retention time of the precursor ion as well as the mass, charge state distribution and relative intensities of the fragment ion signals). The assays that are akin to the availability of specific antibodies for a protein supporting immune reagent-based measurements, allow reproducible, reliable, accurate quantification of each component in the complete canonical proteome map or the subproteome of interest.
Generation of mass spectrometric reference maps
The generation of mass spectrometric reference maps covering a proteome or subproteome of interest to near completion is currently attempted by in-depth sequencing of a proteome through large scale, comprehensive LC–MS/MS-based shotgun proteomic experiments Citation[21,35,36]. There are two different ways to generate spectral libraries for reference maps. One is via deep shotgun sequencing of natural proteins in suitable samples and another is based on sequencing libraries of synthetic peptides .
Figure 2. The generation and targeted navigation of mass spectrometric reference maps associated with specific clinical questions. MS2 spectra are obtained either via the deep-sequencing analysis of the real sample or by the shotgun identification the synthetic peptides representing the proteins of interest. The MS2 information are collected as assays, yielding the mass spectrometric reference maps. The targeted navigation can be then achieved by either SRM-based targeted profiling or SWATH MS-based global profiling. Note that the MS assays from the reference maps are important for both the targeted measurement by SRM and the data extraction step in SWATH analysis.
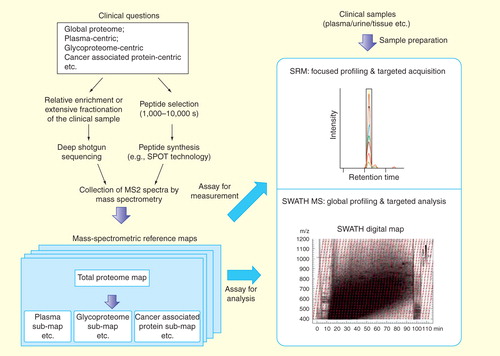
Reference maps based on shotgun sequencing of biological samples
Shotgun proteomic analysis of biological samples identical or similar to those that will be eventually used for targeting measurements will directly generate suitable and relevant spectral libraries to serve as reference maps. Normally, extensive fractionation strategies or relative enrichment methods are used to improve the proteome and peptide coverage . High-quality reference maps are then built by coalescing replicate spectra of the same peptide into a consensus spectrum by using algorithms like SpectraST Citation[32].
Reference maps based on synthetic peptide libraries
To obtain high-quality reference spectra even for those proteins that are difficult to detect in shotgun LC–MS/MS measurements, and more importantly to avoid the identification bias in shotgun sequencing experiments due to biological variations (e.g., patient-to-patient variation), ‘gold standard’ reference spectra have also been generated from fragment ion spectra of chemically synthesized peptide libraries. The use of synthetic peptides eliminates sampling bias of the shotgun measurements and sample preparation steps and generates fragment ion spectra of their bona fide substrates. In this regard, Picotti et al. developed a high-throughput method for SRM assay development that is based on crude synthetic peptide libraries Citation[37] as a reference and an SRM-guided fragment ion spectrum acquisition for each synthetic peptide Citation[38,39]. The technique is dependent on the optimal choice of the components of synthetic peptide libraries . Ideally, such libraries consist of proteotypic peptides (PTPs), defined as experimentally observable tryptic peptides that uniquely identify a specific protein or protein isoform, for every protein of a proteome Citation[30]. Using synthetic peptide libraries, spectral reference maps for 97% of the genome-predicted proteins of the Saccharomyces cerevisiae proteome Citation[35], 93% of the Streptococcus pyogenes proteome Citation[40] and 97% of the Mycobacterium tuberculosis proteome Citation[41] were recently generated.
Repositories for spectral libraries in the reference map
Ideally, the thus generated spectral libraries would be publicly accessible to support any researcher who intends to quantify proteins in samples of his/her interest via targeting MS. Public resources established with this goal in mind include PeptideAtlas Citation[36,42], Human Proteinpedia Citation[43], GPM Proteomics Database Citation[44] and PRIDE Citation[45] etc., which support the retrieval of peptides frequently detected by MS. PeptideAtlas Citation[36,Citation42,101] is a constantly updated compendium of peptides identified in a large number of tandem MS (i.e., discovery-type) proteomics experiments. SRMAtlas Citation[102] is a specific compendium of targeted proteomics assays, resulting from high-quality measurements of natural and synthetic peptides conducted on a QQQ mass spectrometer and is intended as a resource for SRM-based proteomic workflows. Furthermore, to consider the detectability of the SRM assays, PASSEL was created as a combined catalog of best-available transitions selected from PeptideAtlas shotgun data and SRMAtlas, providing the validation information of all assays in the context of a specific sample Citation[46].
Established MS reference maps for clinical research
At present, MS reference maps of different biological or clinical samples have been established or are being developed with respect to specific clinical questions .
Plasma proteomic reference map via deep shotgun sequencing
Human blood plasma is a sample source of particular interest for biomarker discovery. Blood samples can be obtained with a minimally invasive procedure and it presumably contains proteins that hold potential to uncover physiological and pathological changes caused by disease Citation[47]. However, the high complexity and extreme dynamic range of the plasma proteome (12 orders of magnitude) prohibit the sensitive, high-throughput profiling of the plasma proteins in large sample cohorts Citation[48]. A high-quality catalog of plasma proteins detectable by MS is thus an important starting point for the discovery and targeted measurement of blood-based biomarkers. In 2002, the Human Proteome Organization (HUPO) launched the Human Plasma Proteome Project, which generated a total of 889 confidently detected plasma proteins Citation[49]. In 2011, by combining 91 high-confidence shotgun proteomic experiments, Farrah et al. compiled a high-confidence human plasma proteome reference set that includes 1,929 nonredundant protein sequences at an estimated FDR of 1% Citation[50]. Additionally, a spectral counting approach was applied to roughly estimate protein concentrations. This data set represents a useful reference resource for biomarker discovery and SRM measurement in plasma samples.
Global human proteome map via deep shotgun sequencing
The unambiguous identification of any protein or the whole proteome in (clinical) tissues or cells is challenging, but would greatly expand the information recorded for clinical research. Using antibody-based immunoassays to detect target proteins, the Human Protein Atlas Citation[51] has been created to facilitate targeting proteins from more than 15,000 human genes (∼75% of the human protein-coding genes, a current release of 11 March 2013 Citation[52])).
Nowadays, the ability of MS-based proteomics to identify complete sets of proteins expressed by human cells and tissues is rapidly approaching Citation[53]. Two recent papers succeeded in characterizing the expressed human proteomes of human cell lines with substantial depth Citation[54,55]. Both investigated human cancer cell lines (Hela and U2OS) and identified more than 10,000 human proteins, respectively. For real clinical tissue, Wisniewski et al. applied a similar comprehensive shotgun proteomics and quantified over 7,500 proteins extracted from microdissected material of colorectal cancer tissue and determined 1,808 proteins with a significant difference in abundance between colon adenocarcinoma and normal mucosa Citation[56]. Further the HPP of HUPO, through the collective effort of numerous laboratories, has achieved a cumulative coverage of the human proteome of 69% Citation[57], a value that is comparable to that achieved in the PeptideAtlas Citation[58] and GPM database Citation[44] where the data from multiple measurements are integrated. These data indicate that in differentiated human cells approximately half of the human open reading frames are expressed. These large proteomic data sets provide the basis for the development of assays supporting targeting MS for human proteins. At the time of this writing, the generation of mass spectrometric assays for synthetic peptides representing the complete human proteome is now in the finishing stage Citation[39]. In this effort, PTPs for all human proteins were extracted from the above-mentioned data resources or computationally predicted, chemically synthesized and pools of these reagents were used to generate reference spectra on different types of mass spectrometers. Ultimately, these efforts will soon provide a definitive and complete mass spectral reference map for all human proteins as a public resource. In contrast to affinity reagent-based proteomic resources, MS reference maps can be shared electronically, thus avoiding the logistical difficulty of sharing protein-specific affinity reagents.
Synthetic reference map of cancer associated proteins
The definition of a comprehensive list of proteins that are associated with cancer is difficult at present because the molecular mechanisms of cancer biology are far from completely understood. However, a summary list of proteins that have been detected to be differently expressed in human cancers compiled from previous literature citations combined with the ability to reliably and reproducibly quantify them in any sample would be beneficial to fill the gap between basic research and clinical application. Polanski et al. prioritized previous cancer biomarker candidates based on the frequency of literature citations in 2007 Citation[59] resulting in a list of 1,261 candidates. Based on this compiled list and the addition of US FDA-approved protein markers, 1,172 proteins were selected as cancer-associated proteins (CAPs), and SRM assays were developed for 5,426 peptides to represent them. Using these SRM assays in clinically relevant samples, 182 proteins were detected in depleted plasma and 408 CAPs were detected in urine, which has a narrower concentration range than plasma Citation[10]. The expandable reference map of SRM assays for CAPs is a valuable resource for designing and accelerating biomarker verification studies.
Synthetic reference map of the N-linked glycoproteome
Glycosylated proteins (glycoproteins) represent a subproteome that is particularly relevant for clinical research because they are usually found either secreted by tissues, thus representing good candidates for detection in easily accessible bodily fluids, or at the cell surface representing potential drug targets Citation[60,61]. Indeed, nearly 80% of the currently used protein biomarkers and drug targets in the clinics are glycosylated Citation[62]. Furthermore, isolation techniques for N-glycosites (i.e., deglycosylated peptides that were N-glycosylated in the intact protein) from plasma or tissue samples have matured in the past 10 years, such as solid phase extraction of N-linked glycopeptides (SPEG) based on chemical immobilization of glycopeptides Citation[63] and lectin enrichment Citation[64]. The captured glycopeptides can be released via PNGase F enzymatical digestion, resulting in the deamidated peptide form for LC–MS/MS. In particular, the SPEG method has achieved success in both cancer biomarker discovery and validation studies, such as prostate cancer Citation[12,65], hepatocellular carcinoma Citation[66] and nonsmall cell lung cancer Citation[67]. Recently, Hüttenhain et al. developed an SRM assay library for 2,007 humans and 1,353 murine N-glycosylated proteins. This reference map consisting of SRM assays for 5,568 N-glycosites is publicly available via the SRMAtlas Citation[68], which can be used for unbiased analysis in plasma biomarker research.
Navigating mass spectrometric maps of clinical samples
Untargeted global navigation using shotgun proteomics
With the latest advanced MS instruments, up to 20–50 precursor ions can be selected for CID fragmentation from a single survey scan, yielding a deep investigation of the proteome in shotgun proteomics Citation[69,70]. However, shotgun navigation of the proteome is performed in an untargeted fashion and results are inconsistent as discussed above Citation[7,9,25]. A deeper comparative analysis of clinical proteomes can be achieved by comprehensive shotgun navigation combined with extensive sample fractionation , which in turn requires a large amount of valuable clinical materials and an extended experimental time Citation[71]. Directed MS, in which the mass spectrometer is directed to select and fragment sets of precursor ions of interest, increases the analytical depth and reproducibility of shotgun proteomics Citation[7,72].
Figure 3. Performance profiles comparing technical advantages and disadvantages of shotgun proteomics, SRM and SWATH MS. In the radar chart, analytical variables are presented on axes staring from the same point and each variable is represented by a spoke. The length of a spoke indicates the magnitude of the variables. Note that SWATH-MS combines the strengths of shotgun and SRM technologies; however, requires more powerful bioinformatic tools for data analysis.
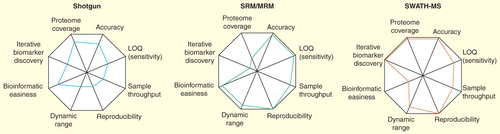
Targeted navigation of clinical proteomes using SRM
With the above-mentioned mass spectrometric reference maps established, researchers can directly perform targeted navigation of a priori selected clinically relevant proteins by SRM . Over the last few years, hundreds of publications have reported achievements and promises of SRM in translational and clinical research, which concluded that targeting MS techniques are compatible with the rigorous requirements for clinical studies such as biomarker validation.
The performance of SRM in navigating clinical proteomes
The direct quantification of proteins in plasma by SRM is preferred because it is simple and efficient permitting high-throughput analysis. In 2009, a multilaboratory study was performed to assess reproducibility, LOD and precision of SRM measurements of proteins in plasma. They reported an intralaboratory CV below 25% for 9 of 10 peptides targeted in plasma Citation[16]. The detection boundary of SRM in unfractionated plasma proteome was determined to be 0.3–1 μg/ml, a range that is further supported by other studies Citation[16,73,74]. Because biomarker candidates such as tissue leakage products are usually of low concentrations (at least nanogram per milliliter), the LOD of SRM for measuring proteins directly in unfractionated plasma is 1–2 orders of magnitudes above the required levels Citation[75]. Consequently, researchers adopted sample preparation strategies to reduce the complexity of plasma. These include depletion of high-abundance proteins, extensive protein- or peptide fractionation, targeted enrichment and isolation of subproteomes or combination of these strategies. These efforts enhanced the sensitivity of SRM assays to 1–10 ng/ml in plasma Citation[76]. Among these strategies, the SPEG enrichment in combination with SRM was shown to facilitate the quantification of N-glycoproteins over a large concentration range reaching nanogram per milliliter levels in plasma Citation[12,77].
Urine is another easily accessible bodily fluid and thus a favored source of biomarker measurements for diagnostics purposes, especially for kidney and bladder diseases. Selevsek et al. quantified 18 proteins by SRM in human urine samples with estimated concentrations between 100 pg/ml and 1 μg/ml and CVs in the range of 10% Citation[78] similar to other reports Citation[10].
In the case of cellular proteomes, Ebhardt et al. estimated the LOD and dynamic range of SRM for unfractionated cellular protein extracts from a human U2OS cell line. They reported that proteins with 25,000 copies per cell were routinely detectable, whereas a lower limit was determined to be 7,500 copies per cell Citation[79].
Taking these data together, it is interesting to compare the different LODs of SRM measurement in different clinical samples (1 μg/ml in total plasma, 1–5 ng/ml in N-glycoproteome of plasma, 0.1ng/ml in urine and 7,500 copies per cell in human cell lines). At first sight, these numbers seem to diverge significantly. However, in fact, the absolute peptide LODs were all determined to be at low attomole range in all the studies. This demonstrates that the observed detection boundaries may merely reflect the loading volume of the processed clinical samples. Importantly, differences in sample complexity seem not to significantly affect the sensitivity of SRM measurements.
Immuno-SRM for increased sensitivity
A technique that has shown potential for the ultrasensitive detection of target peptides is Stable Isotope Standards with Capture by Anti-Peptide Antibodies (SISCAPA Citation[80])) coupled to SRM Citation[81]. In SISCAPA, polyclonal antibodies specific for a particular peptide are immobilized on nanoparticles to capture the target peptide along with its corresponding stable isotope-labeled standard. The eluted peptides are then quantified by SRM. This enrichment significantly increases the sensitivity, particularly for lower abundance proteins in plasma. The reproducibility for the entire immuno-SRM process was demonstrated to have a median intra- and interlaboratory CV below 15% Citation[82,83]. Furthermore, compared to ELISA, SISCAPA has the advantage that the specificity of the assay is provided by MS, significantly relaxing specificity requirements of the antibodies used Citation[82]. Nevertheless, the generation of an acceptable antibody for each peptide might be a limiting factor of this approach. In addition, cross-reactivity between antibodies needs to be tested for multiplexed SISCAPA.
High-throughput navigation & targeted analysis by SWATH MS
SWATH MS combines the strength of discovery proteomics to detect a high number of analytes with the favorable accuracy, dynamic range, sample throughput and reproducibility parameters of SRM . As such, this technique has the capability to address many of the current limitations and questions in clinical biomarker studies. For clinical specimens, such as biopsied tissue, plasma or urine, we propose that the application of SWATH MS will generate a real, quantitative, digitalized proteomic recording (so-called ‘SWATH maps’) as personalized digital representation for each patient. These SWATH maps can be annotated by the above-mentioned mass spectrometric reference maps to achieve the global characterization of the disease proteome . The false-discovery rate (FDR) during this annotation is controlled by the targeted data analysis conceptually derived from SRM using a classical target-decoy searching strategy Citation[14,23].
SWATH MS map in N-linked glycoproteome in human plasma
To assess the potential of SWATH MS for analyzing clinical samples, we compared SWATH MS and SRM-based quantification of N-linked glycoproteins in human plasma. Using dilution series of isotopically heavy labeled peptides representing plasma biomarker candidates, we determined that the sensitivity of SWATH MS is generally only 2–3-times lower compared with SRM (i.e., about 5–10 ng/ml at protein level in the plasma). The average CV of detectable endogenous plasma proteins was found to be 14.90% in SWATH MS and 13.38% in SRM analysis. Moreover, absolute quantification results of SWATH MS and SRM were highly correlated (R2 = 0.9784) Citation[84]. Taken together, SWATH MS coupled with targeted data extraction achieved a slightly lower sensitivity, comparable accuracy, dynamic range and reproducibility compared to state-of-the-art SRM measurements (as summarized in ). This means that SWATH MS analysis, when combined with N-glycosite enrichment, provides a reproducible, deep and quantitative digital map of the human plasma N-glycoproteome, which will permit the concurrent quantification of a significantly higher number of glycoproteins than SRM from similar amounts of sample and in comparable time frames Citation[84].
The complexity and volume of SWATH data sets have led to the development of specialized analysis software tools for SWATH MS . These bioinformatic tools are now available, such as openSWATH (Roest and Rosenberger et al., Manuscript submitted Citation[103]) and SpectronautTM Citation[104], to fulfill the need of confident identification and quantification of proteins in SWATH maps using a target extraction workflow.
Characteristics of SWATH maps
We herein list our perspectives of the key features of applying SWATH maps to clinical research. First, SWATH maps support high-sensitivity detection: Biomarker candidates (e.g., N-linked glycoproteins) can be measured in clinical samples after their enrichment in a single MS run with only a threefold reduced sensitivity compared with SRM. Second, SWATH maps support accurate quantification: the targeted extraction of analytes results in an accuracy comparable to SRM if internal standards are added for the proteins of interest. Third, SWATH maps can be generated in a high-throughput fashion: a single injection combined with an LC gradient of 1–2 h is sufficient to generate the map. Fourth, SWATH maps achieve unprecedented proteome coverage allowing the global profiling and targeted analysis of the detectable fraction of proteomes. Fifth, these maps hold special promises to uncover disease-associated proteomic patterns because the quantitative information for all detectable proteins is recorded for a specific sample type. Sixth, SWATH maps support reproducible quantification just like the SRM technique. Finally, SWATH maps are permanent digital maps that once acquired from different individuals can be always easily and instantly re-examined for the validation of novel emerging biomarkers and new biological hypotheses. They can be easily stored in silico, shared and cross compared.
However, we also would like to emphasize that despite all the promises, the performance of SWATH MS in clinical research still needs to be further evaluated. For example, based on currently available data, SRM is still superior to SWATH MS for the sensitive detection of a small set of target proteins (<100). Because of the limited number of targets in SRM, researchers can determine and report LOD/limit of quantification for all protein targets and perform absolute quantification. Moreover, for diseases based on single mutation, SRM might be a more suitable method to detect and discriminate the mutant form of peptides, because of the narrow precursor filtering window in Q1 and the higher sensitivity Citation[85].
Improved workflows for biomarker studies driven by mass spectrometric maps
Conventional pipeline based on shotgun proteomics
Shotgun proteomics does not require any prior knowledge of the sample composition and has been used as a discovery tool for screening a large number of biomarker candidates in a small set of patient specimens . Despite the use of enrichment procedures, isolation steps Citation[76] and focusing on more relevant biomarker sources (such as cancer secretomes or proximal fluids Citation[86,87])), the discovery phase by shotgun proteomics usually yields 10–100 s candidates even after thorough statistical or biological filtering Citation[88]. Due to the high costs that are accompanied with the development of high-quality immuno-assays (e.g., ELISA kits), only a few candidates (<4–10) are usually selected for verification in suitably powered sample cohorts. Thus, the path from the discovery phase to the final clinical utility is long and uncertain Citation[2]. As a result, researchers tend to make relatively ‘safe choices’ for the verification of candidates, normally based on the literature mining of prior knowledge or already established immunoassays. This leads to the verification of already tested markers rather than testing new promising markers for which no prior knowledge is available Citation[89]. Additionally, selecting only a small number of candidate markers for the verification increases the likelihood of failure. In this case, researchers would need to choose either to test more candidates from the discovered pool, or to go back to the initial steps to recruit more patients or to improve the comprehensiveness of their shotgun experiment .
Figure 4. Models of biomarker pipelines for clinical proteomics adapted for different mass spectrometric approaches. The pipelines are based on shotgun proteomics, SRM technique and the availability of SWATH maps. Note the tendency of less dependency on traditional antibody-based methods and more dependency on MS-based techniques along the evolution of the pipeline models.
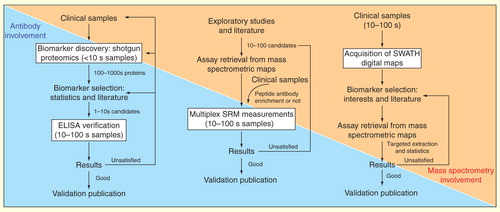
SRM-based alternative pipeline
The application of SRM-based targeted proteomics not only addresses the scarcity of specific affinity reagents available for clinical research, but also presents a compelling alternative pipeline for biomarker discovery and verification . Because of the multiplexing capability of SRM measurements, a higher number of candidates derived from exploratory studies or bioinformatic predictions from genomic data can be verified within a reasonable time scale (<1 h per sample). Two studies published in 2011 applied the SRM-based pipeline to aid discovery and early stage of validation of biomarkers for breast cancer and cardiac injury, respectively Citation[90,91].
A paramount prerequisite in the pipeline is the availability of SRM assays that as proposed in this review can now be directly retrieved from established mass spectrometric reference maps. In this pipeline, if the verification results are unsatisfactory, researchers can focus on selecting more meaningful candidates and perform another cycle of SRM experiments. Currently, the time of measuring 100 proteins in 100 samples is about 2 weeks in experienced labs and this time will increase linearly if either a higher number of proteins or higher number of samples need to be analyzed. Of course, multiple injections will also consume more clinical material.
Novel biomarker pipeline facilitated by SWATH MS & its advantages
We present here a novel pipeline for biomarker studies based on the availability of the SWATH digital maps . Specifically, we propose that SWATH maps should be generated for each clinical sample without prior judgments about proteins to be targeted. In particular, proteomic profiles can then subsequently be extracted in a targeted fashion using assay information derived from mass spectrometric reference maps. Questions on how the proteins in one disease pathway or in one specific functional protein class are regulated could be answered by interrogating the SWATH MS records across sample sets, studies and disease phenotypes. Moreover, because of the theoretical presence of undiscovered biomarkers in the total proteome, pattern recognition algorithms or machine-learning methods could be applied to link SWATH maps with the heterogeneous phenotypes of the samples. Resulting models could then be validated in an independent set of samples. Importantly, the proposed biomarker discovery pipeline is iterative as the number of analytes between discovery and verification phase remains the same here (or, in fact, can be increased as new reference libraries are generated), which is a key advantage compared with shotgun or SRM-based pipelines Citation[92]. This means new sets of biomarkers can be always tested in silico without reanalyzing the same physical sample. This will eventually reduce required resources in terms of time and money for biomarker discovery before early-stage validation .
Example: personalized SWATH digital maps for cancer biomarker discovery
Finally, we provide a possible example of the process for human cancer biomarker discovery that can be accelerated by the unique features of SWATH maps. Traditionally, biomarkers are screened between samples after cancer manifestation, often at relatively late stages in the cancer development after symptoms have occurred . Moreover, clinical samples need to be stored appropriately (e.g., in –80 degree) over a long time and possibly aliquotted to minimize the number of freeze–thaw cycles. The challenges of maintaining suitably annotated samples in biobanks also increases the difficulties to monitor temporal profiles of biomarkers. In contrast, if SWATH maps are immediately acquired after collection, they can be matched and compared between each other, thus allowing for interrogation of diagnostic profiles . This feature will also facilitate the longitudinal profiling of the proteome (e.g., the cancer staging in one patient) and the retrospective identification of biomarkers regulated at the tumor inception stage.
Figure 5. Comparison of the conventional and the new biomarker pipeline assisted by SWATH maps for human cancer. The upper panel indicates the usage of biomarkers in the longitudinal process such as cancer staging (dysplasia to stage IV). Note that, as illustrated in lower panel, once the SWATH map is acquired, it is a permanent record that allows for iterative in silico interrogation for diagnostic profiles.
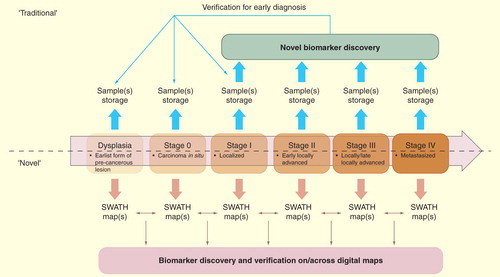
Expert commentary
It has been pointed out that >75% of protein research still focuses on the 10% of proteins that were known and the subject of most protein-based studies before the genome was mapped. This is largely due to the lack of high-quality tools for systematically investigating newly discovered proteins or proteins predicted from the genome sequence Citation[89].
Shotgun proteomics is a routine and mature method to identify and profile peptides and proteins in clinical samples Citation[93]. It is still the method of choice for the characterization of the novel protein variants associated with diseases such as those forms resulted from SNPs Citation[94], alternative splicing Citation[95] and post-translational modifications Citation[96]. Furthermore, label-free quantification in shotgun experiments can be easily applied to clinical tissues and biofluids Citation[97].
The reference mass spectrometric maps provide benchmarks for proteome identification and quantification by any MS strategy. The assay coordinates in these maps not only facilitated SRM measurements and targeted analysis of SWATH data set as we reviewed, but also enhance the protein identification in shotgun proteomics Citation[32]. To maximize the quantitative benefits from SWATH maps, a rigor and thorough experimental design, with various controlling steps during data acquisition, is needed. Based on our experience, we recommend a constant set of internal standards (e.g., a mixture of heavy isotopic peptides corresponding to certain endogenous proteins in different sample types) should be spiked into the sample in same amount. These standards can serve as a benchmark for protein quantification and for normalization of run-to-run variation. Moreover, inclusion of several suitable external intact proteins, for example, from other species allows accounting for variation in protein digestion, whereas the same representative control sample repeatedly measured between sample batches allows to control data quality over long time periods.
Five-year view
In the near future, MS spectral libraries for representative peptides covering the entire human proteome will be available. Also, multiple incremental improvements at each level of MS-based proteomic research will soon enable complete proteome analysis of human samples, with profound impact on both biology and clinical research. The human proteomic research focus will undergo a gradual, though perhaps not complete, switch from protein identification to quantification for specific biological and clinical questions. More reference mass spectrometric maps will be established with respect to particular scientific interests, including completed maps at species level (e.g., human species, animal models like mouse, important pathogens and viruses) and high-resolution maps for specific functionally related subproteomes (protein complexes, transcription factor class, kinases and substrates).
For clinical research, the MS quantification method of choice must be simple, robust, low cost and compatible with the available clinical material. These needs will catalyze a shift from labeling methods (such as SILAC Citation[98] or even iTRAQ Citation[99]) to label-free approaches. Furthermore, since clinical materials are precious and proteomics increasingly becomes capable of analyzing in vivo samples, the issue of handling and analyzing very small tissue amount will come to the fore. In the next few years, another emerging application of targeted proteomics will be the linkage analysis between protein expression and genetic variations, for example, QTL analysis. This type of analysis is crucial to uncover molecular mechanisms underlying complex traits, such as those associated with common diseases like diabetes or cancer Citation[9,35]. We also expect a much wider application of DIA methods such as MSE Citation[100] and SWATH MS in clinical research, because of their unique advantages of monitoring all protein species detectable in a sample at constant sensitivity and reproducibility across large sample cohorts. In particular, SWATH maps will be acquired from tissues and plasma, providing a personalized proteomic blueprint for iterative biomarker studies and eventually for patient-tailored therapies.
Key issues
• The clinical needs of personalized medicine and unbiased biomarker discovery drive the high-throughput, multiplexed, quantitative and cost-effective investigation of clinical proteomes. The better annotation of these proteomes benefits greatly from mass spectrometric reference maps.
• Mass spectrometric reference maps consisting of assays for targeted quantification of PTPs are established or under development such as those of plasma proteome, N-linked glycoproteome, CAPs sets and even the human global proteome.
• The mass spectrometric reference maps are generated by either deep shotgun sequencing of biological samples or by chemically synthesizing a suitable set of peptides that uniquely represent each component in the system. All assays are made publicly accessible through websites such as PeptideAtlas and SRMAtlas.
• Traditional antibody-based protein measurements are the method of choice if a validated assay has been developed. They suffer, however, from the high cost required for their development.
• Shotgun proteomics-based validation, although enabling global protein identification and biomarker screening in unbiased way, mainly suffers from an attrition of proteins detected and quantified between samples.
• SRM-based targeted analysis of proteins in complex samples offers stable, sensitive, quantitative and reproducible quantification. It achieves LOD in the low attmole range in different clinical samples and even 100–1000 lower by utilization of peptide antibody-based enrichment. The SRM-based biomarker discovery pipeline has been widely and successfully used in clinical proteomics. The pipeline is now significantly accelerated by mass spectrometric maps generated for clinical proteomes
• SWATH MS technology was developed to address the problem of low analyte throughput in SRM analysis. As a DIA acquisition method, SWATH MS generates a complete recording of the fragment ion spectra of all analytes detectable in a clinical sample in 2–3 h. SWATH MS coupled with targeted data extraction achieved a slightly lower sensitivity, comparable accuracy, dynamic range and reproducibility compared to state-of-the-art SRM measurements, and therefore combines the strengths of shotgun and SRM analysis.
• The digitalized SWATH maps generated by SWATH MS can be annotated using mass spectrometric reference maps to identify and quantify the global detectable proteome across samples. The SWATH map represents a fast, deep, reproducible and most importantly permanent proteomic recording which promises to avoid sample storage and to support the iterative biomarker discovery and personalized proteome phenotyping.
• We propose that mass spectrometric reference maps and SWATH MS technology will be widely applied in clinical research and the SWATH digital map-assisted biomarker discovery pipeline will to some extent replace the current shotgun or SRM-based pipelines.
Acknowledgment
We would like to acknowledge C Raison for editorial assistance and the whole Aebersold group for the discussion about biomarker discovery projects that led directly to the considerations of the issues discussed in this manuscript.
Financial & competing interests disclosure
Research in the group is supported by early access to advanced instrumentation from AB/Sciex under a collaborative research agreement. The authors acknowledge the support from the National Institutes of Health (NIH Grant # U01CA152813). The authors have no relevant affiliations or financial involvement with any organization or entity with a financial interest in or financial conflict with the subject matter or materials discussed in the manuscript. This includes employment, consultancies, honoraria, stock ownership or options, expert testimony, grants or patents received or pending, or royalties.
No writing assistance was utilized in the production of this manuscript.
Notes
References
- Etzioni R, Urban N, Ramsey S et al. The case for early detection. Nat. Rev. Cancer 3(4), 243–252 (2003).
- Rifai N, Gillette MA, Carr SA. Protein biomarker discovery and validation: the long and uncertain path to clinical utility. Nat. Biotechnol. 24(8), 971–983 (2006).
- Sawyers CL. The cancer biomarker problem. Nature 452(7187), 548–552 (2008).
- Sobin LH. TNM: evolution and relation to other prognostic factors. Semin. Surg. Oncol. 21(1), 3–7 (2003).
- Hamburg MA, Collins FS. The path to personalized medicine. N. Engl. J. Med. 363(4), 301–304 (2010).
- Aebersold R, Anderson L, Caprioli R, Druker B, Hartwell L, Smith R. Perspective: a program to improve protein biomarker discovery for cancer. J. Proteome Res. 4(4), 1104–1109 (2005).
- Domon B, Aebersold R. Options and considerations when selecting a quantitative proteomics strategy. Nat. Biotechnol. 28(7), 710–721 (2010).
- Picotti P, Aebersold R. Selected reaction monitoring-based proteomics: workflows, potential, pitfalls and future directions. Nat. Methods 9(6), 555–566 (2012).
- Picotti P, Bodenmiller B, Aebersold R. Proteomics meets the scientific method. Nat. Methods 10(1), 24–27 (2013).
- Huttenhain R, Soste M, Selevsek N et al. Reproducible quantification of cancer-associated proteins in body fluids using targeted proteomics. Sci. Transl. Med. 4(142), 142ra194 (2012).
- Kalin M, Cima I, Schiess R et al. Novel prognostic markers in the serum of patients with castration-resistant prostate cancer derived from quantitative analysis of the pten conditional knockout mouse proteome. Eur. Urol. 60(6), 1235–1243 (2011).
- Cima I, Schiess R, Wild P et al. Cancer genetics-guided discovery of serum biomarker signatures for diagnosis and prognosis of prostate cancer. Proc. Natl Acad. Sci. USA 108(8), 3342–3347 (2011).
- Gillet LC, Navarro P, Tate S et al. Targeted data extraction of the MS/MS spectra generated by data-independent acquisition: a new concept for consistent and accurate proteome analysis. Mol. Cell. Proteomics 11(6), O111 016717 (2012).
- Reiter L, Rinner O, Picotti P et al. mProphet: automated data processing and statistical validation for large-scale SRM experiments. Nat. Methods 8(5), 430–435 (2011).
- Diamandis EP. The failure of protein cancer biomarkers to reach the clinic: why, and what can be done to address the problem? BMC Med. 10, 87 (2012).
- Addona TA, Abbatiello SE, Schilling B et al. Multi-site assessment of the precision and reproducibility of multiple reaction monitoring-based measurements of proteins in plasma. Nat. Biotechnol. 27(7), 633–641 (2009).
- Roobol MJ, Carlsson SV. Risk stratification in prostate cancer screening. Nat. Rev. Urol. 10(1), 38–48 (2013).
- Karam AK, Karlan BY. Ovarian cancer: the duplicity of CA125 measurement. Nat. Rev. Clin. Oncol. 7(6), 335–339 (2010).
- Del Mastro L, Lambertini M, Bighin C et al. Trastuzumab as first-line therapy in HER2-positive metastatic breast cancer patients. Expert Rev. Anticancer Ther. 12(11), 1391–1405 (2012).
- Domon B, Aebersold R. Mass spectrometry and protein analysis. Science 312(5771), 212–217 (2006).
- Aebersold R, Mann M. Mass spectrometry-based proteomics. Nature 422(6928), 198–207 (2003).
- Sabido E, Selevsek N, Aebersold R. Mass spectrometry-based proteomics for systems biology. Curr. Opin. Biotechnol. 23(4), 591–597 (2012).
- Elias JE, Gygi SP. Target-decoy search strategy for increased confidence in large-scale protein identifications by mass spectrometry. Nat. Methods 4(3), 207–214 (2007).
- Cox J, Mann M. MaxQuant enables high peptide identification rates, individualized p.p.b.-range mass accuracies and proteome-wide protein quantification. Nat. Biotechnology 26(12), 1367–1372 (2008).
- Tabb DL, Vega-Montoto L, Rudnick PA et al. Repeatability and reproducibility in proteomic identifications by liquid chromatography-tandem mass spectrometry. J. Proteome Res. 9(2), 761–776 (2010).
- Kim YJ, Feild B, Fitzhugh W et al. Reference map for liquid chromatography-mass spectrometry-based quantitative proteomics. Anal Biochem. 393(2), 155–162 (2009).
- Schmidt A, Gehlenborg N, Bodenmiller B et al. An integrated, directed mass spectrometric approach for in-depth characterization of complex peptide mixtures. Mol. Cell. Proteomics 7(11), 2138–2150 (2008).
- Jaffe JD, Keshishian H, Chang B, Addona TA, Gillette MA, Carr SA. Accurate inclusion mass screening: a bridge from unbiased discovery to targeted assay development for biomarker verification. Mol. Cell. Proteomics 7(10), 1952–1962 (2008).
- Peterson AC, Russell JD, Bailey DJ, Westphall MS, Coon JJ. Parallel reaction monitoring for high resolution and high mass accuracy quantitative, targeted proteomics. Mol. Cell. Proteomics 11(11), 1475–1488 (2012).
- Lange V, Picotti P, Domon B, Aebersold R. Selected reaction monitoring for quantitative proteomics: a tutorial. Mol. Syst. Biol. 4, 222 (2008).
- Nilsson T, Mann M, Aebersold R, Yates JR 3rd, Bairoch A, Bergeron JJ. Mass spectrometry in high-throughput proteomics: ready for the big time. Nat. Methods 7(9), 681–685 (2010).
- Lam H, Deutsch EW, Eddes JS, Eng JK, Stein SE, Aebersold R. Building consensus spectral libraries for peptide identification in proteomics. Nat. Methods 5(10), 873–875 (2008).
- Lam H, Aebersold R. Spectral library searching for peptide identification via tandem MS. Methods Mol. Biol. 604, 95–103 (2010).
- Lam H, Deutsch EW, Eddes JS et al. Development and validation of a spectral library searching method for peptide identification from MS/MS. Proteomics 7(5), 655–667 (2007).
- Picotti P, Clement-Ziza M, Lam H et al. A complete mass-spectrometric map of the yeast proteome applied to quantitative trait analysis. Nature 494(7436), 266–270 (2013).
- Deutsch EW, Lam H, Aebersold R. PeptideAtlas: a resource for target selection for emerging targeted proteomics workflows. EMBO Rep. 9(5), 429–434 (2008).
- Frank R. The SPOT-synthesis technique. Synthetic peptide arrays on membrane supports--principles and applications. J. Immunol. Methods 267(1), 13–26 (2002).
- Picotti P, Rinner O, Stallmach R et al. High-throughput generation of selected reaction-monitoring assays for proteins and proteomes. Nat. Methods 7(1), 43–46 (2010).
- Ahrens CH, Brunner E, Qeli E, Basler K, Aebersold R. Generating and navigating proteome maps using mass spectrometry. Nat. Rev. Mol. Cell Biol. 11(11), 789–801 (2010).
- Karlsson C, Malmstrom L, Aebersold R, Malmstrom J. Proteome-wide selected reaction monitoring assays for the human pathogen Streptococcus pyogenes. Nat. Commun. 3, 1301 (2012).
- Schubert OT, Mouritsen J, Ludwig C et al. The Mtb Proteome Library: A Resource of Assays to Quantify the Complete Proteome of Mycobacterium tuberculosis. Cell Host Microbe 13(5), 602–612 (2013).
- Desiere F, Deutsch EW, King NL et al. The PeptideAtlas project. Nucleic Acids Res. 34(Database issue), D655–D658 (2006).
- Mathivanan S, Ahmed M, Ahn NG et al. Human Proteinpedia enables sharing of human protein data. Nat. Biotechnol. 26(2), 164–167 (2008).
- Craig R, Cortens JP, Beavis RC. Open source system for analyzing, validating, and storing protein identification data. J. Proteome Res. 3(6), 1234–1242 (2004).
- Jones P, Cote RG, Cho SY et al. PRIDE: new developments and new datasets. Nucleic Acids Res. 36(Database issue), D878–D883 (2008).
- Farrah T, Deutsch EW, Kreisberg R et al. PASSEL: the Pepti deAtlas SRM experiment library. Proteomics 12(8), 1170–1175 (2012).
- Zhang H, Liu AY, Loriaux P et al. Mass spectrometric detection of tissue proteins in plasma. Mol. Cell. Proteomics 6(1), 64–71 (2007).
- Anderson NL, Anderson NG. The human plasma proteome: history, character, and diagnostic prospects. Mol. Cell. Proteomics 1(11), 845–867 (2002).
- States DJ, Omenn GS, Blackwell TW et al. Challenges in deriving high-confidence protein identifications from data gathered by a HUPO plasma proteome collaborative study. Nat. Biotechnol 24(3), 333–338 (2006).
- Farrah T, Deutsch EW, Omenn GS et al. A high-confidence human plasma proteome reference set with estimated concentrations in PeptideAtlas. Mol. Cell. Proteomics 10(9), M110 006353 (2011).
- Taussig MJ, Stoevesandt O, Borrebaeck CA et al. ProteomeBinders: planning a European resource of affinity reagents for analysis of the human proteome. Nat. Methods 4(1), 13–17 (2007).
- Lundberg E, Fagerberg L, Klevebring D et al. Defining the transcriptome and proteome in three functionally different human cell lines. Mol. Syst. Biol. 6, 450 (2010).
- Mann M, Kulak NA, Nagaraj N, Cox J. The coming age of complete, accurate, and ubiquitous proteomes. Mol. Cell 49(4), 583–590 (2013).
- Nagaraj N, Wisniewski JR, Geiger T et al. Deep proteome and transcriptome mapping of a human cancer cell line. Mol. Syst. Biol. 7, 548 (2011).
- Beck M, Schmidt A, Malmstroem J et al. The quantitative proteome of a human cell line. Mol. Syst. Biol. 7, 549 (2011).
- Wisniewski JR, Ostasiewicz P, Dus K, Zielinska DF, Gnad F, Mann M. Extensive quantitative remodeling of the proteome between normal colon tissue and adenocarcinoma. Mol. Syst. Biol. 8, 611 (2012).
- Fagerberg L, Oksvold P, Skogs M et al. Contribution of antibody-based protein profiling to the human Chromosome-centric Proteome Project (C-HPP). J. Proteome Res. (2012).
- Farrah T, Deutsch EW, Hoopmann MR et al. The state of the human proteome in 2012 as viewed through PeptideAtlas. J. Proteome Res. 12(1), 162–171 (2013).
- Polanski M, Anderson NL. A list of candidate cancer biomarkers for targeted proteomics. Biomarker insights 1, 1–48 (2007).
- Wollscheid B, Bausch-Fluck D, Henderson C et al. Mass-spectrometric identification and relative quantification of N-linked cell surface glycoproteins. Nat. Biotechnol. 27(4), 378–386 (2009).
- Roth J. Protein N-glycosylation along the secretory pathway: relationship to organelle topography and function, protein quality control, and cell interactions. Chem. Rev. 102(2), 285–303 (2002).
- Schiess R, Wollscheid B, Aebersold R. Targeted proteomic strategy for clinical biomarker discovery. Mol. Oncol. 3(1), 33–44 (2009).
- Zhang H, Li XJ, Martin DB, Aebersold R. Identification and quantification of N-linked glycoproteins using hydrazide chemistry, stable isotope labeling and mass spectrometry. Nat. Biotechnol. 21(6), 660–666 (2003).
- Zielinska DF, Gnad F, Wisniewski JR, Mann M. Precision mapping of an in vivo N-glycoproteome reveals rigid topological and sequence constraints. Cell 141(5), 897–907 (2010).
- Tian Y, Bova GS, Zhang H. Quantitative glycoproteomic analysis of optimal cutting temperature-embedded frozen tissues identifying glycoproteins associated with aggressive prostate cancer. Anal Chem. 83(18), 7013–7019 (2011).
- Chen R, Tan Y, Wang M et al. Development of glycoprotein capture-based label-free method for the high-throughput screening of differential glycoproteins in hepatocellular carcinoma. Mol. Cell. Proteomics 10(7), M110 006445 (2011).
- Zeng X, Hood BL, Sun M et al. Lung cancer serum biomarker discovery using glycoprotein capture and liquid chromatography mass spectrometry. J. Proteome Res. 9(12), 6440–6449 (2010).
- Huttenhain R, Surinova S, Ossola R et al. N-Glycoprotein SRMAtlas: a resource of mass-spectrometric assays for N-glycosites enabling consistent and multiplexed protein quantification for clinical applications. Mol. Cell. Proteomics 12(4), 1005–1016 (2013).
- Michalski A, Damoc E, Lange O et al. Ultra high resolution linear ion trap Orbitrap mass spectrometer (Orbitrap Elite) facilitates top down LC MS/MS and versatile peptide fragmentation modes. Mol. Cell. Proteomics 11(3), O111 013698 (2012).
- Cristobal A, Hennrich ML, Giansanti P, Goerdayal SS, Heck AJ, Mohammed S. In-house construction of a UHPLC system enabling the identification of over 4000 protein groups in a single analysis. Analyst 137(15), 3541–3548 (2012).
- Wu L, Han DK. Overcoming the dynamic range problem in mass spectrometry-based shotgun proteomics. Expert Rev. Proteomics 3(6), 611–619 (2006).
- Schmidt A, Claassen M, Aebersold R. Directed mass spectrometry: towards hypothesis-driven proteomics. Curr. Opin. Chem. Biol. 13(5–6), 510–517 (2009).
- Liu YS, Luo XY, Li QR et al. Shotgun and targeted proteomics reveal that pre-surgery serum levels of LRG1, SAA, and C4BP may refine prognosis of resected squamous cell lung cancer. J. Mol. Cell Biol. 4(5), 344–347 (2012).
- Kuzyk MA, Smith D, Yang J et al. Multiple reaction monitoring-based, multiplexed, absolute quantitation of 45 proteins in human plasma. Mol. Cellular Proteomics 8(8), 1860–1877 (2009).
- Surinova S, Schiess R, Huttenhain R, Cerciello F, Wollscheid B, Aebersold R. On the development of plasma protein biomarkers. J. Proteome Res. 10(1), 5–16 (2011).
- Huttenhain R, Malmstrom J, Picotti P, Aebersold R. Perspectives of targeted mass spectrometry for protein biomarker verification. Current Opin. Chem. Biol. 13(5–6), 518–525 (2009).
- Stahl-Zeng J, Lange V, Ossola R et al. High sensitivity detection of plasma proteins by multiple reaction monitoring of N-glycosites. Mol. Cell. Proteomics 6(10), 1809–1817 (2007).
- Selevsek N, Matondo M, Sanchez Carbayo M, Aebersold R, Domon B. Systematic quantification of peptides/proteins in urine using selected reaction monitoring. Proteomics 11(6), 1135–1147 (2011).
- Ebhardt HA, Sabido E, Huttenhain R, Collins B, Aebersold R. Range of protein detection by selected/multiple reaction monitoring mass spectrometry in an unfractionated human cell culture lysate. Proteomics 12(8), 1185–1193 (2012).
- Anderson NL, Anderson NG, Haines LR, Hardie DB, Olafson RW, Pearson TW. Mass spectrometric quantitation of peptides and proteins using Stable Isotope Standards and Capture by Anti-Peptide A ntibodies (SISCAPA). J. Proteome Res. 3(2), 235–244 (2004).
- Whiteaker JR, Zhao L, Anderson L, Paulovich AG. An automated and multiplexed method for high throughput peptide immunoaffinity enrichment and multiple reaction monitoring mass spectrometry-based quantification of protein biomarkers. Mol. Cell. Proteomics 9(1), 184–196 (2010).
- Kuhn E, Addona T, Keshishian H et al. Developing multiplexed assays for troponin I and interleukin-33 in plasma by peptide immunoaffinity enrichment and targeted mass spectrometry. Clin. Chem. 55(6), 1108–1117 (2009).
- Kuhn E, Whiteaker JR, Mani DR et al. Interlaboratory evaluation of automated, multiplexed peptide immunoaffinity enrichment coupled to multiple reaction monitoring mass spectrometry for quantifying proteins in plasma. Mol. Cell. Proteomics 11(6), M111 013854 (2012).
- Liu Y, Huttenhain R, Surinova S et al. Quantitative measurements of N-linked glycoproteins in human plasma by SWATH-MS. Proteomics 13(8), 1247–1256 (2013).
- Wang Q, Chaerkady R, Wu J et al. Mutant proteins as cancer-specific biomarkers. Proc. Natl Acad. Sci. USA 108(6), 2444–2449 (2011).
- Makridakis M, Vlahou A. Secretome proteomics for discovery of cancer biomarkers. J. Proteomics 73(12), 2291–2305 (2010).
- Luo X, Liu Y, Wang R, Hu H, Zeng R, Chen H. A high-quality secretome of A549 cells aided the discovery of C4b-binding protein as a novel serum biomarker for non-small cell lung cancer. J. Proteomics 74(4), 528–538 (2011).
- Liu Y, Luo X, Hu H et al. Integrative proteomics and tissue microarray profiling indicate the association between overexpressed serum proteins and non-small cell lung cancer. PloS one 7(12), e51748 (2012).
- Edwards AM, Isserlin R, Bader GD, Frye SV, Willson TM, Yu FH. Too many roads not taken. Nature 470(7333), 163–165 (2011).
- Whiteaker JR, Lin C, Kennedy J et al. A targeted proteomics-based pipeline for verification of biomarkers in plasma. Nat. Biotechnol. 29(7), 625–634 (2011).
- Addona TA, Shi X, Keshishian H et al. A pipeline that integrates the discovery and verification of plasma protein biomarkers reveals candidate markers for cardiovascular disease. Nat. Biotechnol. 29(7), 635–643 (2011).
- Latterich M, Schnitzer JE. Streamlining biomarker discovery. Nat. Biotechnol. 29(7), 600–602 (2011).
- Geiger T, Cox J, Ostasiewicz P, Wisniewski JR, Mann M. Super-SILAC mix for quantitative proteomics of human tumor tissue. Nat. Methods 7(5), 383–385 (2010).
- Li J, Su Z, Ma ZQ et al. A bioinformatics workflow for variant peptide detection in shotgun proteomics. Mol. Cell. Proteomics 10(5), M110 006536 (2011).
- Sheynkman GM, Shortreed MR, Frey BL, Smith LM. Discovery and mass spectrometric analysis of novel splice-junction peptides using RNA-Seq. Mol. Cell. Proteomics 12(8), 2341–2353 (2013).
- Ostasiewicz P, Zielinska DF, Mann M, Wisniewski JR. Proteome, phosphoproteome, and N-glycoproteome are quantitatively preserved in formalin-fixed paraffin-embedded tissue and analyzable by high-resolution mass spectrometry. J. Proteome Res. 9(7), 3688–3700 (2010).
- Pham TV, Piersma SR, Oudgenoeg G, Jimenez CR. Label-free mass spectrometry-based proteomics for biomarker discovery and validation. Expert Rev. Molecular Diagn. 12(4), 343–359 (2012).
- Ong SE, Blagoev B, Kratchmarova I et al. Stable isotope labeling by amino acids in cell culture, SILAC, as a simple and accurate approach to expression proteomics. Mol. Cell. Proteomics 1(5), 376–386 (2002).
- Ross PL, Huang YN, Marchese JN et al. Multiplexed protein quantitation in Saccharomyces cerevisiae using amine-reactive isobaric tagging reagents. Mol. Cell. Proteomics 3(12), 1154–1169 (2004).
- Plumb RS, Johnson KA, Rainville P et al. UPLC/MS(E); a new approach for generating molecular fragment information for biomarker structure elucidation. Rapid Commun. Mass Spectrom. 20(13), 1989–1994 (2006).
Websites
- Peptide Atlas. www.peptideatlas.org
- SRM Atlas. www.srmatlas.org
- The openSWATH. www.openSWATH.org
- The website of bignosys AG. www.biognosys.ch