Abstract
Dithiocarbamates (DTC) are promising compounds with potential applications in antitumoral and glaucoma therapy. Our aim is to understand molecular features affecting DTC interaction with carbonic anhydrases (CAs), zinc-containing enzymes maintaining acid-base balance in blood and other tissues. To this end, we generate QSAR models based on a compound series containing 25 DTC, inhibitors of four human (h) CAs isoforms: hCA I, II, IX and XII. We establish that critical physicochemical parameters for DTC inhibitory activity are: hydrophobic, electronic, steric, topological and shape. The predictive power of our QSAR models is indicated by significant values of statistical coefficients: cross-validated correlation q2 (0.55–0.73), fitted correlation r2 (0.75–0.84) and standard error of prediction (0.47-0.23). Based on the established QSAR equations, we analyse 22 new DTC derivatives and identify DTC dicarboxilic acids derivatives and their esters as potentially improved inhibitors of CA I, II, IX and XII.
Introduction
DTCs recently discovered as CAs inhibitors have a wide range of applications in various fields ranging from agriculture to medicine. Several recent studiesCitation1–4 describe DTCs as effective antitumoral and anti-glaucoma agents. Some of these studiesCitation1,Citation2 demonstrated that DTCs, with the general formula R1R2N-CSS−M+, inhibit in the low micromolar or submicromolar range several hCA isoforms (hCA I, II, IX and XII) deeply involved in tumorigenesis and eye diseases. Furthermore, it was proved that some of these compounds possess intraocular pressure (IOP) lowering properties in an animal model of glaucoma, making them interesting candidates for developing anti-glaucoma and also antitumoral drugsCitation1,Citation2. A series of N-mono- and N,N-disubstituted DTC have been investigated as inhibitors of β-CAs from the fungal pathogens Cryptococcus neoformans, Candida albicans and Candida glabrata and from the bacterial pathogen Mycobacterium tuberculosisCitation4,Citation5. DTCs inhibited these enzymes with efficacies between subnanomolar to the micromolar range, depending on the substitution pattern at the nitrogen atom from the dithiocarbamate zinc-binding group.
Despite the positive pharmacological applications of DTCs, toxic and teratogenic effects of these compounds were reportedCitation6–9. These effects are induced by great chelating capacity of DTCs, especially in fish speciesCitation7,Citation8. Segovia et al.Citation6 demonstrate in their SAR study that the degree of toxicity of DTCs correlates with three molecular features: (i) molecular weight of the compound; (ii) the molecular weight of the smallest chemical radical linked to the DTC group; and (iii) the number of benzene rings in the molecule. Their results show that, in general terms, the toxicity of DTCs decreases exponentially with increasing molecular weight and the number of benzene rings.
Under these circumstances, the quantitative structure-activity relationship (QSAR) approachCitation10–12 represents a suitable alternative to predict the biological activity of new DTCs. Basically, all QSAR methods consider that macroscopic properties are induced by molecular structure and every change into molecular structure leads to modification of these properties. A QSAR generally takes the form of a linear equation: Biological Activity = const+ (c1 * p1) + (c2 * p2) + … + (cn * pn), where parameters p1 through pn describe molecular properties like: molecular volume, dipole moment, etc. and the coefficients c1 through cn are calculated by fitting variations in the parameters and the biological activity.
Only few structure-activity relationship (SAR) and QSAR studies correlated the antitumoral effects of DTCs with various macroscopic descriptorsCitation1,Citation3,Citation13,Citation14. These studies significantly enhance our knowledge on the inhibitory effect of DTCs against crucial human enzymes and, more importantly, propose new DTCs chemical structures with improved inhibitory effect. Wang XJ et al.Citation3 designed, synthesized and tested in vitro antitumor effect of three series of butenolide-containing dithiocarbamates. Their structure-activity relationship analysis showed that the introduction of dithiocarbamate side chains on the C-3 position of butenolide was crucial for antitumor activity. de Paula da Silva et al.Citation12 have used molecular modelling, QSAR and docking methods to study 18 dithiocarbamate compounds as superoxide dismutase (SOD) inhibitors. In this study, a good correlation between experimental and predicted biological activities was obtained using descriptors like: superficial area, heat of formation and logarithm of the partition coefficient, charge of the nitrogen atom from the dithiocarbamate group and charges of the two carbon atoms adjacent to that nitrogen.
SARs study performed by Carta F et al.Citation1 for the inhibition of isoforms hCA I, II, IX and XII concluded that small modifications of compound backbone lead to dramatic changes of biological activity. In this study, the inhibition mechanism of the DTCs was also explained, by resolving the X-ray crystal structure for hCA II complexes with a heterocyclic DTC. The results show that: (i) CS2 in DTCs confers a new zinc-binding function, since it is directly coordinated to the Zn (II) ion from the enzyme active site and also participates in an interaction (hydrogen bond) with the OH moiety of Thr199; (ii) the organic scaffold of the DTC is deeply buried within the enzyme active site and also participates in favourable interactions with it, which leads to a high stabilization of the enzyme–inhibitor adduct; (iii) some of the most potent CAIs detected here showed favourable IOP lowering effects in an animal model of glaucoma. This new class of CAIs may constitute interesting candidates for developing novel anti-glaucoma therapies.
Our study represents an extension of the previous studyCitation1 and an attempt to generate highly accurate QSAR models reflecting DTCs inhibitory activity against hCA I, II, IX and XII. Thus, we establish the contribution of nine molecular descriptors to inhibitory activity of DTCs against hCA isoforms: hydrophobic character expressed by logarithm of the partition coefficientCitation15, internal dipole momentCitation16, compounds polarizabilitiesCitation17, van der Waals surface area of polar and hydrophobic atomsCitation18, hydrophobic atoms countCitation19, rigid and rotatable bonds countCitation20 and compounds shape expressed as globularityCitation21. Also, we propose new derivatives selective for hCA I, II, IX, XII isoforms.
Besides, in agreement with experimental dataCitation1,Citation2,Citation6 showing that descriptors like compounds structure and size are important not only for inhibitory effects of DTC but also for their side effects, our 2D-QSAR models are able to predict both the inhibitory activity of DTCsCitation1 and their side effects. Our major goal is to better understand the inhibitory mechanism and to propose new effective DTCs derivatives to be used in anti-cancer and anti-glaucoma therapies. To this end, we designed 22 new derivatives based on DTC 24a templateCitation1 and predicted their inhibitory activities against hCA I, II, IX and XII isoforms, based on the 2D-QSAR models proposed here. As novelty, we successfully propose several candidates with higher specificity for all four isoforms, knowing that 24a displays higher inhibitory effect for hCA I and hCA II but lower on hCA IX and hCA XIICitation1.
Materials and methods
Dataset for analysis
The inhibitory activity of 25 DTCs against hCAs, expressed as the dissociation constant for the enzyme-inhibitor complex (Ki), was compiled from literatureCitation1. Ki activity originally determined in nanomolar values was converted to a pKi value by taking Log (1/Ki). The pKi values were used as the dependent variable in all QSAR models subsequently developed in this study. This data set was selected according to the following criteria: (i) several compounds have strong inhibitory activity on hCAs; (ii) wide range of experimental inhibitory activities (Ki from 0.88 to 1838 nM at hCA I; Ki from 0.70 to 6910 nM at hCA II; Ki from 3.6 to 1413 nM at hCA IX; Ki from 0.76 to 1105 nM at hCA XII); (iii) different inhibition mechanisms compared with classic CA inhibitors like sulfonamides or trithiocarbonateCitation1; (iv) wide range of DTCs incorporating various aliphatic and/or aromatic moieties at the nitrogen atom; (v) in vitro proven anti-glaucoma effects on animal model. The names, chemical structures and experimental pKi of DTCs are given in .
Table 1. Chemical structures and experimental Ki and pKi of dithiocarbamates inhibitors of carbonic anhydrases hCA I, hCA II, hCA IX and hCA XII R1R2N-CSSH.
Molecular modelling and minimum energy calculation of DTCs
Molecular modelling and minimum energy evaluation of compounds were performed by AM1 semi-empirical method, Conjugate-Gradient algorithm, convergence 0.01 in HyperChemCitation21. In the first step, we generated inhibitors’ 2D structures with corresponding hydrogen atoms. These were then automatically converted into inhibitors’ 3D structures, which were saved in HyperChem specific files. An important objective of the study was to determine the pharmacological characteristics of new derivatives of DTC, obtained by computational design, starting from the DTC 24a. We chose DTC 24a as template for new DTC derivatives in respect with chemical, crystallographic and in vivo tests informationCitation1. It was demonstrated that DTC 24a have very good inhibitory activity for all four hCA isoforms (pKi hCA I = 9.06), (pKi hCA II = 9.02), (pKi hCA IX = 8.21), (pKi hCA XII = 8.47). Also, tests on animal models indicated that 24a is a very good candidate for glaucoma therapies in comparison with other DTC compoundsCitation1. We consider that DTC 24a is appropriate to represent a common template molecule for all four QSAR models, even though the pKi values are not identical for all hCA isoforms, but are very similar. The same remark cannot be made for other compounds considered.
Thus, 22 derivatives obtained from DTC 24a, proposed as possible potent inhibitors of all four hCA isoforms, were modelled and energetically optimized according to the previously described protocols. Modelling strategy included: (i) modifications of the count of electronic and polar contacts by adding various substituents on morpholine ring-hydroxyl, cetoxy, thiocarbonyl, thio-carbon, amino, mono and di-carboxylic acids on and their esters and amides; and (ii) modifications of the count of hydrophobic contacts by adding aliphatic saturated and unsaturated substituents (). All 22 new DTCs derivatives were tested as possible inhibitors of all four I, II, IX and XII hCA isoforms based on the QSAR equations obtained in the present study ().
Descriptors calculation
Initially, we calculated 23 descriptors belonging to two categories: (i) 2D descriptors including physical properties: steric (subdivided van der Waals surface and volume, solvent accessible surface and volume), atom and bond counts (hydrophobic/polar, donor /acceptor atoms, rigid and rotatable bonds) and electronic descriptors (molecular polarizability, molar refractivity, dipole moment); and (ii) 3D molecular descriptors including potential energy descriptors and globularity. In the end, we selected a set of descriptors which was small enough to avoid redundancy and chance correlation, but large enough to allow an accurate validation of QSAR modelsCitation22–24.
The critical descriptors kept in our study were: molecular polarizability (apol)Citation17,Citation21; logarithm of the octanol/water partition coefficient (logP(o/w))Citation15,Citation21; rotatable and rigid bonds count (brot, brigid)Citation20; hydrophobic atoms count (a-hyd)Citation19; dipole moment (dipole)Citation16,Citation21; van der Waals surface areas of hydrophobic/polar atoms (vsa-hyd, vsa-pol)Citation18,Citation21 and globularity (glob)Citation21. A short description of descriptors is presented in .
Table 2. Short description of descriptors used to predict inhibitory activity of DTCs.
Initially in the 2D-QSAR models, descriptors were considered as individual independent variables or in various combinations to predict the inhibitors activity. To avoid redundancy of independent variables and to ensure no chance correlation, the inter-correlation of the independent variables matrix was calculated according to Pearson correlation methodCitation25–27 ().
Table 3. Pearson correlation matrix of independent variables.
In our study, the relevant QSAR equations from statistical point of view included:
QSAR model 1: pKi hCAI = constant+c1*vsa-hyd+c2*glob+c3*b-rigid+c4*b-rot
QSAR model 2: pKi hCAII = constant+c5*vsa-pol+c6*logP(o/w)+c7*b-rigid+c8*apol
QSAR model 3: pKi hCAIX = constant+c9*apol+c10*b-rigid+c11*b-rot+c12*dipole
QSAR model 4: pKi hCAXII = constant+c13*a-hyd+c14*vsa-pol+c15*apol
Statistical calculations
Parameters used to estimate the predictive power of QSAR models were q2- (cross-validated r2)Citation23 and fitted correlation r2Citation24 while validation criteria were: q2 not less than 0.55 and r2 higher than 0.70.
A reliable equation for structure activity relationship should possess a high correlation coefficient, low standard error of estimate prediction (SEE)Citation25–27 and the least possible number of variables. In our study the q2-cross validated and optimum number of PLS components (n = 4)Citation24 were evaluated by applying the cross-validation procedure and the Partial Least Squares (PLS) algorithm. Further, multiple linear regression analysis (MLRA)Citation25,Citation26 was used to generate the control criteria correlation coefficient r2, standard error of estimate prediction and Fisher testCitation25–27.
Training and testing sets
Generation of consistent statistical models depends on the quality of both training and testing sets in terms of structural diversity and property values distribution. From the original data set of 25 DTCs, the structures were partitioned into a training set and a testing set of five or six compounds at random with bias given to both chemical and biological diversity in both the training and testing set molecules (). Several statistically significant QSAR models were generated, with different testing sets, as follows: QSAR model 1 testing set: 4a; 5a; 12a; 16a; 23a; 25a; QSAR model 2 testing set: 13a, 14a, 16a, 20a, 24a; QSAR model 3 testing set: 1a; 4a; 18a; 19a; 21a; 24a; QSAR model 4 testing set: 8a; 9a; 14a; 19a; 24a. A statistical cluster analysis confirmed that the composition of both training and testing sets is representative for the whole data set ().
Table 4. Summary of 2D-QSAR statistical parameters for QSAR models 1–4.
Table 5. QSAR predicted pKi of dithiocarbamates against human carbonic anhydrases and residual values for QSAR models 1-4.
Results
Here we generate multiple QSAR models to predict the DTCs inhibitory activity against hCA. The novelty of our approach consists in two features: (i) our dataset contains novel DTC compounds with inhibitory mechanism against hCA, as demanded by the complexity of tumorigenesis pathologies; and (ii) our models take into account the molecular descriptors with a crucial contribution to biological activity, as indicated by experimental evidenceCitation1.
Initial models, using only individual contributions of descriptors, lead to a very poor correlation between experimental and predicted inhibitory activity against hCA, as described by the statistical parameters: q2 less than 0.40; r2 less than 0.7; SEE higher than 0.50. QSAR models 1–4 using combinations of descriptors detailed in “Experimental” show a clear improvement of statistical coefficients: q2 greater than 0.55; r2 greater than 0.75; SEE less than 0.45. presents the statistical parameters computed for training set: cross-validated correlation q2 fitted coefficient r2, standard error of prediction SEE, the Fisher F and QSAR regression coefficients.
The predictive power of the 2D-QSAR models was assessed by predicting pKi values for testing set molecules. shows the values for predicted pKi of the DTCs belonging to training and testing sets and also the residual values (the differences between pKi experimental and pKi predicted). Bold format indicates molecules in testing set.
We demonstrate that generally good correlations between experimental and predicted biological activities were obtained for all training and testing molecules, for each QSAR model (residual values (0.00–2.17)). Data in is supported by the correlations between experimental and predicted inhibitory activities of DTC against hCA I, II, IX and XII ().
Figure 1. Correlation between experimental and predicted pKi of dithiocarbamates: (a) QSAR model 1 (q2 = 0.66, r2 = 0.80); (b) QSAR model 2 (q2 = 0.70, r2 = 0.80); (c) QSAR model 3 (q2 = 0.55, r2 = 0.75); (d) QSAR model 4 (q2 = 0.73, r2 = 0.84).
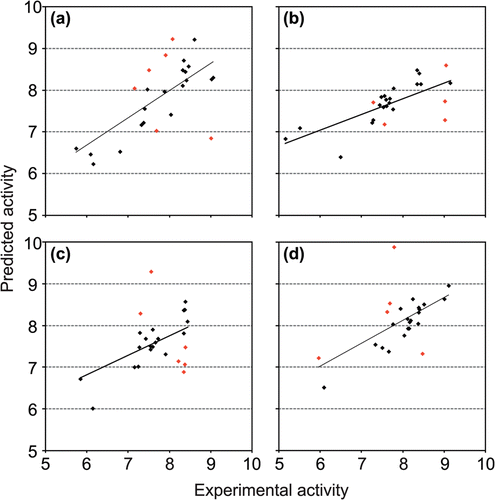
Accordingly, our major goal was to develop a series of DTC derivatives with high affinities for all four isoforms hCA. For this we used as template the very effective DTC inhibitor 24a. The predictive ability of QSAR-models 1–4 () was used to predict the inhibitory activity of these new derivatives (). Bold letters correspond to compounds with higher inhibitory activity. Italic letters indicate compounds with higher activity simultaneously on hCA I, II, IX and XII.
Table 6. Predicted pKi of 24a derivatives, indicating inhibition against hCA I (QSAR model 1), hCA II (QSAR model 2), hCA IX (QSAR model 3), hCA XII (QSAR model 4).
Most of the new derivatives are potent inhibitors against one to three hCA isoforms at most, but there are some exceptions which display a high inhibitory activity for all four CA isoforms, measured by residual activity (the difference between predicted pKi and experimental pKi of parent analogs): 24a-6 (residual activity = 8.22–11.26), 24a-9 (residual activity = 8.28–11.26); 24a-17 (residual activity = 8.82–10.25); 24a-18 (residual activity = 8.90–10.37); 24a-19 (residual activity = 9.49–10.28); 24a-21 (residual activity = 9.24–10.12 ). At the other end of the spectrum are derivatives that do not improve the activity of parent compound, such as 24a-1 (residual activity = 7.17–8.15) and 24a-2 (residual activity = 7.20–8.74) (). Data in is supported by graphical representations of residual values for 24a derivatives obtained in QSAR models 1–4 ( and ). Here we represent the possibly reduced or enhanced antitumoral effect of 24a derivatives by inhibitory activity against hCA I and hCA II isoforms () and possibly reduced or enhanced anti-glaucoma effect of 24a derivatives by inhibitory activity against hCA IX and hCA XII isoforms ().
Discussions
Referring to inhibition of hCAI, our results are generally in agreement with experimental resultsCitation1 indicating a good correlation between experimental and predicted biological activities of DTCs. It is important to emphasize a positive contribution of structure-derived descriptors like globularity and bonds count instead of steric descriptor. In QSAR model 1, the best agreement between experimental and predicted biological activities was seen for compounds 7a (R1 = H, R2 = PhCH2, residual values = 0.05), 11a (R1 = H, R2 = KOOCCH2, residual values = 0.08) and 13a (R1 = Me, R2 = Me, residual values = 0.07). Unexpectedly, a poor correlation between experimental and predicted biological activities was obtained for DTC 16a (R1 = iso-butyl, R2 = iso-butyl residual value = 2.17) in comparison with its isomer DTC 18a (R1 = n-butyl, R2 = n-butyl, residual value = 0.15). We explain this result by different globularity and bonds count induced by iso-butyl and n-butyl moieties. In good agreement with experimental data is also the correlation between experimental and predicted biological activity for heterocyclic compounds of series 24a (R1=O[(CH2CH2)]2, residual value = 0.76), 25a (R1=NaS(S=C)N[(CH2CH2)]2, residual value = −0.93) and 27a (R1 = (S)-[CH2CH2CH2CH(COONa)], residual value = −0.61). For the morpholine DTC 24a, the best DTC hCA I inhibitor ever describedCitation1, we obtained a very good correlation between predicted and experimental biological activities, this allowing us to predict the inhibitory activity of its new derivatives based on QSAR model 1 equation.
Generally, QSAR model 2 illustrating the inhibition of the physiologically dominant cytosolic isoform hCA IICitation1 is in good agreement with experimental data. We notice a positive contribution to predicted biological activity of DTC induced by steric, hydrophobic and bonds count descriptors but not by electronic descriptor. Our results are supported by the experimental findingsCitation1 indicating that the length of the alkyl chain and also the type of substituents also strongly influence activity. The best agreement between experimental and predicted biological activities was obtained for 12a (R1 = H, R2 = imidazol-1-yl-(CH2)3, residual value = 0.00), 21a (R1 = HOCH2CH2, R2 = HOCH2CH2, residual value = 0.00), 4a (R1 = H, R2 = 2-butyl, residual value =−0.05), 17a (R1 = n-Pr, R2 = n-Pr, residual value = 0.05), 18a (residual value = 0.01), 22a (R1 = Me, R2 = Ph residual value = −0.02). Also, for heterocyclic compounds 24a, 25a and 27a we obtained a satisfactory correlation between activities (residual values = 0.23–1.29). Similar to the previous QSAR model, the predictive biological activity was in good correlation with experimental value, this giving us the possibility to study 24a derivatives as possible hCA II inhibitors.
For QSAR model 3 reflecting the inhibition against the tumour-associated isoform hCA IX, the PLS regression resulted in a satisfactory predicted activity for compounds 12a (residual value = 0.00); 5a (R1 = H, R2 = O[(CH2CH2)]2N(CH2)2, residual value = 0.02), 7a (residual value = 0.02) and 2a (R1 = H, R2 = O[(CH2CH2)]2N, residual value = 0.02). QSAR model 3 suggests that effective inhibition of hCA IX is positively influenced by polarizability and bonds count and negatively influenced by dipole moment.
In QSAR model 4, predicted biological activity was in good correlation with experimental biological activity at hCA XII for almost all DTCs, especially for 21a–23a and 27a (residual values = 0.01–0.04). It is important to note that in case of inhibition against hCA XII all three descriptors: steric, electric and structure-based have a positive contribution. For the best inhibitors of series 16a and 25a the predicted biological activity was in good correlation with the experimental value.
The previously synthesized DTCs showed a rather promiscuous inhibitory activity against all four hCA isoforms, although each of these hCAs had a different inhibition profile with each of these compoundsCitation1. The representative examples are DTC 15a which is a subnanomolar inhibitor of hCA I and inhibits the remaining three isoforms with Ki values in the range of 27.5 nM−70.4 nM, or DTC 17a with Ki ranging from 7 nM (hCA XII) to 1838nM (hCA I)Citation1.
Regarding design of new DTCs as improved inhibitors of hCA isoforms I, II, IX and XII based on 24a derivatives, our results suggest that two factors should be considered. First, addition of bulky groups like di-carboxylic acids and their unsaturated esters at morpholine ring improves DTC inhibitory activity against all four hCA isoforms. Second, the presence of methyl and hydroxyl radicals at morpholine ring drastically decreases the inhibitory activity against these hCA isoforms.
We propose that the pharmacophore model of DTC 24a derivatives with high inhibitory activities against all four hCA isoforms should simultaneously have the following properties: (i) smaller dipole moment than parent compound; (ii) increased values for the number of hydrophobic atoms, rigid and rotatable bonds and surface area. This is clearly depicted in illustrating the spatial distribution of the electrostatic field and hydrophobicity on the surface area for DTC 24a and its derivatives with lowest and highest activity (derivatives 24a-1 and 19, respectively). It is also remarkable that the positively charged regions (red) are almost equally distributed on compounds 24a and 24a-1 but there is a large distribution of negatively charged (blue) regions. This can explain the low dipole moment and high value of polarizability for 24a-19, compared to derivative 24a-1. On the other hand, the distribution of hydrophobic (green) and polar (blue) regions is significantly different among three compounds, with a much higher hydrophobic (green-brown) and also polar on derivative 19. Not the same remark can be made for derivative 24a-1 and parent compound 24a where the distributions of hydrophobic and polar areas are almost equivalent.
Figure 3. The distribution of electrostatic (up) and hydrophobic (down) area of 24a-1(left), 24a (middle) and 24a-19 (right).
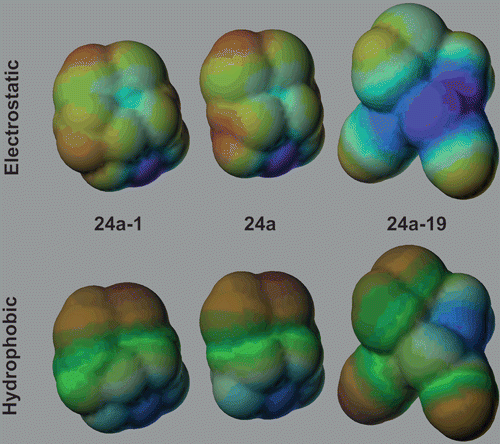
Furthermore, we suggest that our proposed 24a derivatives, 24a-6 (MW = 251.27 Da), 24a-17(MW = 277.31 Da), 24a-18(MW = 275.29 Da), 24a-19 (MW = 299.32 Da) and 24a-21 (MW = 303.35 Da) vs 24a (MW = 163.25 Da ) might have a lower toxicity, in agreement with experimental data indicating that these effects decrease with increased molecular weightCitation6. Focusing on the toxicity of these derivatives, based on experimental results showing that a higher number of benzene rings leads to reduced toxic effectCitation6 we may suggest that the higher count of hydrophobic atoms will also induce a low toxic effect.
Conclusions
Here, we report 2D-QSAR studies on a series of 25 DTCs already tested as inhibitors of four isoforms of hCAs and also on 22 new derivatives of DTC 24a, a potent inhibitor against all four hCA isoforms. DTCs were used to identify the physicochemical properties which are most important for the inhibitory activity. In our study we considered the number of hydrophobic atoms, the count of rotatable and rigid bonds supplemented by molecular area of hydrophobic and polar atoms, dipole moment and polarizability and globularity. Significant statistical coefficients like q2 cross-validated r2, fitted correlation r2 and standard error of estimated prediction were obtained for all four QSAR models (), when using appropriate descriptors: (i) van der Waals area induced by hydrophobic atoms, globularity, count of rigid and rotatable bonds; (ii) van der Waals area induced by polar atoms, hydrophobicity, count of rigid bonds and polarizability; (iii) count of rigid and rotatable bonds, polarizability and dipole; (iv) hydrophobic atoms count, van der Waals area induced by polar atoms and polarizability. The judicious modulation of physicochemical properties may be useful in designing new inhibitors of hCA isoforms with a wide spectrum of medical applications. Based on the QSAR equations established in this study, a set of 22 new DTC was proposed and used to predict the inhibitory activity against hCA. We show that it is critical for hCA inhibition to simultaneously decrease the dipole moment and increase values of the number of hydrophobic atoms, rigid and rotatable bonds and subdivided surface area.
Declaration of interest
The authors declare no conflicts of interest.
References
- Carta F, Aggarwal M, Maresca A, Scozzafava A, McKenna R, Masini E et al. Dithiocarbamates strongly inhibit carbonic anhydrases and show antiglaucoma action in vivo. J Med Chem 2012;55:1721–1730.
- Carta F, Aggarwal M, Maresca A, Scozzafava A, McKenna R, Supuran CT. Dithiocarbamates: A new class of carbonic anhydrase inhibitors. Crystallographic and kinetic investigations. Chem Commun (Camb) 2012;48:1868–1870.
- Wang XJ, Xu HW, Guo LL, Zheng JX, Xu B, Guo X et al. Synthesis and in vitro antitumor activity of new butenolide-containing dithiocarbamates. Bioorg Med Chem Lett 2011;21:3074–3077.
- Monti SM, Maresca A, Viparelli F, Carta F, De Simone G, Mühlschlegel FA et al. Dithiocarbamates are strong inhibitors of the β-class fungal carbonic anhydrases from Cryptococcus neoformans, Candida albicans and Candida glabrata. Bioorg Med Chem Lett 2012;22:859–862.
- Maresca A, Carta F, Vullo D, Supuran CT. Dithiocarbamates strongly inhibit the ß-class carbonic anhydrases from Mycobacterium tuberculosis. J Enzyme Inhib Med Chem 2011. DOI: 10.3109/14756366.2011.641015
- Segovia N, Crovetto G, Lardelli P, Espigares M. In vitro toxicity of several dithiocarbamates and structure-activity relationships. J Appl Toxicol 2002;22:353–357.
- van Boxtel AL, Pieterse B, Cenijn P, Kamstra JH, Brouwer A, van Wieringen W et al. Dithiocarbamates induce craniofacial abnormalities and downregulate sox9a during zebrafish development. Toxicol Sci 2010;117:209–217.
- Tilton F, La Du JK, Vue M, Alzarban N, Tanguay RL. Dithiocarbamates have a common toxic effect on zebrafish body axis formation. Toxicol Appl Pharmacol 2006;216:55–68.
- van Boxtel AL, Kamstra JH, Fluitsma DM, Legler J. Dithiocarbamates are teratogenic to developing zebrafish through inhibition of lysyl oxidase activity. Toxicol Appl Pharmacol 2010;244:156–161.
- Akamatsu M. Current state and perspectives of 3D-QSAR. Curr Top Med Chem 2002;2:1381–1394.
- Oprea TI, Taboureau O, Bologa CG. Of possible cheminformatics futures. J Comput Aided Mol Des 2012;26:107–112.
- de Paula da Silva CH, Sanches SM, Taft CA. A molecular modeling and QSAR study of suppressors of the growth of Trypanosoma cruzi epimastigotes. J Mol Graph Model 2004;23:89–97.
- Qian Y, Ma GY, Yang Y, Cheng K, Zheng QZ, Mao WJ et al. Synthesis, molecular modeling and biological evaluation of dithiocarbamates as novel antitubulin agents. Bioorg Med Chem 2010;18:4310–4316.
- Clark DE. Prediction of intestinal absorption and blood-brain barrier penetration by computational methods. Comb Chem High Throughput Screen 2001;4:477–496.
- Miri R, Firuzi O, Peymani P, Zamani M, Mehdipour AR, Heydari Z et al. Synthesis, cytotoxicity, and QSAR study of new aza-cyclopenta[b]fluorene-1,9-dione derivatives. Chem Biol Drug Des 2012;79:68–75.
- Cisek K, Kuret J. QSAR studies for prediction of cross-ß sheet aggregate binding affinity and selectivity. Bioorg Med Chem 2012;20:1434–1441.
- Labute P. Derivation and applications of molecular descriptors based on approximate surface area. Methods Mol Biol 2004;275:261–278.
- Mayuresh S, Tukaram J, Ujjawala C, Mahanthesh MC, Manish B. QSAR – A novel tool in drug design. Int J Pharm Appl 2010;1:62–75.
- Praba GO, Velmurugan D. Quantitative structure-activity relationship of some pesticides. Indian J Biochem Biophys 2007;44:470–476.
- Oprea TI. Property distribution of drug-related chemical databases. J Comput Aided Mol Des 2000;14:251–264.
- Available at: www.hyper.com. Accessed on: 3 August 2012.
- Cho SJ, Tropsha A. Cross-validated R2-guided region selection for comparative molecular field analysis: A simple method to achieve consistent results. J Med Chem 1995;38:1060–1066.
- Oprea TI, Waller CL. Theoretical and practical aspects of three-dimensional quantitative structure-activity relationships. J Comp Chem 1997;11:127.
- Rodgers JL, Nicewander WA. Thirteen ways to look at the correlation coefficient. The American Statistician 1988;42:59–66.
- Avram S, Duda-Seiman D, Borcan F, Radu B, Duda-Seiman C, Mihailescu D. Evaluation of antimicrobial activity of new mastoparan derivatives using QSAR and computational mutagenesis. Int J Pept Res Ther 2011;17:7–17.
- Avram S, Berner H, Milac AL, Wolschann P. Quantitative structure-activity relationship studies on membrane receptors inhibition by antipsychotic drugs. Application to schizophrenia treatment. Monatshefte für Chemie – Chemical Monthly 2008;139:407–26.
- Avram S, Mihailescu D, Borcan F, Milac AL. Prediction of improved antimicrobial mastoparan derivatives by 3D-QSARCoMSIA/CoMFA and computational mutagenesis. Monatshefte für Chemie – Chemical Monthly 2012;143:535–43.