Abstract
3D-QSAR methods, CoMFA region focusing (CoMFA-RF) and CoMSIA along with docking studies carried out for investigating 32 carbonic anhydrase I inhibitors. These inhibitors have been studied for the development of antiglaucoma, antitumor, antiobesity or anticonvulsant drugs. Docking analysis by GOLD provide conformations which have been realigned in CoMFA and CoMSIA models. Training set for the CoMFA–RF and CoMSIA models using 24 docked conformations gives q2Loo values of 0.615 and 0.637 and r2nv values of 0.701 and 0.713, respectively. The results of CoMFA-RF and CoMSIA with and without docked conformations were compared. The ability of prediction and robustness of the models were evaluated by test set, cross validation (leave-one-out and leave-ten-out), bootstrapping, and progressive scrambling approaches. The all-orientation search (AOS) was used to achieve the best orientation to minimize the effect of initial orientation of the structures. The docking results confirmed CoMFA and CoMSIA contour maps.
Keywords::
Introduction
Carbonic anhydrase(CA) (EC 4.2.1.1) is the first metalloenzyme identified in 1941 by Keilin and ManCitation1. These enzymes are belong to a family of zinc metalloenzymes, which catalyze the reversible hydration of carbon dioxide to bicarbonate ion (CO2 + H2O ⇔ HCO3− + H+)Citation2. So this is a basic reaction for respiration and transportation of CO2 between metabolizing tissues and excretion sitesCitation3. CA enzymes have been classified in five distinct gene families: α-,β-,γ-, δ-, and ξ- that are present in prokaryotes and eukaryoteCitation4,Citation5. There are several cytosolic forms (CA I–III, VII), four membrane-bound isozymes (CA IV, IX, XII, and XIV), one mitochondrial form (CA V), and a secreted CA isozyme, CA VICitation6. The process of enzyme’s acting may be schematically represented by equations (1.1) and (1.2).
The rate determining step is equation (1.2), that is, the proton transfer that reproduce the zinc hydroxide species of the enzymeCitation7. The X-ray structures of CAs have shown that the zinc ion (Zn+2) within the active site is coordinated to three histidine residues and one water molecule (or hydroxide anion) and that the water molecule is replaced by a CA inhibitor as the fourth ligand of Zn+2.Citation8 One of the most prominent classes of CA inhibitors is aromatic/heterocyclic sulfonamides which have been studied for the development of antiglaucoma, antitumor, antiobesity or anticonvulsant drugsCitation9.
Quantitative structure-activity relationship (QSAR) studies generally perform a vital role in drug discovery and design as ligand-based approachesCitation10. Such approaches provide not only the reliable prediction of specific properties of new compounds, but also they help to clarify the possible molecular mechanism of the receptor-ligand interactions, in case that the experimental NMR or crystal structure of the target protein is not availableCitation10.
In recent years, a number of studies have been carried out for predicting new carbonic anhydrase inhibitors such as Clare and Supuran describe a QSAR based almost entirely on quantum theoretically calculated descriptors for a large and heterogeneous group of aromatic and heteroaromatic carbonic anhydrase inhibitors, using orbital energies, nodal angles, atomic charges, and some other intuitively appealing descriptorsCitation3. Gavernet and Coworkers synthesized sulfamides and sulfamates inhibitors and tested in their inhibition to carbonic anhydrase CAII activity, inhibition pattern interpreted by means of molecular modeling techniquesCitation4. Abdel-Hamid et al synthesized a new series of 1,3,4-thiadiazole-2-thione derivatives for the inhibition of three physiologically relevant carbonic anhydrase isozymes, the cytosolic human isozymes I and II, and the transmembrane, tumor-associated hCA IX and use docking studies of the tested compounds in order to predict the affinity and orientation of these compoundsCitation11. Agrawal et al describe a QSAR study on a series of carbonic anhydrase inhibitors using a series of distance-based topological indicesCitation12. Khadikar and coworkers describe a QSAR study on aromatic/heterocyclic sulfonamides containing 8-quinoline-sulfonyl carbonic anhydrase (CA) inhibitors which has been carried out topologically using first-order valence connectivity index (1vv)Citation13. Melagraki and coworkers describe carbonic anhydrase II inhibitory with the method of linear quantitative structure–activity relationship for a series of para-substituted aromatic sulfonamides by using topological index methodologiesCitation14. Potter describes the docking of selected steroidal and non-steroidal estrone sulphatase inhibitorsCitation15. Quantum chemical QSAR expressions have been developed by Clare and Supuran for a heterogeneous group of 36 sulfonamides by using the ACE statistical techniqueCitation16. Another Quantum chemical QSAR study which was performed by Clare and Supuran, have been developed on a group of 1,3,4-thiadiazole- and 1,3,4-thiadiazoline disulfonamides carbonic anhydrase inhibitors base on AM1 calculationsCitation17.
In this study we have investigated inhibition of a series of sulfonamides derivativesCitation18 against carbonic anhydrase I by using 3D-QSAR models, comparative molecular field analysis (CoMFA)Citation19 and comparative molecular similarity indices analysis (CoMSIA). In CoMFA, steric and electrostatic fields surrounding a set of aligned molecules in a grid box sampled and correlated with observed activities. In a similar method, CoMSIA, a probe atom is used to calculate similarity indices, at regularly spaced grid points for the aligned molecules. The molecular fields are calculated with potentials force field e.g. Lennard-Jones and Coulomb. While CoMSIA uses Gaussian-based similarity functions for molecular field calculationsCitation19–21. Docking tools provide key structural features of binding of an inhibitor into the receptor and predicting bioactive conformers.
Experimental and methods
Data set
A series of 32 aromatic sulfonamide derivativesCitation18 were investigated in this study . In vitro CAI inhibitory activities were changed into the corresponding pKi (−log Ki) values. The set of inhibitors was divided into training and test sets. The test set compounds were selected by considering both the distribution of biological data and structural diversity of the molecules. At first the total set of CAI inhibitors (32 compounds) was divided into the training set (26 compounds) and test set (6 compounds), and 2 compounds (compounds no. 1 and 32) showed large residual values, then are identified as outliers and removed from the training set.
Table 1. Structures and activities of aromatic sulfonamide derivatives.
Molecular docking
The crystal structure of human carbonic anhydrase I (CA I) is 1AZM was taken from RCSB protein databank (http://www.pdb.org). This protein in PDB is not complexed with anyone of the understudy ligands, so in docking step its original ligand was removed and then ligands in our data set were docked in the active site of CA I one by one. Ligands preparation step was carried out in SYBYL 7.3 molecular modeling package (Tripos Inc., St. Louis, USA) running on a Red Hat Linux workstation 4.7. The resulting structures were imported into Discovery Studio 2.5 (Accelrys Inc, San Diego, CA, USA), and typed with CHARMm force field then partial charges were calculated by Momany-Rone optionCitation22. The resulting structures were minimized with Smart Minimizer which performs 1000 steps of steepest descent with a RMS gradient tolerance of 3, followed by conjugate gradient minimizationCitation23. For preparation step of enzymes, all complexes were typed with CHARMm force field, hydrogen atoms were added, all water molecules were removed and pH of protein was adjusted to almost neutral, 7.4, using protein preparation protocol. All inhibitors were again minimized in-situ with Smart Minimizer option that is custom for in-situ ligand minimization and consists of some pre-defined minimization steps that have been pre-determined to work well for receptor ligand dataCitation23. A 6.008 Å radius sphere was defined around the bounded ligands to confirm atoms of each ligand and the side-chains of the residues of the receptor within 6.008 Å from the centre of the binding site are free to move. Then bounded inhibitors were removed from the binding site. Other parameters were set by default protocol settings. GOLD program was used to dock inhibitors into receptor Supplementary Figure S1Citation24. Predicting how a small molecule will bind to a protein is complicated, and no method can guarantee success. The best approach is to measure as accurately as possible the reliability of the method (i.e., the chance that it will make a successful prediction in a given instance). For this reason, GOLD has been tested on a large number of complexes extracted from the Protein Data Bank. The overall conclusion of these tests was that the top-ranked GOLD solution was correct in 70–80% of casesCitation25. Different values of the genetic algorithm parameters may be used to control the balance between the speed of GOLD and the reliability of its predictions. GOLD will only produce reliable results if correct atom typing for both protein and ligand is used properly. In this method a site sphere around the ligand is needed and the radius was set to 8.954 Å in this approach.
CoMFA and CoMSIA
Two strategies were used to generate 3D-QSAR models, in first approach the structures of inhibitors were sketched in SYBYL molecular modeling package and partial atomic charges were calculated using the Gasteiger-Hückel method. The compounds were aligned to template molecule (compound 22) on a backbone which is common among all structures for minimizing the sum-of-squares deviation between reference backbone in each inhibitor and the corresponding core in the template. In CoMFA a probe spCitation3 carbon atom with +1 charge was employed to calculate steric and electrostatic interactions between the probe and structures. Electrostatic interactions calculated by using a Coulomb potential and van der Waals interaction using a Lennard-Jones potential function. Various column filtering values were also tested. CoMFA standard scaling applies the equal weight to data from each lattice point in any given field. Region focusing is an iterative procedure which refines a model by improving the weight for those lattice points which are most related to the model. This enhances the resolution and predictive capability (q2; cross validated r2) of a followed PLS analysis. Technically, this corresponds to rotate the model components during a high-order spaceCitation26. PLS region focusing is rationally equivalent to the GOLPE strategy and q2-GRSCitation27,Citation28. In CoMSIA, standard settings (probe with charge +1, radius 1 Å and hydrophobicity +1, hydrogen-bond donating +1, hydrogen-bond accepting +1, grid spacing 2 Å) were used to calculate five different fields: steric, electrostatic, hydrophobic, hydrogen bond acceptor and donor. In 3D-QSAR, PLS analysis was used for modeling in which the independent variables were the CoMFA and CoMSIA fields, and −log Ki data values used as dependent variables.
In the second strategy bioactive conformers were docked and aligned inside the active site of protein by GOLD software, then all conformers from docking were realigned to the template molecule (compound 22) on a common backbone (Supplementary Figure S2) and other steps were performed like first strategy.
Because different space orientations of the molecular collective in the grid box have major effect on 3D-QSAR models, all-orientation search (AOS) was also carried out on initial orientations of aligned structures by the rotation procedure written in SYBYL programming language (SPL)Citation29.
Partial least squares (PLS) analysis
The CoMFA and CoMSIA descriptors were used as independent variables and pKi values as dependent variables in partial least squares regression analysis. Leave one out(LOO) cross-validation method was used to obtain the optimal number of components (latent variables) in the subsequent analysis. The minimum-sigma (column filtering) was used to improve the signal-to-noise ratio in CoMFA and CoMSIA models. To get the highest correlation coefficient (r) and the lowest standard error in the LOO cross-validated predictions, the optimum number of principal components in the final non-cross-validated QSAR equations was determined. Analysis of CoMFA and CoMSIA results and the prediction of the models were performed by non-cross validation method according to SYBYL terminology.
Results and discussion
Docking results
Docking computations were employed to find the probable binding conformations of all carbonic anhydrase I inhibitors. To validate the docking reliability, root-mean-square distance (RMSD) value was calculated between bounded inhibitor and redocked ligand, which were 0.25 in this method. This value shows a high reliability of GOLD method to reproduce the known binding mode of these inhibitors. Docking results shows a hydrogen bond between SO2NH2 substituent and His119, Supplementary Figure S3. Another hydrogen bond was found between the proton of indole and HIS200, Supplementary Figure S3. According to docking results, there is an interaction between inhibitor molecules (in this case compound 22) and Zinc atom, by the oxygen of SO2NH2. shows three residues (His119, His96, and His94) and oxygen of SO2NH2 which have interaction with Zinc atom. MOLCAD surfaces were calculated for the most active compound (M22) to demonstrate electrostatic potential (Supplementary Figure S4a), lipophilic potential (Supplementary Figure S4b) and hydrogen bonding (Supplementary Figure S4c) interactions.
CoMFA and CoMSIA results
PLS analysis of the compounds in training set showed CoMFA-region focusing (CoMFA-RF) QSAR model (grid spacing = 1) with a good q2 value of 0.627 (1 components) that is better than common CoMFA. The optimal number of components evaluated by selecting the highest q2 value corresponds to lowest Spress value. The non cross-validated PLS analysis results in a high conventional showed r2 of 0.706, F = 52.803 a low standard error of estimation (SEE) 0.384, with a column filtering of 3.0.
The CoMSIA analysis was done at a grid spacing 2 Å, and the effect of column filtering was checked with the combination of five fields. The CoMSIA method defines hydrophobic and hydrogen bond donor and acceptor descriptors in addition to the steric and electrostatic fields in CoMFA. The combination of fields was systematically changed to select the optimal results.
By using the combination of five fields, q2 of 0.636 was obtained with 1 component at a column filtering of 2.5 kcal/mol, The non cross-validated analysis results showed r2 of 0.713, F = 54.597, and SEE = 0.380. The q2 values of each independent field of steric, electrostatic, hydrophobic, hydrogen bond donor and hydrogen bond acceptor are 0.648, 0.608, 0.619, 0.593, 0.645 respectively. The contribution of all of these five fields has significant effect on constructed model. The values of experimental and predicted activities are depicted in .
Table 2. The experimental Ki values, predicted Ki values (Pred.) and the residuals (Res.) of the training and test set compounds based on realignment method.
Beside the 3D-QSAR studies described above that constructed and aligned conformers in SYBYL program, all conformers were docked in CAI protein by using GOLD method in discovery studio program and then realigned to the template molecule (compound 22 in this case) in SYBYL program by using rigid alignment method. A CoMFA-RF 3D-QSAR model with a q2 value of 0.615 with 1 PLS components obtained. Also, CoMSIA 3D-QSAR study was performed on the compounds in the realignment method. CoMSIA PLS analysis determined q2 value of 0.637 with 1 PLS components. shows results of models constructed by these two alignment methods.
Table 3. Summary of the statistical results for the constructed models.a
Validation of the 3D-QSAR models
The external set of 6 compounds was used to confirm predictive ability of the models. The r2pred from CoMFA-RF and CoMSIA models without using docking conformer were found to be 0.744 and 0.706, and with using docking conformer were 0.706 and 0.702 respectively, which show models have acceptable predictability. To evaluate the statistical confidence limits of the derived models, bootstrappingCitation19 analysis was carried out with 100 runs. Bootstrapping subsumes the generation of many new datasets from the original dataset after randomly choosing samples from that. A r2bs (average correlation coefficient for bootstrapping) of 0.735 ± 0.071 and a SEEbs (average standard error of estimate for bootstrapping) of 0.353 ± 0.182 for CoMFA-RF model with using docking conformer, and r2bs of 0.738 ± 0.072, and a SEEbs of 0.358 ± 0.144 for CoMSIA model generated by docking conformer, suggested a good internal consistency and the absence of systematic errors of the models. To evaluate the sensitivity of the optimized CoMFA-RF and CoMSIA models to chance correlations, the leave-one-out (LOO), leave 10-out cross-validation and progressive scrambling analyses were performedCitation30. The q2 values of leave 10-out for CoMFA-RF and CoMSIA models without using docking conformer were 0.630 and 0.633 and with using docking conformer were 0.611 and 0.635 respectively. In the progressive scrambling approach, small random perturbations are introduced into a data set and the statistical results, the perturbation prediction (q2), the calculated cross-validated standard error of prediction (cSDEP) as the function of the correlation coefficient between the true values (y) of the dependent variables and the perturbed values (y’) of the dependent variables, and the slope of q2 (cross validated correlation coefficient) with respect correlation of the original dependent variables against the perturbed dependent variables (dq2′/dr2yy’), for CoMFA-RF and CoMSIA models are summarized in .
Table 4. Model progressive scrambling for CoMFA-RF and CoMSIA models based on realignment method.
CoMFA contour maps analysis
In the CoMFA steric contour maps, green contours show regions that are sterically favorable and yellow contours show sterically unfavorable regions, these contours represent 80% and 20% contributions, respectively. Similarly blue contours show the regions that are electropositive charge favorable and red contours show electronegative charge favorable regions that their contributions in the CoMFA electrostatic field represent 80% and 20% respectively.
In the first series (compound 1–15) there is a steric unfavorable yellow contour cover R3 and R2 substituents indicate that bulky groups in these regions will decrease inhibitory activity Supplementary Figure S5. It can be demonstrated by comparing the structures and activities of compound 3 (R3 = Me, Ki = 730) with 6 (R3 = F, Ki = 116) and compound 5 (R2 = F, Ki = 621) with 8 (R2 = Cl, Ki = 640). In the second series (compound 16–32) near R2 and R3 substituents there are steric favorable green contours indicate that in these positions bulky groups increase inhibitory activity . For example compound 29 (R3 = OMe, Ki = 8.6) is more active than compound 27 (R3 = Me, Ki = 110).
Figure 2. CoMFA contour map displaying steric (a) and electrostatic (b) in combination with compound 27 and 22 respectively based on realignment method.
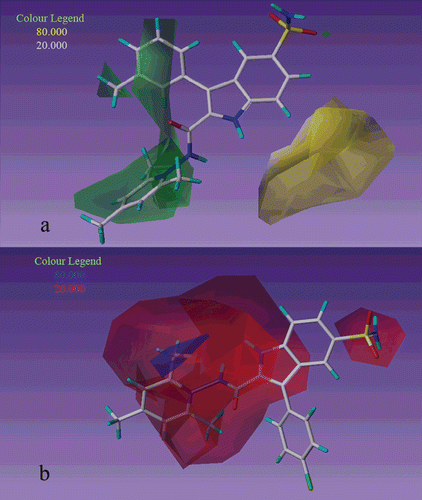
In CoMFA electrostatic contour maps for second series, there is a big red contour near the substituent of pyridine ring () that shows electronegative groups in this situation increase the inhibitory activity, so that activity of second series that has N+ in pyridine ring is more than first series which has not N+. For example compound 22 (R4 = Cl, Ki = 3.2) is more active than compound 10 (R4 = Cl, Ki = 112).
CoMSIA contour map analysis
The steric field distribution of CoMSIA model is shown in . By comparison of with , it can be seen that CoMSIA steric contour maps is similar to CoMFA steric contour maps. In this field for second series there is a big green contour covers pyridine ring indicates that bulky groups in this region increase activity. It can be demonstrated by comparing the activity of second series with first series, for example compound 17 (R2 = F, Ki = 8.5) which is in second series is more active than compound 5 (R2 = F, Ki = 621) in first series.
Figure 3. CoMSIA contour map displaying steric (a), electrostatic (b), hydrophobic (c), hydrogen bond donor (d), hydrogen bond acceptor (f) in combination with 17, 17, 16, 16 and 23 respectively based on realignment method.
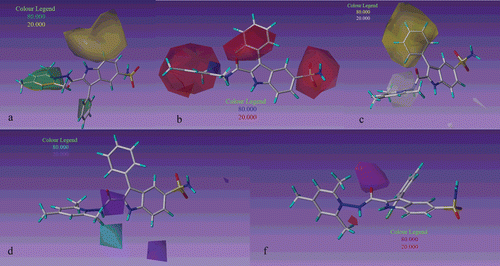
In the CoMSIA electrostatic contour maps in addition to the contours that were shown in CoMFA, for second series there is a big red contour which covers R2 substituent indicates that electronegative groups in this position increase inhibitory activity, . It can be demonstrated that compound 17(R2 = F, Ki = 8.5) is more active than compounds 20(R2 = Cl, Ki = 25.1) and 23(R2 = Br, Ki = 45.5).
The CoMSIA hydrophobic contour maps are shown by . For this field the yellow (hydrophobic favorable) contours and white (hydrophobic unfavorable) contours represent 80% and 20% contributions, respectively. For second series compounds (16–32) there is one big yellow contour covers the phenyl ring which is substitutated in 3-position of indole indicates that hydrophobic groups in this area are preferred for CAI inhibitory activity. According to docking results in this area there are some interaction with hydrophobic residues of receptor, such as LEU131, PHE91 and GLY92 that confirm CoMSIA’s hydrophobic contour Supplementary Figure S6. This is a sensible reason why compound 16 (R = H, Ki = 9.00) has higher activity than compounds 21 and 27 (R = Cl, Ki = 113 and R = Me, Ki = 110).
depicts H-bond donor field distribution of CoMSIA model which is represented by cyan and purple contours. Cyan and purple contours indicate regions where hydrogen bond donor substituents on ligand are favored and disfavored respectively. There is one purple contour cover the−CO group in CONH2 indicate that hydrogen bond donor groups in the receptor enhance the activity of inhibitor, Supplementary Figure S7. Based on hydrogen bond acceptor field in magenta contours show regions where hydrogen bond acceptor groups are favored and red contours indicate regions where hydrogen bond acceptor groups are unfavorable for increasing activity. There is one magenta contour near carbonyl substituent suggest hydrogen bond acceptor in this region is favored. Complementary of this magenta contour in the receptor, is hydrogen bond donor group (His 200). Also presence of electrostatic red contour at this position confirms the magenta contour.
Conclusions
In this study, molecular docking and 3D-QSAR studies were performed on a series of sulfonamide CAI inhibitors. 3D-QSAR models were generated by two different methods, by using docked ligands as a bioactive conformation and without docked ligands. The comparison of these models indicates that CoMFA and CoMSIA models which were generated by docked conformers have more reliable contour maps. The CoMFA and CoMSIA analyses have provided discerning key structural features which affect inhibitory activity of these inhibitors. We identified and confirmed the residues that play key role in the hydrogen bond donors and hydrogen bond acceptors in addition to the hydrophobic, steric and electrostatic interactions. The significant statistical parameters and appropriate predictive ability of the generated models indicate that these models can help to rational design of novel carbonic anhydrase inhibitors.
Declaration of interest
The authors report no conflicts of interest.
Supplementary Material
Download PDF (946.3 KB)References
- Keilin D, Mann T. Carbonic anhydrase. Purification and nature of the enzyme. Biochem J 1940;34:1163–1176.
- Nuti E, Orlandini E, Nencetti S, Rossello A, Innocenti A, Scozzafava A et al. Carbonic anhydrase and matrix metalloproteinase inhibitors. Inhibition of human tumor-associated isozymes IX and cytosolic isozyme I and II with sulfonylated hydroxamates. Bioorg Med Chem 2007;15:2298–2311.
- Clare BW, Supuran CT. A physically interpretable quantum-theoretic QSAR for some carbonic anhydrase inhibitors with diverse aromatic rings, obtained by a new QSAR procedure. Bioorg Med Chem 2005;13:2197–2211.
- Gavernet L, Gonzalez Funes JL, Blanch LB, Estiu G, Maresca A, Supuran CT. Affinity of sulfamates and sulfamides to carbonic anhydrase II isoform: experimental and molecular modeling approaches. J Chem Inf Model 2010;50:1113–1122.
- Tarko L, Supuran CT. QSAR studies for the inhibition of the transmembrane isozymes XII and XIV of human carbonic anhydrase with a series of sulfonamides. Bioorg Med Chem 2007;15:5666–5671.
- Supuran CT. Carbonic anhydrases: novel therapeutic applications for inhibitors and activators. Nat Rev Drug Discov 2008;7:168–181.
- Supuran CT, Winum JY (ed.: Wang B). Wiley, Hoboken, NJ, 2009, p. 19.
- Yoshida T, Munei Y, Hitaoka S, Chuman H. Correlation analyses on binding affinity of substituted benzenesulfonamides with carbonic anhydrase using ab initio MO calculations on their complex structures. J Chem Inf Model 2010;50:850–860.
- Pastorekova S, Parkkila S, Zavada J. Tumor-associated carbonic anhydrases and their clinical significance. Adv Clin Chem 2006;42:167–216.
- Zhang L, Tsai KC, Du L, Fang H, Li M, Xu W. How to generate reliable and predictive CoMFA models. Curr Med Chem 2011;18:923–930.
- Abdel-Hamid MK, Abdel-Hafez AA, El-Koussi NA, Mahfouz NM, Innocenti A, Supuran CT. Design, synthesis, and docking studies of new 1,3,4-thiadiazole-2-thione derivatives with carbonic anhydrase inhibitory activity. Bioorg Med Chem 2007;15:6975–6984.
- Agrawal VK, Banerji M, Gupta M, Singh J, Khadikar PV, Supuran CT. QSAR study on carbonic anhydrase inhibitors: water-soluble sulfonamides incorporating beta-alanyl moieties, possessing long lasting-intra ocular pressure lowering properties–a molecular connectivity approach. Eur J Med Chem 2005;40:1002–1012.
- Agrawal VK, Bano S, Supuran CT, Khadikar PV. QSAR study on carbonic anhydrase inhibitors: aromatic/heterocyclic sulfonamides containing 8-quinoline-sulfonyl moieties, with topical activity as antiglaucoma agents. Eur J Med Chem 2004;39:593–600.
- Melagraki G, Afantitis A, Sarimveis H, Igglessi-Markopoulou O, Supuran CT. QSAR study on para-substituted aromatic sulfonamides as carbonic anhydrase II inhibitors using topological information indices. Bioorg Med Chem 2006;14:1108–1114.
- Vicker N, Ho Y, Robinson J, Woo LL, Purohit A, Reed MJ et al. Docking studies of sulphamate inhibitors of estrone sulphatase in human carbonic anhydrase II. Bioorg Med Chem Lett 2003;13:863–865.
- Clare BW, Supuran CT. Carbonic anhydrase inhibitors. Part 86. A QSAR study on some sulfonamide drugs which lower intra-ocular pressure, using the ACE non-linear statistical method. Eur J Med Chem 2000;35:859–865.
- Supuran CT, Clare BW. Carbonic anhydrase inhibitors − Part 57: Quantum chemical QSAR of a group of 1,3,4-thiadiazole- and 1,3,4-thiadiazoline disulfonamides with carbonic anhydrase inhibitory properties. Eur J Med Chem 1999;34:41–50.
- Güzel O, Maresca A, Hall RA, Scozzafava A, Mastrolorenzo A, Mühlschlegel FA et al. Carbonic anhydrase inhibitors. The beta-carbonic anhydrases from the fungal pathogens Cryptococcus neoformans and Candida albicans are strongly inhibited by substituted-phenyl-1H-indole-5-sulfonamides. Bioorg Med Chem Lett 2010;20:2508–2511.
- Cramer RD, Patterson DE, Bunce JD. Comparative molecular field analysis (CoMFA). 1. Effect of shape on binding of steroids to carrier proteins. J Am Chem Soc 1988;110:5959–5967.
- Klebe G, Abraham U, Mietzner T. Molecular similarity indices in a comparative analysis (CoMSIA) of drug molecules to correlate and predict their biological activity. J Med Chem 1994;37:4130–4146.
- Pirhadi S, Ghasemi JB. 3D-QSAR analysis of human immunodeficiency virus entry-1 inhibitors by CoMFA and CoMSIA. Eur J Med Chem 2010;45:4897–4903.
- Momany FA, Rone R. Validation of the general purpose QUANTA ®3.2/CHARMm® force field. J Comput Chem 1992;13:888–900.
- Discovery Studio, Accelrys Software, Inc, San Diego, CA, 2009.
- Politi A, Durdagi S, Moutevelis-Minakakis P, Kokotos G, Mavromoustakos T. Development of accurate binding affinity predictions of novel renin inhibitors through molecular docking studies. J Mol Graph Model 2010;29:425–435.
- GOLD: User Guides and Tutorials http://www.ccdc.cam.ac.uk/support/documentation/gold/3_1/gold31.pdf (2006).
- Tripos, QSARTM Manual, SYBYL, version 7.3, 2006, St. Louis 2006.
- Baroni M, Costantino G, Cruciani G, Riganelli D, Valigi R, Clementi S. Generating Optimal Linear PLS Estimations (GOLPE): An advanced chemometric tool for handling 3D-QSAR problems. Quant Struct Act Relat 1993;12:9–20.
- Cho SJ, Tropsha A. Cross-validated R2-guided region selection for comparative molecular field analysis: a simple method to achieve consistent results. J Med Chem 1995;38:1060–1066.
- Wang R, Gao Y, Liu L, Lai L. All-orientation search and all-placement search in comparative molecular field analysis. J Mol Model 1998;4:276–283.
- Clark R, Fox P. Statistical variation in progressive scrambling. J Comput Aided Mol Des 2004;18:563–576.