Abstract
Purpose. Dynamic contrast enhanced (DCE) imaging has gained interest as an imaging modality for assessment of tumor characteristics and response to cancer treatment. However, for DCE-magnetic resonance imaging (MRI) tissue contrast enhancement may vary depending on imaging sequence and temporal resolution. The aim of this study is to compare DCE-MRI to DCE-computed tomography (DCE-CT) as the gold standard. Material and methods. Thirteen patients with advanced cervical cancer were scanned once prior to chemo-radiation and during chemo-radiation with DCE-CT and -MRI in immediate succession. A total of 22 paired DCE-CT and -MRI scans were acquired for comparison. Kinetic modeling using the extended Tofts model was applied to both image series. Furthermore the similarity of the spatial distribution was evaluated using a Γ analysis. The correlation between the two imaging techniques was evaluated using Pearson's correlation and the parameter means were compared using a Student's t-test (p < 0.05). Results. A significant positive correlation between DCE-CT and -MRI was found for all kinetic parameters. The results showing the best correlation with the DCE-CT-derived parameters were obtained using a population-based input function for MRI. The median Pearson's correlations were: volume transfer constant Ktrans (r = 0.9), flux rate constant kep (r = 0.77), extracellular volume fraction ve (r = 0.58) and blood plasma volume fraction vp (r = 0.83). All quantitative parameters were found to be significantly different as estimated by DCE-CT and -MRI. The Γ analysis in normalized maps revealed that 45% of the voxels failed to find a voxel with the corresponding value allowing for an uncertainty of 3 mm in position and 3% in value (Γ3,3). By reducing the criteria, the Γ-failure rates were: Γ3,5 (37% failure), Γ3,10 (26% failure) and at Γ3,15 (19% failure). Conclusion. Good to excellent correlations but significant bias was found between DCE-CT and -MRI. Both the Pearson's correlation and the Γ analysis proved that the spatial information was similar when analyzing the two sets of DCE data using the extended Tofts model. Improvement of input function sampling is needed to improve kinetic quantification using DCE-MRI.
Perfusion imaging in locally advanced cervical cancer has the potential to be an early indicator of treatment response in combined radio-chemotherapy [Citation1–4]. Numerous preclinical studies have also highlighted the potential of perfusion imaging to obtain information about the microenvironment of tumor tissue [Citation5–9]. Dynamic Contrast Enhanced Magnetic Resonance Imaging (DCE-MRI) has so far been the preferred technique to DCE-computed tomography (CT) as it allows for combined morphological and contrast imaging with superior soft tissue contrast. However, there are inherent problems with signal-to-contrast conversion for DCE-MRI where DCE-CT has the advantage of a linear relation between signal intensity and contrast concentration. The contrast kinetics for standard Gadolinium-based MRI and iodinated CT contrast media are similar and theoretically the methods should therefore give comparable results. The quantification of tissue specific flow parameters is highly influenced by the initial in-flux of contrast agent to the tissue of interest. For MRI, there is a non-linear relation between the magnitude part of the MRI image and contrast concentration. This non-linearity is most pronounced at higher contrast concentration and thus mostly influences the sampling of the initial peak of the AIF and effects the signal enhancement of the tumor to a lesser extent. Furthermore, B1-field inhomogeneities [Citation10,Citation11], T2* effects at higher concentration [Citation12] and inflow effects [Citation10,Citation11,Citation13,Citation14] also contribute with errors to the sampling of the AIF for the magnitude images.
A number of parameters may be of importance for quantitative comparison; AIF, temporal resolution, injection time/rate. The work by Yang et al. [Citation15] in the cervix showed significant difference between corresponding model parameters as estimated by DCE-CT and -MRI. However, they remark that in their case the low temporal resolution of the DCE-MRI (8 s) may explain the differences found. The AIFs used by Yang et al. included exam specific input function, population-based [Citation16], and the iterative Mutual Reference Tissue Method [Citation17]. The work by Korporaal et al. [Citation18] in the prostate showed no significant difference between produced parameter estimates for the two techniques. Their AIF was based on phase images which have a linear relation between contrast concentration and the measured evolution of phase [Citation19,Citation20].
The aim of this work was to compare quantitative kinetic parameters for DCE-CT and -MRI in terms of quantification and spatial distribution for advanced cervical cancer.
Material and methods
Patients
This prospective study was approved by the local research ethics committee, and all patients signed a written informed consent. A total of 13 patients with locally advanced cervical cancer were enrolled from February 2011 until December 2012. The median patient age was 50 years (22–73) at the start of treatment. FIGO staging for the patients were (IIA) 1/13, (IIB) 7/13, (IIIB) 4/13, and (IVA) 1/13. One patient had adenosquamous cell carcinoma, three had adenocarcinoma and nine had squamous cell carcinoma. Seven patients were lymph node negative while one patient had paraaortic lymph node involvement and six had regional lymph node involvement.
Patients were treated with whole pelvic EBRT of 45 Gy in 25 fractions with concomitant weekly cisplatin and two MRI-guided pulsed dose rate (PDR) brachytherapy treatments with a dose of 17.5 Gy per fraction with one week between treatments [Citation21–23].
Imaging
Twenty-two scans were obtained in 13 patients, with nine patients being scanned both pre-EBRT and at two weeks, and two and two patients having only pre-EBRT or two-weeks scan, respectively. DCE-CT and -MRI were performed on the same day before start of treatment and two weeks after the start of radiotherapy. DCE-MRI was performed using a 3T Philips Achieva scanner and a three-dimensional (3D) saturation recovery spoiled gradient echo technique with 20–24 slices having a 5mm slice spacing, TR/TE of 2.9/1.4 ms, Flip Angle (FA) of 10°, in-plane resolution 2.3 mm and 2.1 s time resolution. The bolus injected was 0.1 mmol/kg Dotarem at 4 ml/s, followed by a 50 ml saline flush. A total of 120 dynamic scans were obtained. A T1 map was constructed before contrast injection and using a 3D gradient recalled echo sequence with five different FA scans (5°, 10°, 15°, 20°, 25°) with the same orientation and field of view as the dynamic scan. DCE-CT was performed using a Philips ICT 256 (Best, Netherland) scanner with collimation 128 × 0.625 mm, 100 kV, 100 mAs, axial scan mode, reconstruction 16 × 5 mm, rotation time 0.4 s, cycle time 2.00 s, FOV 350 mm, reconstruction filter B (soft), CTDIvol 3.9 mGy. The injection protocol for DCE- CT was 60 ml Visipaque (270 mg iodine/ml) at 6 ml/s. Forty-two scans with time resolution of 2.1 s for the first 30 time points and 15 s for the remaining 12 time points were obtained.
Data analysis
The T1 relaxation map was determined using a multi-flip angle approach as described in the article by Brookes et al. [Citation24]. Knowing the T1 map, a contrast concentration map can be calculated from the Solomon-Bloembergen equation [Citation25]. Assuming a contrast agent relaxivity (r1) of 3.6 s−1 mM−1 [Citation26] and the saturation time was Tsat=50 ms. Furthermore, it was assumed that TE<< T2* and using the fact that the 3D saturation recovery spoiled gradient echo is a centric sequence, the concentration of Gd is:
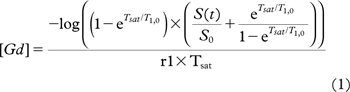
where log is the natural logarithm and T1,0 is the spin-lattice relaxation prior to contrast injection. Due to flow-related artifacts in the external iliac artery, a reference value of T1,0 = 1660 ms was chosen for blood [Citation27].
The CT images were smoothened by a 3D moving average kernel of 0.19 cm3 according to Korporaal et al. [Citation28]. The MRI images were smoothed similarly to avoid any bias in the subsequent modeling steps. The first and last MRI slices were not included in the following kinetic processing.
The contrast enhancement curves can be mathematically fitted allowing for quantitative analysis of flow, leakage and plasma volume. In the regime where the tumor is highly perfused and highly vascularized, an appropriate model is required: The extended Tofts model [Citation29].

Here vp is the plasma fraction, Cp(t) is the concentration of contrast agent in the plasma also known as the input function. The Ktrans is the transport constant from the artery into the interstitium and kep is the transport constant from interstitium back into the capillary. The implementation of the extended Tofts model was done linearly as described by Murase et al. [Citation30]. Cp(t) was obtained from the measured AIF by scaling by (1-r·Hct)/(1-Hct) where r is the vessel ratio and Hct is the hematocrit value [Citation31]. Evaluation of the kinetic fits was performed using the reduced Pearson's χ2/df, where df is the degrees of freedom and χ2 is the sum square difference between observed and the fit divided by the observed data. Fit with a χ2/df >0.5 was omitted from the results. Examples of these fits are shown in Supplementary Figure 2 to be found online at http://informahealth care.com/doi/abs/10.3109/0284186X.2013.813637.
The arterial input functions were determined individually for each patient and each scan before smoothing. The arteries were delineated such that they were not influenced by flow disturbances near the iliac bifurcation. The hematocrit value was chosen to be 0.38 and the vessel ratio 0.7 [Citation15,Citation32]. A region around the left and right external illiac arteries was chosen. By clustering (k-means) by the time-to-peak (TTP), full width half max (FWHM) of the first peak and peak height the non-relevant voxel were excluded, thus improving the choice of voxels for the AIF [Citation33]. Furthermore for the MRI, the individual time-curves were inspected to exclude possible curves that were affected by partial volume effects [Citation34].
If the uncertainty in the measured AIF exceeds 15% the use of population-based input function is considered relevant [Citation35]. For the MRI the population-based AIF published by Parker et al. [Citation16] was therefore also employed.
Finally, the individual AIF from CT was scaled to have the same injected concentration as the corresponding MRI AIF. The conversion from HU to mM was measured to be 19 HU/mM, corresponding well with values reported by Miles et al. [Citation36] and Takanami et al. [Citation37].
The interpatient variation of the extracted AIFs for CT and magnitude MRI was evaluated using the mean, standard deviation (SD) and the coefficient of variation (CV), for the peak height, FWHM of the first peak and the concentration 180 seconds after the peak (SI180).
Delineation of the gross tumor volume (GTV) was performed on a T2w para-tranverse scan. Two rigid registrations were performed with T2 and the first DCE-CT timepoint and T2 with the first DCE-MRI timepoint. Following the transfer of the GTV to the first timepoint of the DCE-CT and -MRI scan. This rigid registration was focused on the soft tissue around the GTV and thus disregarding discrepancies in alignment of the bony anatomy. The registration and delineation was performed by an experienced oncologist.
Comparison of the parameters estimated by CT and MRI was done using the Pearson's correlation, Student's t-test and Bland-Altman plots. P-value < 0.05 was considered as being statistically significant. Direct voxel by voxel comparison would be hampered by registration errors and deformation of the tumor between the CT and MRI acquisition. Therefore a gamma (Γ) analysis was applied [Citation38] which was preferred instead of deformable registration because of the large difference in soft tissue contrast between the CT and MRI. To account for any systematical offset between the two techniques, the MRI estimated parameters were normalized to the median of the CT parameter estimates for the gamma analysis. The gamma values tested were 3% difference within 3 mm (Γ3,3), 5% difference within 3 mm (Γ3,5), 10% difference within 3 mm (Γ3,10), and 15% difference within 3 mm (Γ3,15). If the Γ-value is larger than one, the voxel fail the set criteria, and if the Γ-value is equal to zero there is a perfect correspondence between the voxel on CT and MRI.
Results
The pre-EBRT was obtained with a median of four days ranging from 1 to 11 days before start of external beam radiotherapy. The two-week scans were obtained with a median of 17 days ranging from 7 to 28 days after start of external beam radiotherapy. The DCE-CT scan was performed prior to the DCE-MRI. The median time between contrast injection on MRI and CT was 112 (52–234) minutes. The median time between T2w (used for delineation) and DCE-MRI was 34 (23–48) minutes. Two scans were excluded due to initialization of scan too late after contrast injection or CT scanner breakdown. Two more were excluded due to large pelvic motion. Finally, two more CT scans were excluded due to poor SNR. Thus leaving a total of 16 scans included in the analysis with eight scans at pre-EBRT and eight at two-weeks.
An example of the DCE-CT and DCE-MRI kinetic parameter maps is shown in . A clear spatial correspondence between the two techniques can be identified. An example of quantitative difference between parameters identified by DCE-CT and -MRI using the Parker AIF can be seen from the Bland-Altman plots in . From these and the data summarized in it is seen that there is significant bias between all of the kinetic parameters estimated by the two techniques. Despite the bias a significant correlation between the parameter estimates of the two techniques was present ( and ). The kinetic parameters calculated using the population AIF correlated best with the CT estimated parameters. The parameters estimated with the Parker AIF were significantly closer to CT estimates for Ktrans,kep and vp than the parameters estimated by the magnitude AIF. Furthermore, the parameters estimated with the Parker AIF were significantly closer to CT estimates for ve and vp than the parameters estimated by the CT AIF, but not for Ktrans and kep (Student's t-test). The interpatient variation in AIF extraction is summarized in . The CT AIF and magnitude AIF for the different exams can be found in Supplementary Figure 1 to be found online at http://informahealthcare.com/doi/abs/10.3109/0284186X.2013.813637. Interpatient variation was larger for MR AIFs. Variation of peak height, FWHM and SI180 ranged from 21% to 38% for CT and 21% to 46% for MRI AIF.
Figure 1. Parametric maps estimated by DCE-CT (top row) and the corresponding parametric maps estimated by DCE-MRI (bottom row) using the population-based AIF by Parker et al. [Citation16].
![Figure 1. Parametric maps estimated by DCE-CT (top row) and the corresponding parametric maps estimated by DCE-MRI (bottom row) using the population-based AIF by Parker et al. [Citation16].](/cms/asset/a67a3cdd-4ec2-492f-8647-d42c08f3c747/ionc_a_813637_f0001_b.jpg)
Figure 2. Bland-Altman plots (left) and corresponding scatter plots (right) for Ktrans and ve measured by CT and MRI (using the Parker AIF [Citation16]). The mean voxelwise differences, prediction interval and confidence interval is denoted in bold solid black, solid black and gray line, respectively.
![Figure 2. Bland-Altman plots (left) and corresponding scatter plots (right) for Ktrans and ve measured by CT and MRI (using the Parker AIF [Citation16]). The mean voxelwise differences, prediction interval and confidence interval is denoted in bold solid black, solid black and gray line, respectively.](/cms/asset/77ff8435-cf5d-4d07-adc7-e4860d15ac10/ionc_a_813637_f0002_b.gif)
Table I. Comparison of DCE-CT and -MRI parameter estimates. (*) significant correlation.
Table II. Interpatient variation of AIF observables.
A comparison to other published values along with a selection of scan specific parameters is summarized in Supplementary Table I to be found online at http://informahealthcare.com/doi/abs/10.3109/ 0284186X.2013.813637. As the majority of publications [Citation15, Citation3, Citation39] include evaluation of DCE images prior to chemo-radiation, the two-week scans were excluded for this comparison. Similar to the data summarized in the lower part of Supplementary Table I to be found online at http://informahealth care.com/doi/abs/10.3109/0284186X.2013.813637 the MR kinetic parameters were calculated using the CT AIF and the Magnitude AIF for the pretreatment DCE-MRI data. The pretreatment values using the CT AIF were 0.35 ± 0.16 min−1 (Ktrans), 0.69 ± 0.27 min−1 (kep), 0.52 ± 0.09 (ve) and 0.05 ± 0.03 (vp). Similarly, the pretreatment values using the magnitude AIF were 0.33 ± 0.18 min−1 (Ktrans), 0.85 ± 0.28 min−1 (kep), 0.41 ± 0.05 (ve) and 0.04 ± 0.04 (vp).
The gamma analysis takes both uncertainty in position and value into account in a voxelwise comparison. Example of the data analysis is shown in . The analysis for the whole population of scans using the MRI kinetic parameters estimated with the Parker AIF is shown in showing that almost all voxels fail the Γ3,3 criteria (45% failure) and more voxels passes the Γ3,5 criteria (37% failure). As the criteria is further decreased, more voxels pass, giving 26% failure at the Γ3,10 criteria and 19% failure at the Γ3,15 criteria.
Figure 3. Example of Γ-analysis. A Γ-value > 1 fails the set criteria while Γ-value = 0 corresponds to perfect agreement between the two measures (A) shows the Γ-value using the criteria of 3 mm distance to agreement and 5% uncertainty (B) Γ-value using the criteria of 3 mm distance to agreement and 10% uncertainty. (C) Γ-value using the criteria of 3 mm distance to agreement and 15% uncertainty. (D) Ktrans map estimated using DCE-CT. (E) Ktrans map estimated using DCE-MRI and the Parker et al. AIF [Citation16]. (F) Γ-failure rates for the different tested criteria.
![Figure 3. Example of Γ-analysis. A Γ-value > 1 fails the set criteria while Γ-value = 0 corresponds to perfect agreement between the two measures (A) shows the Γ-value using the criteria of 3 mm distance to agreement and 5% uncertainty (B) Γ-value using the criteria of 3 mm distance to agreement and 10% uncertainty. (C) Γ-value using the criteria of 3 mm distance to agreement and 15% uncertainty. (D) Ktrans map estimated using DCE-CT. (E) Ktrans map estimated using DCE-MRI and the Parker et al. AIF [Citation16]. (F) Γ-failure rates for the different tested criteria.](/cms/asset/aa8bd51d-461c-4010-a6e2-f9df7fe4546a/ionc_a_813637_f0003_b.jpg)
Figure 4. Box-plots for Γ-analysis comparing parametric maps derived using DCE-CT and DCE-MRI with the Parker AIF [Citation16]. The central mark is the median, the edges of the box are the 25th and 75th percentiles, and the range of the whiskers includes 99.3 percentiles of the data. ‘+’ denotes the outliers.
![Figure 4. Box-plots for Γ-analysis comparing parametric maps derived using DCE-CT and DCE-MRI with the Parker AIF [Citation16]. The central mark is the median, the edges of the box are the 25th and 75th percentiles, and the range of the whiskers includes 99.3 percentiles of the data. ‘+’ denotes the outliers.](/cms/asset/7142a432-bed6-4400-b000-c80d1b6d1c85/ionc_a_813637_f0004_b.gif)
Discussion
DCE-CT and -MRI showed significant positive correlation with all kinetic parameters when using all tested AIFs. The quantitative parameter estimates had a high degree of variation depending on the choice of input function for DCE-MRI. The tested AIF that gave the closest quantitative agreement between CT and MRI estimated parameters was the use of the population-based AIF published by Parker et al. [Citation16]. Due to the inverse relation between Ktrans with Cp and vp with Cp it could be inferred that the measured exam AIF concentration was lower than the actual AIF when using the CT estimates as gold standard. The reason why the signal in the iliac artery was too low can most likely be attributed to the shortening of T2* during high concentration of contrast agent. This could possibly be overcome by using the phase images to estimate the exam specific AIF as proposed by Akbudak et al. [Citation19] and successfully implemented by Korporaal et al. in prostate [Citation18]. Another possibility could be simultaneous measurement of T1 and T2* during DCE-MRI imaging as proposed by Bazelaire et al. [Citation12].
The conversion of the CT AIF to MRI concentration should remove the uncertainty of the AIF shape at high concentrations. The remaining difference may be ascribed to the use of contrast agents of different molecular weight. The molecular weight of Dotarem is around one third of Visipaque (Supplementary Table I to be found online at http://informahealthcare.com/doi/abs/10.3109/0284186X.2013.813637). Small molecular (< 1 kDa) and intermediate molecular (1–60 kDa) contrast agents are both extravascular-extracellular contrast agents but the intermediate has a slower extracellular distribution [Citation40]. This is supported by the lower transport constant Ktrans and kep for DCE-CT in this study using Visipaque. The higher Ktrans observed for MRI may also be caused by an erroneous scaling or sampling of the CT AIF, but this will not affect kep thus supporting a difference in extracellular diffusion between the two contrast agents. Finally, the AIF variation analysis ( and Supplementary Table I to be found online at http://informahealthcare.com/doi/abs/10.3109/0284186X.2013.813637) suggests that the variation of the AIF shape is 20–40% which according to Korporaal [Citation35] would warrant the use of a population averaged AIF. From the Bland- Altman plots in a large variation is observed using the Parker AIF which may partly reflect the limitation of using population AIF to describe the heterogeneous flow characteristics within this population.
When reviewing published DCE imaging studies in locally advanced cervix cancer patients (Supplementary Table I to be found online at http://informa healthcare.com/doi/abs/10.3109/0284186X.2013. 813637) a difference was seen in the estimation of quantitative kinetic parameters. By inspecting the imaging parameters and the post-processing approach for derivation of quantitative parameters, it could be seen that there was significant variation of parameters of importance such as contrast agent, AIF, injection rate, estimation of hematocrit value, and time resolution. On top of this there may be other factors of importance related to the imaging protocol. This suggests that estimation of quantitative kinetic parameters in DCE imaging may depend critically on a number of parameters. Caution should therefore be used when trying to compare quantitative parameters across different scanners and protocols.
The choice of hematocrit values and vessel ratio has straightforward impact on the parameter estimation. The scaling of Cp(t) by (1-r·Hct)/(1-Hct) directly translates into the same inverse scaling of the Ktrans and vp values and it is therefore directly possible to compare studies that have assumed different values of hemotocrit and vessel ratio. The MRI parameters used by Donaldson et al. [Citation39] are similar to the parameters used in this study. When using the CT AIF and magnitude AIF for the pretreatment scans only, there was a good agreement between our results and those reported by Donaldson et al. However, when taking into account the different use of hematocrit values and vessel ratio the agreement was not as strong.
Though the Pearson's correlations in showed a good spatial correlation between the corresponding CT and MRI parameter, the Pearson's correlations do not take spatial deformations into account. To take into account a geometrical shift of voxels we chose to use the Γ-analysis most often used to compare two dose distributions. The potential use of parametric maps to define sub-volumes for dose escalation requires knowledge of both quantitative and spatial stability. The results of the Γ-analysis indicated that a margin around one such sub-volume using either of these parametric maps should be 3 mm in order to cover all but in average 26% of the chosen sub-volume, given an uncertainty of 10% of the chosen parameter. This is of course only valid within the time between the two scans (˜2 h), and therefore further investigations need to be carried out in order to gain information about the spatial stability on longer time scales.
The assumptions of this study include an instant exchange of magnetization after introduction of MRI contrast agent, the so called fast exchange limit. Also, it was assumed that the compartments are uniform and well mixed and that the pharmacokinetic parameters are time invariant.
In this study, specific care was taken to make the temporal acquisition parameters the same for the two techniques. For further improvements, a higher filament current (mAs) should be chosen to increase SNR for the DCE-CT. Also the contrast agents should be chosen such that they have similar molecular weights. The use of phase images to gain better determination of the patient specific input function should be pursued. Practically performing the DCE-MRI prior to the DCE-CT will reduce the time between the two scans and would thus minimize difference in patient physiology. Finally, implementation of models that better describe the underlying physiological system as suggested by Donaldson et al. [Citation39] should be further investigated.
In conclusion a good to excellent correlation but poor concordance between kinetic parameters Ktrans, vp and kep estimated using DCE-CT and -MRI was found. ve showed poorer correlation. Γ-analysis indicated that the spatial distribution of perfusion and permeability is stable over 2 h and that both DCE- CT and -MRI can be used to represent the spatial distribution. However, there was a significant bias between the DCE-CT and -MRI parameters which may be partly related to the type of contrast agent. Furthermore, the choice of AIF had considerable impact on the quantitative DCE-MRI parameter estimates. Comparison of quantitative parameters from different institutions revealed variation, and suggests that a number of parameters related to, e.g. contrast agent, imaging protocol and analysis may have considerable impact on the quantitative estimates. Therefore great caution should be used when comparing quantitative parameters across different scanners and protocols. Further research in the influence of DCE imaging protocol and kinetic analysis is strongly warranted, before allowing meaningful multicenter comparison of quantitative kinetic parameters derived from DCE imaging.
Supplementary Table I and Supplementary Figures 1, 2
Download PDF (3.4 MB)Declaration of interest: This study has been supported by research grants from the Danish Cancer Society, Danish Council of Strategic Research, CIRRO – the Lundbeck Foundation Centre for Interventional Research in Radiation Oncology. Funding from the European Programme (FP7/2013–2016) under grant agreement no. [ICT-2011.5.2] (DrTherapat) was received. The authors report no conflicts of interest. The authors alone are responsible for the content and writing of the paper.
References
- Yuh WTC, Mayr NA, Jarjoura D, Wu D, Grecula JC, Lo SS, et al. Predicting control of primary tumor and survival by DCE MRI during early therapy in cervical cancer. Invest Radiol 2009;44:343–50.
- Halle C, Andersen E, Lando M, Aarnes EK, Hasvold G, Holden M, et al. Hypoxia-induced gene expression in chemoradioresistant cervical cancer revealed by dynamic contrast-enhanced MRI. Cancer Res 2012;72:5285–95.
- Andersen EKF, Hole KH, Lund KV, Sundfør K, Kristensen GB, Lyng H, et al. Pharmacokinetic parameters derived from dynamic contrast enhanced MRI of cervical cancers predict chemoradiotherapy outcome. Radiother Oncol 2013;107: 117–22.
- Andersen EKF, Kristensen GB, Lyng H, Malinen E. Pharmacokinetic analysis and k-means clustering of DCEMR images for radiotherapy outcome prediction of advanced cervical cancers. Acta Oncol 2011;50:859–65.
- Ellingsen C, Hompland T, Mathiesen B, Rofstad EK. Microenvironment-associated lymph node metastasis of human cervical carcinoma xenografts. Acta Oncol 2012; 51:465–72.
- Søvik A, Skogmo HK, Bruland OS, Olsen DR, Malinen E. DCEMRI monitoring of canine tumors during fractionated radiotherapy. Acta Oncol 2008;47:1249–56.
- Øvrebø KM, Ellingsen C, Hompland T, Rofstad EK. Dynamic contrast-enhanced magnetic resonance imaging of the metastatic potential of tumors: A preclinical study of cervical carcinoma and melanoma xenografts. Acta Oncol 2013;52:604–11.
- Øvrebø KM, Hompland T, Mathiesen B, Rofstad EK. Assessment of hypoxia and radiation response in intramuscular experimental tumors by dynamic contrast-enhanced magnetic resonance imaging. Radiother Oncol 2012;102: 429–35.
- Gulliksrud K, Øvrebø KM, Mathiesen B, Rofstad EK. Differentiation between hypoxic and non-hypoxic experimental tumors by dynamic contrast-enhanced magnetic resonance imaging. Radiother Oncol 2011;98:360–4.
- Cheng HLM, Wright GA. Rapid high-resolution T(1) mapping by variable flip angles: Accurate and precise measurements in the presence of radiofrequency field inhomogeneity. Magn Reson Med 2006;55:566–74.
- Roberts C, Little R, Watson Y, Zhao S, Buckley DL, Parker GJM. The effect of blood inflow and B(1)-field inhomogeneity on measurement of the arterial input function in axial 3D spoiled gradient echo dynamic contrast-enhanced MRI. Magn Reson Med 2011;65:108–19.
- Bazelaire C, Rofsky N, Duhamel G, Zhang J, Michaelson MD, George D, et al. Combined T2* and T1 measurements for improved perfusion and permeability studies in high field using dynamic contrast enhancement. Eur Radiol 2006;16:2083–91.
- Nazarpoor M. Effect of concentration of contrast agent on the inflow effect for measuring absolute perfusion by use of inversion recovery T1-weighted TurboFLASH images. Radiol Phys Technol 2012;5:86–91.
- Ivancevic MK, Zimine I, Montet X, Hyacinthe JN, Lazeyras F, Foxall D, et al. Inflow effect correction in fast gradient-echo perfusion imaging. Magn Reson Med 2003; 50:885–91.
- Yang C, Stadler WM, Karczmar GS, Milosevic M, Yeung I, Haider MA. Comparison of quantitative parameters in cervix cancer measured by dynamic contrast-enhanced MRI and CT. Magn Reson Med 2010;63:1601–9.
- Parker GJM, Roberts C, Macdonald A, Buonaccorsi GA, Cheung S, Buckley DL, et al. Experimentally-derived functional form for a population-averaged high-temporal-resolution arterial input function for dynamic contrast- enhanced MRI. Magn Reson Med 2006;56:993–1000.
- Yang C, Karczmar GS, Medved M, Stadler WM. Multiple reference tissue method for contrast agent arterial input function estimation. Magn Reson Med 2007;58: 1266–75.
- Korporaal JG, van den Berg CAT, van Osch MJP, Groenendaal G, van Vulpen M, van der Heide UA. Phase-based arterial input function measurements in the femoral arteries for quantification of dynamic contrast-enhanced (DCE) MRI and comparison with DCE-CT. Magn Reson Med 2011;66:1267–74.
- Akbudak E, Conturo TE. Arterial input functions from MR phase imaging. Magn Reson Med 1996;36:809–15.
- Akbudak E, Norberg RE, Conturo TE. Contrast-agent phase effects: An experimental system for analysis of susceptibility, concentration, and bolus input function kinetics. Magn Reson Med 1997;38:990–1002.
- Tanderup K, Nielsen SK, Nyvang GB, Pedersen EM, Røhl L, Aagaard T, et al. From point A to the sculpted pear: MR image guidance significantly improves tumour dose and sparing of organs at risk in brachytherapy of cervical cancer. Radiother Oncol 2010;94:173–80.
- Pötter R, Georg P, Dimopoulos JCA, Grimm M, Berger D, Nesvacil N, et al. Clinical outcome of protocol based image (MRI) guided adaptive brachytherapy combined with 3D conformal radiotherapy with or without chemotherapy in patients with locally advanced cervical cancer. Radiother Oncol 2011;100:116–23.
- Dimopoulos JCA, Petrow P, Tanderup K, Petric P, Berger D, Kirisits C, et al. Recommendations from Gynaecological (GYN) GEC-ESTRO Working Group (IV): Basic principles and parameters for MR imaging within the frame of image based adaptive cervix cancer brachytherapy. Radiother Oncol 2012;103:113–22.
- Brookes JA, Redpath TW, Gilbert FJ, Murray AD, Staff RT. Accuracy of T1 measurement in dynamic contrast-enhanced breast MRI using two- and three-dimensional variable flip angle fast low-angle shot. J Magn Reson Imaging 1999;9:163–71.
- Gowland P, Mansfield P, Bullock P, Stehling M, Worthington B, Firth J. Dynamic studies of gadolinium uptake in brain tumors using inversion-recovery echo-planar imaging. Magn Reson Med 1992;26:241–58.
- Rohrer M, Bauer H, Mintorovitch J, Requardt M, Weinmann HJ. Comparison of magnetic properties of MRI contrast media solutions at different magnetic field strengths. Invest Radiol 2005;40:715–24.
- Sharma P, Socolow J, Patel S, Pettigrew RI, Oshinski JN. Effect of Gd-DTPA-BMA on blood and myocardial T1 at 1.5T and 3T in humans. J Magn Reson Imaging 2006;23: 323–30.
- Korporaal JG, van den Berg CAT, Jeukens CRLPN, Groenendaal G, Moman MR, Luijten P, et al. Dynamic contrast-enhanced CT for prostate cancer: Relationship between image noise, voxel size, and repeatability. Radiology 2010;256:976–84.
- Tofts PS. Modeling tracer kinetics in dynamic Gd-DTPA MR imaging. J Magn Reson Imaging 1997;7:91–101.
- Murase K. Efficient method for calculating kinetic parameters using T1-weighted dynamic contrast-enhanced magnetic resonance imaging. Magn Reson Med 2004;51: 858–62.
- Henderson E, Milosevic MF, Haider MA, Yeung IWT. Functional CT imaging of prostate cancer. Phys Med Biol 2003;48:3085–100.
- Kim SM, Haider MA, Milosevic M, Yeung IWT. A comparison of dynamic contrast-enhanced CT and MR imaging-derived measurements in patients with cervical cancer. Clin Physiol Funct Imaging 2013;33:150–61.
- Murase K, Kikuchi K, Miki H, Shimizu T, Ikezoe J. Determination of arterial input function using fuzzy clustering for quantification of cerebral blood flow with dynamic susceptibility contrast-enhanced MR imaging. J Magn Reson Imaging 2001;13:797–806.
- Kjølby BF, Mikkelsen IK, Pedersen M, Østergaard L, Kiselev VG. Analysis of partial volume effects on arterial input functions using gradient echo: A simulation study. Magn Reson Med 2009;61:1300–9.
- Korporaal JG. Ph.D. Dissertation: Dynamic contrast- enhanced (DCE) imaging for tumor delineation in prostate cancer. Department of Radiotherapy, University Medical Center Utrecht, Utrecht, the Netherlands; 2011.
- Miles KA, Young H, Chica SL, Esser PD. Quantitative contrast-enhanced computed tomography: Is there a need for system calibration?. Eur Radiol 2007;17:919–26.
- Takanami K, Higano S, Takase K, Kaneta T, Yamada T, Ishiya H, et al. Validation of the use of calibration factors between the iodine concentration and the computed tomography number measured outside the objects for estimation of iodine concentration inside the objects: Phantom experiment. Radiat Med 2008;26:237–43.
- Persoon LCGG, Podesta M, van Elmpt WJC, Nijsten SMJJG, Verhaegen F. A fast three-dimensional gamma evaluation using a GPU utilizing texture memory for on-the-fly interpolations. Med Phys 2011;38:4032–5.
- Donaldson SB, West CML, Davidson SE, Carrington BM, Hutchison G, Jones AP, et al. A comparison of tracer kinetic models for T1-weighted dynamic contrast-enhanced MRI: Application in carcinoma of the cervix. Magn Reson Med 2010;63:691–700.
- Turetschek K, Preda A, Novikov V, Brasch RC, Weinmann HJ, Wunderbaldinger P, et al. Tumor microvascular changes in antiangiogenic treatment: Assessment by magnetic resonance contrast media of different molecular weights. J Magn Reson Imaging 2004;20:138–44.