Abstract
Context: Pulmonary hypertension (PH) is a devastating disease characterized by progressive elevation of pulmonary arterial pressure and vascular resistance due to pulmonary vasoconstriction and vessel remodeling. The activation of RhoA/Rho-kinase (ROCK) pathway plays a central role in the pathologic progression of PH and thus the Rho kinase, an essential effector of the ROCK pathway, is considered as a potential therapeutic target to attenuate PH.
Objective: In the current study, a synthetic pipeline is used to discover new potent Rho inhibitors from various natural products.
Materials and methods: In the pipeline, the stepwise high-throughput virtual screening, quantitative structure–activity relationship (QSAR)-based rescoring, and kinase assay were integrated. The screening was performed against a structurally diverse, drug-like natural product library, from which six identified compounds were tested to determine their inhibitory potencies agonist Rho by using a standard kinase assay protocol.
Results: With this scheme, we successfully identified two potent Rho inhibitors, namely phloretin and baicalein, with activity values of IC50 = 0.22 and 0.95 μM, respectively.
Discussion and conclusion: Structural examination suggested that complicated networks of non-bonded interactions such as hydrogen bonding, hydrophobic forces, and van der Waals contacts across the complex interfaces of Rho kinase are formed with the screened compounds.
Introduction
Pulmonary hypertension (PH) is an increase of blood pressure in the pulmonary artery, pulmonary vein, or pulmonary capillaries, together known as the lung vasculature, leading to shortness of breath, dizziness, fainting, leg swelling, and other symptoms. PH has been defined as a resting mean pulmonary arterial pressure (mPAP) >25 mm Hg, or an mPAP with exercise >30 mm Hg (Badesch et al., Citation2009). The development of therapeutic concepts in PH is intimately linked with the unraveling of pathogenetic sequelae, which is involved in the regulation of vasomotion and vascular remodeling that have led to “reverse-remodeling” and regenerative strategies as novel treatment concepts (Pullamsetti et al., Citation2014). Recent in vivo studies showed that the RhoA/Rho-kinase (ROCK) pathway and its essential effector, the Rho kinase, play an important role in PH, due to its lasting effects on vasoconstriction and pulmonary cell proliferation leading to vascular remodeling (Duong-Quy et al., Citation2013). The identification of the ROCK signaling cascade as an important regulator of PH pathologic progression and the development of selective Rho inhibitors such as Y27632 and fasudil provided the opportunity to gain new insights into the chemotherapeutics of PH and its complications (Rowan & McLoughlin, Citation2014).
Rho is a ubiquitously expressed serine–threonine protein kinase involved in diverse cellular functions, including smooth muscle contraction, actin cytoskeleton organization, cell adhesion and motility, and gene expression (Noma et al., Citation2006). Specifically, the biological roles of Rho kinase have been extensively explored with particular attention on the cardiovascular system over the past decade. In many preclinical models of cardiovascular diseases, including vasospasm, arteriosclerosis, hypertension, stroke, ischemia–reperfusion injury, and heart failure, Rho inhibitors have shown a remarkable efficacy in reducing vascular smooth muscle cell hypercontraction, endothelial dysfunction, inflammatory cell recruitment, vascular remodeling, and cardiac remodeling (Shi & Wei, Citation2013).
In this study, we purposed a combination of stepwise high-throughput virtual screening and quantitative structure–activity relationship (QSAR)-based rescoring to discover new potent Rho inhibitors from a structurally diverse, drug-like natural product library. Natural products have significant interest in designing new chemical pharmacophores to fulfill the vast demand for novel kinase inhibitors and address critical unmet medical needs with respect to signal transduction pathways (Liu et al., Citation2012). To solidify the computational findings, we also performed a kinase assay to measure the inhibitory activity of several highly promising compounds raised by the virtual screening against Rho kinase. The molecular mechanism and the structural basis of the intermolecular interactions between Rho kinase and potent inhibitors were also characterized on the basis of modeled complex structure data.
Materials and methods
Overview of the integrative protocol
Schematic representation of integrating stepwise virtual screening and kinase assay to identify potent Rho inhibitors from numerous natural products is shown in . A total of >100 000 natural products were extracted from a variety of natural product databases. First, we carried out structural similarity analysis and drug likeness evaluation to define a structurally diverse, drug-like natural product library. Subsequently, molecular docking methods of rigid DOCK and flexible AutoDock were in turn performed to model the intermolecular interactions between the Rho kinase domain and natural product candidates in the library and, on this basis, the interaction strengths for the docked Rho-compound complexes were rescored by a quantitative structure–activity relationship (QSAR)-based scoring function. Finally, a number of compounds with top scores were extracted and analyzed, from which several identified compounds were tested for their inhibitory activities against Rho kinase.
Natural product library
More than 100 000 natural products were retrieved from the Reaxys, CNPD (Shen et al., Citation2003), HIM (Kang et al., Citation2013), and CamMedNP (Ntie-Kang et al., Citation2013) databases. First, we used daylight fingerprints to characterize these compound structures and then heuristic clustering was employed to analyze their structural diversity. As a result, only ∼40 000 samples were extracted, representing a structurally diverse natural product pool. Further, Lipinski’s Rule of Five (Lipinski et al., Citation2001) and PreADMET (Lee et al., Citation2004) were utilized to evaluate drug likeness for the pool, and ultimately 24 709 compounds passed the assessment and they defined a distinct library of natural products with diverse structures and high drug-likeness, which will be used in subsequent high-throughput virtual screening for discovery of potential Rho binders. The 3D conformations of these compounds were converted from their 2D structures using the CORINA program (Molecular Networks GmbH, Erlangen, Germany) (Sadowski et al., Citation1994) and then minimized by the MMFF94 force field (Halgren, Citation1996).
Stepwise virtual screening
Molecular docking
The high-resolution crystal structure of Rho kinase domain in complex with its cognate inhibitor Y27632 (PDB: 2H9V) was used as a template to perform protein–ligand molecular docking. Before docking, the native ligand Y27632 and water molecules were manually removed from the structure, and hydrogen atoms were added using the REDUCE program (Food & Nutrition Service, Alexandria, VA) (Word et al., Citation1999). The active site of Rho kinase was defined by the cocrystallized Y27632. Two widely used docking algorithms, namely DOCK (Meng et al., Citation1992) and AutoDock (Goodsell & Olson, Citation1990), were employed to conduct molecular-docking calculations at different levels of accuracy and efficiency. The DOCK is a rigid docking method which can be used to efficiently screen against large-size compound set with an acceptable time scale, and the AutoDock is a flexible method that employs the Lamarckian genetic algorithm to fully explore the conformational space for protein–ligand interaction. First, we ran the DOCK to implement fast docking of the 24 709 compounds into the active pocket of Rho kinase, and the top-score samples were further re-docked using the more reliable AutoDock method, which was facilitated with a GUI interface AUDocker LE (Sandeep et al., Citation2011) to automate and speed up this process. The DOCK and the AutoDock were implemented by DOCK3.6 (Mysinger & Shoichet, Citation2010) and AutoDock Vina (Trott & Olson, Citation2010) programs, respectively.
Rescoring
A quantitative structure–activity relationship (QSAR)-based scoring function was recently developed by Wang et al. (Citation2014) for the purpose of evaluating kinase–inhibitor interaction potential. The scoring function was trained by 77 kinase–inhibitor complexes with both known affinities and solved crystal structures, which has been successfully used to design new EGFR mutant inhibitors. Here, we employed the QSAR-based scoring function to rescore the binding strength for the Rho kinase-natural product complexes generated by molecular docking.
Kinase assay
The purified Rho kinase domain (ROCK1) was incubated with peptide substrate biotin-Ahx-AKRRLSSLRA-CONH2 and ATP and the subsequent incorporation of 33P into the peptide was quantified by streptavidin bead capture. Assays for determining inhibitory activity were performed in 96-well plates in a total assay volume of 10 μL, containing 1 nM ROCK1, 1 μM peptide substrate, 1 μM ATP, 1 μCi[γ-33P]ATP, 25 mM HEPES, 15 mM MgCl2, and 0.015% bovine serum albumin (pH 7.4). Compounds were assayed over different concentrations to derive dose–response curves, where data were normalized and expressed as percentage inhibition, and then the curve fitting was performed to determine the inhibitory IC50 value for each compound (Doe et al., Citation2007).
Results and discussion
Evaluation of docking methods and scoring functions
In recent years, computational methods have been widely used to design bioactive molecular entities such as small-molecule drugs, peptides, and proteins (Zhou et al., Citation2013). In order to test the reliability of computational methods engaged in this study, we herein employed the AutoDock and the DOCK to re-dock two widely studied Rho inhibitors, i.e., fasudil and Y-27632, into the active pocket of Rho kinase domain, and performed comparison of the re-docked inhibitor conformations with that of native cocrystallized ligands. As can be seen in , the best docked models (the best models were suggested by the highest docking scores) generated separately by the AutoDock and the DOCK were superposed onto the native cocrystallized ligands with respect to Rho complex crystal structures (PDB: 2ESM and 2ETR for fasudil and Y-27632, respectively). The AutoDock can well reproduce the direction and the location of fasudil and Y-27632 in the pocket, with their rmsd of 0.21 and 0.35 Å relative to the native ligands, respectively. In contrast, DOCK did not give a correct prediction for both inhibitors in the pocket, especially resulting in a deviated direction of Y-27632 compared with its native arrangement. In this respect, the fast DOCK can only be applied to coarse-grained high-throughput screening of vast natural products, and the effective AutoDock will then be used to perform a further screening to identify those promising candidates.
Figure 2. Re-docking of two inhibitors fasudil (A) and Y-27632 (B) into the active pocket of Rho kinase domain using AutoDock and DOCK methods; the docked conformations were superposed onto corresponding native cocrystallized ligands.

We also collected six sophisticated Rho inhibitors with known biological activities (IC50 in ). Here, we separately ran DOCK and AutoDock to model their interactions with Rho kinase and employed different scoring strategies, including DOCK, AutoDock, and QSAR scores, to evaluate the interaction strengths. The DOCK and AutoDock scores are based on physical atomic interactions including van der Waals contacts, electrostatic interactions, and bond forces. The QSAR score was trained by 77 kinase–inhibitor complexes with both known affinities and solved crystal structures (Wang et al., Citation2014). The resulting scoring values against experimentally measured activity are shown in , from which it is evident that the DOCK and AutoDock scores only show a moderate correlation with experimental activity, with Pearson’s correlation coefficients of Rp = −0.353 and −0.203, respectively, while the QSAR score can linearly correlate with the activity fairly well, resulting in a high Rp = 0.743. This is expected if considering that DOCK and AutoDock scores are unsupervised and general-purpose scoring functions, whereas the QSAR score is supervised that was developed specifically for kinase–inhibitor binding phenomenon. In the next study, we will thus employ the QSAR-based scoring function to rescore Rho-natural product interactions based on those docking-generated complex models.
Figure 3. Plot of DOCK, AutoDock, and QSAR scores against experimental activity pIC50 for the six sophisticated Rho inhibitors.
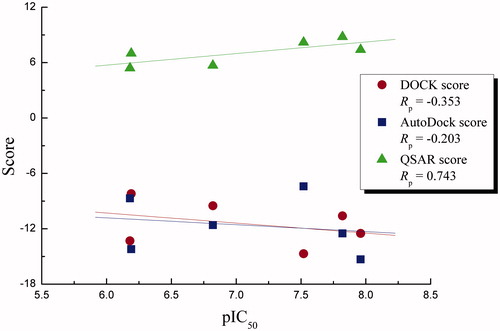
Table 1. Comparison of DOCK, AutoDock, and QSAR scores with inhibitory activity for the six sophisticated Rho inhibitors.
Virtual screening of Rho inhibitor candidates
The fast DOCK method was first conducted to rank the binding strength of 24 709 structurally diverse, drug-like natural products to Rho kinase domain, from which 1000 compounds with top scores were raised; they were re-docked into the active pocket of Rho kinase domain, and were then rescored by the QSAR-based scoring function. The resulting score values for the 1000 samples are shown in .
Since the QSAR score was originally trained by kinase–inhibitor dissociation constant, an indirect indicator of kinase inhibitory activity, ligands with larger score values are always stronger inhibitors than those with smaller values. Therefore, we considered the 100 natural products with top scores as promising candidates, and they are structurally diverse, including classic kinase–inhibitor structures such as quinoline and indole derivatives, as well as some non-classic types. Some of these high-score candidates have been previously found to target an array of kinases involved in diverse cellular events, such as signaling pathway, cell cycle, immune response, and molecular trafficking. Specifically, the promiscuous kinase inhibitor staurosporine, which has previously been reported to have high inhibitory potency against various kinases (Firestone et al., Citation1993), was properly identified by the QSAR-based scoring function as an efficient Rho binder. A number of flavonoids such as quercetin, chrysin and baicalein were suggested to be potent Rho inhibitors.
In order to test the theoretical results arising from virtual screening, we further performed kinase assay to determine the inhibitory activity of six commercially available compounds from the 100 promising candidates against Rho, including baicalein, isoliquiritigenin, kinetin, magnolol, tetrahydropapaverine, and phloretin (). As might be expected, five out of the six tested candidates showed high or moderate inhibitory activities on Rho, with IC50 values ranging from 0.22 (phloretin) to 240 (magnolol) μM. In particular, the biological activities of phloretin and baicalein were determined at the nanomolar level (IC50 = 220 and 950 nM), and three tested compounds tetrahydropapaverine, magnolol, and isoliquiritigenin were also shown relatively high or moderate inhibitory potencies (IC50 = 4, 240, and 45 μM, respectively). However, the kinetin, a class of plant hormone that promotes cell division, has not been detected to have observable efficacy (IC50 > 1000 μM), suggesting that the compound may not be a good lead for further investigation, although it was predicted in silico to have stronger binding capability toward Rho.
Table 2. The six identified natural products.
Structure and affinity analysis of Rho–ligand interactions
Based on complex structures generated from molecular docking, the binding affinities of the two high-activity compounds baicalein and phloretin to Rho kinase domain were minimized and calculated using the YASARA server (Krieger et al., Citation2002). The YASARA is a knowledge-based potential applicable for all atom types in PDB files, which considers the total energy composed of non-bonded interactions and solvent effect. The binding affinities of the two compounds to Rho kinase domain were estimated to be −13.2 and −15.7 kcal/mol, although these values may be slightly deviated from QSAR scores. As known, the YASARA-derived affinities are semi-empirical which do not include the contributions from some physicochemical factors such as entropic effect and conformational flexibility. In contrast, the empirical score employed experimental data to correct theoretical parameters so that it may implicitly involve these physicochemical terms.
The docked complex structure models of baicalein and phloretin with Rho kinase domain are examined in detail, from which it is found that the ligand molecules are well compatible with the active pocket of Rho kinase domain, exhibiting a tight binding mode. The phloretin have a high affinity (ΔG = −15.7 kcal/mol) to Rho, and an intensive non-bonded network was identified at its complex interface with Rho active pocket. Structural examination revealed that a number of hydrogen bonds and hydrophobic interactions (Zhou et al., Citation2009, Citation2012) are formed with Tyr155 and Lys200 and with some non-polar residues such as Val137, Met153, and Leu205. A similar phenomenon was also observed in the complex system of Rho with baicalein, albeit non-polar chemical forces such as hydrophobic potential and van der Waals packing are more significant for the ligand binding as compared with phloretin.
Declaration of interest
The authors report that there are no declarations of interest.
References
- Badesch DB, Champion HC, Sanchez MA, et al. (2009). Diagnosis and assessment of pulmonary arterial hypertension. J Am Coll Cardiol 54:S55–66
- Doe C, Bentley R, Behm DJ, et al. (2007). Novel Rho kinase inhibitors with anti-inflammatory and vasodilatory activities. J Pharm Exp Ther 320:89–98
- Duong-Quy S, Bei Y, Liu Z, Dinh-Xuan AT. (2013). Role of Rho-kinase and its inhibitors in pulmonary hypertension. Pharmacol Ther 137:352–64
- Firestone S, Firestone LL, Ferguson C, Blanck D. (1993). Staurosporine, a protein kinase C inhibitor, decreases the general anesthetic requirement in Rana pipiens tadpoles. Anesth Analg 77:1026–30
- Goodsell DS, Olson AJ. (1990). Automated docking of substrates to proteins by simulated annealing. Proteins 8:195–202
- Halgren TA. (1996). Merck molecular force field. I. Basis, form, scope, parameterization, and performance of MMFF94. J Comput Chem 17:490–519
- Kang H, Tang K, Liu Q, et al. (2013). HIM-herbal ingredients in-vivo metabolism database. J Cheminform 5:28
- Krieger E, Koraimann G, Vriend G. (2002). Increasing the precision of comparative models with YASARA NOVA – A self-parameterizing force field. Proteins 47:393–402
- Lee SK, Chang GS, Lee IH, et al. (2004). The PreADME: PC-based program for batch prediction of ADME properties. EuroQSAR 2004:9.5–10
- Lipinski CA, Lombardo F, Dominy BW, Feeney PJ. (2001). Experimental and computational approaches to estimate solubility and permeability in drug discovery and development settings. Adv Drug Deliv Rev 46:3–26
- Liu J, Hu Y, Waller DL, et al. (2012). Natural products as kinase inhibitors. Nat Prod Rep 29:392–403
- Liu T, Lin Y, Wen X, et al. (2007). BindingDB: A web-accessible database of experimentally determined protein–ligand binding affinities. Nucleic Acids Res 35:D198–201
- Meng EC, Shoichet BK, Kuntz ID. (1992). Automated docking with grid-based energy evaluation. J Comput Chem 13:505–24
- Mysinger MM, Shoichet BK. (2010). Rapid context-dependent ligand desolvation in molecular docking. J Chem Inf Model 50:1561–73
- Noma K, Oyama N, Liao JK. (2006). Physiological role of ROCKs in the cardiovascular system. Am J Physiol Cell Physiol 290:C661–8
- Ntie-Kang F, Mbah JA, Mbaze LM, et al. (2013). CamMedNP: Building the Cameroonian 3D structural natural products database for virtual screening. BMC Complement Altern Med 13:88
- Pullamsetti SS, Schermuly R, Ghofrani A, et al. (2014). Novel and emerging therapies for pulmonary hypertension. Am J Respir Crit Care Med 189:394–400
- Rowan SC, McLoughlin P. (2014). Hypoxic pulmonary hypertension: The paradigm is changing. Exp Physiol 99:837–8
- Sadowski J, Gasteiger J, Klebe G. (1994). Comparison of automatic three-dimensional model builders using 639 X-ray structures. J Chem Inf Comput Sci 34:1000–8
- Sandeep G, Nagasree KP, Hanisha M, Kumar MM. (2011). AUDocker LE: A GUI for virtual screening with AUTODOCK Vina. BMC Res Notes 4:445
- Shen JH, Xu XY, Cheng F, et al. (2003). Virtual screening on natural products for discovering active compounds and target information. Curr Med Chem 10:2327–42
- Shi J, Wei L. (2013). Rho kinases in cardiovascular physiology and pathophysiology: The effect of fasudil. J Cardiovasc Pharmacol 62:341–54
- Trott O, Olson AJ. (2010). AutoDock Vina: Improving the speed and accuracy of docking with a new scoring function, efficient optimization and multithreading. J Comput Chem 31:455–61
- Wang B, Shen W, Yang H, et al. (2014). Targeting EGFR mutants with non-cognate kinase inhibitors in non-small cell lung cancer. Med Chem Res 23:4510--30
- Wang R, Fang X, Lu Y, Wang S. (2004). The PDBbind database: Collection of binding affinities for protein–ligand complexes with known three-dimensional structures. J Med Chem 47:2977–80
- Word JM, Lovell SC, Richardson JS, Richardson DC. (1999). Asparagine and glutamine: Using hydrogen atom contacts in the choice of side-chain amide orientation. J Mol Biol 285:1735–47
- Zhou P, Huang J, Tian F. (2012). Specific noncovalent interactions at protein–ligand interface: Implications for rational drug design. Curr Med Chem 19:226–38
- Zhou P, Wang C, Ren Y, et al. (2013). Computational peptidology: A new and promising approach to therapeutic peptide design. Curr Med Chem 20:1985–96
- Zhou P, Zou J, Tian F, Shang Z. (2009). Fluorine bonding – How does it work in protein–ligand interactions? J Chem Inf Model 49:2344–55