Abstract
Background: The purpose of this study was to evaluate the associations of excess weight (EW) and/or chronic kidney disease (CKD) with cardiovascular autonomic neuropathy (CAN), and to detect the extent to which interaction of EW and CKD has on the outcome in a Chinese sample. Method: We conducted a large-scale, population-based study to analyze the association and interaction of the two factors on CAN in a sample of 2092 Chinese people. Multiple linear regression analysis to include the two main factors and its interaction were employed to detect these relationships. Relative excess risk due to interaction (RERI), the proportion attributable to interaction (AP) and the synergy index (S) were used to estimate the effect of interaction on an additive scale can. Result: Multivariable logistic regression (MLR) indicated that body mass index (BMI) was independently associated with CAN (p = 0.006). In addition, a significant positive interaction between BMI and CKD on CAN was estimated (p = 0.042, RETI = 0.473, 95% CI: 0.0615–0.884, AP = 0.203, 95% CI: −0.055 to 0.461 and S = 1.550, 95% CI: 0.667–2.589). Conclusion: Our findings suggest that BMI is independently associated with CAN and offer evidence to support the hypothesis that excess weight and CKD have significant positive interactions on CAN.
Introduction
Excess weight, such as overweight or obesity, is a medical condition in which excess body fat has accumulated to the extent that it may have a negative effect on health, contributing to increased health problems and/or reduced life expectancy.Citation1 Excess weight has reached epidemic proportions globally, with more than 1 billion adults being either overweight or obese in 2003.Citation2 In 2013 this increased to more than 2 billion.Citation2 Increases have been observed across all age groups. Obesity increases the likelihood of various diseases, particularly heart disease, type 2 diabetes, certain types of cancer and osteoarthritis.Citation1 The body mass index (BMI) is a measure of relative weight based on an individual’s mass and height, which was used to provide a simple numeric measure of a person’s thickness or thinness. Chronic kidney disease (CKD) is also one of the most serious public health problems. The increasing prevalence of this disease in developed and developing countries has led to a global epidemic. CKD may also be identified when it leads to one of its recognized complications, such as cardiovascular disease, anemia or pericarditis.Citation3,Citation4 The prevalence of cardiovascular autonomic neuropathy (CAN) is rapidly growing in all populations worldwide, particularly in the developing world.Citation5 Individuals with previously undiagnosed CAN have an unfavorable cardiovascular risk profile, especially in terms of sudden death, indicating a higher risk of cardiovascular disease.Citation5 In general, this disease was a major factor in the cardiovascular complications of diabetes mellitus (DM).Citation6 Recent studies have indicated that CAN is associated with many other majority segments of the general population, such as patients with abnormal metabolism status and hypertension (HTN).Citation5,Citation7,Citation8
Previous studies indicated that abnormal CA function or low heart rate variability (HRV) was uncovered in patients with overweight or obesity.Citation7,Citation9 Studies also provided evidence that CAN is common in persons with impaired glucose tolerance; and obesity seems to play an important role in the early pathogenesis of CAN.Citation9,Citation10 In addition, our previous studies showed that overweight or obesity was associated with abnormal CA function in the Chinese population.Citation11,Citation12 Others studies also provided evidence that CAN or abnormal CA function was independently associated with CKD, albuminuria and glomerular filtration rate (GFR) in diabetic patients.Citation3,Citation4 Moreover, studies indicated that HRV was an independent risk factor for cardiovascular mobility and mortality in type 1 patients with nephropathy.Citation13 In summary, complex relationships were found in excess weight, CKD and abnormal CA functions.Citation3,Citation5,Citation7,Citation9,Citation10,Citation12–15
Generally, physicians pay more attention to the associations of risk factors and outcomes. However, we often neglect how the interaction of risk factors affects outcomes. It is very important to clarify the relationships of risk factors and how they affect diseases. A classic example is the interaction between smoking and asbestos in connection to the risk of lung cancer.Citation16 The term of interaction refers to the situation where the effect of one risk factor on a certain disease outcome is different across strata of another risk factor, or vice versa. This means if interaction between factor 1 and factor 2 is present, factor 1 and factor 2 are not independent in causing a certain disease. If the combined effect of factor 1 and factor 2 is larger (or smaller) than the sum of the individual effects of the two factors, there is interaction on an additive scale or departure from additivity. In clinical practice, interaction should generally be assessed on an additive scale rather than a multiplicative scale.Citation17–20 Interaction on an additive scale can be calculated using relative risks and different measures quantifying this interaction, which have been described, such as the relative excess risk due to interaction (RERI), the proportion attributable to interaction (AP) and the synergy index (S).Citation21 However, there is little known about the interaction of excess weight and CKD as far as how they affect CAN. According to evidence of associations of excess weight and CKD with CAN, we hypothesized that excess weight and CKD will have an interaction effect associated with the progression of CAN. The purpose of this study was to evaluate the associations of BMI and CKD with CAN, and to estimate the extent of the interaction between BMI and CKD on the outcome in the Chinese population.
Methods
Study population
We performed a CAN factor survey carried out in a random sample of the Chinese population. Participants were recruited from rural and urban communities in Shanghai. Survey participants with undiagnosed CAN, aged 30–80 years, were included in this study. A total of 3012 subjects were invited to a screening visit between 2011 and 2012. This study was approved by the Ethics Committee of the Fudan University Hua Shan Hospital, Shanghai, China.
Some subjects were excluded from the study to eliminate potential confounding factors that may have influenced their CA function. Briefly, the exclusion criteria were as follows: (1) history or findings of arrhythmia and hyperthyroidism or hypothyroidism; (2) pregnancy or lactation and/or (3) serious hepatic or renal dysfunctions (GFR <30 mL/min/1.73 m2). Of these subjects, complete baseline data were obtained for 2092 (69.46%) of the participants. Written consent was obtained from all patients before the study.
The subjects were interviewed for the documentation of medical histories, medication and history of smoking habits. Laboratory assessment of cardiovascular disease risk factors was completed, along with standardized examination for HRV. All study subjects underwent a complete clinical baseline characteristics evaluation after an eight-hour fast, which included: (1) history and physical examination; (2) heart rate and blood pressure; (3) fasting serum glucose and insulin and (4) fasting plasma lipids.
Measurement
Systolic and diastolic blood pressure (BP) values were the means of two physician-obtained measurements taken from the left arm of the seated participant. Fasting plasma glucose (FPG) was quantified by the glucose oxidase procedure; HbA1c was measured by ion-exchange, high-performance liquid chromatography (HPLC; Bio-Rad, Hercules, CA). Serum total cholesterol (TC), high-density lipoprotein (HDL) cholesterol triglyceride (TG) levels, serum creatinine (SCr) and uric acid (UA) were measured by an enzymatic method with a chemical analyzer (Hitachi 7600-020, Tokyo, Japan). Low-density lipoprotein (LDL) cholesterol levels were calculated using the Friedewald formula, and creatinine clearance rate (Ccr) was calculated using the Cockcroft–Gault formula. An enzyme-coupled method was used to measure urine creatinine concentration and calculate the urinary albumin/urinary creatinine ratio (Alb/Cr). The most recently advocated formula for calculating the GFR is one developed by the Modification of Diet in Renal Disease Study Group.Citation22 For creatinine in μmol/L: eGFR = 32788 × serum creatinine−1.154 × age−0.203 × (0.742 if female). The day-to-day and inter-assay coefficients of variation at the central laboratory in our hospital for all analyses were between 1% and 3%.
Definition
HTN was defined as BP ≥140/90 mmHg, or a history of hypertension medication. DM was defined by oral glucose tolerance test (OGTT) and either HbAlc ≥6.5% or the use of insulin or hypoglycemic medications. MetS was diagnosed according to the updated National Cholesterol Education Program/Adult Treatment Panel III criteria (WHO Western Pacific Region obesity criteria) in individuals meeting three or more of the following:Citation23 (1) central obesity; (2) TG levels >1.7 mmol/L or specific treatment for this lipid abnormality; (3) HDL cholesterol <1.03 mmol/L in men and <1.29 mmol/L in women or specific treatment for this lipid abnormality; (4) raised BP, systolic BP >130 mmHg or diastolic BP > 85 mmHg or treatment for previously diagnosed HT and (5) raised FPG level >5.6 mmol/L or previously diagnosed type 2 DM.
BMI was calculated with weight in kilograms divided by the square of height in meters. BMI was classified based on the Chinese criteria: normal BMI < 24.0 kg/m2; overweight 24.0 kg/m2 ≤ BMI < 28.0 kg/m2 and obese BMI ≤ 28.0 kg/m2. High FPG was defined as FPG ≥5.6 mmol/L. Center obesity was defined using ethnicity-specific values for waist circumference (WC) of ≥90 cm in men and ≥80 cm in women.Citation23 In this study, total sample was classed to normal BMI group (code 0) and high BMI group (overweight and obesity, code 1).
CKD was defined as the presence of kidney damage or reduced renal function for more than three months, regardless of the diagnosis. The advanced stage of this condition is known as terminal CKD, or end-stage kidney disease, with progressive and irreversible loss of renal function. CKD is classified on the basis of the GFR, as recommended by the US National Kidney Foundation Kidney Disease Outcome Quality Initiative, which provides the basis for the management of this disease. CKD is classified into five stages: stage 1 – kidney damage with normal or increased GFR ≥90 mL/min/1.73 m2; stage 2 – kidney damage with mildly decreased GFR of 60–89 mL/min/1.73 m2; stage 3 – moderately decreased GFR of 30–59 mL/min/1.73 m2; stage 4 – severely decreased GFR of 15–29 mL/min/1.73m2; stage 5 – kidney failure with a GFR <15 mL/min/1.73 m2. In this study, CKD patients with stages 1–3 were available for this association and interaction study. For this analysis, CKD was defined as the presence of albuminuria or an eGFR <60 mL/min/1.73 m2.
The study outcome
Short-term HRV has good reproducibility, and is more practical for application. In our large-scale, population-based study, this test was applied to evaluate CA function. HRV was measured non-invasively by power spectral analysis. Before CA function assessment, participants were to avoid alcohol, smoking and coffee for 24 h to influence their calm and quiet status. Subjects were studied while awake in the supine position after 20 min of rest. Testing times were from 8:00 to 11:00 in the morning. A type-I FDP-1 HRV non-invasive detecting system was used with software version 2.0 (Department of Biomedical Engineering of the Fudan University, Shanghai, China). Electrocardiography, respiratory signals and beat-to-beat blood pressure were continually and simultaneously recorded for 15 min through an electro-sphygmograph transducer (HMX-3C placed on the radial artery of the dominant arm) and an instrument respiration sensor.
Short-term HRV analysis was performed for all subjects using a computer-aided examination and evaluation system for spectral analysis to investigate changes in autonomic regulation. In this study, CAN was diagnosed based on at least two abnormal cardiovascular autonomic reflex test results based on short-term HRV tests.Citation6,Citation24,Citation25
Statistical analysis
Continuous variables were detected whether followed normal distribution using Kolmogorov–Smirnov test. Variables that were not normally distributed were log-transformed to approximate normal distribution for analysis. Results are described as mean ± SD or median, unless stated otherwise. Differences in variables between subjects with normal BMI and high BMI were determined by unpaired t-test. Between groups differences in properties were accessed by χ2 analysis. Univariate logistic regression was performed to determine variables associated with CAN and to estimate confounding factors possibly disturbing the relation of BMI and/or CKD to CAN. Multivariable logistic regression (MLR) to include BMI and CKD was carried out to control potential confounders for determining independent contribution of variables to CAN. For interaction analysis, MLR was conducted to include two main factors and its interaction item to evaluate the interaction effect. Odds ratios (ORs) with 95% confidence intervals (CIs) were calculated for the relative risk of BMI and/or CKD with CAN.
Three parameters of RERI, AP and S were used to estimate measures of interaction on an additive scale. RERI calculated by formula: ORAB − ORAb − ORaB + 1, means part of the total effect is due to interaction, where Ab is exposed to one factor, aB is exposed to another factor and AB is exposed to both factors. AP refers to proportion of the combined effect due to interaction, which is calculated by RERI/ORAB. S is defined as the ratio between combined effect and individual effects, and derived from the formula: (ORAB − 1)/(ORAb − 1 + ORaB − 1). The first bootstrap percentile method was adopted to calculate the CI around the estimate of interaction. From the original data set, 1000 bootstrap samples (with replacement) were taken, each of which was the same size as the original sample. The three parameters of RERI, AP and S were then estimated in each of these new samples and the 95% CI for the three parameters were estimated as the 2.5th and 97.5th percentiles of the resulting bootstrap sampling distribution. Results were analyzed using the Statistical Package for Social Sciences for Windows version 16.0 (SPSS, Chicago, IL). Tests were two-sided and a p-value of <0.05 was considered significant. For interaction analysis, a p-value of <0.05 was also considered to be significant.
Results
Clinical characteristics of subjects
The baseline clinical characteristics of the 2092 subjects are listed in . There were 381 males and 865 females (mean age, 59.94 ± 8.61 years) in the normal BMI group and 308 males and 528 females (mean age, 61.1 ± 8.74 years) in the high BMI group. Subjects with high BMI were significantly older than subjects with normal BMI (p = 0.0031). A significant difference in the ratio of male to female between the two groups was found in our study sample (p = 0.002). There were worse glucose profiles and lipid profiles in subjects with high BMI as compared with subjects with normal BMI (p < 0.05 for all expect TC and LDL levels). However, there was no significant difference in renal function parameters between the two groups (p < 0.05 for all expect UA levels). HR and TP of HRV indices were significantly different between the two groups (p < 0.05 for both). The prevalence of HTN, DM, MetS and CAN was frequent in subjects with high BMI as compared to subjects with high BMI (p < 0.01 for all), while there was no significant difference in the prevalence of CKD between the two groups (p = 0.497).
Table 1. The clinical baseline of subjects.
Univariate and multiple logistic regression analysis for CAN
Univariate logistic regression models to evaluate the association of various clinical factors and CAN were developed to include demographical information, glucose profiles, lipid profiles, parameters of renal function, HRV indices and medical history (). The univariate logistic analyses indicated that age, SBP, DBP, FPG, PBG, FINS, IR, HR, HTN, DM and MetS were significantly associated with CAN (p < 0.05 for all). However, the variables of CKD were not significantly associated with the outcome (p = 0.081).
Table 2. Univariate logistic regression analysis for cardiovascular autonomic neuropathy.
The proportion of CAN was 16.59% and 21.71% in the normal BMI group and the high BMI group, respectively. In subjects with high BMI, the OR for CAN was 1.068 (95% CI: 1.034–1.046, p < 0.001). MLR demonstrated that BMI remained significantly different between the two groups after adjustment for potential confounders (p = 0.006, OR = 1.288, 95% CI: 1.163–1.426, ). There were no significant associations between CKD and CAN by using MLR (p = 0.763, ).
Table 3. Multiple logistic regression analysis to include body mass index and chronic kidney disease for cardiovascular autonomic neuropathy.
MetS and CKD interaction analysis for CAN
MLR models were developed to include the two main factors of BMI and CKD, and its interaction item among them was detected in the MLR model after adjustment for relevant potential confounders (p = 0.038, ORinter = 1.141, and ). The significant interaction on an additive scale was calculated by using RERI = 0.473 (95% CI: 0.062–0.884), A p = 0.203 (95% CI: −0.055 to 0.461) and S = 1.550 (95% CI: 0.667–2.589). In subjects with high BMI, there was no significant difference in the CAN prevalence between subjects with CKD and subjects without CKD (16.30% vs. 18.58%, p = 0.124). However, in subjects with high BMI, the CAN prevalence was frequent in subjects with CKD as compared without CKD (20.97% vs. 30.02% p = 0.015).
Figure 1. Interaction analysis of body mass index (BMI) and chronic kidney disease (CKD) on cardiovascular autonomic neuropathy (CAN). In subjects with normal BMI, the prevalence of CAN was 16.30% and 18.58% in those subjects with non-CKD and CKD, respectively. In subjects with high BMI, the prevalence of CAN was 20.97% and 30.02% in the subjects with non-CKD and CKD, respectively. CKD was defined as the presence of albuminuria or an eGFR <60 mL/min/1.73 m2.
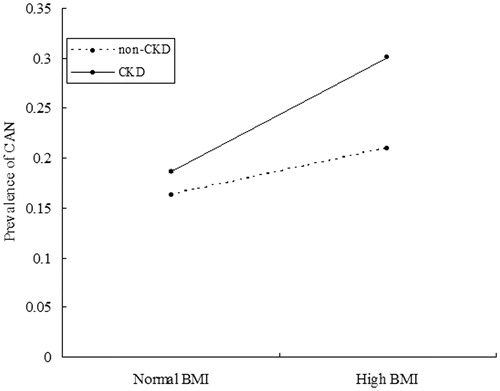
Table 4. The interaction analysis of body mass index and chronic kidney disease on cardiovascular autonomic neuropathy.
Discussion
A large-scale, population-based study was conducted to evaluate the association and interaction of excess weight and CKD on CAN among 2092 participants in the general Chinese population. This sample was an adequate representation of the Chinese population, and the reference values may work similarly well outside the areas studied in China.Citation26,Citation27 Importantly, in a general Chinese population, we first performed an interaction analysis of BMI and CKD for CAN. Physicians must understand the effect of interaction risk factors on outcomes. This is partly because most patients have more than two risk factors for a disease, and we should focus on controlling a modifiable risk factor, such as weight interacting with other factors, to efficiently reduce overall risks for the outcome. In addition, if patients have only one risk factor, we should pay more attention to prevent other risk factors from interacting with that factor to more efficiently prevent progressions of the disease.
Our finding supported the fact that BMI was strongly and independently associated with CAN in the Chinese population. Multiple variable analysis provided evidence to support the finding. These results were consistent with our previous reports and others’ studies that BMI was associated with lower HRV and impaired CAF.Citation14,Citation28–30 Lucini et al. investigated whether overweight might influence autonomic nervous system profile in 103 young subjects to show that overweight is associated to a clear autonomic impairment even in children subjected to an intense aerobic training.Citation31 Laitinen et al. performed a study in 268 individuals to characterize associations between components of MetS and CAN in the Finnish Diabetes Prevention Study cohort,Citation9 and concluded that CAN is common in persons with impaired glucose tolerance and obesity, especially among men, seems to play an important role in the early pathogenesis of CAN. These findings are of particular importance given the direct relationship between excess weight and CAN. Excess weight implied that the multiple complex metabolic reactions involved in glucotoxicity, lipotoxicity and interstitial deposition of triacylglycerol may directly or indirectly impact CA nerves. Furthermore, a large-scale, case-controlled study or cohort study incorporating a more rigorous method of scoring excess weight will be conducted in the future to develop a highly sensitive and specific model that uses BMI information to predict CAN.
Our important finding was a significant positive interaction between BMI and CKD on CAN in our Chinese sample. MLR to include two main factors and its interaction term was performed to detect a significant interaction between BMI and CKD to associate with CAN (p = 0.038). In addition, a positive interaction effect was evaluated by using parameters of RETI > 0, AP > 0 and S > 1, indicating that the combined effect between BMI and CKD on CAN is greater by 47.3% than the sum of the individual effects of the two factors. MLR analysis showed that there was no significant correlation in CKD to CAN in our study sample, suggesting that excess weight may modify CKD to associate with CAN. These findings offered evidence that obese patients with CKD were more susceptible to the influence of the progression of CAN. Lambert et al. conducted a study to evaluate early signs of organ damage and their possible relationships to sympathetic nervous activity in a small sample to suggest that excess weight was associated with subclinical alterations in renal and endothelial function, and that sympathetic activity is closely associated with cardiovascular and renal alterations observed in these individuals.Citation32 Smulders et al. investigated whether impaired CA function was independently associated with albuminuria in subjects with metabolic dysfunction to show that impaired CA function was independently associated with the presence of albuminuria in subjects with impaired glucose tolerance or diabetes.Citation4
Our findings were similar to those studies reporting that CKD was associated with CAN in a special subgroup of subjects with high BMI. These results provided strong evidence that excess weight modifies associations between CKD and the development and progression of CAN. It is biologically plausible that the relationship between CAN and CKD could be mutually influenced in obesity. CKD may contribute to the progression of CAN through the lack of clearance of leptin, which can stimulate the sympathetic nervous system.Citation33,Citation34 The development and progression of CKD might attribute to CAN’s impact on BP and cause hemodynamic changes, which can affect the intraglomerular pressure and the microvasculature surrounding the renal tubules.Citation35,Citation36 As is well known, modification in lifestyle and improvements in metabolic control, such as BP and lipid profiles, can prevent the progression of CAN.
Several limitations of this study warrant comment. Firstly, this study does not cover age groups other than 30–90 years. Secondly, the study data based on a cross-sectional study to perform interaction analysis require a larger sample size and more geographic representations. The interaction results need to be verified by future follow-up studies. Finally, it is important to mention that our study was performed on Chinese individuals, and our findings may not be relevant to people of other ethnicities.
Conclusion
Firstly, our findings suggest that BMI is independently associated with CAN. In addition, our study offers evidence to support that BMI and CKD have positive interactions on CAN. Excess weight may modify CKD to influence the progression of CAN. This observation constitutes evidence that CKD patients to reduce weight or body fat mass may be an extra benefit in the goal of preventing development of CAN, and also provide novel insights into biological functions, which may prove useful in the future.
Declaration of interest
This study was supported by the grant from China National Grant on Science and Technology (grant number: 30570740).
We thank the grant from China National Grant on Science and Technology to support the study.
All authors have no conflicts of interest to declare.
Reference
- Haslam DW, James WP. Obesity. Lancet. 2005;366:1197–1209
- Ng M, Fleming T, Robinson M, et al. Global, regional, and national prevalence of overweight and obesity in children and adults during 1980–2013: A systematic analysis for the Global Burden of Disease Study 2013. Lancet. 2014;384:766–781
- Tahrani AA, Dubb K, Raymond NT, et al. Cardiac autonomic neuropathy predicts renal function decline in patients with type 2 diabetes: A cohort study. Diabetologia. 2014;57:1249–1256
- Smulders YM, Jager A, Gerritsen J, et al. Cardiovascular autonomic function is associated with (micro-)albuminuria in elderly Caucasian sujects with impaired glucose tolerance or type 2 diabetes: The Hoorn Study. Diabetes Care. 2000;23:1369–1374
- Hazari MA, Khan RT, Reddy BR, Hassan M. Cardiovascular autonomic dysfunction in type 2 diabetes mellitus and essential hypertension in a South Indian population. Neurosciences. 2012;17:173–175
- Spallone V, Ziegler D, Freeman R, et al. Cardiovascular autonomic neuropathy in diabetes: Clinical impact, assessment, diagnosis, and management. Diabetes/Metab Res Rev. 2011;27:639--653
- Garruti G, Giampetruzzi F, Vita MG, et al. Links between metabolic syndrome and cardiovascular autonomic dysfunction. Exp Diabetes Res. 2012;2012:615835
- Iodice V, Low DA, Vichayanrat E, Mathias CJ. Cardiovascular autonomic dysfunction in MSA and Parkinson's disease: Similarities and differences. J Neurol Sci. 2011;310:133–138
- Laitinen T, Lindstrom J, Eriksson J, et al. Cardiovascular autonomic dysfunction is associated with central obesity in persons with impaired glucose tolerance. Diabetic Med. 2011;28:699–704
- dos Reis Santos I, Danaga AR, de Carvalho Aguiar I, et al. Cardiovascular risk and mortality in end-stage renal disease patients undergoing dialysis: Sleep study, pulmonary function, respiratory mechanics, upper airway collapsibility, autonomic nervous activity, depression, anxiety, stress and quality of life: A prospective, double blind, randomized controlled clinical trial. BMC Nephrol. 2013;14:215
- Li Z, Tang ZH, Zeng F, Zhou L. Associations between the severity of metabolic syndrome and cardiovascular autonomic function in a Chinese population. J Endocrinol Invest. 2013;36:993–999
- Lu Y, Tang ZH, Zeng F, Li Y, Zhou L. The association and predictive value analysis of metabolic syndrome combined with resting heart rate on cardiovascular autonomic neuropathy in the general Chinese population. Diabetology Metabolic Syndrome. 2013;5:73--80
- Astrup AS, Tarnow L, Rossing P, et al. Cardiac autonomic neuropathy predicts cardiovascular morbidity and mortality in type 1 diabetic patients with diabetic nephropathy. Diabetes Care. 2006;29:334–339
- Assoumou HG, Pichot V, Barthelemy JC, et al. Metabolic syndrome and short-term and long-term heart rate variability in elderly free of clinical cardiovascular disease: The PROOF study. Rejuvenation Res. 2010;13:653–663
- Lakka HM, Laaksonen DE, Lakka TA, et al. The metabolic syndrome and total and cardiovascular disease mortality in middle-aged men. JAMA. 2002;288:2709–2716
- Schabath MB, Spitz MR, Delclos GL, et al. Association between asbestos exposure, cigarette smoking, myeloperoxidase (MPO) genotypes, and lung cancer risk. Am J Industrial Med. 2002;42:29–37
- Andersson T, Alfredsson L, Kallberg H, Zdravkovic S, Ahlbom A. Calculating measures of biological interaction. Eur J Epidemiol. 2005;20:575–579
- Rothman KJ, Greenland S, Walker AM. Concepts of interaction. Am J Epidemiol. 1980;112:467–470
- Hallqvist J, Ahlbom A, Diderichsen F, Reuterwall C. How to evaluate interaction between causes: A review of practices in cardiovascular epidemiology. J Intern Med. 1996;239:377–382
- VanderWeele TJ, Robins JM. The identification of synergism in the sufficient-component-cause framework. Epidemiology. 2007;18:329–339
- Assmann SF, Hosmer DW, Lemeshow S, Mundt KA. Confidence intervals for measures of interaction. Epidemiology. 1996;7:286–290
- Levey AS, Bosch JP, Lewis JB, et al. A more accurate method to estimate glomerular filtration rate from serum creatinine: A new prediction equation. Modification of Diet in Renal Disease Study Group. Ann Inter Med. 1999;130:461–470
- Grundy SM, Hansen B, Smith SC Jr, Cleeman JI, Kahn RA. Clinical management of metabolic syndrome: Report of the American Heart Association/National Heart, Lung, and Blood Institute/American Diabetes Association conference on scientific issues related to management. Circulation. 2004;109:551–556
- Zeng F, Tang ZH, Li Z, Yu X, Zhou L. Normative reference of short-term heart rate variability and estimation of cardiovascular autonomic neuropathy prevalence in Chinese people. J Endocrinol Invest. 2014;37:385--391
- Tang ZH, Zeng F, Yu X, Zhou L. Bayesian estimation of cardiovascular autonomic neuropathy diagnostic test based on baroreflex sensitivity in the absence of a gold standard. Int J Cardiol. 2014;171:e78–e80
- Bian RW, Lou QL, Gu LB, et al. Delayed gastric emptying is related to cardiovascular autonomic neuropathy in Chinese patients with type 2 diabetes. Acta Gastroenterol Belg. 2011;74:28–33
- Ding W, Zhou L, Bao Y, et al. Autonomic nervous function and baroreflex sensitivity in hypertensive diabetic patients. Acta Cardiol. 2011;66:465–470
- Lee K, Park J, Choi J, Park CG. Heart rate variability and metabolic syndrome in hospitalized patients with schizophrenia. J Korean Acad Nurs. 2011;41:788–794
- Chang YW, Lin JD, Chen WL, et al. Metabolic syndrome and short-term heart rate variability in adults with intellectual disabilities. Res Dev Disabil. 2012;33:1701–1707
- Jarczok MN, Li J, Mauss D, Fischer JE, Thayer JF. Heart rate variability is associated with glycemic status after controlling for components of the metabolic syndrome. Int J Cardiol. 2013;167:855--861
- Lucini D, de Giacomi G, Tosi F, et al. Altered cardiovascular autonomic regulation in overweight children engaged in regular physical activity. Heart. 2013;99:376–381
- Lambert E, Sari CI, Dawood T, et al. Sympathetic nervous system activity is associated with obesity-induced subclinical organ damage in young adults. Hypertension. 2010;56:351–358
- Krishnan AV, Kiernan MC. Uremic neuropathy: Clinical features and new pathophysiological insights. Muscle Nerve. 2007;35:273–290
- Nasrallah MP, Ziyadeh FN. Overview of the physiology and pathophysiology of leptin with special emphasis on its role in the kidney. Sem Nephrol. 2013;33:54–65
- Kuehl M, Stevens MJ. Cardiovascular autonomic neuropathies as complications of diabetes mellitus. Nat Rev Endocrinol. 2012;8:405–416
- Salman IM, Ameer OZ, Sattar MA, et al. Renal sympathetic nervous system hyperactivity in early streptozotocin-induced diabetic kidney disease. Neurourol Urodyn. 2011;30:438–446