Abstract
Purpose: The purpose of our study was to assess the feasibility and reliability of 3D ultrasound-ultrasound (US-US) automatic registration-based analysis of the hepatic vessel tree (VT) (3D VT-based automatic registration) in clinical applications. Materials and methods: A total of 70 pairs of 3D ultrasound data were acquired from the livers of 10 healthy volunteers enrolled in the study. An automatic registration method was applied to the acquired volumetric data pairs, and anatomic landmarks were picked by an experienced sonographer as ‘ground truth’. The influences of respiration phase, subject posture, and liver lobe on data acquisition and scan volumetric angle on the registration accuracy and robustness were investigated. The registration time, success rate, median registration error distance, and sonographer’s subjective feedback were assessed. Results: The time required for the 3D VT-based automatic registration was approximately 15∼20 s. Overall, the success rate for the hepatic vessel-based registration was 71% (50/70), and the median registration error distance was 1.72 mm (0.57∼4.71 mm). When the influential factors were well controlled, the optimal registration accuracy (median registration error distance = 1.22 mm) could be obtained with an excellent success rate of 100% (10/10). According to the subjective assessment of the sonographer, over 90% (45/50) of the automatic registration results were not inferior to the ground truth. Among them, 42% (21/50) were superior to the fusion results from the ground truth. Conclusions: The results suggest that the 3D VT-based automatic registration is feasible and reliable and has potential for guidance and evaluation of intraoperative ablation of hepatocellular carcinoma.
Introduction
Hepatocellular carcinoma (HCC) results in a significant disease burden in China due to the high incidence of hepatitis B virus (HBV) infection. Minimally invasive procedures, such as radio-frequency ablation (RFA) and microwave ablation (MWA), have attracted much attention worldwide for HCC treatment [Citation1–5]. Research has shown that RFA can be locally curative for HCC and might represent a first-line treatment for patients with early stage HCC [Citation6]. Ultrasound plays a very important role in HCC interventional treatment. It has been extensively used for tumour localisation, real-time guidance of ablative procedures, timely treatment evaluation and follow-up. However, locating small-sized HCC, monitoring the development of tumour necrosis and precisely evaluating the ablated zone immediately after the ablation procedure is difficult with conventional B-mode ultrasound. Usually, pre-ablation computed tomography (CT) or a magnetic resonance (MR) image is fused with a real-time intraoperative ultrasound image to facilitate better localisation of the targeted regions [Citation7–12]. For treatment evaluation, contrast-enhanced computed tomography (CECT) or contrast-enhanced magnetic resonance imaging (CEMRI) is used after ablation treatment. The advantage of using multimodality images is obvious: it can provide more accurate tumour localisation and treatment evaluation. However, the additional CT/MRI scans increase the procedure cost, operation time and radiation dosage. For the efficiency of the ablation procedure, it is recommended that the hospital have a well-built picture archiving and communication system (PACS) or at least the interventional doctor should have easy access to the digital CT/MRI data of the patient. These requirements also limit the clinical application of multimodality image guidance for liver ablation treatment.
Contrast-enhanced ultrasound (CEUS) can demonstrate microcirculatory perfusion of liver lesions and greatly improves the capability of conventional ultrasound [Citation13–16]. Reports also suggest that CEUS can be used for assessing the treatment response of hepatic tumour RFA and performs almost equivalently to CECT and CEMRI [Citation17,Citation18]. With B-mode ultrasound to provide real-time RFA guidance and CEUS for pre-procedure tumour localisation and post-procedure treatment evaluation, it is now feasible to perform accurate liver tumour RFA under only ultrasound guidance, which is cost-effective, radiation-free, requires minimal CT/MRI resources, and could easily be integrated into an operation room with limited space.
Routine two-dimensional (2D) ultrasound is very operator-dependent. It requires significant user experience to maintain the imaging plane before, during and immediately after the RFA procedures to monitor and assess the treatment. Additionally, treatment evaluation based on 2D ultrasound images is sometimes inadequate and inaccurate. Recent developments in ultrasound technology that hold promise for user-independent volumetric quantification include three-dimensional (3D) ultrasound, which displays in much the same way as CT or MRI. Needless to say, scanning the index tumour with a volumetric field of view (FOV) is much easier than with a planar FOV. RFA treatment assessment with volumetric data will more precisely reveal whether the ablation zone has covered the range of the ablative margin around the index tumour.
Three-dimensional ultrasound-ultrasound image registration is very important for ultrasound-guided intervention. Fusion of the 3D US/CEUS images before, during and after ablation treatment can help the clinicians to accurately monitor and assess the therapeutic results and ultimately lead to a reduction in re-treatment rate. Efficient and reliable image fusion can greatly reduce the dependency on operator experience and increase the quality of the therapy.
In this clinical validation study, the feasibility and reliability of a hepatic vessel tree (VT)-based 3D US-US automatic fusion method was validated using volunteer data. The aim of the study is not to develop the best 3D US-US automatic fusion method, but rather to evaluate the major impact factors that could affect the image fusion using a quite mature and stable registration method. We also propose how we might control these factors in the clinical environment to achieve better fusion results.
Materials and methods
Subjects of study
Ten healthy volunteers were enrolled in the study, comprising five men and five women aged 24.7 ± 1.7 years. They had no chronic hepatitis or history of other liver diseases. Biochemical examinations showed that their hepatic functions were normal, and an ultrasonic scan of the liver revealed it to be normal in size and texture, which excluded fatty liver and liver cirrhosis. The study was approved by the institutional ethics review board. Informed consent was obtained from each participant.
Volumetric data acquired protocol
A Philips iU22 ultrasound imaging system with a 3D xMatrix probe X6-1 (central frequency 2.5–5.0 MHz) was used to acquire 3D ultrasound data (Philips, Bothell, WA, USA). To control the data quality, the 3D volumetric data were acquired by a sonographer with 6 years of ultrasound experience. The ‘abdominal vascular’ pre-set was selected, and the scan depth was set to 12–16 cm. The gain and focus were tuned so that the hepatic vessel wall was as clear as possible and the vessel lumen appeared anechogenic. The acoustic window was adjusted to avoid interference from the lungs and the gastrointestinal tract. The scan plane angle for 3D US data acquisition was set to 90 degrees to allow a wider FOV for the liver.
The principal prerequisites and requirements regarding the data acquisition of 3D VT-based automatic registration were as follows:
The range of the scan volume should cover as many hepatic vessels as possible and have optimal spatial image resolution. The bifurcations of the portal vein or hepatic vein should be visible in the cross-sectional plane, located in the middle slice of the current volume.
The image gain, penetration depth and focus were required to be properly set to produce an intravascular homogeneous anechoic status and maintain good contrast between the vessel wall (high-echo) and intravascular fluid (low-echo or anechoic) in the conventional B-mode.
The study was designed with several potential influential factors to evaluate their effects on the feasibility and reliability of 3D VT-based automatic registration. The influential factors included respiration phase (end inspiration, end expiration), subject posture (prostration, right anterior oblique), fusion liver lobe (external left liver lobe, internal lobe, anterior right liver lobe, posterior lobe) and scan volumetric angle (45°, 90°). When one factor was considered, the other potentially influential factors were kept the same during data acquisition.
Each volumetric dataset was labelled with the influential factor information and correspondingly grouped into registration pairs in the data pool as the reference image and/or floating images for further registration. From the 10 healthy volunteers, 70 pairs of 3D ultrasound data were acquired and divided into groups, including the liver lobe group (40 image pairs), the respiration group (10 image pairs), the posture group (10 image pairs) and the angle group (10 image pairs). Ten image pairs acquired from the anterior right liver lobe at end expiration were chosen as the control group. The details of the data grouping are described in .
Table 1. Influential factors and grouping.
Data processing
New 3D US-US image fusion software was developed for this registration validation study. This program integrates a DICOM 3D ultrasound data management tool, a graphical user interface component for landmark identification by sonographers, 3D VT-based ultrasound registration, fusion result visualisation, manual and automatic registration comparison and a report archive function. shows a flow diagram of the software application. The software was run on an HP EliteBook 8570w mobile workstation with 8 GB memory.
Each 3D US image pair was imported into the validation software and reviewed by an experienced sonographer. Anatomic landmarks on the reference and floating images were manually selected. At least three pairs of landmarks were chosen and used as ‘ground truth’. Manual registration was then implemented based on the landmarks, and the fusion result was reviewed by the sonographer. Once the sonographer was satisfied with the manual registration, the VT-based automatic registration was applied, and the fusion result was displayed side by side with the manual registration for assessment. The registration error distances were calculated using the least mean squared (LMS) method. For HCC radio-frequency ablation, a 5-mm safety margin was typically used. Image fusion with less than 3–5 mm error has been accepted in many clinical studies [Citation12,Citation19]. In our study, a test was considered to be successfully performed if the registration error distance was less than 5 mm.
The following data were recorded for further analysis: (1) the time taken for the 3D VT-based automatic registration, (2) the success rate of the 3D VT-based automatic registration, (3) the registration accuracy of the 3D VT-based automatic registration, including the registration error distance and the sonographer’s subjective judgement, comparing with the manual fusion used as ‘ground truth’, (4) the factors that influenced the 3D VT-based automatic registration.
The 3D VT-based automatic registration algorithm used in the study was developed by the Medisys Lab at Philips Research in Europe and relies on the following main steps: segmentation of the vessel tree, extraction of the centrelines of the vessel tree and rigid registration of the 3D ultrasound image based on the vessel tree centrelines.
Segmentation of the vessel tree: The segmentation algorithm [Citation20] of the vessel trees was intended to extract dark, elongated structures from the ultrasound data, combining tube detection, front propagation and reconstruction of the lumen in a carefully optimised, model-based design. The segmentation of the vessel tree was fully automatic, and the computation time was approximately 5 s in a multi-core central processing unit (CPU). The vessel segmentation result of the 3D ultrasonic data acquired for the anterior right liver lobe is shown in .
Extracting the centrelines of the vessel tree [Citation20]: Vessel-based registration relies on the centrelines of the segmented vessels. The skeletonisation process was very rapid and resulted in a vessel tree described as a list of 3D coordinates labelled according to their path. Working on the centrelines allows for faster computation, as pre- and post-operative volumes can be described as a set of salient features encompassing vessels paths and bifurcations. illustrates the centrelines tree of the segmentation. Bifurcations are depicted as small spheres.
Registration of the vessel tree centrelines: The registration based on vessel tree centrelines was intended to extract the 3D registration of two vessel trees at an identical hepatic segment. The rigid transformation matrix was calculated by minimising the mean squared error of the two sets of bifurcations that described the two vessel trees. The process was fully automatic, and computation time was approximately 5 s. The registration and fusion based on the vessel tree centrelines are shown in .
Statistical analysis
The statistical analysis was performed using SPSS version 11.0.1 (Chicago, IL, USA). The measurement data were tested for normality. The data were described as the mean ± standard deviation if they were normally distributed; otherwise, the results were described as the median and range. The fusion success rates among groups were compared using a chi-square test. The fusion errors were analysed using analysis of variance (ANOVA) (for normal distribution) or a rank test (for non-normal distribution). The correlations between groups were tested using Spearman’s rank correlation analysis. The statistical significance level was set at a p-value of less than 0.05.
Figure 2. (A–C) Basic 3D ultrasonic images of the anterior right liver lobe in the transverse, coronal and sagittal planes. (D) Vessel tree recognition. (E) Vessel tree segmentation and extraction.
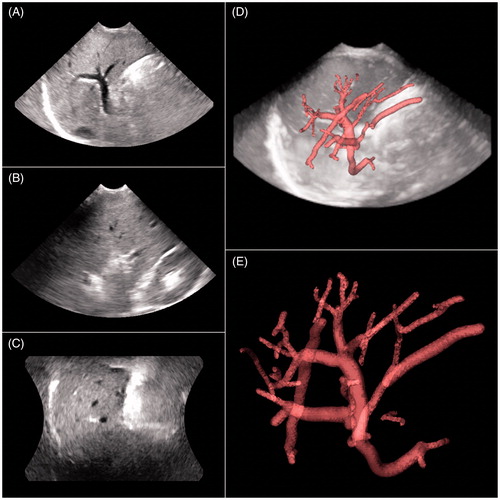
Results
General results of 3D VT-based automatic registration
Seventy pairs of 3D US volumetric images were acquired, and 50 pairs were successfully registered and fused, as shown in . The remaining 20 pairs failed, as shown in . The success rate was 71% (50/70). The time taken for the 3D VT-based automatic registration was approximately 15∼20 s. The median (range) registration error distance of 3D VT-based automatic registration was 1.72 mm (0.57∼4.71 mm).
Figure 5. (A) Reference image of the anterior right liver lobe in the transverse, sagittal and coronal planes. (B) Floating image of the anterior right liver lobe in the identical acquisition conditions as A. (C) Fusion image of A and B; the vessel trees of A and B mainly overlapped, and the registration error distance was 1.23 mm. This result indicated that the image fusion of the 3D VT-based automatic registration was successful.
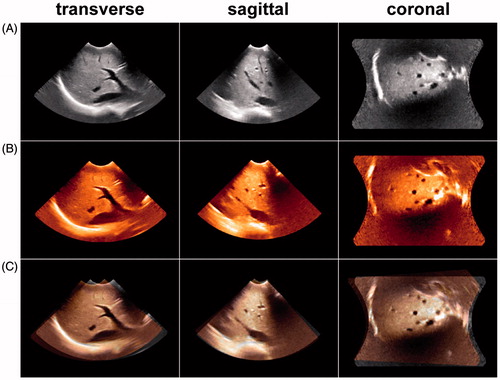
Figure 6. (A) Reference image of the anterior right liver lobe in the transverse, sagittal and coronal planes acquired at end inspiration. (B) Floating image of the anterior right liver lobe acquired at end expiration with the same acquisition conditions as in A. (C) Fusion image of A and B; the vessel trees of A and B did not overlap, and the registration error distance was 14.67 mm. This result indicated that the image fusion of the 3D VT-based automatic registration was a failure.
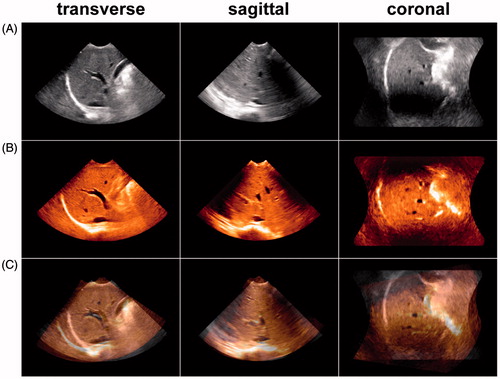
Success rate and accuracy of 3D VT-based automatic registration in different liver lobes
As shown in , the success rate of the automatic fusion was higher for the right liver lobe (100%), though the difference was not significant compared with the left liver lobe (p = 0.217). The registration error was smaller in the right liver and significantly different (p = 0.006) compared with the left liver lobe. The optimal registration accuracy (average median registration error distance of 1.22 mm) was obtained, with an excellent success rate of 100% (10/10) in the anterior right liver lobe. The right anterior segment seems the best acoustic window for data acquisition.
Table 2. The success rate and accuracy of 3D VT-based automatic registration in the liver lobe group.
Success rate and accuracy of 3D VT-based automatic registration with other influential factors
We chose the right anterior lobe group as the control group because it outperformed the other liver lobe groups. Changing one factor at a time while keeping all other influential factors the same, we acquired 3D ultrasound data at the right anterior segment. The results of the image fusion are shown in . Different breath phases, postures and scan volumetric angles affected the success rate and accuracy of the 3D VT-based automatic registration. Compared with the control group, the posture of the volunteers and the scan volumetric angle significantly decreased the success rate (40% and 30%, p = 0.011 and 0.003), and the posture of the volunteers significantly increased the registration error distance (3.01 mm, p = 0.002).
Table 3. The success rate and accuracy of 3D VT-based automatic registration with other influential factors.
The sonographer’s subjective judgment of the 3D VT-based automatic registration
With visual inspection by the sonographer of the registration results of the 3D VT-based automatic registration and the fusion image based on ‘ground truth’, over 90% (45/50) of the 3D VT-based automatic registration results were not inferior to the ‘ground truth’. Among them, 42% (21/50) were better than the ‘ground truth’, 48% (24/50) were similar to the ‘ground truth’; and the remaining 10% (5/50) were worse than the ‘ground truth’.
Discussion
3D ultrasound, as a cheap and radiation-free imaging modality, has the potential to be used to guide and monitor the RFA treatment of liver cancer. However, reliable and accurate treatment guidance and evaluation require fusion of ultrasound images before, during and after the RFA procedure. In this study, we assessed whether hepatic vessel tree-based automatic registration could be used to fulfil this clinical need and how to achieve the optimal image fusion.
Our previous study [Citation12] on US-CT/MR image fusion using a commercial navigation system suggested that manual registration of the US-CT/MR image requires 360∼720 s, even for an experienced interventional ultrasound doctor. The time required for the 3D VT-based automatic registration was only 15∼20 s, which is more clinically acceptable. The success rate of the 3D VT-based automatic registration was not inferior to US-CT/MR image fusion (81.6%) [Citation12]. The success rate was very high in the right liver lobe (100%) but lower in the left liver lobe (80%). These findings are consistent with our clinical knowledge that the right liver lobe has a better acoustic window for acquiring 3D volumetric data as well as more bifurcated vessels. 3D volumetric data acquisition in the left liver lobe is easily interfered with by the lung, heart movement and xiphoid. These factors made the VT-based registration even harder if there were not enough vessel bifurcations extracted at the end expiration in the left liver.
The accuracy of image registration is of great clinical importance, yet its clinical validation is very difficult, predominantly because of the lack of an absolute ‘ground truth’. Typically, registration accuracy is measured by referencing other registration methods or simulation data in which the ground truth is known [Citation21–23]. In our clinical volunteer study neither visual inspection nor anatomical landmark-based validation methods could provide an absolute quantitative measure of the automatic registration accuracy. Thus, the manually selected landmark-based automatic registration had to be used as the ‘ground truth’. Our results showed that the median registration error distance of the 3D VT-based automatic registration was 1.72 mm, which was similar to the successful registration standard reported in the literature [Citation21]. According to the subjective feedback of the sonographer, over 90% (45/50) of the automatic registrations were not inferior to the ‘ground truth’, and 42% (21/50) of them were superior. When the influential factors were controlled (the anterior right liver lobe, end expiration, prostration and a 90° volumetric angle were used as the acquisition conditions in the control group), optimal registration accuracy (a mean registration error distance of 1.22 mm) was achieved, with an excellent success rate of 100% (10/10). These results indicate that the 3D VT-based automatic registration technique would be feasible and reliable in intraoperative ablation of HCC.
Several influential factors (respiration phase, subject posture and volumetric scan angle) were further investigated. When 3D ultrasonic images of the same liver lobe taken at different respiration phases were automatically fused, our results showed that the success rate and registration accuracy of 3D VT-based automatic registration decreased; however, the difference was not significant compared with the control group (70% versus 100%, p = 0.211; 2.40 versus 1.22 mm, p = 0.055, respectively). Differences in scan posture and scan volumetric angle had the strongest influences on the success rate. When the subject’s posture changed, the liver shape and vessel trees were deformed, which affected the success rate, and the registration accuracy significantly decreased (40%, 3.01 mm). In addition, when the scan volumetric angle was changed from 90° to 45°, the success rate also decreased considerably (to 30%). The reason for this result is that the view of the vessel tree was occluded when the scan was acquired at a 45° volumetric angle. In clinical practice, scan posture and volumetric angle are two factors that can be easily controlled. Thus, this should not be regarded as a major limitation of VT-based registration.
There were limitations in this study. First, the use of healthy volunteers negated the possibility of estimating target registration error because no target tumours appeared in the volunteer data. A small registration error distance does not guarantee good tumour registration performance, as the landmarks used to assess the image registration might not be located at sites of interest. Liver movement and deformation as well as the distance from the target tumour to the vessel tree can all contribute to the overall tumour registration performance. We are now collecting liver cancer patient data to further evaluate our proposed solution. Second, a comparison with other registration methods was not performed. Third, vessel tree extraction was a critical module in the 3D VT-based automatic registration, and it heavily relied on the contrast between the vessel wall (high-echo) and the intravascular fluid (low-echo) in the conventional B-mode. The performance of this module could be affected in patients with fatty liver or liver cirrhosis because of blurred vessel trees. Further experimental phantom studies with built-in cross-wire targets and mimicked vessel trees are being performed to assess the target registration errors of the VT-based registration. Patients with liver diseases will also be recruited for the next stage of the study. Fourth, the sample size for each influential factor group was small (N = 10) in this preliminary study, which could impair the credibility of the statistical conclusions. However, the results clearly indicated which of the influential factors most strongly affected the success rate and accuracy of the image registration. Designing a clinical study with a larger amount of patients will be an important next step.
Conclusions
The results from this volunteer study show that 3D VT-based automatic registration is fast and accurate. The success rate is acceptable if influential factors (particularly scan posture and volumetric angle) can be controlled. This method has the potential to be used for the guidance and evaluation of intraoperative ablation of HCC.
Declaration of interest
The study was supported by funds from the National Natural Science Foundation of China (numbers 81271669, 81301931 and 81430038). The authors report no conflicts of interest. The authors alone are responsible for the content and writing of the paper.
References
- Qi X, Tang Y, An D, Bai M, Shi X, Wang J, et al. radiofrequency ablation versus hepatic resection for small hepatocellular carcinoma: A meta-analysis of randomized controlled trials. J Clin Gastroenterol 2014;48:450–7
- Lee DH, Lee JM, Lee JY, Kim SH, Yoon JH, Kim YJ, et al. Radiofrequency ablation of hepatocellular carcinoma as first-line treatment: Long-term results and prognostic factors in 162 patients with cirrhosis. Radiology 2014;270:900–9
- Andreano A, Galimberti S, Franza E, Knavel EM, Sironi S, Lee FT, et al. Percutaneous microwave ablation of hepatic tumors: Prospective evaluation of postablation syndrome and postprocedural pain. J Vasc Interv Radiol 2014;25:97–105
- Yu XL, Liu FY, Liang P, Era AD, Cheng ZG, Han ZY. Microwave ablation assisted by a computerized tomography–ultrasonography fusion imaging system for liver lesions: An ex vivo experimental study. Int J Hyperthermia 2011;27:172–9
- Huang H, Liang P, Yu XL, Cheng ZG, Han ZY, Yu J, et al. Safety assessment and therapeutic efficacy of percutaneous microwave ablation therapy combined with percutaneous ethanol injection for hepatocellular carcinoma adjacent to the gallbladder. Int J Hyperthermia 2015;31:40–7
- Shiina S, Tateishi R, Arano T, Uchino K, Enooku K, Nakagawa H, et al. Radiofrequency ablation for hepatocellular carcinoma: 10-year outcome and prognostic factors. Am J Gastroenterol 2012;107:569–77
- Crocetti L, Lencioni R, Debeni S, See TC, Pina CD, Bartolozzi C. Target liver lesions for radiofrequency ablation: An experimental feasibility study using CT–US fusion system. Invest Radiol 2008;43:33–9
- Lee MW, Rhim H, Cha DI, Kim YJ, Choi D, Kim YS, et al. Percutaneous radiofrequency ablation of hepatocellular carcinoma: Fusion imaging guidance for management of lesions with poor conspicuity at conventional sonography. Am J Roentgenol 2012;198:1438–44
- Liu FY, Yu XL, Liang P, Cheng ZG, Han ZY, Dong BW, et al. Microwave ablation assisted by a real-time virtual navigation system for hepatocellular carcinoma undetectable by conventional ultrasonography. Eur J Radiol 2012;81:1455–9
- Song KD, Lee MW, Rhim H, Cha DI, Chong Y, Lim HK. Fusion imaging-guided radiofrequency ablation for hepatocellular carcinomas not visible on conventional ultrasound. Am J Roentgenol 2013;201:1141–7
- Min JH, Lee MW, Rhim H, Cha DI, Lim S, Choi SY, et al. Local tumor progression after loco-regional therapy of hepatocellular carcinomas: Value of fusion imaging-guided radiofrequency ablation. Clin Radiol 2014;69:286–93
- Zhong-Zhen S, Kai L, Rong-Qin Z, Er-Jiao X, Ting Z, Ao-Hua Z, et al. A feasibility study for determining ablative margin with 3D-CEUS-CT/MR image fusion after radiofrequency ablation of hepatocellular carcinoma. Ultraschall Med 2012;33:E250–5
- Liu F, Yu X, Liang P, Cheng Z, Han Z, Dong B. Contrast-enhanced ultrasound-guided microwave ablation for hepatocellular carcinoma inconspicuous on conventional ultrasound. Int J Hyperthermia 2011;27:555–62
- Claudon M, Dietrich CF, Choi BI, Cosgrove DO, Kudo M, Nolsoe CP, et al. Guidelines and good clinical practice recommendations for contrast enhanced ultrasound (CEUS) in the liver – update 2012. Ultrasound Med Biol 2013;39:187–210
- Solbiati L, Lerace T, Tonolini M, Cova L. Guidance and monitoring of radiofrequency liver tumor ablation with contrast-enhanced ultrasound. Eur J Radiol 2004;51:S19–23
- Jung EM, Schreyer AG, Schacherer D, Menzel C, Farkas S, Loss M, et al. New real-time image fusion technique for characterization of tumor vascularization and tumor perfusion of liver tumors with contrast-enhanced ultrasound, spiral CT or MRI: First results. Clin Hemorheol Microcirc 2009;43:57–69
- Choi D, Lim HK, Lee WJ, Kim SH, Kim YH, Kim SH, et al. Early assessment of the therapeutic response to radio frequency ablation for hepatocellular carcinoma: Utility of gray scale harmonic ultrasonography with a microbubble contrast agent. J Ultrasound Med 2003;22:1163–72
- Bo XW, Xu HX, Sun LP, Zheng SG, Guo LH, Liu F, et al. Bipolar radiofrequency ablation for liver tumors: Comparison of contrast-enhanced ultrasound with contrast-enhanced MRI/CT in the post treatment imaging evaluation. Int J Clin Exp Pathol 2014;7:6108–16
- Lange T, Eulenstein S, Hünerbein M, Schlag PM. Vessel-based non-rigid registration of MR/CT and 3D ultrasound for navigation in liver surgery. Comput Aided Surg 2003;8:228–40
- Pizaine G, Angelini E, Bloch I Makram-Ebeid S. Implicit medial representation for vessel segmentation, Proc SPIE Medical Imaging: Image Processing 2011;7962:79623Q
- Appelbaum L, Solbiati L, Sosna J, Nissenbaum Y, Greenbaum N, Goldberg SN. Evaluation of an electromagnetic image-fusion navigation system for biopsy of small lesions: Assessment of accuracy in an in vivo swine model. Acad Radiol 2013;20:209–17
- Mäkelä T, Clarysse P, Sipilä O, Pauna N, Pham QC, Katila T, et al. A review of cardiac image registration methods. IEEE Trans Med Imaging 2002;21:1011–21
- Zhe L, Deng D, Guang-Zhi W. Accuracy validation for medical image registration algorithms: A review. Chin Med Sci J 2012;27:176–81