Abstract
The glutaminyl cyclase inhibitory activity of a series of imidazoles has been analyzed through combinatorial protocol in multiple linear regressions (CP-MLR) and partial least square using different topological and structural descriptors. The QC activity was found to be correlated with 2D-autocorrelation (2DAUTO) and atom centered fragments (ACF) descriptors. The descriptor from 2DAUTO class showed that molecular structure frames of one, six and seven path length associated with atomic van der Waals volumes and polarizability hold scope for modulating QC inhibitory activity. The ACF descriptors suggested that the unsubstituted alkyl fragments and methyl substituted imidazole ring are favorable, while unsaturation in the same and C=N-C≡N are unfavorable for activity. The molar refractivity (MR) is conducive for activity. The descriptors identified in the study collectively highlight the significance of molecular volume and polarizability to the QC inhibitory activity of imidazoles. The models are statistically significant and showed good predictivity.
Introduction
The N-terminal modification of peptides by the generation of a pyro-glutamyl (pGlu) moiety from the glutamine precursors is an important process in the maturation of bioactive neuropeptides, hormones and cytokinesCitation1,Citation2. This process is catalyzed by glutaminyl cyclase (QC)Citation3. QCs are acyltransferases found in mammalian neuroendocrine tissues and are highly conserved from yeast to humanCitation4. Its protein sequence is highly conserved throughout the different mammalian species. In human, QC activity is highly abundant in secretory and neuronal tissues and is preferentially confined to certain brain regions such as hypothalamus and pituitaryCitation5,Citation6.
It was observed that QC is involved in the formation of several plaque-forming peptides, like amyloid-β (Aβ) peptides and collagen-like amyloid plaques and play a pivotal role in Alzheimer’s disease (AD)Citation7–9. It was reported that QC is probably the responsible enzyme for the formation of N-terminal pGlu residues after the liberation of the N-terminus of glutamate at position 3 of the Aβ-peptide, thereby exhibiting a glutamate cyclase activityCitation10. These pGlu containing peptides are resistant to proteolysis, exhibit neurotoxicity and have a tendency to aggregate. The formation of these modified Aβ species is likely to be crucial in the initiation and the progress of the ADCitation11–13.
The inhibition of QC activity has been suggested as a new strategy for the treatment of AD. A study reported by Schilling et al. shows that application of a QC inhibitor results in reducing Aβ(3(pE)-42) burden as well as the total plaque load in different transgenic mouse models of AD with improved performance in context of memory and spatial learning testsCitation8. Recently, Buchholz et al. have reported a series of imidazoles with their in vitro QC inhibitory activityCitation14. These compounds were also evaluated for their efficacy on the inhibition of pGlu-Aβ3(pE)-40/43 formation in a cell culture. Good correlation was observed between inhibitory potency and the efficacy in the cellular modelCitation14. This further emphasizes the role of QC in the formation of modified amyloid-β (Aβ) peptides in progress of AD.
Rationale drug design approaches, which include QSAR and molecular modeling protocols, cull out structural and functional requirements of chemical entities desirable for biological response. Addressing this aspect, the QSAR and molecular modeling protocols project the optimal structural and functional requirements for the desirable biological response. Establishing a correlation between the structure and the associated activity is a prior measure for identifying the structural/functional requirements of the activity. In these studies parameterization of chemical structure plays a pivotal role. In enumeration of chemical structures, it is important to note that in isolation, a data point is only a qualified number. A collection of such qualified numbers makes a variable or descriptor. In mathematical models each and every (independent; X) variable communicate with the target (dependent; Y) variable. A meaningful communication between X and Y variables result in the evolution of models with predictive value. With this philosophy a quantitative structure-activity relationship (QSAR) study has been contemplated on a series of imidazoles () to rationalize their QC inhibitory activity profileCitation14. For the study different topological and structural descriptors of the compounds have been opted from DRAGON softwareCitation15. With all these descriptors as independent parameters, the structure-activity relations of the QC inhibitory activity of the imidazoles () have been discovered using the combinatorial protocol in multiple linear regression (CP-MLR) approach, which is a variable selection procedure for model development in QSAR and QSPR studiesCitation16–18. A partial least squares (PLS) analysis has been carried out on the descriptors identified in CP-MLR to derive a composite PLS model highlighting their significance to the activityCitation18. The procedural aspects and the results are being presented here.
Table 1. Observed and predicted glutaminyl cyclase (QC) inhibitory activity of imidazoles.
Material and methods
Dataset
In this study forty five imidazoles have been considered from the literature report for the structure database ()Citation14. The biological activity was reported as Ki (nanomolar) for inhibition of human glutaminyl cyclases (hQC). The inhibitory activity (Ki) has been transformed in the form of logarithm of inverse of inhibitory activity (−logKi or pKi). The structures of the compounds have been drawn in ChemDrawCitation19 software using the standard procedure. All these structures have been ported to DRAGON softwareCitation15 for computing the descriptors. The DRAGON software offers several hundreds of diverse descriptors corresponding to constitutional, topological and structural framework of the molecules and their structural fragmentsCitation15. The descriptors from each class were calculated separately. Descriptors with constant value inside each class were discarded. Also, the descriptors with inter-correlation value equal to or more than 0.99 with other descriptors within class were excluded from the study. This exclusion was used to reduce collinear descriptors. Further, for external validation of QSAR models, the compounds dataset has been divided in training (33 compounds) and test sets (12 compounds) using single linkage hierarchical clustering of all the descriptors (). The computational procedure of model development is briefly described below.
QSAR procedure
The CP-MLR and PLS methods have been used to generate QSAR models. The CP-MLR is a ‘filter’ based variable selection procedure for model development in QSAR studiesCitation16–18. Its procedural aspects and implementation are discussed in some of the recent publicationsCitation16–18,Citation20–24. It involves a combinatorial strategy with appropriately placed ‘filters’ interfaced with MLR and extracts diverse models having unique combination of descriptors from the dataset. In CP-MLR, the filter-1 seeds the variables by way of limiting inter-parameter correlations to predefined level (default acceptable value ≤0.3); filter-2 controls the variables entry to a regression equation through t-values of coefficients (threshold value ≥2.0) which confer more than 95% regression coefficients significant levelCitation16; filter-3 provides comparability of equations with different number of variable in terms of square-root of adjusted multiple correlation coefficient of regression equation ‘r-bar’ and filter-4 estimates the consistency of the equation in terms of cross-validated R2 or Q2 with “leave-one-out” (LOO) cross-validation as default option (threshold value 0.3 ≤ Q2 ≤ 1.0). In this study, for the filters-1, 2, 3 and 4 of CP-MLR the thresholds were assigned as 0.3, 2.0, 0.71 and 0.3 ≤ Q2 ≤ 1.0, respectively. All the identified models were reassessed for the chance correlations if any, by repeated randomization of the activityCitation20. Each identified model was subjected to one hundred simulation runs with scrambled activity and the emerging correlations were counted to express the percent chance correlation of the model under examination. The proposed models were validated through test set. Furthermore, the descriptors identified in CP-MLR models were evaluated in the PLS procedure in order to derive composite single-window MLR-like PLS equation comprising all identified descriptorsCitation18.
Result and discussion
The study has begun in CP-MLR with the individual descriptor classes for the identification of one and two parameter models i.e. baseline models. The base line models offer information content of descriptor classes corresponding to the activity under investigationCitation17. Out of ten descriptor classes, only the Topological (TOPO), 2D-autocorrelation (2DAUTO) and Atom centered fragment (ACF) classes were emerged with one and two-parameter models. The descriptors from these classes were merged and further analyzed in CP-MLR to identify contributing descriptors in modeling the activity. This has resulted in 127 one and two-parameter models sharing 59 descriptors among them. These 59 descriptors were reused to discover the higher models (three and four parameter model) for the activity. This has resulted in 406 three-parameter models and 85 four-parameter models. The following is a selected four-parameter model for the activity from the pool of equations.
In this and all regression equations, n is number of compounds, r is correlation coefficient, Q2 is cross-validated R2 from leave-one-out (LOO) procedure, Q2L3O is cross-validated R2 from leave-three-out procedure (where a group of three compounds are randomly kept outside the analysis each time in such a way that all compounds are in the predictive groups for once), s is standard error of the estimate and F is F-ratio between the variances of calculated and observed activities. The values given in the parentheses (in regression equation) are the 95% confidence limits of the regression coefficients. The ryrand(SD) is the mean correlation coefficient of the regressions in the activity (Y) randomization study with its standard deviation from 100 simulations. In the randomization study, none of the identified models has shown any chance correlation. Additional statistical parameters such as, the “Akaike’s information criterion” (AIC)Citation25,Citation26, the Kubinyi function ‘FIT’Citation27,Citation28 and the Friedman’s “lack of fit” (LOF)Citation29 have also been calculated to further validate the derived QSAR models. The AIC takes into account the statistical goodness of fit and the number of parameters that have to be estimated to achieve that ‘degree of fit’. The Kubinyi function ‘FIT’ closely related to the F-value, proved to be a useful parameter for assessing the quality of the models. The model that produces the lowest AIC value and highest FIT value is considered potentially the most useful. The LOF factor takes into account the number of terms used in the equation and is not biased, as are other indicators, toward large number of parametersCitation30. The equation 1 have been validated with a test set of 12 compounds (; R2T = R2 of test set compounds). The test sets predictions are in agreement with their experimental values ().
Figure 1. Plots of training (*) and test (•) sets predicted activities versus observed activity corresponding to equation 1 and PLS model. Number of compounds in training set is 33 and test set is 12.
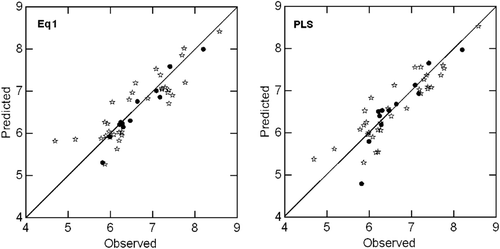
The QSAR equations expected to serve the predictive and diagnostic aspects of the study. As the study is parametric approach, the notional properties of the parameters indicate the plausible forces of interaction between compound and its hypothetical receptor. Accordingly, the descriptors involved in the QSAR equation are discussed. In equation 1, the descriptors MATS1p, GATS6p and GATS7v belong to 2DAUTO class of descriptors. The 2DAUTO descriptors considered in the study have their origin in autocorrelation of topological structure of Broto-Moreau (ATSnw), of Moran (MATSnw) and of Geary (GATSnw)Citation31. The descriptors MATS1p, GATS6p and GATS7v represent the autocorrelations of 1, 6 and 7 path lengths associated with atomic polarizability (p) and atomic van der Waals volumes (v). They suggest the importance of respective lags (length of structural fragment) weighted by physicochemical property in explaining the QC inhibitory activity compounds. The MATS1p and GATS6p showed positive contribution while GATS7v showed negative contribution to the QC inhibitory activity of imidazoles. The complete molecular structure in terms of different lags (fragment lengths) is involved in the computation of these descriptors. Hence, notionally each descriptor refers to whole structure with specific lag. They suggest potential dispersion forces and polar interactions between the compounds and their receptor. The concepts embedded in these descriptors and their computational aspects are discussed in different sourcesCitation15,Citation31. Among these 2DAUTO descriptors, GATS6p showed highest influence on the activity (r = 0.601, ).
Table 2. Pearson correlation coefficient values of parameters of equation 1–3 with QC inhibitory activity of imidazoles.
The H-048 is an atom centered fragment (ACF) descriptorsCitation32. The H-048 represents number of H-atoms attached to an unsaturated carbon (sp2) and the next carbon attached to a hetero atom (-CH*=CR-X like ACF, X = hetero atom)Citation32. In this series of compounds, it refers to the ‘N-C*R2=CR3-N’ ACF of the imidazole ring (comp. no. 1–24 and 30–33 where R2 = H) and (NO2)-CH*=C(N)2 ACF of substituent groups at R1 (comp. no. 10–18, 33 and 41–45). This alone has correlated with activity to the tune of 0.634 (r = −0.634). Its negative coefficient shows that presence of hydrogen in unsaturated carbon in such fragments is detrimental for the QC inhibitory activity. In compounds 25 to 29 and 34–45, the H is replaced with methyl group (R2 = CH3). This may be suggested that methyl group at R2 position is favorable for the activity.
Traditionally hydrophobicity, molar refractivity and Hammett’s sigma are some of the commonly used parameters for explaining the drug–receptor interactions. The non-availability of appropriate substituent constants is an often encountered limitation of these parameters. It is especially true for Hammett’s sigma constants. The DRAGON software provides the estimates of hydrophobicity and molar refractivity of the compounds under variable names MlogP and MR, respectively. For the compounds of this investigation, the MlogP and MR have not entered into any model within the set limits of model search perimeter. However, keeping in view the importance of hydrophobicity and molar refractivity in modeling drug–receptor interactions, we have extended the study to examine the scope of MlogP and MR in equation 1 with MATS1p, GATS6p, GATS7v and H-048 for explaining the activity of these compounds. For the dataset under investigation, the correlation between MlogP and the activity is 0.301 (r = 0.301) whereas the correlation between MR and the activity is 0.673 (r = 0.673). Also, MR and MATS1p are intercorrelated (r = 0.630). The MATS1p being an autocorrelation of lag 1 weighed by polarity, its intercorrelation with MR is not unexpected. In the regression model (Eq. 1) the parameters MATS1p and MR appears to be interchangeable without loss of much significance (Eq. 2). Here, the positive coefficient of MR signifies the role of dispersion or van der Waals forces in drug–receptor interactions.
Among the other descriptors of equation 1, H-048 and MlogP are correlated to the extent of 0.527 (r = −0.527). H-048 is a measure of hydrogens attached to C2(sp3)/C1(sp2)/C0(sp) carbons (X-C2(sp3)-X’; X-C1(sp2)-R; R-C0(sp) where X, X’ are hetero atoms, R is alkyl etc). Indirectly this represents the carbons embedded in the immediate vicinity of heteroatom and/or electron rich surroundings. Due to this H-048 may represent potential electronic as well as hydrophilic fragment(s) in the molecules. Unlike H-048, the MlogP is an attribute of the whole molecule for its hydrophobicity. The negative correlation observed between H-048 and MlogP is justified as they account for mutually opposite molecular forces. The H-048 and the activity are correlated to an extent of 0.634 (r = −0.634). Also, the regression coefficient of H-048 in equation 1 clearly suggests in favor of reducing the C2(sp3)/C1(sp2)/C0(sp) content to improve the activity. The activity of the compounds is poorly correlated with MlogP (r = 0.301) which is a measurement for the composite hydrophobicity of the whole molecule. Also, replacement of H-048 with MlogP (Eq. 3) deteriorated the equation 1.
The above equations while underlining the importance of MR and MlogP in model building for the activity, they also provided the diagnosis of forces involved in drug–receptor interactions.
In modeling studies, different equations take into account different sub-structural regions in terms of descriptors of the compound in explaining the predictive and diagnostic aspects of the chosen phenomenon. A study of different sub-structural regions, in terms of descriptors, provides scope to understand the diagnostic aspects of these regions beyond the individual models. Altogether the CP-MLR has led to the identification of 59 descriptors as contributing structural features to modulate the activity of the compounds under investigation. A PLS analysis has been carried out on these identified descriptors (The MLR-like PLS model for the QC inhibitory activity of imidazoles with CP-MLR identified 59 descriptors is given in of supporting information). In the PLS cross-validation procedureCitation33, three components were found to be the optimum for these fifty nine descriptors to explain the activity. The PLS model elucidates 79.8% of predictive (LOO) as well as 84.6% of explained variance in the QC inhibitory activity of imidazoles (r2 = 0.846, Q2 = 0.798, s = 0.346, F = 53.62). The MLR-like PLS coefficients of these 59 descriptors are given in supporting information. The shows a plot of the fraction contribution of normalized regression coefficients of these descriptors to the activity. The descriptors are ranked according to their fraction contribution in PLS modelCitation33. Out of the 59 descriptors, the top 13 have collectively contributed more than 51% to the explained variance of the activity. The fraction contribution of these descriptors to the activity is shown in gray shaded lines (). They include MATS1v, MATS7v, MATS6p, MATS7p, GATS6v, GATS7v, GATS6p, C-033, C-034, C-001, H-048, H-052 and Lop.
Figure 2. Plot of fraction contribution of MLR-like PLS coefficients (normalized) of the 59 descriptors to the activity. The horizontal axis refers to descriptors’ serial numbers. The 13 most significant descriptors are identified by gray shaded lines.
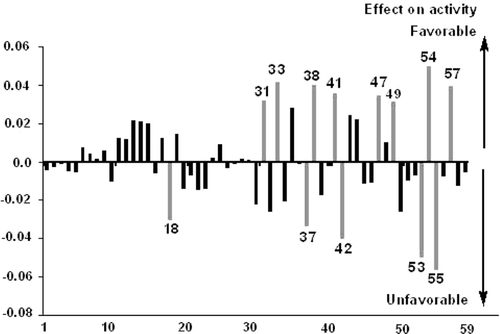
In order to make the structure-activity relations lucid, attempts are made to translate numerical values of the descriptors in terms of desirable structural moieties for the activity. The descriptors H-048, H-052, C-001, C-033 and C-034 are from ACF class. Among these features, H-048 maximally influenced the activity. The H-052 accounts for the number of H atoms attached to a carbon (sp3) with one hetero atom attached to next carbon. Its positive contribution in PLS model suggests that this kind of fragment in imidazole is favorable for the QC inhibitory activity. For these compounds descriptor C-001 represents the count of CH3R fragments. The positive coefficient of this feature in the PLS model suggests its favorability for activity. The C-033 and C-034 represent R-C*H-X and R-C*R-X type of atom centered fragments respectively, where X is a hetero atom and R is an aliphatic groupCitation32. In these compounds, C-033 refers to the N–C*R2=C*R3-N atom centered fragments in the imidazole ring where R2=R3=H (comp. no 1–23). The C-033 shows that hydrogen at R2 or R3 is unfavorable for the activity. C-034 account for presence of methyl group (-CH3)’ at R2 or R3 in imidazole ring (comp. 24–45) and is favorable for the activity. In the PLS model C-033 and C-034 showed negative and positive influence on the activity, respectivelyCitation33. Collectively, these two descriptors indicate that methyl substituted imidazole ring is better for the QC inhibitory activity.
The significant descriptors from 2DAUTO class include MATS1v, MATS7v, MATS6p, MATS7p, GATS6v, GATS7v, GATS6p (). Among these, the descriptors MATS1v, MATS7v, MATS7p, GATS6v and GATS6p showed positive influence on QC inhibitory activity of imidazoles while the others namely MATS6p and GATS7v showed negative influence on the inhibitory activity (; Ref.Citation33). The lags and weights of 2DAUTO descriptors respectively suggest the involvement of structural fragment of specific length and physicochemical/electronic property in explaining the activity. The foregoing 2DAUTO descriptors highlight the importance of fragments of 6-7 lags weighed by atomic van der Waals volumes and polarizability. It may be suggested that the polarizability of specific structural fragments may modulate the inhibitory activity through dispersion interactions. Several other descriptors in PLS model such as X0v (chi zero order average connectivity index), ATS1v, ATS4v, ATS6v, ATS3p and ATS7p (Broto-Moreau autocorrelation descriptors, v is atomic van der Waals volumes and p is atomic polarizability) are highly correlated with molar refractivity (r > 0.8). These findings support the effect of bulkiness and atomic polarizability of imidazoles on their glutaminyl cyclase inhibitory activity.
Among the topological class of descriptors, Lop (Lopping centric index) is the most significant one. Its negative coefficient in PLS model suggest that a decreasing lop centricity is favorable for the activity. The above discussed 13 descriptors have collectively accounted for more than 51% variance in the explained activity. All these suggest a step towards modifying these analogues in optimizing the activity. The remaining descriptors showed lower order of significance in comparison to these 13 descriptors (). A brief description of all the 59 descriptors is given in supporting information. More details of these descriptors are available in referenceCitation15.
Conclusions
In summary, the QC inhibitory activity of imidazoles () has been found to be correlated with 2DAUTO and atom centered fragment descriptors. The descriptors from these classes were used to develop MLR and PLS models. The descriptor from 2DAUTO class showed that Moran and Geary autocorrelation descriptors of one, six and seven path length associated with atomic van der Waals volume or atomic polarizability as physicochemical property hold scope for modulating QC inhibitory activity of imidazole analogue. The ACF class of descriptors, H-048, H-052, C-001, C-033 and C-034 show significance of different atom centered fragments in imidazoles to the QC inhibitory activity. The H-048 shows that presence of hydrogens on unsaturated carbon is not favorable for the activity. The H-052 suggest for the more number of hydrogen on unsubstituted carbon (sp3) for better activity. The C-033 and C-034 collectively suggested that methyl substituted imidazole ring for better activity. An empirical parameter molar refractivity is conducive for the activity. The descriptors identified in the study collectively highlighted the importance of molecular volume, molar refractivity and polarizability to the QC inhibitory activity. These features play important role in the binding of an inhibitor with the receptor. They also reflect the nature of complimentary binding sites in the glutaminyl cyclase. The study contribute to the understanding of the designing of new/novel imidazoles in terms of optimum molecular fragment lengths, their physicochemical properties and atom centered fragments for better QC inhibitory activity. The developed MLR and PLS models are statistically significant and can be used for In Silico activity prediction of new compounds.
Supplementary data
Molecular descriptors for structure database; PLS factor scores, loadings, weights and sensitivity of independent and dependent descriptors of the PLS models.
Supplementary Material
Download PDF (327.7 KB)Acknowledgement
Authors thank Director, CDRI, Lucknow and Chairman, ISF College of Pharmacy, Moga for the facilities to carry out the research work.
Declaration of interest
The authors report no conflicts of interest.
References
- Awade AC, Cleuziat P, Gonzales T, Robert-Baudouy J. Pyrrolidone carboxyl peptidase (Pcp): An enzyme that removes pyroglutamic acid (pGlu) from pGlu-peptides and pGlu-proteins. Proteins 1994;20:34–51.
- Van Coillie E, Proost P, Van Aelst I, Struyf S, Polfliet M, De Meester I, Harvey DJ, Van Damme J, Opdenakker G. Functional comparison of two human monocyte chemotactic protein-2 isoforms, role of the amino-terminal pyroglutamic acid and processing by CD26/dipeptidyl peptidase iv. Biochemistry 1998;37:12672–12680.
- Busby WH, Jr., Quackenbush GE, Humm J, Youngblood WW, Kizer JS. An enzyme(s) that converts glutaminyl-peptides into pyroglutamyl-peptides. Presence in pituitary, brain, adrenal medulla, and lymphocytes. J Biol Chem 1987;262:8532–8536.
- Sykes PA, Watson SJ, Temple JS, Bateman RC Jr.. Evidence for tissue-specific forms of glutaminyl cyclase. FEBS Lett 1999;455:159–161.
- Bockers TM, Kreutz MR, Pohl T. Glutaminyl-cyclase expression in the bovine/porcine hypothalamus and pituitary. J Neuroendocrinol 1995;7:445–453.
- Pohl T, Zimmer M, Mugele K, Spiess J. Primary structure and functional expression of a glutaminyl cyclase. Proc Natl Acad Sci USA 1991;88:10059–10063.
- Morgan C, Colombres M, Nunez MT, Inestrosa NC. Structure and function of amyloid in Alzheimer’s disease. Prog Neurobiol 2004;74:323–349.
- Schilling S, Zeitschel U, Hoffmann T, Heiser U, Francke M, Kehlen A, Holzer M, Hutter-Paier B, Prokesch M, Windisch M, Jagla W, Schlenzig D, Lindner C, Rudolph T, Reuter G, Cynis H, Montag D, Demuth HU, Rossner S. Glutaminyl cyclase inhibition attenuates pyroglutamate Abeta and Alzheimer’s disease-like pathology. Nat Med 2008;14:1106–1111.
- Miravalle L, Calero M, Takao M, Roher AE, Ghetti B, Vidal R. Amino-terminally truncated Abeta peptide species are the main component of cotton wool plaques. Biochemistry 2005;44:10810–10821.
- Schilling S, Hoffmann T, Manhart S, Hoffmann M, Demuth HU. Glutaminyl cyclases unfold glutamyl cyclase activity under mild acid conditions. FEBS Lett 2004;563:191–196.
- Schilling S, Lauber T, Schaupp M, Manhart S, Scheel E, Bohm G, Demuth HU. On the seeding and oligomerization of pGlu-amyloid peptides (in vitro). Biochemistry 2006;45:12393–12399.
- Youssef I, Florent-Bechard S, Malaplate-Armand C, Koziel V, Bihain B, Olivier JL, Leininger-Muller B, Kriem B, Oster T, Pillot T. N-truncated amyloid-beta oligomers induce learning impairment and neuronal apoptosis. Neurobiol Aging 2008;29:1319–1333.
- Russo C, Violani E, Salis S, Venezia V, Dolcini V, Damonte G, Benatti U, D’Arrigo C, Patrone E, Carlo P, Schettini G. Pyroglutamate-modified amyloid beta-peptides-abetaN3(pE)-strongly affect cultured neuron and astrocyte survival. J Neurochem 2002;82:1480–1489.
- Buchholz M, Hamann A, Aust S, Brandt W, Bohme L, Hoffmann T, Schilling S, Demuth HU, Heiser U. Inhibitors for human glutaminyl cyclase by structure based design and bioisosteric replacement. J Med Chem 2009;52:7069–7080.
- Dragon software version 3.0-2003 and manual. Todeschini R, Consonni V, Mauri A, Pavan M. Milano, Italy. http://disat.Unimib.It/chm/Dragon.Htm.
- Prabhakar YS. A combinatorial approach to the variable selection in multiple linear regression analysis of Selwood et al. data set- A case study. QSAR Comb Sci 2003;22:583–595.
- Gupta MK, Prabhakar YS. Topological descriptors in modeling the antimalarial activity of 4-(3’,5’-disubstituted anilino)quinolines. J Chem Inf Model 2006;46:93–102.
- Saquib M, Gupta MK, Sagar R, Prabhakar YS, Shaw AK, Kumar R, Maulik PR, Gaikwad AN, Sinha S, Srivastava AK, Chaturvedi V, Srivastava R, Srivastava BS. C-3 alkyl/arylalkyl-2,3-dideoxy hex-2-enopyranosides as antitubercular agents: synthesis, biological evaluation, and QSAR study. J Med Chem 2007;50:2942–2950.
- ChemDraw Ultra 6.0 and Chem3D Ultra, Cambridge Soft Corporation, Cambridge, USA.
- Prabhakar YS, Rawal RK, Gupta MK, Katti SB. CP-MLR/PLS directed structure-activity modeling of the HIV-1RT inhibitory activity of 2,3-diaryl-1,3-thiazolidin-4-ones. QSAR Comb Sci 2004;23:234–244.
- Gupta MK, Sagar R, Shaw AK, Prabhakar YS. CP-MLR directed QSAR studies on the antimycobacterial activity of functionalized alkenols-topological descriptors in modeling the activity. Bioorg Med Chem 2005;13:343–351.
- Prabhakar YS, Gupta MK, Roy N, Venkateswarlu Y. A high dimensional QSAR study on the aldose reductase inhibitory activity of some flavones: topological descriptors in modeling the activity. J Chem Inf Model 2006;46:86–92.
- Gupta MK, Prabhakar YS. QSAR study on tetrahydroquinoline analogues as plasmodium protein farnesyltransferase inhibitors: a comparison of rationales of malarial and mammalian enzyme inhibitory activities for selectivity. Eur J Med Chem 2008;43:2751–2767.
- Sharma S, Sharma BK, Prabhakar YS. Juglone derivatives as antitubercular agents: a rationale for the activity profile. Eur J Med Chem 2009;44:2847–2853.
- Akaike H. Information theory and an extension of the minimum likelihood principle. Akademiai kiado: Budapest. 1973:267–281.
- Akaike H. A new look at the statistical identification model. IEEE Trans Autom Control 1974:AC-19:716–723.
- Kubinyi H. Variable selection in QSAR studies. I. An evolutionary algorithm. Quant Struct-Act Relat 1994;13:285–294.
- Kubinyi H. Variable selection in QSAR studies. II. A highly efficient combination of systematic search and evolution. Quant Struct-Act Relat 1994;13:393–401.
- Friedman J. Technical Report No. 102. Laboratory for Computational Statistics. In Stanford University: Stanford; 1990.
- Sharma BK, Pilania P, Singh P. Modeling of cyclooxygenase-2 and 5-lipooxygenase inhibitory activity of apoptosis-inducing agents potentially useful in prostate cancer chemotherapy: derivatives of diarylpyrazole. J Enzyme Inhib Med Chem 2009;24:607–615.
- Broto P, Moreau G, Vandycke C. Molecular structures: perception. Autocorrelation descriptor and SAR studies. Eur J Med Chem -Chim Ther 1984;19:66–70.
- Viswanadhan VN, Ghose AK, Revankar GR, Robins RK. Atomic physicochemical parameters for three dimensional structure directed Quantitative Structure-Activity Relationships. 4. Additional parameters for hydrophobic and dispersive interactions and their application for an automated superposition of certain naturally occurring nucleoside antibiotics. J Chem Inf Comput Sci 1989;29:163–172.
- Wold S. Cross-validatory estimation of the number of components in factor and principal component models. Technometrics 1978;20:397–405.