Abstract
Aim. The purpose was to analyse the properties of two models for the assessment of return to work after sickness certification, a manual one based on clinical judgement including non-measurable information (‘gut feeling’), and a computer-based one.
Study population. All subjects aged 18 to 63 years, sickness-certified at a primary health care centre in Sweden during 8 months (n = 943), and followed up for 3 years.
Methods. Baseline information included age, sex, occupational status, sickness certification diagnosis, full-time or part-time current sick-leave, and sick-leave days during the past year. Follow-up information included first and last day of each occurring sick spell. In the manual model all subjects were classified, based on baseline information and gut feeling, into a high-risk (n = 447) or a low-risk group (n = 496) regarding not returning to work when the present certificate expired. It was evaluated with a Cox’s analysis, including time and return to work as dependent variables and risk group assignment as the independent variable, while in the computer-based model the baseline variables were entered as independent variables.
Results. Concordance between actual return to work and return to work predicted by the analysis model was 73%–76% during the first 28–180 days in the manual model, and approximately 10% units higher in the computer-based model. Based on the latter, three nomograms were constructed providing detailed information on the probability of return to work.
Conclusion. The computer-based model had a higher precision and gave more detailed information than the manual model.
Introduction
Sickness absence is a major public health and economic problem in Sweden. In 2012, social insurance expenditures totalled approximately SEK 203 billion or 6% of GDP, of which more than half was used for people with an illness or disability (Citation1). Almost 9% (504,000 people) of registered insured persons in the age range 16–64 years received sickness benefit at some point in 2012. Compensated sickness absence rates vary considerably over time. They more than doubled from the end of the 1990s to the beginning of the 2000s, of which long-term sick-leave spells (>59 days) explained the greater part.
Long-term sickness absence usually starts with recurrent periods of short-term sick-leave that tend to increase in duration, interspersed with shorter and shorter non-sick-leave intervals (Citation2). In 2002 long-term sick-leave constituted 30% of all sick-leave in women as well as in men, then declined during the following years to 15% in 2010, but then increased again in the last few years to around 20%. The variations in sickness absence over time are affected by change of compensation levels, adoption of a sick pay period, business cycle, and other factors (Citation1).
All residents in Sweden are covered by the National Social Insurance, which includes the right to see a physician of one’s own choice, access to hospital care at heavily subsidized rates, to have sickness benefit for income loss in case of reduced work capacity due to injury or disease, and a number of other items. The National Social Insurance is regulated by the Social Insurance Code (2010:110) and is managed by the National Social Insurance Agency (SIA), a government agent with offices in all municipalities across the country.
In case of sick-leave, a patient’s self-certification is accepted for an initial period of 7 days. After this period a sickness certificate form has to be completed by the patient’s physician and sent to the patient’s work-place. The employer is responsible for sick-leave pay during the first 14 days. If the sickness absence persists after this time, the sick-leave certificate is sent to the local SIA office, which then takes responsibility for sickness compensation and for further handling. For unemployed subjects SIA takes responsibility for sickness compensation from day 1. Otherwise, the same procedure as for employed subjects was followed (Social Insurance Code 2010:110).
All physicians in Sweden are allowed to issue sickness certificates, but the role of sickness certification falls predominately on the general practitioners (GPs) (Citation3), who regard sickness certification a common but problematic task (Citation4-6). A study from Ireland concluded that GPs face many challenges and complexities in sickness certification in day-to-day practice and that these problems are similar to those in other European countries (Citation7). One difficulty when issuing a sickness certificate is the assessment as to whether the patient will return to work (RTW) or not after a defined period of sick-leave, important because of the risk of long-term sick-leave.
However, GPs’ ability to predict RTW has been questioned. Reiso et al. found that physicians’ prediction of RTW was highly accurate for short-term episodes but less so for more long-term ones (Citation8). Fleten et al. found that sick-listed individuals predicted their length of sick-leave more accurately than professionals did (Citation9).
A possible reason for these prediction difficulties may be the lack of assessment tools. In other research areas various instruments for the prediction of outcomes have been forwarded, of which the most common are so-called nomograms, where the combined effects of various levels of predictors for the risk of a specific outcome may be arrived at. Examples are the risk nomograms for myocardial infarction (Citation10-12), the WHO Fracture Risk Prediction Tool (FRAX) for evaluation of the probability of a fracture (Citation13), and the asthma risk nomogram to assess the risk in preschool children of developing asthma during the next 5 years (Citation14). No similar instrument for the prediction of RTW has been presented so far.
This report is based on a longitudinal study of RTW after sickness certification. The aim was to compare the predictive properties of a traditional GP clinical assessment of RTW, with those based on a multivariate analysis of potential determinants presented as nomograms for RTW.
Methods
Setting
The study has been described in detail elsewhere (Citation15). It was performed in the city of Eskilstuna, Sweden, an industrial city, located 110 kilometres west of Stockholm, with 91,000 residents in 2004. The study was performed at one of the County Council-operated primary health care centres, with 10 GPs serving a population of approximately 25,000 residents.
Study population
The study was designed as a 3-year prospective cohort study with recruitment from 1 January until 31 August 2004. During the recruitment period copies of all sickness certificates, whether new or prolongation ones, issued at the primary health care centre, were obtained. All individuals aged 18 to 63, who were sickness-certified by a physician at the centre at any time during the recruitment period, and who were not already included in a medical or vocational rehabilitation programme, were included, altogether 943 subjects (482 women and 461 men).
Data collection
Once a week all new sickness certificates were examined by a team of experienced rehabilitation professionals including a physiotherapist, a physician (first author), and a clerk from the local SIA office. The members of the team were the same during the 8-month inclusion period. From the sickness certificate information on age, sex, occupational status (in gainful work or not), sick-leave diagnosis (according to the WHO International Classification of Diseases (ICD-10)) (Citation16), and degree of sick-leave (25%, 50%, 75%, or 100%) was obtained. Information on sickness absence during the year preceding the baseline examination and during the 3 years following baseline was obtained from the SIA national database, and included first and last day of each sickness spell, type of sickness benefit (compensation for sickness or rehabilitation), sick-leave diagnosis, whether a disability pension was granted during follow-up, information on marital status, income, whether born in Sweden, and for immigrants whether being a Swedish citizen. Sick certification data were checked with the primary health care medical records in order to get valid information on the sick-leave during the first 14 days (employer-paid sick-leave salary).
Information on vital status was obtained from the National Cause of Death Register, providing date of death for those who died (n = 6). The study was approved by the Regional Ethical Review Board in Stockholm, Sweden.
Statistical considerations
Data were analysed with the SAS software, version 9.3. There were no missing data. In Sweden people may earn a living in five ways: they may have a job with a salary, they may be on sickness benefit/disability pension, they may be on unemployment benefit, they may rely on municipality income support, or they may rely on the informal economy sector (student, artist, own capital, ‘black market’, etc.). RTW was therefore defined as conclusion of the sick certification in effect at baseline (even though the vast majority of subjects, 78%, returned to work). The 3-year sick-leave follow-up data were converted into a day-by-day matrix starting with variable day 0 (baseline day) and ending with variable day 1095 (end of follow-up), each variable measuring whether the subject was on sick-leave (=1) or not (=0) on that day.
Based on this matrix a RTW variable was computed. For each sick spell the following two RTW criteria were applied; 1) the sick spell was followed by a sick-leave-free interval of more than 28 days, regardless of the length of any following sick spell, 2) the sick spell was followed by a sick-leave-free interval of more than 7 days, and that interval was longer than the next sick spell. When at least one of the criteria was satisfied, RTW was presumed to have occurred on the first non-sick-leave day. If none of the criteria were satisfied at end of follow-up no RTW presumably had occurred. Follow-up time from baseline to RTW or end of follow-up was measured as number of days from baseline.
In this study the power of two RTW prediction models were compared. According to the manual model sick-listed subjects were classified into a high-risk or a low-risk group for not returning to work as expected at the time of sick certification, in a way similar to the GP assessment in everyday clinical practice. The classification was performed by the team (same members throughout the study) and based on sickness certification during the last year for more than 28 days, sickness certified at baseline because of musculoskeletal disease (ICD code M) or a psychiatric disease (F), being unemployed, being more than 45 years old, and being a woman. The three first factors were given a high weight, the fourth somewhat less weight, and the two last were valid only in borderline cases as reinforcement of risk.
Typically, a woman aged 55, unemployed, and with sick-listing periods in the previous year for a diagnosis of fibromyalgia, would be categorized as high-risk, while a man, 37 years old, employed, and presently having a full sick-leave, might be categorized as low-risk. However, if this patient had many days of sick-leave last year with a diagnosis of stress, he might be classified as high-risk. Moreover, the classification contained an element of intuition (‘gut feeling’) as well, based on the assessor’s experience. Altogether 447 subjects were classified as having a high risk and 496 as having a low risk regarding RTW at expected time.
The manual model was analysed using proportional hazards regression (Cox’s analysis), with RTW and the day from baseline when it occurred as dependent variables and high/low risk group assignment as the independent variable.
The computer-based model was also based on the proportional hazards regression technique in its multivariate form. In a previous publication based on the same data set as used here, the significant determinants for RTW were age at baseline, number of days of sick-leave during the year preceding baseline, sick-leave diagnosis, degree of sick-leave, and occupational status (Citation15). These determinants were used also in this study. RTW and the day from baseline when it occurred were entered as dependent variables, and the determinants mentioned above were entered as independent variables. The sick-leave diagnoses were grouped as ICD-10 codes F and G (labelled –2 in the analysis), I, K, and M (labelled –1), A, B, O, and L (labelled +1), N (labelled +2), H and J (labelled +3), and all other diagnoses (labelled 0). The labelling was based on Cox’s analyses with outcome and diagnoses only. The diagnostic labels reflected the association with RTW, label –2 having the slowest and label +3 the fastest RTW.
The proportionality assumption in both models was checked by the SAS ‘Lifetest’ procedure and found acceptable. During the analyses of model 2 there were signs of collinearity between occupational status, sick-leave diagnosis, and number of sick-leave days during the last year, all highly intercorrelated. After elimination of the variable with the weakest association with outcome, occupational status, no signs of collinearity remained.
From the Cox’s analysis of the computer-based model, data were obtained showing proportion returning to work for combinations of age, number of sick-leave days last year, and sick-leave diagnosis groups on day 28, day 90, and day 180 from baseline (used by SIA as times for instituting rehabilitation). These data were entered in the nomograms in .
Figure 1. Nomograms showing proportion (%) returning to work on day 28 (a), 90 (b), and day 180 (c) from baseline. Age is attained age at baseline, sick-leave days during the last year are the total number of days of sick-leave, and ICD-10 diagnostic codes are the ICD-10 chapter codes F = psychiatric disease, G = nervous system disease, I = cardiovascular disease, K = gastrointestinal disease, M = musculoskeletal disease, A = infectious disease, B = parasite disease, O = obstetric disorders, L = dermatology disease, N = genitourinary system diseases, H = eye or ear disease, J = respiratory disease. The return to work probability is obtained by drawing a line connecting the actual age and the number of sick-leave days last year. Where the line crosses the bold vertical line in the middle of the nomogram, the return to work probability (%) is found by moving horizontally to the sick-leave diagnosis group. For example, the proportion returning to work within the first 28 days in a 54-year-old subject with 73 sick-leave days last year and an F diagnosis is approximately 38%, and 78% if the diagnosis was J. The corresponding proportions at day 90 are approximately 56% and 92%, respectively.
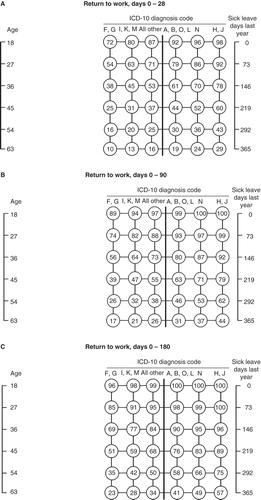
Degree of explanation was measured as agreement between the real (crude) outcome and outcome according to the analysis models, with concordance c index derived from logistic regression. All tests were two-tailed, and the significance level was set at p < 0.05.
Results
Characteristics of the study population
Slightly more than half of the subjects were women, mean and median age were 39 years, more than 75% were employed, more than 93% were Swedish citizens, 44% were never married and somewhat more than one-third were married, mean number of days of sick-leave during the year preceding baseline was 51, and the vast majority were on full-time sick-leave (). The most common sickness certification diagnoses in rank order were musculoskeletal disease, psychiatric disease, and respiratory system disease.
Table I. Characteristics of the study population.
At baseline all subjects were on sick-leave. Among both men and women, 50% of the subjects had returned to work within 14 days, 75% of the men within 55 days, and 75% of the women within 80 days. At end of the follow-up, 6 (1.3%) men and 9 (1.9%) women were still on sick-leave.
Return to work according to the manual model
As shown in , those classified as being at high risk of not returning to work as expected were significantly older, were women and unemployed to a larger extent, were on full-time sick-leave to a lesser extent, had more often more than 28 days of sick-leave last year, and had musculoskeletal or psychiatric diagnoses to a larger extent than those at low risk.
Table II. Characteristics of the risk groups and effects of the risk grouping on return to work.
The hazard ratio of RTW in the high-risk group in relation to the low-risk group was 0.29 (0.24–0.34) after the first 28 days, 0.32 (0.28–0.38) during the first 90 days, and 0.35 (0.30–0.40) during the first 180 days of follow-up, indicating 71%, 68%, and 65% less RTW during the three time periods in the high-risk group than in the low-risk group. The agreement between the real (crude) outcome and outcome according to the analysis model was 76.0%, 74.0%, and 73.2% for the three time periods, respectively.
Return to work according to the computer-based model
The results are shown in . During the first 28, 90, and 180 days, increasing age, increasing number of sick-leave days last year, and being on full-time sick-leave decreased the probability for RTW. Moreover, there was a gradient among the sick-leave diagnoses with a progressively larger decrease of RTW probability with more ‘heavy’ diagnoses. According to Wald’s chi-square, the most influential determinants during all the three time periods were number of sick-leave days last year and sick-leave diagnosis followed by age, while degree of sick-leave was of minor importance. The agreement between the real (crude) outcome and analysis model outcome was 86.1%, 88.0%, and 89.4%, approximately 10% units higher than in the manual model.
Table III. Effects of possible determinants on return to work during three time periods.
The proportion of the study population returning to work in relation to age at baseline, days of sick-leave, and sick-leave diagnosis is shown in . During the first 28 days RTW ranged from 10% among the oldest subjects with the most ‘heavy’ diagnoses (F and G), and a long track record of previous sick-leave, to 98% among the youngest with the ‘lightest’ diagnoses (H and J) and no sick-leave during the last year.
also shows RTW after 90 and 180 days. On day 90 from baseline the RTW ranged from 17% among the oldest with the most ‘heavy’ diagnoses (F and G), and a long track record of previous sick-leave, to 100% among the youngest with the lightest diagnoses (H and J) and no sick-leave during the last year. The corresponding range of proportions on day 180 was 23% to 100%.
Discussion
The two RTW assessment models performed well, with a high agreement between actually occurring RTW and RTW according to the two analysis models, even though the computer-based one had a better precision (89% agreement) than the manual model (76%) and also provided more detailed information on the probability of RTW. Both models were stable over time, at least to day 180, when the vast majority of subjects had returned to work.
The study population included all patients that were sickness-certified during a certain time period (time window), which means that the study population might be regarded as equivalent to a random sample. Moreover, the data on which the two models were based were obtained from official sources, such as sick-leave certificates, medical records, and the national SIA database. During the study period the Eskilstuna population had similar age and sex distribution and similar socio-economic characteristics as the national Swedish population (Citation17). Moreover, the Swedish cities of Gothenburg, Uppsala, and Eskilstuna followed the same track regarding proportion of the population being on sick-leave from 1986 and onwards (Citation2). This means that the study population appears to be representative for a Swedish sickness-certified population, and the data on which RTW was based have high face validity.
Regarding the relevance of the results for other countries than Sweden, it is well known from other research areas, for instance cardiovascular disease research, that the level of risk for an incident varies geographically (Citation18), but the determinants, or risk factors, for an incident are the same irrespective of geography (Citation11,12). The situation appears to be the same for RTW after sickness certification. In Dutch, Danish, and British studies, sick-leave track record data have been shown to be a risk marker of future sickness absence and disability pension (Citation19-21). The importance of sick-leave diagnosis in relation to delay of RTW is well studied (Citation22-28), and so is the variation in prevalence of sickness certification (Citation29). This means that nomograms like those in this report perhaps have to be revised regarding level (probability of RTW), but the main construct may be used internationally.
The strengths of the study include the large study population, the complete data set, and the high precision in the analyses. A possible limitation could be the choice of determinants for the analysis, since other variables might serve the same purpose. However, the degree of explanation in the regression-based model, close to 90%, means that the possibility that any overlooked strong determinant might alone provide 10% explanation is unlikely.
The models tested in this report were supposed to have different underlying properties, in spite of the fact that they basically relied on the same variables. The manual model was mimicking the way GPs assess risk of long-term sick-leave, based on presence or absence of known risk factors but also with intuitive elements. GPs often face complicated, vague problems in situations of uncertainty, which have to be solved in a brief consultation. In these situations, ‘gut feeling’ has been shown to play a significant role in the diagnostic process, also described as a third track beside medical decision-making and medical problem-solving (Citation30). Furthermore, gut feeling is a specific form of intuition based on the interaction between patient information and the GP’s own knowledge and experience. In a qualitative Dutch GP study, two types of gut feeling were distinguished, a sense of reassurance, and a sense of alarm (Citation31). The GPs explained the first as being confident about prognosis and therapy, although they might not have a clear diagnosis in mind. The latter was described as a feeling of something being wrong even though arguments for this circumstance were lacking. In another study the presence of gut feeling in diagnostic reasoning among GPs could be measurable with a validated questionnaire (Citation32). In the manual model the intuitive element, the gut feeling of the highly experienced rehabilitation team, was similar to a sense of alarm in cases classified into the high-risk group (‘something is wrong with the sick-listing course of this patient’).
The computer-based model was based on the standard method to construct nomograms. A priori, the manual model, being more flexible in its structure and perhaps catching important non-measurable information by its intuitive component, was assumed to perform at least as well or better than the computer-based model. However, the opposite turned out to be the case.
In both models RTW was inversely related to age, number of sick-leave days during the last year, and being on full-time sick-leave, and increased across the diagnosis code groups from F and G to H and J. In the manual model female sex was used as a factor associated with a moderate delay in RTW. In the computer-based model sex did not affect RTW, since it was competed out by other factors. The manual model provides only a yes/no grading of increased risk of delayed RTW, while the computer-based model provided a graded probability of RTW, ranging from only a few per cent for those with the slowest RTW up to 100% for those with the fastest.
The outcome in both models was the actually occurring RTW. The number of subjects returning to work according to the two models was the same as the actual RTW. The difference between the models refers to their precision in correctly identifying subjects that actually returned to work and those that did not. The proportion of correctly identified subjects returning or not returning to work was 73%–76% with the manual model, indicating that a quarter of the subjects were misclassified. In the computer-based model 86%–89% were correctly classified, the proportion misclassified thus being less than half compared to the manual method.
The development of nomograms to predict RTW among sickness-certified patients is a novelty of this study. An implication for clinical practice of the findings in this study might be that the nomograms can be used not only by GPs when assessing their sickness-certified patients’ time for RTW, but also for rehabilitation teams and stakeholders in vocational rehabilitation. Patients with high risk may be referred to a rehabilitation team for a deeper assessment of choice of therapy and whether vocational rehabilitation would enable the patient to regain work ability. In a third step, systematic rehabilitation in co-operation between employer, occupational health service, and the local SIA might be useful (Citation33).
Conclusions
Both models performed well, with a high concordance between actually occurring RTW and RTW according to the analysis models. However, the computer-based model had a moderately higher precision and provided more detailed information on probability of RTW than the manual model.
Acknowledgements
The study was supported by grants from Samordningsförbundet RAR, Sörmland; Centre for Clinical Research Sörmland; Uppsala University, Eskilstuna; Sörmland County Council; and Uppsala University, Sweden. Thanks are due to Professor Hans Wedel, Gothenburg, for valuable comments on the analysis model and to Hans G. Eriksson, Eskilstuna, for help with the data set. A.S.v.C. was responsible for the conception and design of the study and data collection. K.S. analysed the data. A.S.v.C., T.W., and K.S. drafted the manuscript. All authors participated in the interpretation of the results, the revision of the manuscript, and the approval of the final manuscript version.
Declaration of interest: The authors report no conflicts of interest. The authors alone are responsible for the content and writing of the paper.
References
- Swedish Social Insurance Agency. Social Insurance in Figures 2013. Available at https://www.forsakringskassan.se/wps/wcm/connect/49584364-d0d8-46ad-a982-elf915b30870/sfis_2013_ e.pdf?MOD=AJPERES.
- Wallman T, Wedel H, Palmer E, Rosengren A, Johansson S, Eriksson H, et al. Sick-leave track record and other potential predictors of a disability pension. A population based study of 8,218 men and women followed for 16 years. BMC Public Health. 2009;9:104.
- Lofgren A, Hagberg J, Arrelov B, Ponzer S, Alexanderson K. Frequency and nature of problems associated with sickness certification tasks: a cross-sectional questionnaire study of 5455 physicians. Scand J Prim Health Care. 2007;25:178–85.
- Arrelov B, Alexanderson K, Hagberg J, Lofgren A, Nilsson G, Ponzer S. Dealing with sickness certification - a survey of problems and strategies among general practitioners and orthopaedic surgeons. BMC Public Health. 2007;7:273.
- Swartling M, Peterson S, Wahlstrom R. Views on sick-listing practice among Swedish General Practitioners–a phenomenographic study. BMC Fam Pract. 2007;8:44.
- Wynne-Jones G, Mallen CD, Main CJ, Dunn KM. What do GPs feel about sickness certification? A systematic search and narrative review. Scand J Prim Health Care. 2010;28:67–75.
- Foley M, Thorley K, Denny M. ‘The sick note’: a qualitative study of sickness certification in general practice in Ireland. Eur J Gen Pract. 2012;18:92–9.
- Reiso H, Gulbrandsen P, Brage S. Doctors’ prediction of certified sickness absence. Fam Pract. 2004;21:192–8.
- Fleten N, Johnsen R, Forde OH. Length of sick leave - why not ask the sick-listed? Sick-listed individuals predict their length of sick leave more accurately than professionals. BMC Public Health. 2004;12:46.
- Oppenheimer GM. Becoming the Framingham Study 1947-1950. Am J Public Health. 2005;95:602–10.
- Wilhelmsen L, Wedel H, Tibblin G. Multivariate analysis of risk factors for coronary heart disease. Circulation. 1973;48:950–8.
- Conroy RM, Pyorala K, Fitzgerald AP, Sans S, Menotti A, De Backer G, et al. Estimation of ten-year risk of fatal cardiovascular disease in Europe: the SCORE project. Eur Heart J. 2003;24:987–1003.
- Kanis JA, McCloskey EV, Johansson H, Oden A, Strom O, Borgstrom F. Development and use of FRAX in osteoporosis. Osteoporos Int. 2010;21:407–13.
- Broms K, Norback D, Sundelin C, Eriksson M, Svardsudd K. A nationwide study of asthma incidence rate and its determinants in Swedish pre-school children. Eur J Epidemiol. 2012;27:695–703.
- von Celsing AS, Svardsudd K, Eriksson HG, Bjorkegren K, Eriksson M, Wallman T. Determinants for return to work among sickness certified patients in general practice. BMC Public Health. 2012;12:1077.
- WHO. International statistical classification of diseases and related health problems 1992. Tenth revision (ICD-10). Stockholm. The national board of health and welfare. In Swedish version ICD-10-SE. 2011:15–629.
- Statistics Sweden. 1.4.1 Population by sex and by municipality. 2004. Open database available at http://www.scb.se/statistik_publikationer/BE0101_2004A01_BR_06BE0105TAB.pdf.
- Nichols M, Townsend N, Scarborough P, Rayner M. Cardiovascular disease in Europe: epidemiological update. Eur Heart J. 2013;34:3028–34.
- Roelen CA, Koopmans PC, Schreuder JA, Anema JR, van der Beek AJ. The history of registered sickness absence predicts future sickness absence. Occup Med. 2011;61:96–101.
- Lund T, Kivimaki M, Labriola M, Villadsen E, Christensen KB. Using administrative sickness absence data as a marker of future disability pension: the prospective DREAM study of Danish private sector employees. Occup Environ Med. 2008;65:28–31.
- Whittaker W, Sutton M, Maxwell M, Munoz-Arroyo R, Macdonald S, Power A, et al. Predicting which people with psychosocial distress are at risk of becoming dependent on state benefits: analysis of routinely available data. BMJ. 2010;341:c3838.
- Mallen CD, Wynne-Jones G, Dunn KM. Sickness certification for mental health problems: an analysis of a general practice consultation database. Prim Health Care Res Dev. 2011;12:179–82.
- Alexanderson KA, Borg KE, Hensing GK. Sickness absence with low-back, shoulder, or neck diagnoses: an 11-year follow-up regarding gender differences in sickness absence and disability pension. Work. 2005;25:115–24.
- Bergstrom G, Bodin L, Bertilsson H, Jensen IB. Risk factors for new episodes of sick leave due to neck or back pain in a working population. A prospective study with an 18-month and a three-year follow-up. Occup Environ Med. 2007;64:279–87.
- Ferrie JE, Vahtera J, Kivimaki M, Westerlund H, Melchior M, Alexanderson K, et al. Diagnosis-specific sickness absence and all-cause mortality in the GAZEL study. J Epidemiol Community Health. 2009;63:50–5.
- Gjesdal S, Haug K, Ringdal P, Maeland JG, Hagberg J, Roraas T, et al. Sickness absence with musculoskeletal or mental diagnoses, transition into disability pension and all-cause mortality: a 9-year prospective cohort study. Scand J Public Health. 2009;37:387–94.
- Gjesdal S, Bratberg E. Diagnosis and duration of sickness absence as predictors for disability pension: results from a three-year, multi-register based* and prospective study. Scand J Public Health. 2003;31:246–54.
- Koopmans PC, Bultmann U, Roelen CA, Hoedeman R, van der Klink JJ, Groothoff JW. Recurrence of sickness absence due to common mental disorders. Int Arch Occup Environ Health. 2011;84:193–201.
- Wynne-Jones G, Mallen CD, Welsh V, Dunn KM. Rates of sickness certification in European primary care: a systematic review. Eur J Gen Pract. 2009;19:1–10.
- Stolper E, Van de Wiel M, Van Royen P, Van Bokhoven M, Van der Weijden T, Dinant GJ. Gut feelings as a third track in general practitioners’ diagnostic reasoning. J Gen Intern Med. 2011;26:197–203.
- Stolper E, van Bokhoven M, Houben P, Van Royen P, van de Wiel M, van der Weijden T, et al. The diagnostic role of gut feelings in general practice. A focus group study of the concept and its determinants. BMC Fam Pract. 2009;10:17.
- Stolper CF, Van de Wiel MW, De Vet HC, Rutten AL, Van Royen P, Van Bokhoven MA, et al. Family physicians’ diagnostic gut feelings are measurable: construct validation of a questionnaire. BMC Fam Pract. 2013;14:1.
- Karrholm J, Ekholm K, Ekholm J, Bergroth A, Ekholm KS. Systematic co-operation between employer, occupational health service and social insurance office: a 6-year follow-up of vocational rehabilitation for people on sick-leave, including economic benefits. J Rehabil Med. 2008;40:628–36.