Abstract
Objective: Most studies so far have focused on the performance of individual biomarkers to detect early acute kidney injury (AKI) in the adult intensive care unit (ICU) patients; however, they have not determined the predictive ability of their combinations. The aim of this study was to compare the predictive abilities of plasma neutrophil gelatinase-associated lipocalin (pNGAL), urine neutrophil gelatinase-associated lipocalin (uNGAL), plasma cystatin C (pCysC), serum creatinine (sCr), and their combinations in detecting AKI in an adult general ICU population. Methods: A total of 100 consecutive ICU patients were included in the analysis. AKI was defined according to RIFLE criteria. Biomarker predictive abilities were evaluated by area under the curve (AUC), net reclassification improvement (NRI), and integrated discrimination improvement (IDI). Results: AKI occurred in 36% of patients 7 days post-admission. All three novel biomarkers as well as sCr had moderate predictive abilities for AKI occurrence. The most efficient combinations (pNGAL + sCr and pNGAL + uNGAL + sCr) were selected to participate in the subsequent analyses. Both combinations, when added to a reference clinical model, increased its AUC significantly (0.858, p = 0.04). Their NRI (0.78, p = 0.0002) was equal to that of pNGAL, but higher than that of the other three biomarkers, whereas their IDI was higher than that of any individual biomarker (0.23, p = 0.0001). Both combinations had better specificities, positive likelihood ratios, and positive predictive values than those of any individual biomarker. Conclusion: The biomarker combinations had better predictive characteristics compared with those of each biomarker alone.
INTRODUCTION
The incidence of acute kidney injury (AKI), previously known as acute renal failure, in critically ill patients is currently rising,Citation1 with a mortality rate of 50% which has remained constant during the last decades.Citation2 The lack of an early biomarker is an obstacle for the development of new preventive strategies and timely interventions against AKI.Citation3 Recent research for the discovery of novel biomarkers with early diagnostic and/or prognostic value has revealed several candidates. A number of studies have investigated the prognostic abilities of neutrophil gelatinase-associated lipocalin (NGAL),Citation4–11 cystatin C (CysC),Citation11–16 kidney injury molecule-1,Citation11 interleukin-18,Citation10,11 l-type fatty acid-binding protein,Citation10 and N-acetyl-β-d-glucosaminidaseCitation10 for early AKI development in the heterogeneous population of adult critically ill patients, having found only moderate predictive abilities so far. However, none of them has evaluated their combined use in adult intensive care unit (ICU) patients. Because AKI represents a complex, multifactorial, and heterogeneous clinical condition, we hypothesized that a panel of AKI biomarkers would be superior to each biomarker alone.
The main purpose of this study was to compare the predictive abilities of admission plasma neutrophil gelatinase-associated lipocalin (pNGAL), plasma cystatin C (pCysC), urine neutrophil gelatinase-associated lipocalin (uNGAL), serum creatinine (sCr), and their combinations for AKI development within 7 days post-ICU admission.
MATERIALS AND METHODS
Study Design
This was a prospective observational study of adult patients admitted to a 30-bed general Critical Care Department of a tertiary hospital of Athens from October 2010 to March 2011. All consecutive patients admitted to the ICU were screened for eligibility. The exclusion criteria are shown in . The lowest sCr level found within 12 months prior to ICU admission was used as baseline. If it was not available, then the lowest of the on-admission sCr or final ICU sCr was used. A similar approach has been shown to be more accurate than using the Modification of Diet in Renal Disease (MDRD) equation with the same assumed glomerular filtration rate (GFR) to back-calculate a baseline sCr.Citation18 Patients were enrolled within 12 h of ICU admission at the latest. The protocol was approved by the Institutional Ethics Committee (Scientific Committee of “Evangelismos” General Hospital) and informed consent was obtained from all patients’ next-of-kin persons.
Figure 1. Study flowchart.
Notes: ESRD was defined according to KDIGO (CKD–MBD) Work Group definition: eGFR < 15 mL/h or hemodialysis.Citation17 Chronic kidney diseases included glomerulonephritis, interstitial renal disease, and obstructive uropathy. AKI, acute kidney injury; ESRD, end-stage renal disease; ICU, intensive care unit; HDU, high-dependency unit.
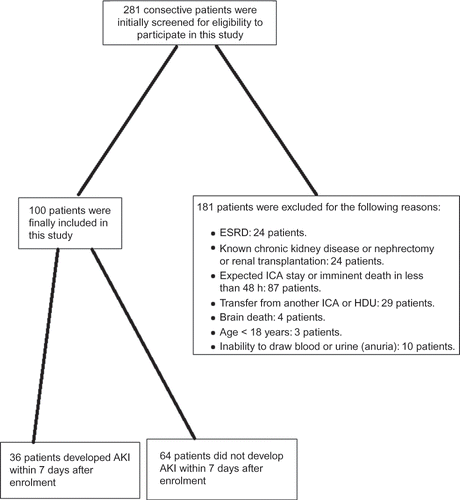
Figure 2. Receiver-operating characteristic (ROC) curves of the individual biomarkers and the two best combinations of them.
Notes: pNGAL, plasma neutrophil gelatinase-associated lipocalin; pCysC, plasma cystatin; uNGAL, urine neutrophil gelatinase-associated lipocalin; sCr, serum creatinine; AUC, area under curve. Open circle indicates the ideal cut-off point of each curve, according to the Youden’s index.Citation21
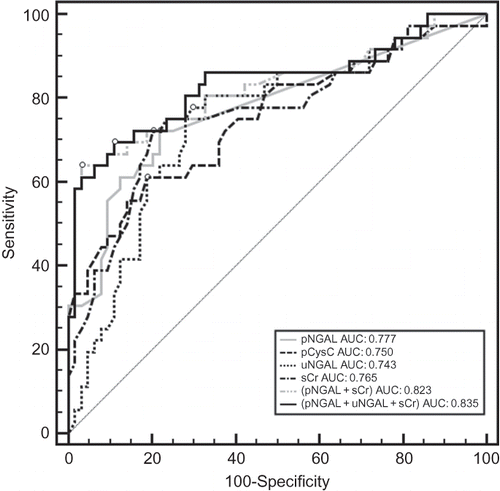
AKI was defined using the sCr and urine output criteria of the RIFLE classification,Citation19 and patients were scored daily for AKI development and RIFLE staging according to the above criteria. Demographics, comorbidities, admission diagnosis, recent exposure to nephrotoxic agents, disease severity scores, routine laboratory data, and outcome measures, such as ICU mortality, renal replacement therapy (RRT) initiation, and ICU length of stay, were also recorded.
Biomarker Measurements
Blood samples were collected within 1 h, at the latest, of enrolment. Arterial blood was sampled in ethylenediaminetetraacetic acid (EDTA) tubes from an arterial line and was centrifuged at 3000 rpm at 4°C for 10 min. The supernatants were stored at −70°C. Urine samples were also collected within 1 h after enrolment by the urine catheter reservoir and immediately stored at −70°C. pNGAL was measured using the Triage® NGAL test in conjunction with the Triage Meter (Biosite Inc., San Diego, CA, USA), a point-of-care, fluorescence-detected immunoassay. The Triage assay has a detection range of 60–1300 ng/mL with a coefficient of variation of 10–15%. Creatinine levels in both serum and urine specimens were determined by routine methods in the biochemistry laboratory of our hospital and pCysC levels were quantified by using an MNII nephelometer (Dade Behring GmbH, Marburg, Germany). uNGAL was measured by a chemiluminescent microparticle assay using the ARCHITECT platform (Abbott Diagnostics Inc., Abbott Park, IL, USA). Personnel performing the biomarker measurements were blinded to each patient’s clinical information.
Statistical Analysis
Continuous variables were expressed as mean ± standard deviation, if normally distributed, or median (interquartile range), if the normality assumption did not hold, and their comparisons were made by t-test or Mann–Whitney U-test, respectively. Categorical variables were expressed as number (%) and compared with the χ2 or Fischer’s exact test. Biomarker values in all statistical analyses refer to time-of-admission samples.
In order to assess the discriminative ability for AKI prediction, univariate and multivariate logistic regressions were used for individual biomarkers and all their possible combinations, respectively. Receiver-operating characteristic (ROC) curves were generated for each biomarker and combination. The areas under the curve (AUC) were compared using the nonparametric method developed by DeLong et al.,Citation20 and the most efficient combinations (with AUCs higher than those of the individual biomarkers) were included in the subsequent analyses. Optimal cut-off values for AKI detection were determined for each biomarker and their combinations using the Youden’s index,Citation21 based on which sensitivity (Sn), specificity (Sp), positive and negative predictive values, and positive and negative likelihood ratios were calculated.
We quantified the improvement of biomarkers and their combinations on AKI risk prediction with modern statistical methods: the category-free net reclassification improvement (NRI) and integrated discrimination improvement (IDI) indices.Citation22–24 First, the most efficient clinical model (containing simplified acute physiology score III (SAPS III) and international normalized ratio (INR)) was determined by stepwise backward elimination. Potential predictor variables for AKI development 7 days after ICU admission included age, gender, acute physiology and chronic health evaluation II (APACHE II), sequential organ failure assessment, SAPS III, hemodynamic instability, diabetes mellitus, white blood cell count, INR, lactate, and pH on admission. Multiple logistic regression analyses were conducted to assess the performance of the predictive models while combining individual biomarkers and their combinations with the reference clinical model. Improvements in the model were evaluated by AUC, NRI, and IDI. All variables were log-transformed before entering the logistic regressions because of their skewed distributions.
All tests were two-sided and significance was accepted at p < 0.05. MedCalc v.12.2.0 (MedCalc, Mariakerke, Belgium) software was used for AUC comparisons and the calculation of Sn, Sp, predictive values, and likelihood ratios. NRI and IDI were calculated using R v.2.10.1 (R Foundation, Vienna, Austria). All other statistical analyses were performed using SPSS v.12 (SPSS Inc., Chicago, IL, USA).
RESULTS
Patient Characteristics
The study flowchart is depicted in . Of the 100 patients enrolled, 36 developed AKI within 7 days, 10 needed RRT during ICU stay, while their ICU mortality rate was 33%. Among AKI patients, maximum RIFLE classes were as follows: RIFLE-R 52%, RIFLE-I 36%, and RIFLE-F 12%. Patient’s admission characteristics, renal parameters, and outcome measures are shown in . Patients who developed AKI were older, had higher APACHE II and SAPS III scores, higher baseline and admission renal parameters, and higher mortality and RRT need. uNGAL and pNGAL were moderately correlated on entry to the ICU (Spearman’s r = 0.52, p < 0.005).
Table 1. Patient’s admission characteristics, renal parameters, and outcomes.
Predictive Abilities of Individual Biomarkers and Their Combinations for AKI Development within 7 Days Post-Admission
All three novel biomarkers and sCr had moderate predictive ability for AKI occurrence and none of them had significantly higher AUC over the others, as shown in . The vast majority of their combinations had higher AUCs than that of each biomarker alone, but only two of these biomarker combinations (pNGAL + sCr and pNGAL + uNGAL + sCr) reached statistical significance (p < 0.05 vs. sCr) and were selected to participate in the subsequent analyses (). Notably, in the multivariate logistic regression model that combined all four biomarkers, only pNGAL and sCr were independently associated with AKI development (). shows the formula for the fitted multiple regression model of each combination, as first described by Han et al.Citation26,27 The ROC curves of the two combinations and the individual biomarkers are depicted in . demonstrates various predictive characteristics of each biomarker and their two best combinations based on the ideal cut-off value according to Youden’s index. Both combinations had better Sp, positive likelihood ratios [(+) LRs], and positive predictive values (PPVs) than those of any individual biomarker. Of note, the combination of pNGAL with sCr had outstanding values for Sp (97%), (+) LR (20), and PPV (92%).
Table 2. Predictive performance of each individual biomarker and all their possible combinations for AKI development within 7 days post-admission.
Table 3. Formula for fitted multiple logistic regression model for combining biomarkers.
Table 4. Predictive characteristics of admission biomarkers and their combinations for AKI development within 7 days post admission.
We then developed the most efficient reference clinical model for AKI prediction, which included SAPS III and INR, using multivariate logistic regression with stepwise backward elimination. This model could predict AKI with reasonable certainty (AUC = 0.756). Each biomarker was then added to the reference model to assess improvement in the predictive ability of the model (). Of the individual biomarkers, only the addition of pNGAL to the model increased the AUC significantly from 0.756 to 0.852 (p = 0.03). Moreover, the addition of pNGAL to the reference model improved its predictive ability, as measured by the category-free NRI (0.78, p = 0.0002) and IDI (0.16, p = 0.0004) indices, more than each of the other three biomarkers did (). Next, we followed the same procedure with the two biomarker combinations. Both of them, when added to the reference model, increased AUC significantly and slightly more than pNGAL did (0.858, p = 0.04); their NRI was equal to that of pNGAL (0.78, p = 0.0002), but higher than that of the other three biomarkers, whereas their IDI was higher than that of each individual biomarker (0.23, p = 0.0001) ().
Table 5. Category-free NRI and IDI of including biomarkers and their combinations in the risk factor model to predict AKI development within 7 days post-admission.
DISCUSSION
We performed a prospective observational study in a heterogeneous adult ICU population, the main finding of which was that biomarker combinations had better performance for AKI prediction compared with each biomarker alone. More specifically, we compared the predictive ability of four renal biomarkers (pNGAL, pCysC, uNGAL, and sCr) and their most efficient combinations for AKI development within 1 week after admission. Each biomarker combination had better AUC, higher IDI, and higher Sp, (+) LR, and PPV, compared with each biomarker alone. The NRI of each combination was equal to that of pNGAL, but higher than that of the other three biomarkers. Of individual biomarkers, pNGAL had higher NRI and IDI compared with those of pCysC, uNGAL, and sCr, although its AUC was not significantly higher than that of the aforementioned three biomarkers. Compared with the two combinations, pNGAL had the same NRI, but lower AUC, IDI, Sp, (+) LR, and PPV.
It is speculated that the increases in NGAL levels after renal tubular injury may serve to limit injury even at the beginning of the insult. In renal tubular injury, human pNGAL levels are increased on the order of 7- to 16-fold, and human uNGAL levels increased by 25- to 100-fold.Citation28 Synthesis of NGAL protein in the distal nephron and secretion into the urine appears to promote cell survival and proliferation. With respect to pNGAL, it is considered that it originates from distant organs (AKI results in a dramatically increased NGAL mRNA expression in liver and lungs)Citation29; from neutrophils, macrophages, and other immune cells as an acute phase reactant, whereas any decrease in GFR could result in its accumulation in systemic circulation.Citation30 On the other hand, pCysC is primarily a sensitive marker of reduction in GFRCitation31 and appears to be a good biomarker in the prediction of AKI.Citation32
NGAL and CysC have been used as markers of AKI in observational studies of adult ICU patients, with AUCs ranging from 0.67 to 0.92Citation4,5,7–9 for pNGAL, 0.64 to 0.86 for uNGAL,Citation4,6,8–11 and 0.72 to 0.88 for pCysC,Citation13,15,16 respectively. The vast majority of the above studies did not use indices of reclassification improvement (NRI and/or IDI), and their predictions were mainly based on AUCs. De Geus et al.Citation4 reported no significant NRIs for pNGAL and uNGAL, and Siew et al.Citation6 reported no significant NRI for uNGAL. However, both studies categorized the patients into three groups for NRI estimation and, therefore, their results cannot be compared with ours, which are based on category-free NRI estimation. Moreover, none of the above studies estimated IDI.
Most studies so far have focused on the performance of only one of these biomarkers to detect AKI in critically ill patients, before an sCr rise. However, none of them has determined the predictive ability of their combinations in the adult ICU setting, where the etiology of AKI is multifactorial and not well understood. Because many insults are involved, it is speculated that a single biomarker will be insufficiently sensitive and specific across the full spectrum of AKI, and combinations (panels) of biomarkers with different characteristics may prove more accurate. The utility of biomarker combinations in AKI prediction has been assessed by a number of studies in cardiac surgery patients, who reported improved predictive performance for the various combinations they used.Citation26,27,33–35 Nevertheless, which are the optimal combinations of biomarkers remain unclear. We found that the combinations of novel biomarkers with a conventional one (sCr) are promising in the heterogeneous population of a general adult ICU. Larger studies that will identify the most efficient and cost-effective biomarker combinations and will develop and validate AKI scoring systems are required.
The strengths of our study are the following: first, we used modern indices of risk stratification improvement and did not rely solely on AUC comparisons. Second, all novel biomarkers were determined by methods that are, or shortly will be, widely available to clinicians. We acknowledge that our study has certain limitations. First of all, it is a single-center study and its findings are not as generalizable as those of a multicenter study. Second, the relatively small sample size could reduce the power of the study. Third, the enrolment of only 100 patients from a total of 281 patients who were initially screened could have led to selection bias, and because the main reason of exclusion was an expected ICU stay less than 48 h, the more severely ill patients might have been selected. Fourth, the extent to which AKI per se contributes to pNGAL levels could be confounded by the release of NGAL into the bloodstream in patients with SIRS or sepsis.
We analyzed our data by using both absolute uNGAL and normalized to urine creatinine (uNGAL/uCr) values, and found that their AUCs did not significantly differ. More specifically, the AUC of uNGAL/uCr for AKI prediction was 0.69 (CI 95%: 0.58–0.80, p < 0.15 vs. absolute uNGAL). However, when it was used in the various multivariate models instead of absolute uNGAL, the performances of its combinations were inferior to those of absolute uNGAL. Therefore, we decided to select absolute uNGAL for all further statistical analyses. Throughout the literature, the authors use either the absolute uNGAL or uNGAL/uCr value in a spot urine sample. To date, there are no strong arguments in favor of one of them. However, there are some pitfalls when reporting absolute (raw) concentrations of a urinary biomarker; for example, oliguria will cause an increase, and polyuria a decrease, in its absolute concentration, the production and excretion rates of which are constant. Therefore, normalizing a urinary biomarker concentration to uCr takes into account the differences in urinary flow rate. On the other hand, when normalizing, one must also take into consideration the assumption that uCr excretion is constant. However, Waikar et al.Citation36 by using computer simulations of creatinine kinetics under nonsteady-state conditions, such as AKI, found that the uCr excretion rate changes over time. Consequently, normalization by uCr may result in an underestimation or overestimation of the biomarker excretion rate depending on the clinical context. The previous study found that the most accurate method to quantify urine biomarkers requires the collection of timed urine specimens to estimate the actual excretion rate.Citation36 However, this method has got some practical difficulties; for example, many patients in the ICU are anuric, and therefore, urine collection would be impossible. Moreover, it is time-consuming compared to the spot urine sample, in which case the clinician can have the results quite shortly.
In conclusion, when we evaluated the predictive ability for AKI occurrence only by means of AUC, none of the three novel biomarkers was superior to each other or to sCr, while the two combinations were better than sCr. However, when we assessed the predictive performance with modern indices of reclassification improvement, namely the category-free NRI and the IDI, the two combinations were superior not only to sCr but also to the other three novel biomarkers. Regarding each biomarker individually, pNGAL was superior to the other three. Early AKI detection by a combination of renal biomarkers could lead to timely interventions (e.g., avoidance of nephrotoxic drugs, early RRT initiation) to prevent the detrimental effects of AKI in critically ill patients.
ACKNOWLEDGMENTS
The authors thank Cyparissia Avrami for her assistance with samples collection and express appreciation to the patients and their families for their participation. The study was partially funded by a grant from the Special Account for Research Grants of National and Kapodistrian University of Athens.
Declaration of interest: The authors report no conflicts of interest. The authors alone are responsible for the content and writing of the article.
REFERENCES
- Kellum JA. Acute kidney injury. Crit Care Med. 2008; 36: S141–S145.
- Ympa YP, Sakr Y, Reinhart K, Vincent JL. Has mortality from acute renal failure decreased? A systematic review of the literature. Am J Med. 2005; 118:827–832.
- Devarajan P. Neutrophil gelatinase-associated lipocalin (NGAL): A new marker of kidney disease. Scand J Clin Lab Invest. 2008; 241(Suppl):89–94.
- De Geus H, Bakker J, Lesaffre E, Noble L. Neutrophil gelatinase-associated lipocalin at ICU admission predicts for acute kidney injury in adult patients. Am J Resp Crit care Med. 2011; 183:907–914.
- Cruz DN, de Cal M, Garzotto F, . Plasma neutrophil gelatinase-associated lipocalin is an early biomarker for acute kidney injury in an adult ICU population. Intensive Care Med. 2010; 36:444–451.
- Siew ED, Ware LB, Gebretsadik T, . Urine neutrophil gelatinase-associated lipocalin moderately predicts acute kidney injury in critically ill adults. J Am Soc Nephrol. 2009; 20: 1823–1832.
- Constantin JM, Futier E, Perbet S, . Plasma neutrophil gelatinase-associated lipocalin is an early marker of acute kidney injury in adult critically ill patients: A prospective study. J Crit Care. 2010; 25:176.e1–176.e6.
- Martensson J, Bell M, Oldner A, . Neutrophil gelatinase-associated lipocalin in adult septic patients with and without acute kidney injury. Intensive Care Med. 2010; 36:1333–1340.
- Bagshaw SM, Bennett M, Haase M, . Plasma and urine neutrophil gelatinase-associated lipocalin in septic versus non-septic acute kidney injury in critical illness. Intensive Care Med. 2010; 36:452–461.
- Doi K, Negishi K, Ishizu T, . Evaluation of new acute kidney injury biomarkers in a mixed intensive care unit. Crit Care Med. 2011; 39:2464–2469.
- Endre ZH, Pickering JW, Walker RJ, . Improved performance of urinary biomarkers of acute kidney injury in the critically ill by stratification for injury duration and baseline renal function. Kidney Int. 2011; 79:1119–1130.
- Nejat M, Pickering JW, Walker RJ, . Urinary cystatin C is diagnostic of acute kidney injury and sepsis, and predicts mortality in the intensive care unit. Crit Care. 2010; 14:R85.
- Nejat M, Pickering JW, Walker RJ, Endre ZH. Rapid detection of acute kidney injury by plasma cystatin C in the intensive care unit. Nephrol Dial Transplant. 2010; 25:3283–3289.
- Ahlström A, Tallgren M, Peltonen S, Pettilä V. Evolution and predictive power of serum cystatin C in acute renal failure. Clin Nephrol. 2004; 62:344–350.
- Royakkers A, Korevaar J, van Suijlen J, . Serum and urine cystatin C are poor biomarkers for acute kidney injury and renal replacement therapy. Intensive Care Med. 2011; 37:493–501.
- Herget-Rosenthal S, Marggraf G, Husing J, . Early detection of acute renal failure by serum cystatin C. Kidney Int. 2004; 66:1115–1122.
- Kidney Disease: Improving Global Outcomes (KDIGO) CKD–MBD Work Group. KDIGO clinical practice guideline for the diagnosis, evaluation, prevention, and treatment of chronic kidney disease–mineral and bone disorder (CKD–MBD). Kidney Int. 2009; 76(Suppl. 113):S1–S130.
- Pickering JW, Endre ZH. Back-calculating baseline creatinine with MDRD misclassifies acute kidney injury in the intensive care unit. Clin J Am Soc Nephrol. 2010; 5:1165–1173.
- Bellomo R, Ronco C, Kellum JA. The ADQI Workgroup: Acute renal failure – definition, outcome measures, animal models, fluid therapy and information technology needs: The Second International Consensus Conference of the Acute Dialysis Quality Initiative (ADQI) Group. Crit Care. 2004; 8: R204–R212.
- DeLong ER, DeLong DM, Clarke-Pearson DL. Comparing the areas under two or more correlated receiver operating characteristic curves: A nonparametric approach. Biometrics. 1988; 44:837–845.
- Youden MJ. Index for rating diagnostic tests. Cancer. 1950; 3:32–35.
- Pencina MJ, D’Agostino Sr, RB, D’Agostino Jr, RB, Vasan RS. Evaluating the added predictive ability of a new marker: From area under the ROC curve to reclassification and beyond. Stat Med. 2008; 27:157–172.
- Pencina MJ, D’Agostino Sr, RB, Steyerberg EW. Extensions of net reclassification improvement calculations to measure usefulness of new biomarkers. Stat Med. 2011; 30:11–21.
- Pencina MJ, D’Agostino Sr, RB, Demler OV. Novel metrics for evaluating improvement in discrimination: Net reclassification and integrated discrimination improvement for normal variables and nested models. Stat Med. 2012; 31:101–113.
- Levey AS, Bosch JP, Lewis JB, . A more accurate method to estimate glomerular filtration rate from serum creatinine: A new prediction equation, Modification of Diet in Renal Disease Study Group. Ann Intern Med. 1999; 130: 461–470.
- Han WK, Waikar SS, Johnson A, . Urinary biomarkers in the early diagnosis of acute kidney injury. Kidney Int. 2008; 73:863–869.
- Han WK, Wagener G, Zhu Y, . Urinary biomarkers in the early detection of acute kidney injury after cardiac surgery. Clin J Am Soc Nephrol. 2009; 4:873–882.
- Mori K, Lee HT, Rapoport D, . Endocytic delivery of lipocalin-siderophore-iron complex rescues the kidney from ischemia-reperfusion injury. J Clin Invest. 2005; 115:610–621.
- Schmidt-Ott KM, Mori K, Li JY, . Dual action of neutrophil gelatinase-associated lipocalin. J Am Soc Nephrol. 2007; 18:407–413.
- Devarajan P. Review: Neutrophil gelatinase-associated lipocalin: A troponin-like biomarker for human acute kidney injury. Nephrology. 2010; 15:419–428.
- Dharnidharka VR, Kwon C, Stevens G. Serum cystatin C is superior to serum creatinine as a marker of kidney function: A meta-analysis. Am J Kidney Dis. 2002; 40:221–226.
- Zhang Z, Lu B, Sheng X, Jin N. Cystatin C in prediction of acute kidney injury: A systemic review and meta-analysis. Am J Kidney Dis. 2011; 58(3):356–365.
- Che M, Xie B, Xue S, . Clinical usefulness of novel biomarkers for the detection of acute kidney injury following elective cardiac surgery. Nephron Clin Pract. 2010;115: c66–c72.
- Krawczeski CD, Goldstein SL, Woo JG, . Temporal relationship and predictive value of urinary acute kidney injury biomarkers after pediatric cardiopulmonary bypass. J Am Coll Cardiol. 2011; 58:2301–2309.
- Katagiri D, Doi K, Honda K, . Combination of two urinary biomarkers predicts acute kidney injury after adult cardiac surgery. Ann Thorac Surg. 2012; 93:577–583.
- Waikar SS, Sabbisetti VS, Bonventre JV. Normalization of urinary biomarkers to creatinine during changes in glomerular filtration rate. Kidney Int. 2010; 78:486–494.