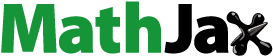
Abstract
Biodistribution of nanoparticles is dependent on their physicochemical properties (such as size, surface charge, and surface hydrophilicity). Clear and systematic understanding of nanoparticle properties’ effects on their in vivo performance is of fundamental significance in nanoparticle design, development and optimization for medical applications, and toxicity evaluation. In the present study, a physiologically based pharmacokinetic model was utilized to interpret the effects of nanoparticle properties on previously published biodistribution data. Biodistribution data for five poly(lactic-co-glycolic) acid (PLGA) nanoparticle formulations prepared with varied content of monomethoxypoly (ethyleneglycol) (mPEG) (PLGA, PLGA-mPEG256, PLGA-mPEG153, PLGA-mPEG51, PLGA-mPEG34) were collected in mice after intravenous injection. A physiologically based pharmacokinetic model was developed and evaluated to simulate the mass-time profiles of nanoparticle distribution in tissues. In anticipation that the biodistribution of new nanoparticle formulations could be predicted from the physiologically based pharmacokinetic model, multivariate regression analysis was performed to build the relationship between nanoparticle properties (size, zeta potential, and number of PEG molecules per unit surface area) and biodistribution parameters. Based on these relationships, characterized physicochemical properties of PLGA-mPEG495 nanoparticles (a sixth formulation) were used to calculate (predict) biodistribution profiles. For all five initial formulations, the developed model adequately simulates the experimental data indicating that the model is suitable for description of PLGA-mPEG nanoparticle biodistribution. Further, the predicted biodistribution profiles of PLGA-mPEG495 were close to experimental data, reflecting properly developed property–biodistribution relationships.
Introduction
It is well established that adjusting nanoparticle properties such as size and surface charge can modify the biodistribution of nanoparticles. Optimized nanoparticle formulations with specific distribution (targeting) within the body for medical applications, including drug delivery and cancer diagnosis, are highly sought. Many chemicals, polymers, preparation methods, and surface ligands have been, and are currently being, developed to specifically achieve high nanoparticle accumulation in target tissues such as tumors. However, it is not explicitly clear how nanoparticle properties influence nanoparticle absorption, distribution, metabolism, elimination, and toxicity. Quantitative methods beyond pharmacokinetics of the therapeutic are needed to describe and predict the effects of nanoparticle properties on in vivo performance. Without clear understanding of such property-absorption/distribution/metabolism/elimination relationships, the effects of any property modification can only be tested with animal experimentation, which is resource (time and cost) intensive. Considering the numerous materials and methods for nanoparticle preparation, it is impractical to screen them all in vivo. Rational methodologies are urgently needed to assist in the evaluation of nanoparticle targeting/biodistribution and analysis of available data for optimization and designation of nanoparticles.
Biodistribution of nanoparticles is determined by their properties when other conditions are held constant (ie, animal models and administration routes). Therefore, relationships should exist between nanoparticle properties and biodistribution, similar to the quantitative structure-activity relationships of chemical molecules.Citation1 Specific description of such relationships would greatly aid the design of nanoparticles for medical applications. Although numerous studies have qualitatively proven the existence of such relationships,Citation2 no work to date has defined them with quantitative analysis. To build such quantitative relationships, the biodistribution of a series of nanoparticle formulations should be obtained. Both nanoparticle properties and biodistribution need to be quantified for mathematical description of nanoparticle property– biodistribution relationships. Nanoparticle properties are relatively easy to measure, although the required degree and type of characterization is still not well established.Citation3 It is much more difficult to quantify biodistribution. Generally, biodistribution is reported as nanoparticle tissue concentrations or nanoparticle mass in tissues at various terminal time points. It is difficult to compare such static and isolated data points among different studies, especially when different study designs are used (ie, nanoparticle doses, animal models, administration routes, and sampling time points). A more rational method should be adopted for quantifying biodistribution kinetic profiles, and physiologically based pharmacokinetic (PBPK) models may prove to be the ideal tool for this purpose.Citation4
PBPK models list tissues and organs as individual compartments and the nanoparticle transportation among them is described by mathematical rate equations. With PBPK modeling, biodistribution is quantified using a set of parameters, which are only dependent on nanoparticle properties, but relatively independent to study design. These parameters can then be more easily compared among different studies. PBPK models have been used for simulation of the biodistribution of small chemical molecules for more than 30 years,Citation4 and have advantageously been used in toxicity and biodistribution studies. A specific application with demonstrated success is the building of structure-pharmacokinetic relationshipsCitation5 that quantitatively correlate the chemical structures of drugs to their pharmacokinetic profiles. Similarly, the authors believe they may be used to build the relationships between nanoparticle properties and their biodistribution.
Initially, fitting experimental biodistribution data into the PBPK model can generate a set of parameters. The parameters can then be correlated to nanoparticle properties by mathematical equations. These equations could be used to analyze the contributions of nanoparticle properties to individual transportation processes within the body. Apart from aiding the understanding of nanoparticle property effects on biodistribution, such property–biodistribution relationships could have further applications. For example, biodistribution kinetic parameters of new nanoparticle formulations could be calculated based on their properties and used for the prediction of their biodistribution. These relationships could also guide designation of nanoparticles with particular properties in order to quantitatively control their biodistribution for specific applications.
The application of PBPK models to nanoparticle biodistribution studies is a recently emerging field, as was discussed in greater detail in a recent review.Citation6 It is more difficult to build PBPK models for nanoparticles than for small molecules due to distinct differences in their physiological processes within the body. Despite the limited application of PBPK models to nanoparticles (and the limited complexity of the models used to date), PBPK modeling has great potential in nanoparticle research. In one such work, Lankveld et alCitation7 use PBPK modeling to compare the distribution kinetics of silver nanoparticles of different sizes. Although this work represents the only study to date that defines a quantitative relationship between a nanoparticle property (size) and tissue kinetics, no significant relationship could be determined despite significant differences in the experimental data. Lankveld et al hypothesized that other properties, such as surface properties, were key determinants of biodistribution kinetics.
In a previous study, the biodistribution of five nanoparticle formulations prepared with poly(lactic-co-glycolic) acid (PLGA) with varied content of monomethoxypoly (ethyleneglycol) (mPEG) (PLGA, PLGA-mPEG256, PLGA-mPEG153, PLGA-mPEG61, and PLGA-mPEG34) was investigated.Citation8 Physicochemical properties of these nanoparticle formulations (size, surface charge, and surface PEG content) varied as a function of mPEG content. It was demonstrated that distribution of these nanoparticles is influenced by their properties. Here, a PBPK model is used to explain the in vivo behavior of these nanoparticle formulations, and furthermore, establish quantitative relationships between nanoparticle properties and biodistribution. These relationships were then used to predict biodistribution of a sixth nanoparticle formulation (PLGA-mPEG495).
Materials and methods
Experimental data
Most of the experimental data used in this study, and the characterization of the described nanoparticles, has been reported elsewhere.Citation8 The tissue distribution of the 125I-CA (5-cholesten-3-[N-phenyl]amine) label and the 125I-CA-labeled PLGA and PLGA-mPEG nanoparticles was determined in female Swiss-De mice weighing 25–30 g. The animals, three per group, were injected in the tail vein with 100 μL of nanoparticles (300 μg polymer per mouse). At 0, 0.033, 0.5, 1, 3, and 6 hours, the mice were sacrificed and their tissues (liver, spleen, lungs, muscle, bone – femur of left hind leg, intestines, kidney, urinary bladder, brain, and thyroid) were excised. Tissues were then quickly washed with cold water to remove surface blood, and counted for radioactivity. Blood samples (0.07–0.08 g) were obtained in duplicate by cardiac puncture in preweighed heparinized tubes. The radioactivity remaining in the tail was also measured and taken into consideration in the calculation of total radioactivity dose administered to the animals. In the calculations of the percentage dose per organ, blood, bone, and muscle were considered to constitute the 7%, 10%, and 43% of the body weight, respectively.Citation9
In a previous paper,Citation8 the authors reported the mass-time courses of blood, liver, spleen, bones, muscles, intestine, lungs, and urine. Some other tissues with lower distribution were not presented (kidneys, thyroid, stomach, and brain), but are included here. To be consistent with the experiments, the data in each compartment are presented as percentage of initial dose. As nanoparticle recovery from the experiments was very good (more than 95% of initial dose, ), it is assumed that no significant distribution occurred into other tissues that were not tested.
Table 1 Dose recovery of poly(lactic-co-glycolic) acid and poly(lactic-co-glycolic) acid- monomethoxypoly (ethyleneglycol) nanoparticles at various time points
PBPK model development and evaluation
The first step of PBPK model building is to determine the organs and tissues to be identified as compartments. Based on the experimental data, the whole body was divided into seven compartments (blood, lungs, gastrointestinal tract (GI), liver, spleen, kidneys, and body) with the blood connecting all compartments. The liver and spleen were the major organs for nanoparticle distribution in previous work.Citation8 A GI lumen compartment was included for nanoparticles excreted through bile, and the kidney compartment includes a subcompartment for urine excretion. The remaining organs and tissues were combined into one compartment named the body (bones, muscles, thyroid, and brain). It was assumed that degradation did not occur within the relatively short time period of experiments (6 hours).
Experimentally, bile-excreted nanoparticles were not separated from those within the GI tissue. To evaluate bile excretion, the data should be divided. Because the mass of nanoparticles in the GI was low for all nanoparticle formulations (the highest value was 6.15%), both the distribution into GI tissue and bile excretion must be lower than that, and thus have little influence on the distribution of other tissues. Based on this consideration, nanoparticles in the GI were first considered as all in GI tissue without any bile excretion (no nanoparticles in GI lumen). With model simulation, predicted values of each time point were obtained. Then the experimental data were considered as solely bile excretion (all nanoparticles in GI lumen), and predicted values generated again with model simulation. The ratio of the simulated values at each time point from both simulations was calculated and experimental data were normalized by the ratio and used for the final simulation.
PBPK models are generally divided into two groups according to transportation mechanisms: blood flow-limited and membrane-limited models.Citation4 The blood flow-limited model assumes that every organ is a well-stirred compartment and nanoparticle distribution between blood and tissue instantly reaches equilibrium. The only factor limiting the transportation between blood and tissue is the blood flow rates for each tissue. For membrane-limited models, it is assumed that transportation between blood and tissue is limited by permeability of the blood capillary membrane or tissue cell membrane. In this case, only the blood capillary wall and/or cellular barriers determine transportation of nanoparticles between blood and tissue.
Both blood flow-limited and membrane-limited models were both built in this work (the structures of these models are presented in ), and evaluated for how well the experimental data can be simulated by each as follows:
Figure 1 Schematic diagrams of the blood flow-limited model (A) and the membrane-limited model (B). Arrows indicate the transportation of nanoparticles with the dashed arrows in (B), indicating differing transportation equations from solid arrows in (A).
Note: Triangles represent the intravenous administration.
Abbreviation: GI, gastrointestinal tract.

EquationEquation 1(1) evaluates the differences between estimated data and observed data as described previously.Citation9 An R2 value close to unit means the best prediction of the experimental data by the model.
For all five formulations, the membrane-limited model performed better than the blood flow-limited model (). As a result, the membrane-limited model was selected for all other work described in this study.
Table 2 Evaluation of physiologically based pharmacokinetic model structures for nanoparticle formulations (R2)
Mass transfer rate equations and parameter optimization
Rate equations for each compartment were obtained using the basic principle of mass balance conservation: the net amount of nanoparticles in the organ must equal the sum of all nanoparticles that enter the organ minus all nanoparticles that leave the organ. For this type of model, a single mass balance differential equation describes the nanoparticle distribution for each separate organ as shown in the following equation:
where Cp and Mp refer to the nanoparticle concentration and amount in the blood, Ci, Mi, Ri, ki, Vi, and CLi refer to the nanoparticle concentration, nanoparticle amount, tissue–blood partition coefficient, diffusion coefficient, tissue volume, and excretion coefficient in tissue i, respectively. For tissues that have no excretion, the CLi will be zero. Nanoparticle amounts in tissues were obtained from experimental data as previously described. Tissue volume values were collected from the literature.Citation10
The mass balance equation for the blood compartment is:
Parameter optimizations were performed with MATLAB® software (version R2009a; Mathworks, Natick, MA) using the least square optimization method with all parameters estimated simultaneously. This is a commonly used method for nonlinear curve fitting. The diffusion coefficients and partition coefficients were allowed to vary within limitations, which were determined as described below.
An open-loop method was first carried out to calculate the initial values of diffusion coefficients for final estimation. The blood mass-time data were fitted into a biexponential function:
where A and B are the intercepts for each exponential segment of the blood mass-time curve. EquationEquation 4(4) describes the blood mass-time profile and serves as a forcing function.Citation11 It was held constant in the PBPK model and used as the input function to fit the nanoparticle values for each compartment individually, allowing values for the diffusion coefficients to be obtained and used as the initial values for further optimization.
Initial values for excretion coefficients were also estimated. The accumulation of nanoparticles excreted from the kidney compartment is expressed as:
where Murine is the accumulated nanoparticle mass in the urine, CLkidney is the excretion coefficient for the kidney, and Mkidney is the nanoparticle mass in kidney at time t. To calculate the initial excretion value, the average nanoparticle mass in the kidney compartment (M̄kidney) during the entire study period (0–6 hours) was used. As M̄kidney is a constant, the above equation converts to:
Experimental data was fit into this linear equation to calculate the initial excretion value from the kidney compartment (kCL,kidney). The initial value of the liver excretion coefficient was estimated the same way.
First, upper and lower limitations of diffusion and excretion coefficients were determined. The lower limitation was set at zero (all values are positive). The upper limits of diffusion coefficients were determined based on blood flow rates from the literature,Citation10 considering that nanoparticle distribution from blood into tissues could not be faster than the rate at which blood carries nanoparticles into that tissue. Similar upper limits were set for excretion coefficients:
where Qi is the blood flow rate of tissue i and Vp is the volume of plasma.
With the initial values determined, a closed-loop method was used to globally estimate all the parameters simultaneously for each of the five nanoparticle formulations. The model parameters were adjusted by the software to minimize the difference between simulated values and experimental data points.
PBPK model implementation
After the parameters were determined, the mathematical equations describing the mass-time profiles of the five nanoparticle formulations were programmed into the MATLAB software. Simulation was then carried out producing mass- time curves for comparison with experimental data.
Sensitivity analysis
Some model parameters may be more sensitive than others in shaping the mass-time profiles of the tissue compartments. Slight changes in these parameters could result in significant changes in the simulated nanoparticle distribution. Identification and consideration of these sensitive parameters is needed when optimizing nanoparticle biodistribution simulation. As the general purpose of PEG-modified nanoparticles is to increase blood circulation time, sensitivity analysis was evaluated using the area under the curve (AUC) of blood from time zero to 6 hours. To test sensitivity, the value of an individual parameter was increased by 1%, model simulations repeated, and the blood AUC recalculated. This process was done for each parameter of the model. Relative sensitivity coefficients for all parameters were calculated using the following equation:Citation11
ie, the percentage change in AUC divided by the percentage change in the parameter k.
Correlation of diffusion coefficients with nanoparticle properties
Although biodistribution is determined by many individual nanoparticle properties, it is difficult to interpret the interplay of multiple properties and how these influence the interaction between nanoparticles and the human body. Since multiple properties simultaneously determine individual transportation parameters, they should be evaluated simultaneously for individual in vivo processes. For example, both surface charge and size have each been shown to influence liver excretion of nanoparticles.Citation12–Citation14 Given this, it would be difficult to reach clear conclusions by correlating transportation parameters to a single nanoparticle property. A commonly used method to analyze the influence of more than one independent variable on a single dependent property is multivariate regression.Citation15 With multivariate regression analysis, the effects of multiple properties on one particular parameter can be simultaneously evaluated.Citation15 Currently, there is little known about how nanoparticle properties affect individual transportation kinetic parameters. Reported here for the first time is a linear multivariate regression between each parameter and three nanoparticle properties.
The nanoparticle properties (size, zeta potential, NPEG) evaluated are listed in . NPEG is the number of PEG molecules per unit surface area as previously reported. The equation for each parameter takes the following format:
Table 3 Nanoparticle properties
where x1, x2, and x3 are nanoparticle size, zeta potential, and NPEG values, and A, B, and C are the constants to be estimated.
Prediction of biodistribution profiles of PLGA-mPEG495 nanoparticles
Once the relationship between nanoparticle properties and an individual transportation parameter is established, the value of that parameter for specific nanoparticle properties is predictable. For a nanoparticle formulation of known properties, all necessary parameters for the PBPK model can be calculated resulting in a predicted simulation of the biodistribution mass-time curves.
Here, the parameters for PLGA-mPEG495 nanoparticles from its properties were calculated () and the property- distribution relationship equations generated above. These parameters were then used to simulate the biodistribution profiles using the membrane-limited model structure. PLGA-mPEG495 nanoparticles were then tested for their biodistribution at 3 hours and compared with predicted values.
Results
PBPK model development and evaluation
Both blood flow-limited and membrane-limited models were analyzed to determine which could best simulate nanoparticle biodistribution data. It was found that the membrane-limited model performed better for all five formulations (). For PLGA nanoparticles without PEG, the blood flow-limited model also fit experimental data well (R2 values were 0.9528 and 0.9947 for the blood flow-limited and the membrane-limited models, respectively). This is in agreement with experimental results of rapid blood clearance of PLGA nanoparticles compared to mPEG-containing nanoparticles. However, for all nanoparticle formulations with mPEG, the R2 values for the blood flow-limited model were much lower. This indicates that the blood to tissue distribution of nanoparticle formulations is largely membrane-limited rather than blood flow-limited.
Parameter estimation and analysis
Parameters generated from PBPK model simulation (membrane-limited) are listed in , including diffusion, tissue–blood partition, and excretion coefficients. Higher diffusion coefficients mean faster transportation rates of nanoparticles from blood into tissues, and higher excretion coefficients (urine and bile) represent faster clearance rates of nanoparticles from the kidneys and liver. Higher partition coefficients indicate nanoparticles have higher resident time within tissue than within blood, which leads to slower transportation rates from tissues back into blood circulation.
Table 4 Diffusion, partition, and clearance coefficients in the membrane-limited model
In general, all parameters were significantly different for PLGA nanoparticles compared to PEG-containing nanoparticles. For all nanoparticles, both diffusion and partition coefficients were highest in liver, kidney, and body, but lowest in lung (except PLGA-mPEG34), GI, and spleen. For PLGA nanoparticles, bile excretion was lowest and renal excretion was highest. The high renal excretion of PLGA nanoparticles is consistent with experimental data, but bile excretion is new information generated from the model. The high renal excretion of PLGA, which had the largest size among all nanoparticle formulations, indicates that size may not be the most important factor in determination of nanoparticle clearance from kidneys, for the formulation in this study. On the other hand, low bile excretion of nanoparticles indicates that liver excretion of nanoparticles may be determined by nanoparticle properties in a different pattern from that of renal excretion.
Parameters for the PEG-containing nanoparticle formulations were relatively close to each other and there were no obvious linear relationships between PEG content and individual parameters. For most parameters, values changed irregularly with increasing PEG content. This may be a result of the interplay between multiple properties. Generally, with increased PEG content, the size reduced and surface charge became less negative. The contribution of increased PEG content to the change in PBPK model parameters could be reduced (or even reversed) by the effects of reduced size and/or less negative surface charge. One interesting observation is that excretion rates from the liver and kidneys were opposite (ie, high liver excretion occurred simultaneously as low renal excretion and vice versa) for all five nanoparticle formulations, indicating that the effects of nanoparticle properties on liver excretion oppose renal excretion. For example, PLGA-mPEG153 and PLGA-mPEG61 nanoparticles had the highest liver excretion rates, but the lowest renal excretion rates. This cannot be explained by any of the three properties separately, because for all properties (size, zeta potential, NPEG), PLGA-mPEG61 nanoparticles were in the middle among the five formulations.
Simulation of experimental data
Although both the blood flow-limited model and membrane-limited model were evaluated, only simulation results using the later are shown. Simulation was compared with experimental data as shown in . Parameters, including transportation coefficients and partition coefficients, used in this simulation are shown in . There is good consistency between simulated and experimental data, indicating the parameters have been properly estimated and the model is suitable in interpreting the experimental data. The simulated curves fit the experimental data best for tissues with high nanoparticle levels (blood, liver, and body). For tissues with low nanoparticle levels (lungs, GI, kidney, and spleen), the simulated curves are less consistent with experimental data. This is because the model is less sensitive to lower nanoparticle levels.
Figure 2 Simulation of experimental data using the membrane-limited model. (A) poly(lactic-co-glycolic) acid, (B), poly(lactic-co-glycolic) acid-monomethoxypoly (ethyleneglycol)256, (C) poly(lactic-co-glycolic) acid-monomethoxypoly (ethyleneglycol)153, (D) poly(lactic-co-glycolic) acid-monomethoxypoly (ethyleneglycol)61, and (E) poly(lactic-co-glycolic) acid-monomethoxypoly (ethyleneglycol)34 nanoparticles.
Note: Dots represent experimental data and lines represent simulated mass-time curves.
Abbreviations: GI, gastrointestinal tract; ID, initial dose.

The simulated profiles show that the increased blood circulation time of PEG-containing nanoparticles primarily results from reduced liver and spleen distribution. Considering the volume of spleen (0.1 g for mice), the relative concentrations were high. For other tissues (lungs, kidneys, body, and GI), there was no significant difference between PLGA- and PEG-containing nanoparticle formulations. This is in agreement with the understanding that PEG modification of nanoparticles reduces the uptake by the reticuloendothelial system, which is primarily in the liver and spleen.Citation16
From the simulated profiles of the five nanoparticle formulations, it is obvious that most of the nanoparticles distribute into tissues within the initial minutes after administration. For PLGA nanoparticles, the major portion of distribution was complete within a few minutes, and distribution reached steady-state in less than half an hour. For PEG-containing nanoparticle formulations, the distribution reaches steady-state within half an hour in lungs, GI, and kidneys, but there is a continual increase (no steady-state) in liver, body, and spleen. The significant and slower distribution into the body compartment could be due to two reasons: first, the body compartment includes muscles and bones, having a much larger volume than other compartments; and second, macrophages in bone marrow may actively uptake nanoparticles.
Simulated bile and renal excretion is shown in . For renal excretion, there is good agreement between simulated curves and experimental results. For bile excretion, the simulation fit cannot be determined as no experimental data exists for comparison. The PLGA nanoparticles showed much higher renal excretion, but lower bile excretion than PEG-containing nanoparticles. Among the PEG-containing nanoparticle formulations, there were no relationships between PEG content and bile or renal excretion. This is consistent with the trend of the excretion coefficients as discussed above.
Figure 3 Simulation of renal (A) and bile (B) excretion for poly(lactic-co-glycolic) acid and poly (ethyleneglycol)-containing nanoparticle formulations.
Notes: For renal excretion, dots represent experimental data. Data for bile excretion from the original experimental do not exist.
Abbreviations: ID, initial dose; mPEG, monomethoxypoly (ethyleneglycol); PLGA, poly(lactic-co-glycolic) acid.

Sensitivity analysis
Sensitivity analysis results are listed in . Positive values refer to an increase in the blood AUC when the parameter increases and negative values refer to a decrease in the blood AUC when the parameter increases. The influence of parameters on all five formulations was similar, and the most influential parameters were diffusion coefficients and partition coefficients of the kidneys and body compartments. Although liver had the highest accumulation, kinetic parameters into and out of it were not the most influential.
Table 5 Sensitivity analysis of biodistribution parameters in blood area under the curve of poly(lactic-co-glycolic) acid and poly(lacticco- glycolic) acid-monomethoxypoly (ethyleneglycol) nanoparticles
Property–biodistribution relationship and prediction of PLGA-mPEG495 biodistribution
Regression-generated values of relationship constants are listed in . Although no obvious linear relationships were found between an individual property and biodistribution parameters, the multivariate regression analysis resulted in good linear relationships for most parameters (an R2 value, the coefficient of determination, close to unit indicates a good relationship). For a few parameters, including the diffusion coefficient of liver (0.6664), tissue–blood partition coefficient of GI (0.6341), and renal excretion coefficient (0.7522), the R2 values were relatively lower and the linear relationship was moderate.Citation17
Table 6 Relationship equations of nanoparticle properties and biodistribution kinetic parameters
The estimated relationships were used to calculate transportation parameters according to Equationequation (9)(9) for PLGA-mPEG495 nanoparticles. The biodistribution profiles of PLGA-mPEG495 nanoparticles were then simulated using the membrane-limited PBPK model. Predicted distribution profiles are presented in . Experimental data for PLGA-mPEG495 nanoparticles (at 3 hours for all tissues) were compared with predicted values. It was found that the predicted values for PLGA-mPEG495 were close to experimental data for all tissues ().
Figure 4 Predicted biodistribution kinetic profiles of poly(lactic-co-glycolic) acid-monomethoxypoly (ethyleneglycol) 495 nanoparticles (A) and comparison of experimental and predicted values of tissue distribution of poly(lactic-co-glycolic) acid-monomethoxypoly (ethyleneglycol) 495 nanoparticles at 3 hours (B).
Abbreviations: GI, gastrointestinal tract; ID, initial dose.

Discussion
PBPK modeling was used to analyze the biodistribution of PLGA-mPEG nanoparticles with various PEG content. Kinetic parameters were estimated and analyzed to understand the influence of particle properties (size, surface charge, and PEG content) on biodistribution parameters. Based on the optimized transportation and tissue–blood partition coefficients, the mass-time profiles in individual tissues of five nanoparticle formulations were simulated. The estimated parameters were then correlated to nanoparticle properties mathematically, and used to calculate the kinetic coefficients for PLGA-mPEG495 to predict the biodistribution. By simulating the biodistribution of five nanoparticle formulations using a PBPK model, the aim was to further understand the mechanisms of nanoparticle biodistribution and the relationships between nanoparticle properties and biodistribution. Deeper insight into these issues could be very helpful in further studies of nanoparticle applications and formulation design.
PBPK modeling has been applied to various types of nanoparticles.Citation7,Citation18–Citation20 In these previous works, blood flow-limited models were used. A blood flow-limited model assumes that the nanoparticle concentrations in blood and tissues instantly reach equilibrium, thus, the transportation rate of nanoparticles from blood into tissues is dependent solely on blood flow rates. However, such an assumption, in some cases, did not result in very satisfactory simulation of experimental data. As has been reported, the blood clearance half-life of nanoparticles of various components and properties could range from minutes to hours.Citation6 Therefore, nanoparticle distribution from blood to tissues is likely slower than most small chemical molecules, and may not be dependent on blood flow rates. To obtain satisfactory simulation of experimental data, model structures can be evaluated first for proper model selection. In this study, after evaluation of two model types, it was confirmed that a membrane-limited model should be adopted. The evaluation results also indicate that for PLGA nanoparticles, which were cleared most quickly from the blood, a blood flow-limited model will also adequately simulate the experimental data.
Apart from transportation mechanisms, the structures of the PBPK models also need to be carefully selected. There are no well-established rules for determination of PBPK model structures and it may depend on both the available data and knowledge in the general area. More complicated PBPK structures have been utilizedCitation20 with more compartments and subcompartments. However, much more data need to be collected to support the parameter estimation, and, in some cases, this may not be feasible. Evaluation of the model structure is a critical factor in all PBPK models of nanoparticles. In this study, tissues with very low levels of nanoparticle distribution are not listed as individual compartments (brain and thyroid).Citation8 This is based on the understanding that parameter estimation for tissues with very low levels of distribution may result in high error, which may mislead interpretations.
The developed model simulated the experimental data quite well for all five nanoparticle formulations. The simulated results indicated that for all five formulations, there was a very fast initial distribution phase and then later a slower distribution phase. The fast initial distribution could be due to the blood circulation carrying nanoparticles into every tissue, while the later slower phase is more likely determined by the properties of each tissue. This may explain redistribution of nanoparticles among tissues. The data shows that after the initial distribution phase, nanoparticle concentrations in some compartments such as lungs and GI continually reduced, indicating particle redistribution into other tissues such as liver, spleen, and body (containing bone marrow). For PEG-containing nanoparticle formulations, blood nanoparticle concentration remained high for many hours without further significant distribution into lungs, GI, and kidneys. This is consistent with previous studies, which show a rapid low-level distribution of PEG-modified nanoparticles initially, and then a slow decrease in levels for most tissues.Citation21
However, in vitro cellular models have shown endothelial cell uptake of PLGA nanoparticles, with various capacities in different cell types,Citation22–Citation24 with uptake half-lives much more than a few minutes or even hours.Citation25,Citation26 These contradicting findings indicate that there are tremendous differences between in vivo and in vitro nanoparticle kinetics. The complexity of in vivo behavior may not be easily represented by cellular models.
Besides systemically explaining nanoparticle biodistribution, kinetic parameters estimated by the PBPK model may provide quantitative evaluation of the effects of nanoparticle properties on their interaction with individual tissues. It was found that most of the parameters of PLGA were significantly different from those of PEG-containing nanoparticles, indicating different distribution kinetics. However, no clear trends were found in kinetic parameters with increasing PEG content among PEG-containing nanoparticles. This could be due to the influence of other properties (size, surface charge), which also changed with increased PEG content. Based on this consideration, the authors tried to correlate all three nanoparticle properties with the kinetic parameters. The multivariate regression-generated linear relationships between nanoparticle properties and biodistribution parameters provided a good fit for most parameters. For some parameters, the relationships were moderate, and the following explanations are hypothesized. First, the high inherent variability and complexity of biological systems result in uncertainty in experimental results.Citation4 Variation of experimental data directly influences the precision of parameter estimation, and further, the property–biodistribution relationships. Second, the property–biodistribution relationships are very complex. Assumption of linear relationship may be a simplification, at least for some organs. More complex, nonlinear equations might better describe the property– biodistribution relationships. However, at the present stage, there is limited knowledge to determine the patterns of property–biodistribution relationships, and an arbitrary selection of more advanced relationships may lead to misinterpretation of results. In fact, nonlinear relationships were also screened using nonlinear multivariate regression analysis (data not shown). However, the predicted biodistribution of PLGA-mPEG495 nanoparticles was not improved compared with that predicted by linear analysis.
It also needs to be noted that any models have limitations in application. The property–biodistribution relationships developed in this study were based on the specific properties for these PLGA and PLGA-mPEG nanoparticles. The relationships, very possibly, will change when other nanoparticle formulations are used. This model may be restricted from being directly applied to other nanoparticles, primarily because nanoparticle biodistribution is not determined solely by general properties such as size and surface charge, but also chemical components, such as PEG content in this study. The influence of chemical components on the in vivo performance of nanoparticles are significant, but far from well understood. This means nanoparticle formulations with different chemical components may have different biodistribution profiles, even if they have same size or surface charges. With this understanding, there may not be any universal property–biodistribution relationships available for nanoparticles of various chemical components, unless the effects of chemical components can be exclusively modeled across various materials. The same limitations of the model could be stated for numerous other nanoparticle properties not considered here, including morphology and particles outside the size range studied here. The significance of this work is that it proved the feasibility of developing such relationships, and provides the general methodologies, which, the authors believe, can be applied universally to other nanoparticle formulations.
The correlation between nanoparticle properties and biodistribution may have far-reaching significance in nanoparticle research, including evaluation, development, and design. First, such relationships would help in understanding the contributions of each property to the in vivo behaviors of nanoparticles within the body. Second, the property– biodistribution relationships can be used to predict the biodistribution of nanoparticles based on their properties. Finally, these relationships may guide design and development of new nanoparticle formulations with controlled properties to obtain specific biodistribution profiles.
Conclusion
The biodistribution profiles of PLGA nanoparticles with various PEG content were mathematically described by a PBPK model. The model simulated the experimental results of tissue concentration-time curves quite well. The model also provided insights into the kinetics of nanoparticle in vivo distribution and the influence of multiple properties. The correlation between nanoparticle properties and biodistribution parameters enabled the relatively accurate prediction of biodistribution for another nanoparticle formulation. This is the first work using PBPK modeling to interpret the effects of multiple nanoparticle properties on their biodistribution. This illustrates the significance of a PBPK modeling approach for the building of nanoparticle property-distribution relationships in vivo. The applications of this work are far-reaching including, but not limited to, nanoparticle toxicity assessment, rational carrier design for drug delivery and imaging, and data interpretation from in vivo bioimaging/biomarker systems.
Disclosure
The authors report no conflicts of interest in this work.
References
- KnaakJBDaryCCOkinoMSParameters for carbamate pesticide QSAR and PBPK/PD models for human risk assessmentRev Environ Contam Toxicol20081935321220614344
- AlexisFPridgenEMolnarLKFarokhzadOCFactors affecting the clearance and biodistribution of polymeric nanoparticlesMol Pharm20085450551518672949
- HagensWIOomenAGde JongWHCasseeFRSipsAJWhat do we (need to) know about the kinetic properties of nanoparticles in the body?Regul Toxicol Pharmacol200749321722917868963
- NestorovIWhole body pharmacokinetic modelsClin Pharmacokinet2003421088390812885263
- FouchecourtMBeliveauMKrishnanKQuantitative structure-pharmacokinetic relationship modellingSci Total Environ20012741–312513511453289
- LiMAl-JamalKTKostarelosKReinekeJPhysiologically based pharmacokinetic modeling of nanoparticlesACS Nano20104116303631720945925
- LankveldDPOomenAGKrystekPThe kinetics of the tissue distribution of silver nanoparticles of different sizesBiomaterials201031328350836120684985
- AvgoustakisKBeletsiAPanagiZEffect of copolymer composition on the physicochemical characteristics, in vitro stability, and biodistribution of PLGA-mPEG nanoparticlesInt J Pharm20032591–211512712787641
- MacCalmanLTranCLKuempelEDevelopment of a bio-mathematical model in rats to describe clearance, retention and translocation of inhaled nano particles throughout the bodyJ Phys Conf Ser20091511012028
- DaviesBMorrisTPhysiological parameters in laboratory animals and humansPharm Res1993107109310958378254
- DavdaJPJainMBatraSKGwiltPRRobinsonDHA physiologically based pharmacokinetic (PBPK) model to characterize and predict the disposition of monoclonal antibody CC49 and its single chain Fv constructsInt Immunopharmacol20088340141318279794
- EgbariaKFriedmanMAdsorption of fluorescein dyes on albumin microspheresPharm Res1992956296351608894
- SourisJSLeeCHChengSHSurface charge-mediated rapid hepatobiliary excretion of mesoporous silica nanoparticlesBiomaterials201031215564557420417962
- ChoMChoWSChoiMThe impact of size on tissue distribution and elimination by single intravenous injection of silica nanoparticlesToxicol Lett2009189317718319397964
- SarbuCOnisorCPosaMKevresanSKuhajdaKModeling and prediction (correction) of partition coefficients of bile acids and their derivatives by multivariate regression methodsTalanta200875365165718585127
- HuynhNTRogerELautramNBenoitJPPassiraniCThe rise and rise of stealth nanocarriers for cancer therapy: passive versus active targetingNanomedicine (Lond)2010591415143321128723
- BermanEMEssential Statistics for Public Managers and Policy Analysts2nd edWashington DCCQ Press2006
- LeeHALeavensTLMasonSEMonteiro-RiviereNARiviereJEComparison of quantum dot biodistribution with a blood-flow-limited physiologically based pharmacokinetic modelNano Lett20099279479919128005
- LinPChenJWChangLWComputational and ultrastructural toxicology of a nanoparticle, Quantum Dot 705, in miceEnviron Sci Technol200842166264627018767697
- PeryARBrochotCHoetPHNemmarABoisFYDevelopment of a physiologically based kinetic model for 99 m-technetium-labelled carbon nanoparticles inhaled by humansInhal Toxicol200921131099110719814607
- MosqueiraVCLegrandPMorgatJLBiodistribution of long-circulating PEG-grafted nanocapsules in mice: effects of PEG chain length and densityPharm Res200118101411141911697466
- PanyamJLabhasetwarVDynamics of endocytosis and exocytosis of poly(D,L-lactide-co-glycolide) nanoparticles in vascular smooth muscle cellsPharm Res200320221222012636159
- ZhangSLiJLykotrafitisGBaoGSureshSSize-dependent endocytosis of nanoparticlesAdv Mater20092141942419606281
- CartieraMSJohnsonKMRajendranVCaplanMJSaltzmanWMThe uptake and intracellular fate of PLGA nanoparticles in epithelial cellsBiomaterials200930142790279819232712
- ChithraniBDChanWCElucidating the mechanism of cellular uptake and removal of protein-coated gold nanoparticles of different sizes and shapesNano Lett2007761542155017465586
- SerdaREGuJBurksJKFerrariKFerrariCFerrariMQuantitative mechanics of endothelial phagocytosis of silicon microparticlesCytometry A200975975276019610127