Abstract
Novel acute kidney injury (AKI) biomarkers offer promise of earlier diagnosis and risk stratification, but have yet to find widespread clinical application. We measured urinary α and π glutathione S-transferases (α-GST and π-GST), urinary l-type fatty acid-binding protein (l-FABP), urinary neutrophil gelatinase-associated lipocalin (NGAL), urinary hepcidin and serum cystatin c (CysC) before surgery, post-operatively and at 24 h after surgery in 93 high risk patient undergoing cardiopulmonary bypass (CPB) and assessed the ability of these biomarkers alone and in combination to predict RIFLE-R defined AKI in the first 5 post-operative days. Twenty-five patients developed AKI. π-GST (ROCAUC = 0.75), lower urine Hepcidin:Creatine ratio at 24 h (0.77), greater urine NGAL:Cr ratio post-op (0.73) and greater serum CysC at 24 h (0.72) best predicted AKI. Linear combinations with significant improvement in AUC were: Hepcidin:Cr 24 h + post-operative π-GST (AUC = 0.86, p = 0.01), Hepcidin:Cr 24 h + NGAL:Cr post-op (0.84, p = 0.03) and CysC 24 h + post-operative π-GST (0.83, p = 0.03), notably these significant biomarkers combinations all involved a tubular injury and a glomerular filtration biomarker. Despite statistical significance in receiver–operator characteristic (ROC) analysis, when assessed by ability to define patients to two groups at high and low risk of AKI, combinations failed to significantly improve classification of risk compared to the best single biomarkers. In an alternative approach using Classification and Regression Tree (CART) analysis a model involving NGAL:Cr measurement post-op followed by Hepcidin:Cr at 24 h was developed which identified high, intermediate and low risk groups for AKI. Regression tree analysis has the potential produce models with greater clinical utility than single combined scores.
Introduction
Acute kidney injury (AKI) is associated with increased morbidity and mortalityCitation1 and is of particular clinical significance in the intensive care unit. Conventional diagnosis remains dependent on measurement of plasma creatinine.Citation2 However, plasma creatinine has many limitations, in particular, diagnosis is often delayed and imprecise in the setting of critical illness.Citation3,Citation4 Delay in diagnosis of AKI may preclude early and effective intervention. A number of early markers of AKI have been identified by proteomic analysis of plasma and urine from patients who go on to develop AKI.Citation5,Citation6 These biomarkers may accelerate AKI diagnosis and provide insights into its pathogenesis. However, while performance can be very good in homogeneous patient groups with predictable timing of renal injury, such as pediatric cardiopulmonary bypass – CPB,Citation7 diagnostic utility can be less good in a mixed populations of adults with chronic kidney disease,Citation8 or when renal insults vary in timing, nature and severity.Citation9 Furthermore, biomarkers of AKI are heterogeneous molecules. Some appear to be produced directly within the kidney as a result of tubular injury or stress (urinary neutrophil gelatinase-associated lipocalin – NGALCitation10); others may appear in the urine due to failure of proximal tubular uptake (urinary cystatin cCitation11) while others again may simply be more precise markers of alteration in glomerular filtration rate (serum cystatin c – CysCCitation11). Finally, some biomarkers of AKI may also be markers of systemic inflammation, complicating their interpretation; for instance the major source of plasma NGAL may be neutrophils in normal conditions and during systemic infection, but can be kidney derived during AKI.Citation12
Thus, markers of tubular injury may specifically detect some patients with early AKI and relatively preserved GFR, while markers of GFR changes may detect hemodynamically mediated decreases in glomerular filtration rate in the absence of significant tubular injury. Logically, therefore, examination of multiple biomarkers may inform us not only of the likely occurrence of AKI, but its nature, extent and pathogenesis.
Accordingly, we examined the diagnostic utility of a panel of six biomarkers measured at baseline and at two post-operative time-points in a single-centre randomized pilot controlled trial conducted in higher-risk patients undergoing surgery involving CPB.Citation13 We hypothesized that some combinations of biomarkers would provide better diagnostic accuracy than either alone and that these combinations might involve biomarkers that reflect differing aspects and phases of the pathogenesis of AKI in this population.
Materials and methods
Patient population
Samples were obtained from 93 patients enrolled in the Cardiopulmonary bypass, Renal injury and Atorvastatin Trial (CREAT—Clinicaltrials.Gov Nct00910221), a single-centre randomized controlled trial of peri- and post-operative atorvastatin versus placebo for the prevention of AKI in higher risk patients undergoing CPB. This study was approved by Human Research Ethics Committee of the Austin Hospital. In this study, statin therapy did not influence the incidence of post-operative AKI or biomarkers of renal injury.Citation13,Citation14 Renal function was monitored for 5 days post-operatively. Samples of serum and urine were obtained pre-operatively, immediately on return to ICU after surgery (mean time of 4.50 h; 3.58–9.13 h after CPB) and 24 h after surgery. Of the 100 patients randomized, five withdrew or had surgery rescheduled and in two patients, full sets of serum and urine were not collected for clinical reasons, so that 93 complete sets of urine samples were available for analysis. Demographic and clinical data were collected pre-operatively and over the first 24 h post-surgery. Serum creatinine was measured pre-operatively and daily for 5 days after surgery. AKI was defined using the creatinine criteria of the Risk, Injury, Failure, Loss, End-stage kidney disease (RIFLE) consensus definition of AKI.Citation15 As data on hourly urine output were not available for the complete study period, and in keeping with previous studies of CPB-associated AKI,Citation16,Citation17 urine output definitions in the RIFLE criteria were not employed. Primary definition of AKI was the occurrence of RIFLE Class R or greater (a >50% rise in creatinine from baseline) in the five post-operative days. Secondary biomarker analysis for RIFLE-Injury or greater and a composite of need for renal replacement therapy or death was also conducted.
Biomarkers measurements
Samples for biomarker analysis were stored at −70 °C until the end of the study period and dispatched on dry ice for analysis. Co-investigators performing biomarker assays were blinded to patient details and AKI classification. We assessed four biomarkers of tubular injury: urinary α and π glutathione S-transferases (α-GST and π-GST),Citation17–19 urinary L-type fatty acid-binding protein (l-FABP)Citation20 and urinary NGAL,Citation5,Citation21,Citation22 and two biomarkers that potentially reflect changes in glomerular filtration: urinary hepcidinCitation23–25 and serum CysC.Citation11 We have reported on the utility of urinary hepcidin and NGAL for diagnosis of AKI in this study cohort previously.Citation13,Citation14 α-GST and π-GST were measured by enzyme immunoassay (Argutus Medical, Ltd., Dublin, Ireland)Citation26 and expressed in ng/mL. For urinary L-FAB all samples were assayed using a sandwich ELISACitation27 and reported in ng/mL. Urinary NGAL was measured by enzyme-linked immunosorbent assayCitation7 and reported in ng/mL. Urinary hepcidin was measured by competitive enzyme-linked immunoassay (C-ELISA) as previously described at Intrinsic LifeSciences, LLC (La Jolla, CA) and reported in ng/mL. Serum CysC was measured using nephelometric technology on a Beckman Image Analyzer (Beckman Coulter, Brea, CA) and reported in mg/L. Serum and urinary creatinine was measured using the modified Jaffe method standardized by isotope dilution mass spectroscopy. Urinary biomarkers were assessed both with and without normalization against urinary creatinine concentration to control for urinary dilution where recommended.
Statistical analysis
Receiver–operator characteristic (ROC) analysis, logistic regression analysis, Net Reclassification Index (NRI) calculation and decision tree analysis were performed using R: A language and environment for statistical computing (R Development Core Team, R Foundation for Statistical Computing, Vienna, Austria. http://www.R-project.org) utilizing the packages pROC,Citation28 rpart and rms (http://biostat.mc.vanderbilt.edu/rms).
Categorical data were reported as percentages, and compared using Fisher’s exact test. Continuous data were reported as median with inter-quartile range (IQR) and compared using the Mann–Whitney U test. For comparisons, statistical significance was denoted by two sided p values of < 0.05, adjustment for multiple comparisons was performed using the Bonferroni correction.
The ability of biomarkers to predict AKI was assessed by plotting ROC curves and reported as area under the curve (AUC) with 95% confidence intervalsCitation29 and p value for significance deviation from the null model AUC of 0.5. ROC curve optimal cut-off values for diagnosis, for curves with a statistically significant AUC, were defined as the point which maximized the Youden index, defined as: sensitivity + specificity−1.Citation30 Biomarkers that were associated significantly differed between No AKI and RIFLE R or greater AKI on univariate comparison after correction for multiple comparisons were considered in combination. When assessing combinations NGAL and Hepcidin were considered normalized to urinary creatinine. Combinations of biomarkers were screened by multiple logistic regression analysis and considered significant if the p value for the least significant biomarker reached significance level after correction for multiple comparisons. Predictive models for significant biomarker combinations were then derived from the optimal weighted linear combination of the two biomarker measurements (see Supplementary material), an approach that makes no assumptions about the distributions of sample data.Citation31 Assessment of the statistical difference between paired ROC curves for optimal combination of biomarkers and individual biomarkers was performed using DeLong’s test for two correlated ROC curvesCitation32 with a one-tail comparison of ROC curves. For significant biomarker combinations, the enhancement predictive ability after addition of a second biomarker was assessed by calculation of the NRI and Integrated Discrimination Improvement (IDI).Citation33,Citation34 In addition, the ROC curve optimal cut-off for AKI was assessed against the cut-off of the best individual biomarker by calculation of the two-category NRI. Finally all individual biomarkers significantly predictive of AKI univariate analysis were considered in a classification and regression tree (CART) analysis for combined prediction of AKI. The CART method involves the segregation of different values of classification variables through a decision tree composed of progressive binary splits and has been applied to risk prediction in critical illness.Citation35 To avoid over-fitting, the tree was pruned to minimize the cross-validated error and risk of AKI was calculated for each of the terminal nodes in the CART to generate the risk stratification model.
Results
Patient characteristics
Of the patients in this study, 25 of 93 developed AKI as defined by RIFLE ≥ R (27%). Fourteen patients developed RIFLE ≥ I AKI (15%) and 10 RIFLE F (9.3%). Five patients received renal replacement therapy (RRT) and two died in hospital (one received RRT and died). Of the 25 patients with RIFLE-R or greater AKI, 16 had achieved RIFLE-R by creatinine criteria (50% rise in serum creatinine) by post-operative day 1. However, only 8 of 14 patients going on to develop RIFLE I or F and only 5 of 10 developing RIFLE F satisfied creatinine criteria for RIFLE-R on post-operative day 1. Patient characteristics are summarized in .
Table 1. Patient characteristics.
Individual biomarkers
Individual biomarkers performance is provided in . No biomarker was significantly associated with AKI when measured pre-operatively (). All urinary biomarkers rose significantly after CPB both in patients who developed AKI and those that did not. Immediately after surgery, urinary NGAL, urinary NGAL:Cr ratio, urinary π-GST, urinary L-FAB and serum CysC were associated with statistically significant ROC-AUC’s (). The best ROC-AUC was for π-GST at 0.75. At the 24 h time-point, urinary L-FAB and π-GST were not associated with AKI; however higher urinary NGAL, NGAL:Cr ratio and serum CysC remained associated with AKI, while lower urinary hepcidin and hepcidin:creatinine ratios were significantly associated with AKI. Again the best AUC was 0.77 for the hepcidin:Cr ratio at 24 h.
Table 2. ROC AUC analysis for a panel of biomarkers of AKI-risk.
Most biomarkers showed lesser ability to predict RIFLE I or F (Supplemental Table 1). However, urinary hepcidin at 24 h and serum CysC at 24 h were associated with increased ability to predict more severe AKI (AUC 0.84 and 0.80, respectively). Due to the small number of patients with RIFLE I this category was not considered separately. When considering patients who required RRT and/or died in hospital (6/93), urinary hepcidin at 24 h, urinary NGAL at 24 h and serum CysC at 24 h best predicted these outcomes during ICU admission with AUCs of 0.8–0.91, however, the number of outcomes was small and confidence intervals wide (Supplemental Table 2).
Biomarker combinations
Post-operative urinary NGAL:Cr ratio and urinary π-GST, and 24 h urinary hepcidin:Cr ratio and serum CysC significantly differed between AKI and no-AKI after correction for multiple comparison () and were considered in combination. Three combinations were significant: urinary NGAL:Cr post-operatively with Hepcidin:Cr ratio at 24 h (AUC 0.84, p = 0.03); urinary π-GST post-operatively with urinary Hepcidin:Cr ratio at 24 h (AUC 0.86, p = 0.01) and urinary π-GST post-operatively with serum CysC at 24 h (AUC 0.83, p = 0.03) (Supplementary Table 3). ROC curves for the three best combinations of biomarkers NGAL:Cr (post-op) − 1.32 × Hepcidin:Cr (24 h); π-GST (post-op) − 0.03 × Hepcidin:Cr (24 h) and π-GST (post-op) + 87.35 × CysC (24 h) are shown ().
Figure 1. ROC curves for three AKI biomarker combinations with statistically significant increase in AUC. (Panel A) urine π-GST post-op and serum Cystatin-c at 24 h; (Panel B) urine π-GST post-op and urine Hepcidin:Creatinine at 24 h; (Panel C) urine NGAL:Creatinine post-op and urine Hepcidin:Creatinine at 24 h. We considered linear combinations of biomarkers in the form: C = B1 + α × B2 and determined the value of α (range −∞ to +∞) that provided maximal ROC-AUC for the ability of C to predict AKI. Only combinations of structural biomarkers (NGAL, π-GST) and filtered substances (Hepcidin, CysC) demonstrated statistical significance suggesting markers assessing different aspects of the pathophysiology of AKI may have greater combined diagnostic value.
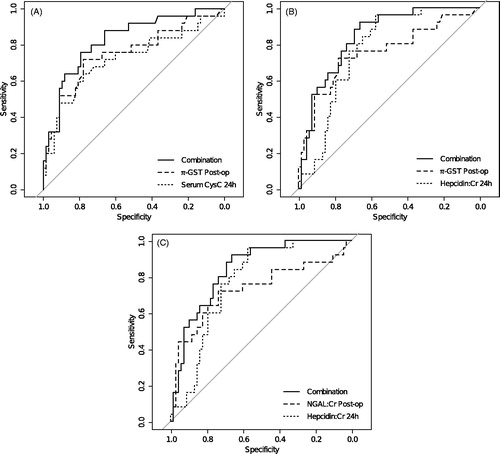
Biomarker combinations demonstrated increased discrimination of AKI by uncategorized NRI against the prediction provided by the best individual biomarker in the combination (); for combinations involving 24 h urinary hepcidin, this was predominantly by enhancement of the ability to exclude AKI (significant NRI for non-events only). While proportion of patients with probability of AKI reclassified in the correct direction appears good (NRIs 0.45–1.02 out of a maximum 2.00), the absolute change in probability was low with IDI ranging from 0.06 to 0.16 for biomarker combinations (). Furthermore, when the clinical utility of the combinations was assessed by examining ability to categorize higher or lower risk of AKI using the optimal cut-off value of the combination compared against the cut-off value of the best individual biomarker, the two-category NRI was not significantly different from zero.
Table 3. Linear combinations of biomarkers and decision tree analysis.
When we assessed the predictive ability of all biomarkers in CART analysis, only NGAL:Cr post-operatively and Hep:Cr at 24 h remained in the final pruned decision tree (). Terminal nodes regression tree identified high, medium and low risk of AKI groups in our cohort and in particular enabled classification of a high risk group immediately post-operatively and a very low risk group at 24 h after surgery (). However reducing the decision tree to binary classification (high & intermediate vs. low risk) resulted in sensitivity and specificity similar to that at cut-offs of the best linear combinations of biomarkers and non-significant NRI compared to Hepcidin:Cr 24 h alone (, ).
Figure 2. Classification and Regression Tree (CART) analysis for biomarkers in combination. All biomarkers were included but only NGAL:Cr post-op and Hepcidin:Cr at 24 h remained in the final model. Terminal nodes identified high, medium and low risk groups for AKI. This model allows early identification of a high-risk group immediately post-operatively and then a low risk classification at 24 h.
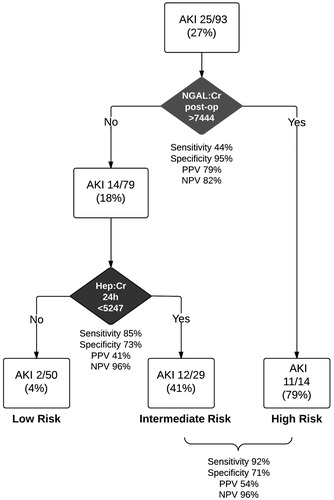
Discussion
Summary of findings
Individual biomarkers alone had only fair ability to predict RIFLE-R defined AKI after cardiopulmonary bypass. However, some markers (hepcidin and CysC) performed better at predicting more severe AKI (RIFLE ≥ I). Certain pairs of biomarkers (urinary hepcidin at 24 h or serum CysC at 24 h combined with urinary NGAL at 6 h or urinary π-GST at 6 h) produced significantly improved combined ROC-AUCs and achieved values above 0.8. The best combinations involved one biomarker of tubular injury (NGAL or π-GST) and one biomarker glomerular filtration rate (GFR) (Hepcidin or CysC) and/or one biomarker measured early, and one later in the post-operative course. This suggests combination of markers of different aspects and stages in the pathophysiology of AKI improves prediction. However, combinations did not significantly improve reclassification of risk category. CART analysis identified a higher cut-off for 6 h NGAL:Cr as defining a high risk of AKI while, in those without early elevated NGAL, a higher level of hepcidin at 24 h was effective at excluding AKI, suggesting that CART analysis has the potential for more efficient and clinically applicable diagnostic modeling.
Relationship to previous biomarker research
Moderate diagnostic performance of urinary NGAL and l-FABP in this cohort is in keeping with previous studies in similar groups of adults after CPB.Citation36,Citation37 Urinary NGAL remained associated with AKI at the 24 h time-point, in keeping with reported performance an older patient population and associated chronic kidney disease.Citation38 Results regarding hepcidin changes and AKI in this cohort confirmed in two other studies of post-CPB AKI.Citation39,Citation40 Finally, CysC has been shown to predict CPB-AKI very early in the post-operative courseCitation41 with similar predictive value to that shown in this study.
Combinations of AKI biomarkers have been described in a several publications.Citation42–44 Han et al.Citation43 showed that a combination of proximal tubular injury biomarkers kidney injury molecule 1 (KIM-1) and N-acetyl-β-d-glucosaminidase (NAG) had no additive ability to predict AKI in children undergoing CPB. The same authors demonstrated that combination of three biomarkers (KIM-1, NAG and NGAL) was significantly improved prediction or early AKI at 3 h after cardiac surgery in the however, there was no added value for diagnosis of late AKI (>24 h).Citation44 A combination of l-FABP and NAG at 4 h after surgery was associated with an improved ROC-AUC for the prediction of AKI and addition to a clinical risk prediction model produced a further significant increase in AUC.Citation42 Most recently the ability of 32 potential urinary AKI biomarkers to predict worsening of AKI or death, was studied in 95 patients with mild AKI after cardiac surgery. In this study a combination of KIM-1 (a tubular damage marker) and IL-18 (an inflammatory mediator) was most predictive of death or advanced AKI.Citation45
Strengths and limitations
As a small single centre study conclusions regarding clinical risk classification for AKI are limited. However, it is similar in size to other examining multiple combinations of AKI biomarkers and makes important points about the biological nature of complementary biomarkers and the potential disconnect between statistical and clinically significant improvement in risk prediction. We employed a wide panel of candidate biomarkers and measured biomarkers at two post-operative time points. Predictive ability of biomarkers was only fair, but was comparable to many studies in similar patient populations, including many older patients with patients with CKD. This study was restricted to post-CPB AKI and findings may not be generalizable. However, post-CPB AKI is a clinically relevant setting for the investigation of biomarkers of AKI. We only used creatinine-normalized values for urinary biomarkers where recommended by the developers. However, normalization did not significantly improve ROC-AUC of individual biomarkers and the validity of normalization against urinary creatinine has been questioned.Citation46,Citation47 Our clinical trial patient population was pre-selected for high risk of AKI this provided a high event rate to permit analysis of biomarkers in combination in a small overall sample; however as patients were selected for AKI risk factors it was not designed to permit analysis of biomarkers in combination with clinical risk prediction models.
Statistical analysis of combined diagnostic predictors is complex. Our analysis is strengthened by use of a robust method to combine biomarkers, weighted linear combination. Use of an uncategorized NRI has been criticized as over-sensitive and self-fulfilling.Citation48 However, at least it is likely to be an indicator that certain combinations of biomarkers provide complementary information and thus potential insights into the pathogenesis of AKI. Moreover, this analysis was tempered by examination of the two-category NRI, a better measure of clinical utility. CART models can capture non-linearity much more easily than regression models, are very well suited to predictive modeling and are easy to interpret, however they are prone to over-fitting and results can be variable depending on the dataset used to develop the tree. To address this concern our model was subject to pruning, a form of internal cross-validation to minimize over-fitting. The model performed similarly to linear combination of predictors when considered as a binary outcome, supporting our primary finding with an alternative methodology. Any model developed from a single sample set does require external validation and the CART model is presented to highlight the potential of this methodology to develop more clinically applicable models for examining multiple biomarkers and/or clinical risk factors in combination and to support our primary analysis.
Finally while certain biomarker combinations resulted in better assignment of risk of AKI practical ability to better categorize groups high and low risk of AKI did not eventuate. However, serum creatinine has significant limitations as a gold standard for diagnosis of renal tubular injury due to alterations in creatinine production, volume of distribution in acute systemic illness and the indirect relationships between tubular injury, GFR changes and alteration in serum creatinine. An imprecise gold standard will intrinsically limit the performance of even the best biomarkersCitation49 and thus might prevent meaningful improvement of prediction when they are examined in combination. One way to escape this limitation is to examine creatinine independent outcomes such as need for RRT and/or mortality.Citation50,Citation51 In this study some individual biomarkers (urinary hepcidin at 24 h, urinary NGAL at 24 h and serum CysC at 24 h) did predict need for RRT or death, however as the event rate was small statistical analysis of combinations was not feasible.
Conclusions
AKI biomarkers may aid in the early diagnosis of AKI, however performance in this groups of high-risk adult patients was, at best, fair. Combinations of biomarkers are able to increase diagnostic discrimination, but this may not result in significantly better reclassification of risk of AKI when applied as a simple diagnostic cut-off. Biomarkers that indicate tubular injury and those that may be related to functional changes in GFR may be most informative in combination and should be the target of future research.Citation52
Supplementary material available online
Supplementary Tables 1–3
Biomarkersupp
Download MS Word (257.5 KB)Declaration of interest
This study has been supported by Austin Health ICU Research Fund. Dr MW is a shareholder, President and CEO of Intrinsic LifeSciences, developer and distributor of an ELISA assay for hepcidin. Dr VA is a shareholder and officer of Intrinsic LifeSciences. Dr JRP and Professor RB are named in a US patent in conjunction with Dr MW and Prof PD is a co-inventor on NGAL patents for the diagnosis of acute kidney injury. SB, PD, JP and RB have received consulting/speaking fees from Alere Inc. RB has received consulting fees from Abbot Diagnostics Inc. JRP is responsible for statistical design and analysis.
References
- Chertow GM, Burdick E, Honour M, Bonventre JV, Bates DW. Acute kidney injury, mortality, length of stay, and costs in hospitalized patients. J Am Soc Nephrol. 2005;16(11):3365–3370
- Uchino S. Creatinine. Curr Opin Crit Care. 2010;16(6):562–567
- Doi K, Yuen PST, Eisner C, et al. Reduced production of creatinine limits its use as marker of kidney injury in sepsis. J Am Soc Nephrol. 2009;20(6):1217–1221
- Endre ZH, Pickering JW, Walker RJ. Clearance and beyond: The complementary roles of GFR measurement and injury biomarkers in acute kidney injury (AKI). Am J Physiol Renal Physiol. 2011;301(4):F697–F707
- Mishra J, Ma Q, Prada A, et al. Identification of neutrophil gelatinase-associated lipocalin as a novel early urinary biomarker for ischemic renal injury. J Am Soc Nephrol. 2003;14(10):2534–2543
- Ichimura T, Bonventre JV, Bailly V, et al. Kidney injury molecule-1 (KIM-1), a putative epithelial cell adhesion molecule containing a novel immunoglobulin domain, is up-regulated in renal cells after injury. J Biol Chem. 1998;273(7):4135–4142
- Mishra J, Dent C, Tarabishi R, et al. Neutrophil gelatinase-associated lipocalin (NGAL) as a biomarker for acute renal injury after cardiac surgery. Lancet. 2005;365(9466):1231–1238
- McIlroy DR, Wagener G, Lee HT. Neutrophil gelatinase-associated lipocalin and acute kidney injury after cardiac surgery: The effect of baseline renal function on diagnostic performance. Clin J Am Soc Nephrol. 2010;5(2):211–219
- Glassford NJ, Schneider AG, Xu S, et al. The nature and discriminatory value of urinary neutrophil gelatinase-associated lipocalin in critically ill patients at risk of acute kidney injury. Intensive Care Med. 2013;39(10):1714–1724
- Paragas N, Qiu A, Zhang Q, et al. The Ngal reporter mouse detects the response of the kidney to injury in real time. Nat Med. 2011;17(2):216–222
- Bagshaw SM, Bellomo R. Cystatin C in acute kidney injury. Curr Opin Crit Care. 2010;16(6):533–539
- Kanda J, Mori K, Kawabata H, et al. An AKI biomarker lipocalin 2 in the blood derives from the kidney in renal injury but from neutrophils in normal and infected conditions. Clin Exp Nephrol. 2014 Mar 6. [Epub ahead of print]. doi 10.1007/s10157-014-0952-7
- Prowle JR, Calzavacca P, Licari E, et al. Pilot double-blind, randomized controlled trial of short-term atorvastatin for prevention of acute kidney injury after cardiac surgery. Nephrology (Carlton). 2012;17(3):215–224
- Prowle JR, Ostland V, Calzavacca P, et al. Greater increase in urinary hepcidin predicts protection from acute kidney injury after cardiopulmonary bypass. Nephrol Dial Transplant. 2012;27(2):595–602
- Bellomo R, Ronco C, Kellum JA, Mehta RL, Palevsky P, Acute Dialysis Quality Initiative workgroup. Acute renal failure – definition, outcome measures, animal models, fluid therapy and information technology needs: The Second International Consensus Conference of the Acute Dialysis Quality Initiative (ADQI) Group. Crit Care. 2004;8(4):R204–R212
- Herget-Rosenthal S, Marggraf G, Husing J, et al. Early detection of acute renal failure by serum cystatin C. Kidney Int. 2004;66(3):1115–1122
- Koyner JL, Vaidya VS, Bennett MR, et al. Urinary biomarkers in the clinical prognosis and early detection of acute kidney injury. Clin J Am Soc Nephrol. 2010;5(12):2154–2165
- Walshe CM, Odejayi F, Ng S, Marsh B. Urinary glutathione S-transferase as an early marker for renal dysfunction in patients admitted to intensive care with sepsis. Crit Care Resusc. 2009;11(3):204–209
- McMahon BA, Koyner JL, Murray PT. Urinary glutathione S-transferases in the pathogenesis and diagnostic evaluation of acute kidney injury following cardiac surgery: a critical review. Curr Opin Crit Care. 2010;16(6):550–555
- Doi K, Noiri E, Sugaya T. Urinary l-type fatty acid-binding protein as a new renal biomarker in critical care. Curr Opin Crit Care. 2010;16(6):545–549
- Mishra J, Mori K, Ma Q, Kelly C, Barasch J, Devarajan P. Neutrophil gelatinase-associated lipocalin: a novel early urinary biomarker for cisplatin nephrotoxicity. Am J Nephrol. 2004;24(3):307–315
- Mori K, Lee HT, Rapoport D, et al. Endocytic delivery of lipocalin–siderophore–iron complex rescues the kidney from ischemia-reperfusion injury. J Clin Invest. 2005;115(3):610–621
- Ho J, Lucy M, Krokhin O, et al. Mass spectrometry-based proteomic analysis of urine in acute kidney injury following cardiopulmonary bypass: A nested case-control study. Am J Kidney Dis. 2009;53(4):584–595
- Prowle JR, Westerman M, Bellomo R. Urinary hepcidin: an inverse biomarker of acute kidney injury after cardiopulmonary bypass? Curr Opin Crit Care. 2010;16(6):540–544
- Haase M, Bellomo R, Haase-Fielitz A. Novel biomarkers, oxidative stress, and the role of labile iron toxicity in cardiopulmonary bypass-associated acute kidney injury. J Am Coll Cardiol. 2010;55(19):2024–2033
- Westhuyzen J, Endre ZH, Reece G, Reith DM, Saltissi D, Morgan TJ. Measurement of tubular enzymuria facilitates early detection of acute renal impairment in the intensive care unit. Nephrol Dial Transplant. 2003;18(3):543–551
- Ferguson MA, Vaidya VS, Waikar SS, et al. Urinary liver-type fatty acid-binding protein predicts adverse outcomes in acute kidney injury. Kidney Int. 2010;77(8):708–714
- Robin X, Turck N, Hainard A, et al. pROC: An open-source package for R and S+ to analyze and compare ROC curves. BMC Bioinformatics. 2011;12:77
- Hanley JA, McNeil BJ. The meaning and use of the area under a receiver operating characteristic (ROC) curve. Radiology. 1982;143(1):29–36
- Youden WJ. Index for rating diagnostic tests. Cancer. 1950;3(1):32–35
- Pepe MS, Cai T, Longton G. Combining predictors for classification using the area under the receiver operating characteristic curve. Biometrics. 2006;62(1):221–229
- DeLong ER, DeLong DM, Clarke-Pearson DL. Comparing the areas under two or more correlated receiver operating characteristic curves: A nonparametric approach. Biometrics. 1988;44(3):837–845
- Pencina MJ, D'Agostino RB Sr, D'Agostino RB Jr, Vasan RS. Evaluating the added predictive ability of a new marker: From area under the ROC curve to reclassification and beyond. Stat Med. 2008;27(2):157–172; discussion 207–112
- Pencina MJ, D'Agostino RB Sr, Steyerberg EW. Extensions of net reclassification improvement calculations to measure usefulness of new biomarkers. Stat Med. 2011;30(1):11–21
- Wong HR, Lindsell CJ, Pettila V, et al. A multibiomarker-based outcome risk stratification model for adult septic shock. Crit Care Med. 2014;42(4):781–789
- Haase M, Bellomo R, Devarajan P, Schlattmann P, Haase-Fielitz A, Group NM-aI. Accuracy of neutrophil gelatinase-associated lipocalin (NGAL) in diagnosis and prognosis in acute kidney injury: A systematic review and meta-analysis. Am J Kidney Dis. 2009;54(6):1012–1024
- Matsui K, Kamijo-Ikemori A, Sugaya T, Yasuda T, Kimura K. Usefulness of urinary biomarkers in early detection of acute kidney injury after cardiac surgery in adults. Circ J. 2012;76(1):213–220
- Endre ZH, Pickering JW, Walker RJ, et al. Improved performance of urinary biomarkers of acute kidney injury in the critically ill by stratification for injury duration and baseline renal function. Kidney Int. 2011;79(10):1119–1130
- Haase-Fielitz A, Mertens PR, Plass M, et al. Urine hepcidin has additive value in ruling out cardiopulmonary bypass-associated acute kidney injury – An observational cohort study. Crit Care. 2011;15(4):R186
- Ho J, Reslerova M, Gali B, et al. Urinary hepcidin-25 and risk of acute kidney injury following cardiopulmonary bypass. Clin J Am Soc Nephrol. 2011;6(10):2340–2346
- Wald R, Liangos O, Perianayagam MC, et al. Plasma cystatin C and acute kidney injury after cardiopulmonary bypass. Clin J Am Soc Nephrol. 2010;5(8):1373–1379
- Katagiri D, Doi K, Honda K, et al. Combination of two urinary biomarkers predicts acute kidney injury after adult cardiac surgery. Ann Thorac Surg. 2012;93(2):577–583
- Han WK, Waikar SS, Johnson A, et al. Urinary biomarkers in the early diagnosis of acute kidney injury. Kidney Int. 2008;73(7):863–869
- Han WK, Wagener G, Zhu Y, Wang S, Lee HT. Urinary biomarkers in the early detection of acute kidney injury after cardiac surgery. Clin J Am Soc Nephrol. 2009;4(5):873–882
- Arthur JM, Hill EG, Alge JL, et al. Evaluation of 32 urine biomarkers to predict the progression of acute kidney injury after cardiac surgery. Kidney Int. 2014;85(2):431–438
- Ralib AM, Pickering JW, Shaw GM, et al. Test characteristics of urinary biomarkers depend on quantitation method in acute kidney injury. J Am Soc Nephrol. 2012;23(2):322–333
- Waikar SS, Sabbisetti VS, Bonventre JV. Normalization of urinary biomarkers to creatinine during changes in glomerular filtration rate. Kidney Int. 2010;78(5):486–494
- Pepe MS. Problems with risk reclassification methods for evaluating prediction models. Am J Epidemiol. 2011;173(11):1327–1335
- Waikar SS, Betensky RA, Emerson SC, Bonventre JV. Imperfect gold standards for kidney injury biomarker evaluation. J Am Soc Nephrol. 2012;23(1):13–21
- Haase M, Devarajan P, Haase-Fielitz A, et al. The outcome of neutrophil gelatinase-associated lipocalin-positive subclinical acute kidney injury: A multicenter pooled analysis of prospective studies. J Am Coll Cardiol. 2011;57(17):1752–1761
- Nickolas TL, Schmidt-Ott KM, Canetta P, et al. Diagnostic and prognostic stratification in the emergency department using urinary biomarkers of nephron damage: A multicenter prospective cohort study. J Am Coll Cardiol. 2012;59(3):246–255
- McCullough PA, Shaw AD, Haase M, et al. Diagnosis of acute kidney injury using functional and injury biomarkers: Workgroup statements from the tenth Acute Dialysis Quality Initiative Consensus Conference. Contrib Nephrol. 2013;182:13–29