Abstract
Purpose: The objective of the current work was to simulate radiofrequency ablation treatment in computational models with realistic human anatomy, in order to investigate the effect of realistic geometry in the treatment outcome.
Materials and methods: The body sites considered in the study were liver, lung and kidney. One numerical model for each body site was obtained from Duke, member of the IT’IS Virtual Family. A spherical tumour was embedded in each model and a single electrode was inserted into the tumour. The same excitation voltage was used in all cases to underline the differences in the resulting temperature rise, due to different anatomy at each body site investigated. The same numerical calculations were performed for a two-compartment model of the tissue geometry, as well as with the use of an analytical approximation for a single tissue compartment.
Results: Radiofrequency ablation (RFA) therapy appears efficient for tumours in liver and lung, but less efficient in kidney. Moreover, the time evolution of temperature for a realistic geometry differs from that for a two-compartment model, but even more for an infinite homogenous tissue model. However, it appears that the most critical parameters of computational models for RFA treatment planning are tissue properties rather than tissue geometry.
Conclusions: Computational simulations of realistic anatomy models show that the conventional technique of a single electrode inside the tumour volume requires a careful choice of both the excitation voltage and treatment time in order to achieve effective treatment, since the ablation zone differs considerably for various body sites.
Introduction
After the first, rather serendipitous, atrioventricular block induced by electric current [Citation1] the clinical use of radiofrequency ablation (RFA) has advanced in cardiology. However, RFA has also managed to establish itself in cancer treatment as the most widely used minimally invasive thermal therapy until now. It is employed for the treatment of hepatocellular carcinoma (HCC) [Citation2,Citation3], renal cell carcinoma (RCC) [Citation4–6], non-small cell lung cancer (NSCLC) [Citation7], and in osseous metastases for the palliation of pain [Citation8,Citation9].
Apart from clinical studies, several works have appeared in the literature on the computational modelling of RFA, in an effort to better understand the mechanism of heat transfer in human tissues and optimise treatment. These computational studies have mainly focused on the liver, investigating either a one-compartment model, where tumour and healthy tissue have the same electrical and thermal properties, or a two- or multi-compartment model, which apply different properties to healthy tissue and tumour [Citation10–12]. The approximation of the local anatomy is rather simple, even when major blood vessels are included in the model [Citation13].
A comprehensive attempt to numerically solve the non-linear partial differential equations that govern RFA treatment was made by Kröger et al. [Citation14], who used the finite element technique for a realistically vascularised model of a liver. Other groups [Citation15,Citation16] have also reported on the treatment planning of RFA in realistic anatomy models of the liver. However, in these latter works, the actual electrothermal problem is not solved numerically; computer graphics and visualisation techniques (weighted distance fields and transform) are used to approximate the necrosis zone, without considering the potential effects of local tissue properties.
In this work we employed realistic anatomy models, obtained from three different body sites of the human numerical model of Duke, member of the IT’IS Foundation Virtual Family [Citation17]. The sites considered were liver, lung and kidney, with a spherical tumour embedded into them. Moreover, background tissue and tumour properties (electrical conductivity and perfusion) were chosen in such a way as to represent clinically realistic cases of cancer. The results (e.g. ablation zone size, maximum temperature rise at the periphery of the tumour) from the anatomically detailed models were compared to those of two-compartment models with the same tissue and tumour properties, in order to determine the impact of realistic anatomy on treatment outcome, which was the first objective of the present study. The second objective was to investigate the suitability of a simplified calculation model that can be used for the solution of the thermal problem and, consequently, the estimation of the maximum expected temperature rise.
Materials and methods
Electrostatic problem
The wavelength of electromagnetic fields inside the human body is several orders of magnitude larger than the size of the ablation electrode at the frequency range of 450–550 kHz, where RFA is performed (e.g. at the frequency of 500 kHz the relative permittivity of liver is 2,770 [Citation18] and the wavelength in it is about 11 m). Therefore, a quasi-static approximation can be used to solve the electromagnetic problem, by solving the generalised Laplace equation (1)
Where σ is the electrical conductivity (S/m), and Vis the electric potential (volts). Throughout the current work we assumed that σ was independent of temperature, because the highest temperatures examined were well below 100 °C, where it has been shown [Citation19] that the differences in the resulting temperatures computed with and without temperature-dependent conductivity are approximately 5–8%. Moreover, as it can be deduced from the above equation, conduction currents were assumed to dominate compared to displacement currents, which is true for most human tissues at the frequencies used in RFA, with an error smaller than 1.5% in the calculated electric field [Citation20]. The electrostatic problem was solved numerically to obtain the electrical potential (V) distribution inside the computational domain.
Thermal problem
The thermal problem was solved by using a modified Pennes bioheat equation [Citation21]
where Qm is the metabolic heat generation, which is negligible compared to the locally dissipated energy of the electromagnetic field, ρ is mass density, c is the specific heat capacity, and k is thermal conductivity of tissue. All quantities with subscript b refer to blood and ωb is blood perfusion in the tissue measured per time unit (1/s). The above parameters of tissues were considered constant during the simulations and the liquid-to-vapour phase change was not taken into account in the model; this may lead to an overestimation of the ablation zone by up to 20% [Citation22]. The solution of the thermal problem either numerically or analytically (see below in this section) resulted in either the transient or the steady-state temperature distribution inside the computational domain, respectively.
Tissue damage model
Besides the temperature distribution, the Arrhenius model was used to estimate tissue damage as another treatment parameter. Biological damage depends on both temperature and time and can be quantified by the function of tissue injury Ω, which is defined as Equation 3.
Where T is the temperature (K) calculated locally inside the computational domain, R is the gas constant (8.314 J/mol K), A is a frequency factor (s−1) and ΔE (J/mol) is an activation energy barrier, which tissue constituents must overcome to denature. Both A and ΔE are kinetic coefficients evaluated for each tissue type from experimental data and for the three tissues of interest here these values are presented in [Citation23,Citation24]. The kinetic coefficients for lung could not be found in the literature, thus the parameters for cell death damage were used [Citation25]. Two volumes of Arrhenius damage were calculated, namely for Ω = 1 and Ω = 4.3, corresponding to 63% and 99% tissue destruction respectively.
Table 1. Kinetic coefficients for the three tissues of interest.
Numerical technique
The finite difference method (FDM), implemented with in-house developed matlab (mathworks, Natick, MA) code, was used as a numerical technique to solve the electrothermal problem with appropriate boundary conditions [Citation26]. The computational domain consisted of a cubic region with an edge of 12 cm that was obtained from three different body sites of the numerical model of Duke (adult male, age 34 years, height 1.77 m, body mass 72.4 kg, number of segmented tissues 77), member of the IT’IS Virtual Family [Citation17]. The sites considered were liver, lung and kidney (). The inserted electrode had a diameter of 0.15 cm and an active part of 2 cm (equal to the assumed tumour diameter), and was fully emerged in the tumour; it was always set at a voltage of 20 V. The grid step (voxel edge size) was 0.05 cm in the plane normal to the electrode and 0.10 cm for the z axis, along the electrode.
Figure 1. Human models with a spherical tumour embedded (indicated by the arrows) within them: (A) a simplistic model of two compartments, and three realistic anatomy models for (B) liver, (C) kidney, and (D) lungs. The active part of the electrode was placed into the tumour.
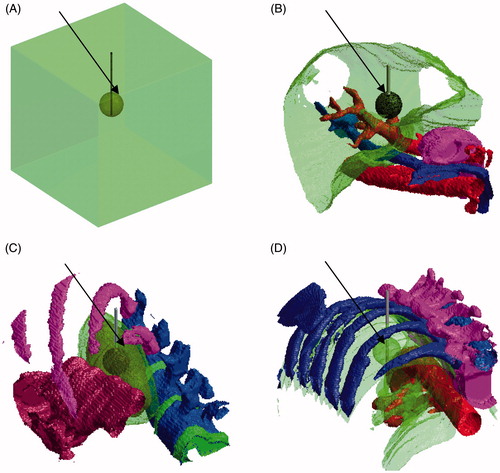
The boundaries of the computational domain were set at a Dirichlet condition with potential of 0 V (simulating the return electrode) for the electrostatic and a temperature of 37 °C for the thermal problem. Homogeneous Neumann boundary conditions (electrical and thermal insulation) were assumed for the inactive part of the electrode. At the active part of the electrode, a Dirichlet condition for the electric potential was chosen, but thermal insulation was still applied. The selection of thermal insulation along the whole electrode in the computational domain implies that no heat conduction along it was allowed, resulting in an overestimation of the temperature rise in the tissue. Moreover, this condition implies that the electrode studied was not water cooled.
The background volume electric and thermal properties (except for perfusion of cirrhotic liver) were obtained from the database of the IT’IS foundation [Citation18], and the tumour electric and thermal properties from theoretical and experimental studies [Citation27–31]. In order to approximate the most realistic cases, especially for tumour perfusion, the values were obtained from experimentally measured values, and scaling was performed to the reported tissue values, accordingly. In and the properties of background and cancerous tissues used in the simulations are presented, respectively.
Table 2. Electric and thermal properties of background tissue (460 kHz).
Table 3. Electric and thermal properties of cancerous tissue (tumour volume).
Validation of numerical technique
In order to validate the simulations performed for the complicated anatomically realistic models, a comparison with experimental results of a single electrode model [Citation19,Citation32] was made, for an agarose phantom and porcine liver in vivo. Values for agar dielectric and thermal properties were obtained from the literature [Citation33,Citation34], while porcine liver properties were assumed the same as human. More specifically, for agar the following values were used: σ = 0.15 S/m, k = 0.75 W/m/°C, and c = 4200 J/kg/°C. Finally, in the validation simulations the potential, and consequently the Dirichlet boundary condition of the electrostatic problem, at the active part of the electrode were set at the appropriate values, representing the experimental case examined. Also, the thermal boundary at the electrode was set at a constant temperature of 12 °C (not insulation, as in the actual realistic anatomies) to correspond to the water-cooled electrode used in the experimental set-up.
Analytical approximation of temperature distribution
In addition to the numerical method that can be used for the solution of the thermal problem, it is possible to calculate its Green’s function in a homogeneous tissue model of infinite dimensions (i.e. without any thermal boundaries to limit the computational domain), as proposed by Yeung and Atalar [Citation35]. Then, for this approximation of a single tissue compartment, the time for the temperature to reach a steady-state condition can be assumed as 5τ, where τ is the time constant proposed by Yeung and Atalar [Citation35] (Equation 4).
Moreover, in Yeung and Atalar [Citation35] the concept of a scaling factor (SF) is proposed (Equation 5)
which correlates average specific absorption rate (SAR) with temperature rise and is expressed in (W/kg)/°C. In the current work we compare the values of the time to reach steady-state and the scaling factor for tumour using either of the two calculation models (numerical technique and analytical approximation). The 1 g averaged SAR and the temperature rise were used to calculate the numerical SF, and for the analytical approximation the perfusion which used, was found by calculating the average perfusion within the volume comprising the averaged 1 g SAR.
Results
Validation of numerical technique
The numerical results obtained with a one-compartment model for a single water-cooled electrode inserted in an agarose phantom and in pig liver were compared with the measurements of the corresponding experimental set-ups reported by Goldberg et al. [Citation32]; this comparison is shown in .
Table 4. Comparison of numerical and experimental results for validation purposes.
The choice for the size of the cubic computational domain (edge of 12 cm) was made after a literature review [Citation12,Citation13,Citation19,Citation24] and was validated by comparing the solution for the electric field intensity (the square of which pertains directly to the SAR and the temperature rise) in computational domains of various edges (from 9 to 14 cm). In the electric field for different truncations of the cubic computational domain is presented for the same discretisation step inside the one-compartment model.
Effect of computational model and anatomy on treatment outcome
The electrostatic and thermal problems, as presented in the previous section, were solved numerically for the six models of . The parameters used to characterise treatment efficiency (assumed treatment time of 15 min) were (1) the maximum temperature achieved within the computational domain, and (2) the Arrhenius volumes for two injury fractions. These parameters are reported in for the different sites of the body (with different anatomies). The same parameters calculated with the computational model of two compartments are also given in to show the effect of inhomogeneous tissue distribution in the realistic anatomy models.
Table 5. Parameters used to characterise treatment efficiency (obtained numerically).
In order to further elucidate the differences in the resulting temperature distributions as well as the temperature evolution in different anatomies in the body, and show snapshots at various times of the temperature profiles along lines across the models. The divergence of the realistic cases from the two-compartment model is clear.
Figure 3. Profiles of the normalised temperature rise distribution along a line of the computational models of tumour tissue embedded in normal liver tissue. The line is at a distance of 0.5 cm below the electrode tip. (A) Two-compartmental liver model (maximum ΔT = 0.63 °C). (B) Realistic geometry (maximum ΔT = 2.24 °C).
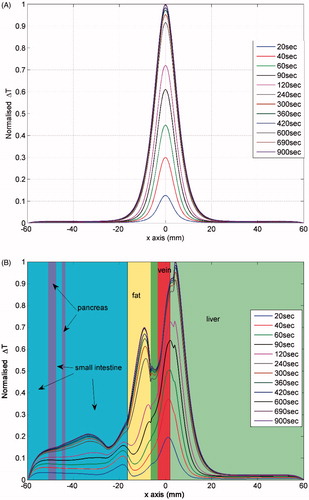
Figure 4. Profiles of the normalised temperature rise distribution along a line of the computational models of tumour embedded in normal kidney tissue. The line is at a distance of about 1 cm and parallel to the electrode. (A) Two-compartmental kidney model (maximum ΔT = 2.43 °C). (B) Realistic geometry (maximum ΔT = 2.04 °C).
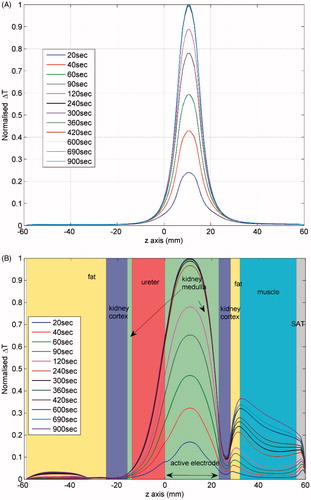
Furthermore, the temperature rise for a point at the periphery of the tumour on a plane normal to the middle of the active part of the electrode is shown in , to indicate the differences in temperature evolution for the various anatomically realistic computational models.
Suitability of calculation model
As mentioned above, a second objective of the current work was to assess the suitability of using a simple calculation model of an analytical approximation [Citation35] in the anatomically detailed computational models, for which a numerical approach is usually taken in the literature. The results of this effort are shown in .
Table 6. Numerically calculated and analytically approximated thermal results.
Discussion
Validation of numerical technique
The temperature measured experimentally at various distances from the electrode for specific times of heating (15 min and 12 min for phantom and tissue, respectively) are in excellent agreement with the numerical results (), taking into account the standard deviation of measurements and the uncertainty of the numerical calculations that can be assumed due to an error in the tissue properties values. The latter has been studied for interstitial hyperthermia in the brain, which is close to RFA in terms of application, and it was found that an error of 25% in the dielectric properties resulted in an averaged error of less than 5% in the temperature distribution [Citation37]. Moreover, it should be repeated here that this agreement was accomplished, even though the numerical technique implemented in the computational model did not consider the non-linear changes in tissue properties.
With respect to the effect of computational domain truncation on the results, it can be deduced from that the variation of the electric field intensity for various sizes of the computational domain is less than 33.47% and less than 25.83% at distances of 1.0 and 1.5 cm, respectively. These two distances have been chosen because the first represents the size of the tumour assumed in the current study, and the second is mentioned as the maximum tumour size in liver indicated for RFA treatment.
Effect of computational model and anatomy on treatment outcome
The results presented in show that the expected treatment outcome, in terms of maximum temperature rise and ablation volume, does not depend on the tissue distribution around the tumour. In all cases of the sites in the assumed body, the simplistic model of two compartments gave similar results to the anatomically detailed model, when the same tissue properties were used. The difference was less than 6% in maximum temperature achieved and less than 10% for the ablation zone (volume for Ω = 4.3). It is clear that when the tumour volume does not include a vessel that can compromise treatment, the approach taken in Villard et al. [Citation15] and Rieder et al. [Citation16] can give a reliable estimation of treatment efficiency. On the contrary, when it is necessary to plan treatment with constraints of sensitive tissues around the tumour, it is necessary to take a numerical approach, since and indicate that not only the existence of blood vessels is important for the final temperature distribution, but tissue inhomogeneity, as well.
Another important observation in and , which is better shown in , is the temporal evolution of temperature at various points. In the former two figures the effect of anatomy (especially of fat, which displays a delay in temperature rise) can be seen, whereas in the latter the effect of the properties used is more pronounced, since the point investigated lies at the tumour periphery. It is interesting to note that the maximum temperature is reached at different times for the computational models, which may have an implication for expected treatment time depending on the site of the body.
The values of the various properties necessary to solve the problem numerically play a more critical role than anatomy in the evaluation of the treatment outcome. At the same body site, a change in the perfusion and/or conductivity contrast between the targeted tumour and the surrounding tissues can result in larger variations in the treatment parameters than a change in the anatomical composition of the computational model. This result is in agreement with previous studies [Citation11] and confirms that the changes in maximum temperature reached are mainly due to differences in perfusion rates, especially in the tumours, and not of thermal conductivity (i.e. no ‘oven-effect’ [Citation38] can be expected with the used values). For instance, kidney is a highly perfused tissue compare to the other two, and thus the temperature rise achieved was lower for the same electrode voltage.
Suitability of calculation model
The results of show that both the numerical technique and the analytical approximation using the Green’s function can predict the time constant of temperature evolution closely only for the simple computational model of two compartments. The introduction of complex anatomy in the computational models leads to a delay in reaching the maximum temperature. As far as the scaling factor is concerned, it can be said that the same energy deposition in tissue in terms of 1 g averaged SAR results in a lower estimated maximum temperature rise in liver and in higher estimated temperature rise in kidney and lung compared to the numerical technique. As can be seen, the anatomy influences in a different way the scaling factor, contrary to the results of the numerical technique that show an increase from the two compartment to the anatomical model. This is an indication that the analytical approach cannot be employed for the estimation of the maximum temperature.
Conclusions
In this work the RFA treatment was modelled inside computational models of realistic human anatomy to illustrate the fact that clinicians cannot rely on the same treatment settings for different sites of the body. It was shown that tumour treatment with the conventional single electrode technique and the same voltage was not efficient in all cases. In particular, kidney tissue appears to be a location in the body where special care should be taken to achieve the desired treatment outcome. Moreover, it was clear that complex tissue anatomy either increases (in liver) or decreases (in lung and kidney) the predicted maximum temperature rise, and affects (increases) the expected treatment time, as comparisons with an infinite homogeneous tissue model have shown. On the contrary, a two-compartment model is already a good approximation in terms of anatomical geometry, but care must be taken to use realistic tissue properties in each compartment, since the estimated treatment outcome strongly depends on them.
Declaration of interest
This research has been co-financed by the European Union (European Social Fund) and Greek national funds through the Operational Program ‘Education and Lifelong Learning’ of the National Strategic Reference Framework Research Funding Program: Heracleitus II. Investing in knowledge society through the European Social Fund. The authors alone are responsible for the content and writing of the paper.
References
- Joseph JP, Rajappan K. Radiofrequency ablation of cardiac arrhythmias: Past, present and future. QJM 2012;105:303–14
- Lencioni R, Crocetti L. Radiofrequency ablation of liver cancer. Tech Vasc Interv Radiol 2007;10:38–46
- Lau WY, Lai EC. The current role of radiofrequency ablation in the management of hepatocellular carcinoma: A systematic review. Ann Surg 2009;249:20–5
- Stone MJ, Venkatesan AM, Locklin J, Pinto P, Linehan M, Wood BJ. Radiofrequency ablation of renal tumors. Tech Vasc Interv Radiol 2007;10:132–9
- Park S, Cadeddu JA. Outcomes of radiofrequency ablation for kidney cancer. Cancer Control 2007;14:205–10
- Hoffmann RT, Jakobs TF, Trumm C, Helmberger TK, Reiser MF. RFA of renal cell carcinoma in a solitary kidney. Abdom Imaging 2008;33:230–6
- Zhu JC, Yan TD, Morris DL. A systematic review of radiofrequency ablation for lung tumors. Ann Surg Oncol 2008;15:1765–74
- Hoffmann RT, Jakobs TF, Kubisch CH, Trumm CG, Weber C, Duerr HR, et al. Radiofrequency ablation in the treatment of osteoid osteoma-5-year experience. Eur J Radiol 2010;73:374–9
- Thanos L, Mylona S, Galani P, Tzavoulis D, Kalioras V, Tanteles S, Pomoni M. Radiofrequency ablation of osseous metastases for the palliation of pain. Skeletal Radiol 2008;37:189–94
- Liu Z, Lobo SM, Humphries S, Horkan C, Solazzo SA, Hines-Peralta AU, Lenkinski RE, Goldberg SN. Radiofrequency tumor ablation: Insight into improved efficacy using computer modeling. Am J Roentgenol 2005;184:1347–52
- Ahmed M, Liu Z, Humphries S, Goldberg SN. Computer modeling of the combined effects of perfusion, electrical conductivity, and thermal conductivity on tissue heating patterns in radiofrequency tumor ablation. Int J Hyperthermia 2008;24:577–88
- Chang IA. Considerations for thermal injury analysis for RF ablation devices. Open Biomed Eng J 2010;4:3–12
- Hariharan P, Chang I, Myers MR, Banerjee RK. Radio-frequency ablation in a realistic reconstructed hepatic tissue. J Biomech Eng 2006;129:354–64
- Kröger T, Altrogge I, Preusser T, Pereira PL, Schmidt D, Weihusen A, Peitgen HO. Numerical simulation of radio frequency ablation with state dependent material parameters in three space dimensions. Lect Notes Comput Sci 2006;4191:380–8
- Villard C, Soler L, Gangi A. Radiofrequency ablation of hepatic tumors: Simulation, planning, and contribution of virtual reality and haptics. Comput Methods Biomech Biomed Eng 2005;8:215–27
- Rieder C, Kröger T, Schumann C, Hahn HK. GPU-based real-time approximation of the ablation zone for radiofrequency ablation. IEEE Trans Visual Comput Graph 2011;17:1812–21
- Christ A, Kainz W, Hahn EG, Honegger K, Zefferer M, Neufeld E, et al. The Virtual Family – Development of surface-based anatomical models of two adults and two children for dosimetric simulations. Phys Med Biol 2010;55:N23–38
- Hasgall PA, Neufeld E, Gosselin MC, Klingenböck A, Kuster N. IT’IS database for thermal and electromagnetic parameters of biological tissues. www.itis.ethz.ch/database (accessed 26 September 2011)
- Chang I. Finite element analysis of hepatic radiofrequency ablation probes using temperature-dependent electrical conductivity. Biomed Eng Online 2003;2:12
- Barchanski A, De Gersem H, Gjonaj H, Weiland T. Impact of the displacement current on low-frequency electromagnetic fields computed using high-resolution anatomy models. Phys Med Biol 2005;50:N243–9
- Pennes HH. Analysis of tissue and arterial blood temperatures in the resting human forearm. J Appl Physiol 1948;1:93–122
- Abraham JP, Sparrow EM. A thermal-ablation bioheat model including liquid-to-vapor phase change, pressure- and necrosis-dependent perfusion, and moisture-dependent properties. Int J Heat Mass Transfer 2007;50:2537–44
- Chang IA, Nguyen UD. Thermal modeling of lesion growth with radiofrequency ablation devices. Biomed Eng Online 2004;3:27
- Pop M, Molckovsky A, Chin L, Kolios M, Jewett M, Sherar M. Changes in dielectric properties at 460 kHz of kidney and fat during heating: Importance for radio-frequency thermal therapy. Phys Med Biol 2003;48:2509–25
- Pearce JA. Relationship between Arrhenius models of thermal damage and the CEM43 thermal dose. Proc SPIE 7181, Energy-based Treatment of Tissue and Assessment V, 718104(February23, 2009). doi:10.1117/12.807999
- Berjano EJ. Theoretical modeling for radiofrequency ablation: state-of-the-art and challenges for the future. Biomed Eng Online 2006;5:24
- Haemmerich D, Schutt DJ, Wright AW, Webster JG, Mahvi DM. Electrical conductivity measurement of excised human metastatic liver tumors before and after thermal ablation. Physiol Meas 2009;30:459–66
- Anderson H, Yap JT, Wells P, Miller MP, Propper D, Price P, et al. Measurement of renal tumor and normal tissue perfusion using positron emission tomography in a phase II clinical trial of razoxane. Br J Cancer 2003;89:262–7
- Taylor I, Bennett R, Sherriff S. The blood supply of colorectal liver metastases. Br J Cancer 1979;39:749–56
- Ovali GY, Sakar A, Goktan C, Celik P, Yorgancioglu A, Nese N, Pabuscu Y. Thorax perfusion CT in nonsmall cell lung cancer. Comput Med Imaging Graph 2007;31:686–91
- Seegenschmiedt MH, Fessenden P, Vernon CC. Thermoradiotherapy and thermochemotherapy. Vol. 1: Biology physiology and physics. Philadelphia, Berlin: Springer Verlag, 1995
- Goldberg SN, Ahmed M, Kruskal JB, Huertas JC, Halpern EF, Oliver BS. Radio-frequency thermal ablation with NaCl solution injection: Effect of electrical conductivity on tissue heating and coagulation-phantom and porcine liver study. Radiology 2001;219:157–65
- Kao TJ, Saulnier GJ, Isaacson D, Szabo TL, Newell JC. A versatile high-permittivity phantom for EIT. IEEE Trans Biomed Eng 2008;55:2601–7
- Solazzo SA, Liu Z, Lobo SM, Ahmed M, Hines-Peralta AU, Lenkinski RE, et al. Radiofrequency ablation: Importance of background tissue electrical conductivity – An agar phantom and computer modeling study. Radiology 2005;236:495–502
- Yeung CJ, Atalar E. A Green’s function approach to local RF heating in interventional MRI. Med Phys 2001;28:826–32
- Crocetti L, de Baere T, Lencioni R. Quality improvement guidelines for radiofrequency ablation of liver tumours. Cardiovasc Intervent Radiol 2010;33:11–17
- Van de Kamer JB, Van Wieringen N, De Leeuw AAC, Lagendijk JJW. The significance of accurate dielectric tissue data for hyperthermia treatment planning. Int J Hyperthermia 2001;17:123–42
- Liu Z, Ahmed M, Weinstein Y, Yi M, Mahajan RL, Goldberg SN. Characterization of the RF ablation-induced ‘oven effect’: The importance of background tissue thermal conductivity on tissue heating. Int J Hyperthermia 2006;22:327–42