Abstract
Objectives: (1) Develop reference ranges of neonatal adiposity using air displacement plethysmography. (2) Use new reference ranges for neonatal adiposity to compare two different methods of evaluating neonatal nutritional status. Methods: Three hundred and twenty-four normal neonates (35–41 weeks post-menstrual age) had body fat (%BF) and total fat mass (FM, g) measured using air displacement plethysmography shortly after delivery. Results were stratified for 92 of these neonates with corresponding fetal biometry using two methods for classifying nutritional status: (1) population-based weight percentiles; and (2) a modified neonatal growth assessment score (m3NGAS51). Results: At the 50th percentile, %BF varied from 7.7% (35 weeks) to 11.8% (41 weeks), while the corresponding 50th percentiles for total FM were 186–436 g. Among the subset of 92 neonates, no significant differences in adiposity were found between small for gestational age (SGA), appropriate for gestational age (AGA), and large for gestational age (LGA) groups using population-based weight standards. Classification of the same neonates using m3NGAS51 showed significant differences in mean %BF between corresponding groups. Conclusions: Population-based weight criteria for neonatal nutritional status can lead to misclassifications on the basis of adiposity. A neonatal growth assessment score, that considers the growth potential of several anatomic parameters, appears to more effectively classify under- and over-nourished newborns.
Introduction
A primary goal of obstetrical care is the timely detection of malnourished fetuses because they have increased the risks for perinatal morbidity, neurodevelopmental disorders, short stature, and metabolic abnormalities in adult life [Citation1]. Pediatricians usually categorize the nutritional state of newborns using birth weight although other techniques such as skin-fold measurements or dual-energy X-ray absorptiometry have also been described for this purpose [Citation2,Citation3]. Obstetricians have alternatively used sonographic measurements of the head, trunk, and limb to estimate fetal weight [Citation4]. However, this indirect calculation is associated with random errors that typically range between 8.1 and 11.8% [Citation5].
Fetal growth is a result of simultaneous changes in body mass, shape, and composition. Adipose tissue development may be particularly sensitive to variations in fetal growth [Citation6]. Human newborns are uniquely distinct from other mammals because they contain relatively large amounts of fat—up to approximately 15% of their body weight at term [Citation7,Citation8]. Adipose tissue is an efficient system, to store energy for the process of subsequent neonatal growth [Citation9]. From an evolutionary perspective, mammalian fat provides an energy store that supports rapid fetal brain growth and protects newborns against the possibility of nutritional deprivation [Citation10]. Fetal fat deposition alone represents over half of the caloric accretion during the early third trimester [Citation6]. By late pregnancy, this process increases to about 90% of the caloric accretion at a time when total caloric requirement is 90–100 kcal/kg/day [Citation6]. A more comprehensive understanding of neonatal body composition may facilitate our identification of malnourished fetuses with regards to their specific nutrient and energy requirements.
A poor correlation has been reported between estimated fetal weight (EFW) and neonatal adiposity [Citation11,Citation12]. Although neonatal fat mass constitutes only 14% of total birth weight, it accounts for 46% of its variance in newborns [Citation13]. Malnourished fetuses can even express abnormal changes in newborn body composition and hormonal concentrations (e.g. IGF-I and IGFBP-3) despite birth weights within the normal range [Citation14,Citation15]. Such observations are particularly relevant to studies that define intrauterine growth retardation (IUGR) on the basis of fetal abdominal circumference alone [Citation16,Citation17].
The current investigation uses noninvasive air displacement plethysmography to develop reference ranges for neonatal adiposity in a large number of preterm and term newborns shortly after delivery. These results are used to compare two methods of neonatal nutritional assessment according to adiposity: (1) population-based percentile ranges based on weight only; and (2) a composite scoring system that, in addition to weight, also considers the growth potential of multiple growth parameters.
Methods
This was a prospective, cross-sectional investigation of newborns delivered to women, who were invited to participate in this study. All women had provided written informed consent to participate in this study that was approved by the Institutional Review Boards at William Beaumont Hospital, Wayne State University, Detroit, MI, USA and the Eunice Kennedy Shriver National Institute of Child Health and Human Development, Bethesda, MD, USA. The protocol enrollment was not consecutive. Inclusion criteria consisted of neonates that were delivered during their third trimester of pregnancy. Pregnancies with poor menstrual dating criteria, multiple gestations, and fetuses with congenital anomalies were excluded from this study.
The normal population sample (n = 324) predominantly consisted of uncomplicated pregnancies with the exception of risk factors that included preeclampsia (n = 15) and smoking (n = 16). Two approaches for nutritional classification were employed: The first one was based on birth weight and post-menstrual age (PMA [Citation18]); the second method used a previously described modified neonatal growth assessment score [Citation19,Citation20]. This comparison included an additional cohort of 25 neonates from mothers with a history of diabetes (Gestational diabetes = 13; Type 1 diabetes = 9; Type 2 diabetes = 3). Maternal age, menstrual age at time of scan, neonatal gender, ethnicity, and presence of obstetrical complications were documented. Ninety-six neonates from the current cohort have been previously reported in two other investigations: the correlation between fetal biometry to percentage body fat (%BF) in 87 term newborns [Citation14] and eight other fetuses that were measured for a soft tissue parameter known as fractional limb volume [Citation21].
Birth age was based on the first day of the last normal menstrual period and confirmed by either first or early second trimester ultrasound scans. A normal last menstrual period was defined as regular cyclic menses without antecedent oral contraceptive use. Age estimates in the first trimester were based on crown–rump length measurements [Citation22]. Age estimates in the second trimester were determined by using biparietal diameter (BPD), head circumference (HC), abdominal circumference (AC), and femoral diaphysis length (FDL) measurements [Citation23–26]. Sonographic age was used to adjust menstrual age if there was more than 1-week discrepancy between menstrual dating and sonographic assessment.
Neonates were examined within approximately 24–48 h of delivery. For purposes of this investigation, the age of neonatal body composition studies was expressed as “post-menstrual age [Citation27]”. Anthropometric assessment included weight and length measurements (Statiometer™, Ellard Instrumentation Ltd., Seattle, WA, USA). Growth assessment was based on measurements of body weight (WT), HC, AC, mid-thigh circumference (ThC), and crown–heel length (CHL) as previously described [Citation28].
Percentage body fat (%BF) and total body fat mass (FM, g) were estimated using direct measurements of volume and body mass based on principles of whole body densitometry (Pea Pod Infant Body Composition System, Life Measurements, Inc., Concord, CA, USA). The standard algorithm compensates for physiological changes in hydration in both term and preterm neonates by adjusting the contribution of total body water to corresponding fat-free density values during the first few days following delivery [Citation29]. The validity, accuracy and reliability of air displacement plethysmography have been previously described for assessment of %BF in adults and neonates [Citation30–32].
Newborns were examined without clothing for air displacement plethysmography studies. Small adjustments for the weight and volume of an umbilical cord clip and name bracelet were compensated by separately subtracting them from the whole body densitometry measurement. Volume measurement artifacts in babies with excessive amounts of hair were minimized from the use of baby oil. After body weight was obtained on an electronic scale, the neonate was placed into a temperature-controlled enclosed chamber that permitted a body volume measurement from well-known pressure/volume relationships. The volume measurement was completed within approximately 2 min. Body density was calculated from these data and applied to the two-compartment model to estimate %BF [Citation8]. Fat mass (g) was calculated by multiplying body mass by the %BF.
Statistical analysis
Standard statistical methods were used to compute means, standard deviations and medians. Mean values were expressed ±1 standard deviation (1 SD). Histograms were constructed to inspect data distributions and Kolmogorov–Smirnov tests were used to evaluate normality. Linear regression models were examined with their Pearson correlation coefficients to investigate the relationship between PMA and neonatal adiposity. Where appropriate, group comparisons were performed with ANOVA and Levene’s test for equality of variances was used. p values less than 0.05 were considered statistically significant. Among the subset of 92 patients, after using ANOVA to test for differences among groups and Levene’s test to verify equality of their variances, Student–Newman-Keuls tests were applied for pairwise comparisons between groups.
Reference ranges were calculated using the methodology outlined by Royston to determine the optimal model and its z-scores [Citation33,Citation34]. Percent BF and absolute FM were the dependent variables; post-menstrual age and newborn weight were the independent variables. The simplest, best model fit was determined and plots of the resulting residuals (difference between observed data and predicted values from the model) were examined to determine whether they conformed to a normal, bell-shaped distribution. Kolmogorov–Smirnov tests for normality were applied. Using the method suggested by Altman [Citation35], the standard deviation of the residuals was examined across the range of values for the independent variable. If there were significant heteroscedasticity, then regression of absolute residuals versus the independent variables was used to adjust the standard deviation.
Based on prior estimates of standard deviation for %BF in newborns of approximately 3% [Citation36–38], we estimated that equal samples sizes of approximately 150 for each gender would be required to detect a difference of 1% BF between males and females on the basis of 80% power and p < 0.05, MedCalc (v. 12.1.4.0, 2011, MedCalc Software bvba, Mariakerke, Belgium). Both linear and multiple regression models were used to evaluate the outcome variables %BF and FM, with inputs of newborn weight, gestational age, gender, race and pregnancies complicated by gestational diabetes. For multiple regression, stepwise methods were used and variables entered the model if p < 0.05 and removed if p > 0.10. The final model included only those variables with p < 0.05. Data analyses were performed with Excel (Microsoft, 2003), MedCalc (v. 12.1.4.0, 2011, MedCalc Software bvba, Mariakerke, Belgium) and S-plus (v. 7.0, 2005, S-Plus Software, Palo Alto, CA, USA).
Body fat estimates were stratified using two different methods of classifying neonatal nutritional status. The first method was based on a population study of 6 690 717 singleton neonates who were delivered between 22 and 44 weeks in the United States [Citation18]. Birth weights and gender were used to define small for gestational age (SGA, birth weight < 10th percentile, AGA, birth weight between 10th and 90th percentile, and LGA, birth weight > 90th percentile. A second classification system was applied to a subset of 92 neonates using both fetal sonographic and neonatal measurements. summarizes key formulas required to evaluate neonatal growth outcome based on the use of each fetus as its own control. Rossavik growth models were used to predict birth characteristics of each newborn on the basis of second trimester fetal biometry (HC, AC, FDL, ThC, FDL). The analysis used an assumption of growth cessation at either birth age [delivery before 38 weeks, PMA] or the actual value of 38 weeks [delivery after 38 weeks [Citation28]]. A Growth Potential Realization Index (GPRI) was then calculated as an outcome variable for neonatal growth status by comparing predicted to actual birth measurements [Citation28].
Figure 1. Equations used for neonatal growth assessment score. WT, weight; HC, head circumference; AC, abdominal circumference; ThC, mid-thigh circumference; CHL, crown–heel length [Citation19,Citation20,Citation39].
![Figure 1. Equations used for neonatal growth assessment score. WT, weight; HC, head circumference; AC, abdominal circumference; ThC, mid-thigh circumference; CHL, crown–heel length [Citation19,Citation20,Citation39].](/cms/asset/95cad06e-2084-4790-8f2b-6b35fb0001aa/ijmf_a_683084_f0001_b.gif)
Deter and colleagues [Citation39] have previously described a composite neonatal growth assessment score (NGAS) that combines weight (WT), head circumference (HC), abdominal circumference (AC), thigh circumference (ThC), and crown–heel length (CHL). The NGAS score originally assumed that each component had equal importance in determining neonatal growth status although weight and soft tissue development (i.e. thigh circumference) are abnormal more often in fetuses with growth abnormalities. A refinement of this NGAS score, the modified neonatal growth assessment score (m3NGAS51), was subsequently developed with weighting factors for each growth parameter using principal components analysis [Citation19,Citation20]. Unlike the conventional approach that relies on population-based standards, the m3NGAS51 evaluates the growth potential of five weighted parameters on the basis of multiple GPRI values. The sum of the products of parameter-specific weighting factors with their fetus-specific GPRI values gives the m3NGAS51 for each neonate. The scores were used to classify neonatal growth outcome into one of three nutritional states: IUGR, normal, and macrosomia [Citation20]. More detailed explanations of the modified neonatal growth assessment score and application of Rossavik growth models are provided as a downloadable “Frequently Asked Questions” Supplementary File (S1).
Results
The primary study cohort consisted of 324 neonates (SGA = 42, AGA = 240, LGA = 42) who were delivered between August 2004 and April 2009. The mean maternal age was 29.3 ± 6.0 years with a median birth age of 38.9 weeks (interquartile range 37.6–39.7 weeks, PMA). Birth weights were normally distributed (mean BW = 3296 ± 560 g, range 1935–4739 g). Ethnic groups included Caucasian (66.0%), African–American (25.6%), Asian (6.2%), other (2.2%). All neonates were studied within 60 h after birth (range = 1–59 h, median age = 22.3 h) with 95% of the pediatric examinations being performed within 48 h after delivery. Average weight loss from delivery time to performance of the neonate body composition study was 116 ± 79 g (−3.5 ± 2.4%, paired t-test = 26.3, p < 0.0001).
Reference ranges for %BF and FM (g) in newborns, delivered between 35–41 weeks PMA, are summarized in . The best model for predicting %BF and FM was with simple, linear regression. While the residuals for each model conformed to a normal distribution, the standard deviation of %BF remained constant as PMA increased, but the standard deviation of FM increased significantly with PMA. Therefore, the standard deviation of %BF was constant (3.8%), but the standard deviation of FM increased with PMA (SD = −399 + 14.3 × PMA). At the 50th percentile, mean %BF ranged between 7.7 and 11.8%, whereas total FM ranged from 186 to 436 g (with respective SD of 102–187 g). A downloadable Supplementary File includes a breakdown of %BF and FM by gender (S2).
Table I. Percentage body fat and fat mass percentiles by post-menstrual age (n = 324).
Similarly, using newborn weight as the independent variable for prediction of %BF and FM, the best models were simple, linear regression. Again, the residuals were normally distributed and the standard deviation of %BF was constant across the range of newborn weights examined, while the standard deviation of FM increased proportionately to newborn weight.
Relationship of post-menstrual age to %BF versus total fat mass
Weak linear correlations were found between post-menstrual age (PMA) and either %BF () or FM () among neonates born during the third trimester of pregnancy. These correlations were not significantly different by Fisher’s z-test. Considerable overlap occurred between the SGA, AGA and LGA subgroups. Regression analyses indicate that the AGA and SGA subsets were statistically indistinguishable, although LGA neonates had a slightly greater increase of %BF and FM with increasing PMA.
Figure 2. Body fat versus post-menstrual age expressed as percentage (a) or absolute amount (b) of body fat. Regression equation for percentage body fat = −15.9 + 0.675 × weeks, r2 = 0.075, p < 0.001; for fat mass = −1270 + 41.6 × weeks, r2 = 0.152, p < 0.001. Regression lines = predicted mean value with 5th and 95th percentiles. While the standard deviation in percentage body fat did not significantly change as post-menstrual age increased, the standard deviation in fat mass increased with increasing post-menstrual age. SGA, small for gestational age; AGA, appropriate for gestational age; LGA, large for gestational age.
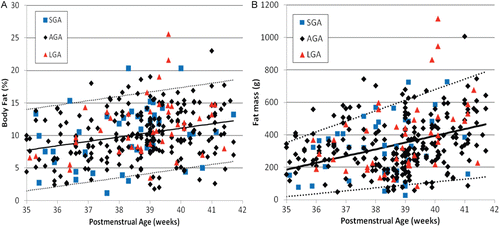
Relationship of neonatal weight to %BF versus total fat mass
Neonatal body composition studies were performed at a median of 22 h after delivery. Hence, the analyses of FM and %BF were based on body weight at the time of the body composition study, rather than birth weight. Both %BF (r2 = 0.370, p < 0.001) () and FM (r2 = 0.623, p < 0.0001) () progressively increased with increasing total weight. Once again, there was considerable overlap between SGA, AGA and LGA groups. The correlation was significantly greater when these results were expressed as FM (Fisher’s z-test, p < 0.0001), as compared to %BF. Similar to the relationship between PMA and adiposity, regression analyses indicate that the AGA and SGA subsets were statistically indistinguishable. Large for gestational age neonates had a slightly greater increase of %BF and FM with increasing body weight.
Figure 3. Body fat versus neonatal weight at the time of air displacement plethysmography expressed as percentage (a) or absolute amount (b) of body fat. Regression equation for percentage body fat = −4.01 + 0.00447 × weight, r2 = 0.370, p < 0.001; for fat mass = −461 + 0.251 × weight, r2 = 0.623, p < 0.0001. Regression lines = predicted mean value with 5th and 95th percentiles. While the standard deviation in percentage body fat did not significantly change as newborn weight increased, the standard deviation in fat mass increased with larger newborn weight. SGA, small for gestational age; AGA, appropriate for gestational age; LGA, large for gestational age.
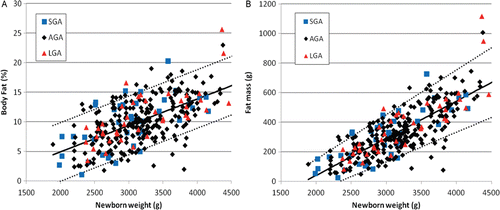
Gender and ethnic differences in %BF versus total fat mass
There were essentially equal proportions of male (n = 160, 49.4%) and female neonates (n = 164, 50.6%) in the study sample. Females had greater %BF (10.8 ± 4.2%) as compared to male neonates (9.5 ± 3.6%) (t-test = 2.98, p = 0.003) during the third trimester (). No significant sex differences were found for FM (351 ± 183 [females] vs. 323 ± 161 g [males]), (t-test = 1.47, p = 0.14) (). However, a significant effect of neonatal sex was found for both %BF and FM after controlling for body weight and PMA.
Figure 4. Effect of gender on percentage body fat (%BF) and fat mass. Females had slightly greater mean %BF (10.8 ± 4.2%) when compared to male neonates (9.5 ± 3.6%) (t-test = 2.98, p = 0.003) (a). These differences disappeared when mean fat mass was compared between females (351 ± 183 g) and males (323 ± 161 g) (t-test = 1.47, p = 0.14) (b). On average, males had 2.3 ± 0.34% less %BF (t = 6.78, p < 0.0001) and 78 ± 11 g less FM than females (t = 6.94, p < 0.0001). Both graphs show mean ± 1 SD for each group.
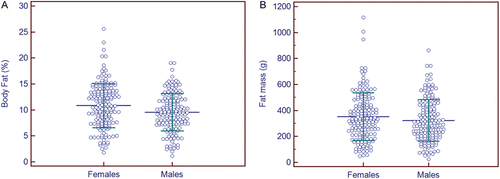
No significant differences in %BF or FM were initially identified between African–American versus Caucasian neonates (other ethnic groups had insufficient sample sizes). Mean FM (g) was 343 ± 182 (Caucasian, n = 214) versus 319 ± 148 (African–American, n = 83), t-test = 1.09, p = 0.28); mean %BF was 10.1 ± 4.2 (Caucasian) versus 10.4 ± 3.6% (African–American), t-test = 0.55, p = 0.58. However, significant ethnic differences in %BF and FM were identified after adjusting for neonatal weight, PMA and gender. On average, African–American neonates had 1.9 ± 0.4% greater %BF (t = 4.82, p < 0.0001) and 55 ± 13 g greater FM (t = 4.26, p < 0.0001) than did Caucasian neonates. These ethnic differences are further considered in a separate analysis for a subset of the same study cohort (Lampl M, et al. Ethnic differences in the accumulation of fat and lean mass in late gestation. Am J Hum Biol, in press).
Comparison of newborns from diabetic pregnancies with normal controls
In addition to the 324 neonates of non-diabetic mothers that were used to develop reference ranges, 25 additional neonates (14 females, 11 males) were studied from pregnancies complicated by diabetes. The mean birth weight of this diabetic group (3619 ± 620 g) was greater than neonates from non-diabetic pregnancies (3296 ± 560 g) (t = 2.85, p < 0.01). Both total FM (552 ± 210 vs. 337 ± 173 g, t = 5.88, p < 0.001) and %BF (15.4 ± 4.2 vs. 10.2 ± 4.0%, t = 6.21, p < 0.001) were significantly greater than the normal controls. Mean gestational ages were not significantly different: 38.6 ± 1.6 (normal) versus 38.1 ± 1.3 weeks (diabetes), t = 1.50, p = 0.13). Neonates of diabetic pregnancies had significantly greater %BF and FM after adjusting for newborn weight, gestational age, gender and ethnicity. On average, neonates of diabetic pregnancies had 2.3 ± 0.7% more %BF (t = 3.29, p < 0.001) and 84 ± 24 g greater FM (t = 3.54, p < 0.001) than infants of non-diabetic pregnancies. An important study limitation was the unavailability of precise diagnostic criteria for classifying diabetic pregnancies. A larger and more detailed cohort will be necessary to analyze which conditions of maternal glycemic control are associated with altered adiposity.
Classification of newborn adiposity using population-based percentiles versus modified neonatal growth assessment scores
Neonatal growth outcomes were initially classified using conventional criteria based on cross-sectional percentile ranges that considered birth weight, gender, and PMA [Citation18]: SGA (n = 45, %BF = 10.3 ± 4.8%), AGA (n = 259, %BF = 10.5 ± 4.1%), and LGA (n = 45, %BF = 11.4 ± 4.4 %) (mean ± SD) There was no significant difference in %BF by ANOVA between or among these groups (F ratio = 0.37, p = 0.69). No significant differences in FM were found among these growth outcome groups: (SGA = 332 ± 198 g, AGA = 349 ± 174 g, LGA = 397 ± 221 g, (mean ± SD) (ANOVA, F ratio = 1.68, p = 0.19).
A subset of 92 neonates with corresponding prenatal ultrasound measurements permitted a comparison employing the m3NGAS51, including one AGA neonate from a mother with gestational diabetes. These neonates were classified using population-based stratification into small (SGA, n = 11), appropriate (AGA, n = 74) or large for gestational age (LGA, n = 7) nutritional categories. A comparison of %BF identified no significant differences by nutritional category ( and ). Subsequent classification of the same neonates by m3NGAS51, however, identified significant differences in %BF among the nutritional assessment groups: IUGR (n = 7, %BF = 5.5 ± 2.7%), normal (n = 81, %BF = 9.7 ± 3.7%), and macrosomia (n = 4, %BF = 14.2 ± 1.3%) (ANOVA, F ratio = 7.85, p = 0.001) ( [Citation20]). This multivariate score led to a more robust classification of neonates into these nutritional categories, particularly when the results were expressed as total FM ().
Figure 5. Nutritional classification of percentage body fat and fat mass using population-based weight standards. No significant differences were found between groups by either percentage body fat (a), (ANOVA, F ratio = 0.37, p = 0.69), or fat mass (b), (ANOVA, F ratio = 0.14, p = 0.87); similarly, all pairwise comparisons were not significant. Mean values ± 1 SD. SGA, small for gestational age; AGA, appropriate for gestational age; LGA, large for gestational age.
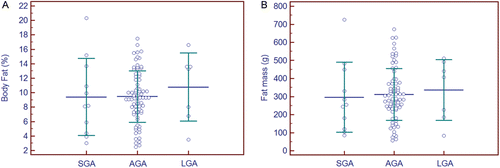
Figure 6. Nutritional classification of percentage body fat and fat mass using a neonatal growth assessment score (m3NGAS51). The same subset of 92 neonates from is stratified into different nutritional groups: intrauterine growth retardation (IUGR, n = 7), normal (n = 81) or macrosomia (n = 4). Significant differences were observed for both percentage body fat (a), F ratio = 7.85, p < 0.005, and fat mass (b) (ANOVA, F ratio = 11.9), p < 0.001. Mean value ± 1 SD are shown; all pairwise comparisons were significant at p < 0.05. SGA, small for gestational age; AGA, appropriate for gestational age; LGA, large for gestational age.
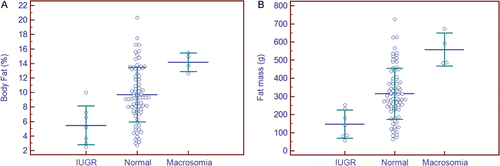
summarizes the poor correlation between the population-based assessment and the modified neonatal growth assessment score for classification of nutritional status. Overall agreement between the two classification methods was poor (κ = −0.011, p > 0.05). Categorical distribution of the data was also not different from chance (χ2 = 1.64, p = 0.80).
Table II. Neonatal nutritional classification: comparison between population standard versus modified neonatal growth assessment score (m3NGAS51)
Discussion
Increased fetal adiposity has been proposed as a more sensitive and specific outcome measure at birth among neonates of pregnant women with mild glucose intolerance [Citation40]. This report provides reference ranges of whole body adiposity among neonates delivered of non-diabetic mothers from the largest series of neonatal body fat measurements to date, assessed within hours of delivery. Both %BF and FM increased linearly with PMA and neonatal weight. Although the standard deviation for %BF was constant across the range of PMA and newborn weights, the standard deviation for FM increased with increasing PMA or newborn weight. This dependence was not surprising, since FM itself is defined as the product of %BF and newborn weight. Based on our sample size of 324, the predicted error on the reference range of 5th–95th percentiles was 8.5% of the range [Citation33] and was 12.0% when references were calculated by gender. A sample size of approximately 1000 would be required to decrease the error to only 5% of the 5th–95th percentile reference range.
Relatively few studies have evaluated neonatal whole body adiposity shortly after birth, especially prior to 38 weeks gestation [Citation14,Citation15,Citation17]. Smaller cohorts of newborns have been previously described using air displacement plethysmography [Citation36–38,Citation41] and dual-energy X-ray absorptiometry [Citation17,Citation42–44] (). Others have described patterns of regional adiposity with neonatal skin-fold measurements [Citation16,Citation45–52]. A recent multicenter longitudinal study of breast-fed neonates used air displacement plethysmography to develop gender-specific %BF and fat-free mass curves in 160 neonates up to 6 months after delivery; however, only 35 neonates were studied just after birth [Citation53].
Table III. Newborn infant body composition-total body fat stores
Previous reports have noted that female neonates have a slightly greater proportion of %BF than their male counterparts on the basis of dual-energy X-ray absorptiometry [Citation42–44], skin-fold thickness [Citation49,Citation52], and multi-compartment body composition models [Citation8,Citation53]. Small but significant sex differences in %BF disappeared when our results were expressed as FM (g). This reflects the greater body mass of male neonates when compared to their female counterparts. Hence, while female neonates have a higher %BF, their total body mass is less than males, so the product (%BF × body mass) remains similar for both sexes. A much larger sample size would be required to confirm these observations: At least 1500 neonates would need to be studied to demonstrate a difference of only 25 g of FM (≥80% power, p < 0.05 level of significance).
Over four decades ago, the novel introduction of using birth weight for classifying neonatal nutritional status into clinical practice was an important advance in the management of newborns at risk for adverse outcomes [Citation54]. Our findings confirm that %BF and FM are significantly correlated with neonatal body weight [Citation3,Citation8,50,53]. However, a comparison of adiposity according to the traditional population-based classification of nutritional status identifies that a substantial number of misclassifications do occur. A pediatric investigation that used dual-energy X-ray absorptiometry study also found that birth weight categorization does not precisely reflect %BF in preterm and term newborns [Citation43]. More frequent misclassifications would be expected to occur in fetuses since the variability of calculated EFW is much greater than actual birth weight.
Accurate assessment of newborn adiposity is important because alterations in early nutritional programming of body composition and subsequent growth patterns may increase the risk of metabolic syndrome during adult life [Citation55,Citation56]. More sophisticated methods for the early detection of malnourished fetuses could more precisely define the relationships between maternal weight gain or body mass index to neonatal body composition as potential factors for future adult disease risk [Citation57–59]. Differential growth of fetal tissues raise the possibility that the quantification of fat provide a more sensitive and specific marker of abnormal growth than lean body mass [Citation60–62]. Although no single prenatal measurement is currently optimal, it is likely that a combination of parameters will more precisely reflect fetal adiposity and generalized nutritional status. Fractional limb volume is one example of a reproducible fetal soft tissue parameter that could be used for this purpose—as a direct measurement [Citation21,Citation63,64], combined with Doppler flow studies, or as part of a more precise fetal weight estimation procedure [Citation65–69].
In summary, population-based weight criteria can lead to misclassifications of neonatal nutritional status on the basis of adiposity. A neonatal growth assessment score, that considers the growth potential of multiple parameters, more effectively classifies under- and over-nourished newborns. The latter approach provides a more comprehensive assessment of neonatal growth and corrects for confounding variables such as differences in age and growth potential. A potential limitation, however, is the need for the second trimester fetal biometry, from a time of assumed normal growth that is required to estimate growth potential. Nonetheless, a more comprehensive assessment of neonatal nutritional status should improve our understanding of fundamental mechanisms that affect intrauterine accumulation of energy stores and their potential impact on postnatal outcome. The clinical significance of our findings will require further examination in larger prospective studies using different population samples.
Supplementary Material
Download PDF (75.3 KB)Acknowledgements
The Authors wish to acknowledge the technical assistance of Melissa Powell, RDMS; Beverley McNie, BS, CCRP; Elizabeth Kring, RNC, BSN, CCRC; and Jennifer DeRidder, RN, BSN.
Declaration of Interest: This research was supported (in part) by the Perinatology Research Branch, Division of Intramural Research, Eunice Kennedy Shriver National Institute of Child Health and Human Development, NIH, DHHS. R. Romero contributed to this work as part of his official duties as an employee of the United States Federal Government. None of the other authors have disclosed a conflict of interest.
References
- Clayton PE, Cianfarani S, Czernichow P, Johannsson G, Rapaport R, Rogol A. Management of the child born small for gestational age through to adulthood: a consensus statement of the International Societies of Pediatric Endocrinology and the Growth Hormone Research Society. J Clin Endocrinol Metab 2007;92:804–810.
- Beattie RB, Johnson P. Practical assessment of neonatal nutrition status beyond birthweight: an imperative for the 1990s. Br J Obstet Gynaecol 1994;101:842–846.
- Hammami M, Koo WW, Hockman EM. Body composition of neonates from fan beam dual energy X-ray absorptiometry measurement. JPEN J Parenter Enteral Nutr 2003;27:423–426.
- Hadlock FP, Harrist RB, Sharman RS, Deter RL, Park SK. Estimation of fetal weight with the use of head, body, and femur measurements – a prospective study. Am J Obstet Gynecol 1985;151:333–337.
- Melamed N, Yogev Y, Meizner I, Mashiach R, Bardin R, Ben-Haroush A. Sonographic fetal weight estimation: which model should be used? J Ultrasound Med 2009;28:617–629.
- Sparks JW, Girard JR, Battaglia FC. An estimate of the caloric requirements of the human fetus. Biol Neonate 1980;38:113–119.
- Spray CM, Widdowson EM. The effect of growth and development on the composition of mammals. Br J Nutr 1950;4:332–353.
- Fomon SJ, Nelson SE. Body composition of the male and female reference infants. Annu Rev Nutr 2002;22:1–17.
- Zafon C. Oscillations in total body fat content through life: an evolutionary perspective. Obes Rev 2007;8:525–530.
- Kuzawa CW. Adipose tissue in human infancy and childhood: an evolutionary perspective. Am J Phys Anthropol 1998;Suppl 27:177–209.
- Lee W, Balasubramaniam M, Deter RL, Hassan SS, Gotsch F, Kusanovic JP, Gonçalves LF, Romero R. Fetal growth parameters and birth weight: their relationship to neonatal body composition. Ultrasound Obstet Gynecol 2009;33:441–446.
- Moyer-Mileur LJ, Slater H, Thomson JA, Mihalopoulos N, Byrne J, Varner MW. Newborn adiposity measured by plethysmography is not predicted by late gestation two-dimensional ultrasound measures of fetal growth. J Nutr 2009;139:1772–1778.
- Catalano PM, Tyzbir ED, Allen SR, McBean JH, McAuliffe TL. Evaluation of fetal growth by estimation of neonatal body composition. Obstet Gynecol 1992;79:46–50.
- Hemachandra AH, Klebanoff MA. Use of serial ultrasound to identify periods of fetal growth restriction in relation to neonatal anthropometry. Am J Hum Biol 2006;18:791–797.
- Verkauskiene R, Beltrand J, Claris O, Chevenne D, Deghmoun S, Dorgeret S, Alison M et al. Impact of fetal growth restriction on body composition and hormonal status at birth in infants of small and appropriate weight for gestational age. Eur J Endocrinol 2007;157:605–612.
- Hecher K, Bilardo CM, Stigter RH, Ville Y, Hackelöer BJ, Kok HJ, Senat MV, Visser GH. Monitoring of fetuses with intrauterine growth restriction: a longitudinal study. Ultrasound Obstet Gynecol 2001;18:564–570.
- Bilardo CM, Wolf H, Stigter RH, Ville Y, Baez E, Visser GH, Hecher K. Relationship between monitoring parameters and perinatal outcome in severe, early intrauterine growth restriction. Ultrasound Obstet Gynecol 2004;23:119–125.
- Oken E, Kleinman KP, Rich-Edwards J, Gillman MW. A nearly continuous measure of birth weight for gestational age using a United States national reference. BMC Pediatr 2003;3:6.
- Deter RL, Nazar R, Milner LL. Modified neonatal growth assessment score: a multivariate approach to the detection of intrauterine growth retardation in the neonate. Ultrasound Obstet Gynecol 1995;6:400–410.
- Deter RL, Spence LR. Identification of Macrosomic, normal and intrauterine growth retarded neonates using the modified Neonatal Growth Assessment Score. Fetal Diagn Ther 2004;19:58–67.
- Lee W, Balasubramaniam M, Deter RL, Hassan SS, Gotsch F, Kusanovic JP, Gonçalves LF, Romero R. Fractional limb volume–a soft tissue parameter of fetal body composition: validation, technical considerations and normal ranges during pregnancy. Ultrasound Obstet Gynecol 2009;33:427–440.
- Hadlock FP, Shah YP, Kanon DJ, Lindsey JV. Fetal crown–rump length: reevaluation of relation to menstrual age (5-18 weeks) with high-resolution real-time US. Radiology 1992;182:501–505.
- Hadlock FP, Deter RL, Harrist RB, Park SK. Fetal biparietal diameter: a critical re-evaluation of the relation to menstrual age by means of real-time ultrasound. J Ultrasound Med 1982;1:97–104.
- Hadlock FP, Deter RL, Harrist RB, Park SK. Fetal head circumference: relation to menstrual age. AJR Am J Roentgenol 1982;138:649–653.
- Hadlock FP, Deter RL, Harrist RB, Park SK. Fetal abdominal circumference as a predictor of menstrual age. AJR Am J Roentgenol 1982;139:367–370.
- Warda AH, Deter RL, Rossavik IK, Carpenter RJ, Hadlock FP. Fetal femur length: a critical reevaluation of the relationship to menstrual age. Obstet Gynecol 1985;66:69–75.
- Engle WA; American Academy of Pediatrics Committee on Fetus and Newborn. Age terminology during the perinatal period. Pediatrics 2004;114:1362–1364.
- Deter RL, Hill RM, Tennyson LM. Predicting the birth characteristics of normal fetuses 14 weeks before delivery. J Clin Ultrasound 1989;17:89–93.
- Roggero P, Giannì ML, Orsi A, Piemontese P, Amato O, Moioli C, Mosca F. Neonatal period: body composition changes in breast-fed full-term newborns. Neonatology 2010;97:139–143.
- Fields DA, Goran MI, McCrory MA. Body-composition assessment via air-displacement plethysmography in adults and children: a review. Am J Clin Nutr 2002;75:453–467.
- Ma G, Yao M, Liu Y, Lin A, Zou H, Urlando A, Wong WW et al. Validation of a new pediatric air-displacement plethysmograph for assessing body composition in infants. Am J Clin Nutr 2004;79:653–660.
- Ellis KJ, Yao M, Shypailo RJ, Urlando A, Wong WW, Heird WC. Body-composition assessment in infancy: air-displacement plethysmography compared with a reference 4-compartment model. Am J Clin Nutr 2007;85:90–95.
- Royston P. Constructing time-specific reference ranges. Stat Med 1991;10:675–690.
- Royston P, Wright EM. Goodness-of-fit statistics for age-specific reference intervals. Stat Med 2000;19:2943–2962.
- Altman DG. Construction of age-related reference centiles using absolute residuals. Stat Med 1993;12:917–924.
- Fields DA, Krishnan S, Wisniewski AB. Sex differences in body composition early in life. Gend Med 2009;6:369–375.
- Eriksson B, Löf M, Forsum E. Body composition in full-term healthy infants measured with air displacement plethysmography at 1 and 12 weeks of age. Acta Paediatr 2010;99:563–568.
- Roggero P, Giannì ML, Orsi A, Piemontese P, Amato O, Liotto N, Morlacchi L et al. Quality of growth in exclusively breast-fed infants in the first six months of life: an Italian study. Pediatr Res 2010;68:542–544.
- Deter RL, Harrist RB, Hill RM. Neonatal growth assessment score: a new approach to the detection of intrauterine growth retardation in the newborn. Am J Obstet Gynecol 1990;162:1030–1036.
- Catalano PM, Thomas A, Huston-Presley L, Amini SB. Increased fetal adiposity: a very sensitive marker of abnormal in utero development. Am J Obstet Gynecol 2003;189:1698–1704.
- Carberry AE, Colditz PB, Lingwood BE. Body composition from birth to 4.5 months in infants born to non-obese women. Pediatr Res 2010;68:84–88.
- Koo WW, Walters JC, Hockman EM. Body composition in neonates: relationship between measured and derived anthropometry with dual-energy X-ray absorptiometry measurements. Pediatr Res 2004;56:694–700.
- Schmelzle HR, Quang DN, Fusch G, Fusch C. Birth weight categorization according to gestational age does not reflect percentage body fat in term and preterm newborns. Eur J Pediatr 2007;166:161–167.
- Rigo J, Nyamugabo K, Picaud JC, Gerard P, Pieltain C, De Curtis M. Reference values of body composition obtained by dual energy X-ray absorptiometry in preterm and term neonates. J Pediatr Gastroenterol Nutr 1998;27:184–190.
- Yau KI, Chang MH. Weight to length ratio–a good parameter for determining nutritional status in preterm and full-term newborns. Acta Paediatr 1993;82:427–429.
- Catalano PM, Thomas AJ, Avallone DA, Amini SB. Anthropometric estimation of neonatal body composition. Am J Obstet Gynecol 1995;173:1176–1181.
- Guihard-Costa AM, Grangé G, Larroche JC, Papiernik E. Sexual differences in anthropometric measurements in French newborns. Biol Neonate 1997;72:156–164.
- Rolland-Cachera MF, Brambilla P, Manzoni P, Akrout M, Sironi S, Del Maschio A, Chiumello G. Body composition assessed on the basis of arm circumference and triceps skinfold thickness: a new index validated in children by magnetic resonance imaging. Am J Clin Nutr 1997;65:1709–1713.
- Rodríguez G, Samper MP, Ventura P, Moreno LA, Olivares JL, Pérez-González JM. Gender differences in newborn subcutaneous fat distribution. Eur J Pediatr 2004;163:457–461.
- Koo WW, Walters JC, Hockman EM. Body composition in human infants at birth and postnatally. J Nutr 2000;130:2188–2194.
- Singh KA, Huston-Presley LP, Mencin P, Thomas A, Amini SB, Catalano PM. Birth weight and body composition of neonates born to Caucasian compared with African–American mothers. Obstet Gynecol 2010;115:998–1002.
- Luque V, Mendez G, Capdevila F, Closa R, Ferre N, Reina Garcia M, Escribano J. Subcutaneous fat stores related to weight in full-term neonates. Ann Hum Biol 2009;36:88–97.
- Fields DA, Gilchrist JM, Catalano PM, Giannì ML, Roggero PM, Mosca F. Longitudinal body composition data in exclusively breast-fed infants: a multicenter study. Obesity (Silver Spring) 2011;19:1887–1891.
- Battaglia FC, Lubchenco LO. A practical classification of newborn infants by weight and gestational age. J Pediatr 1967;71:159–163.
- Sayer AA, Cooper C. Fetal programming of body composition and musculoskeletal development. Early Hum Dev 2005;81:735–744.
- Wells JC, Chomtho S, Fewtrell MS. Programming of body composition by early growth and nutrition. Proc Nutr Soc 2007;66:423–434.
- Hull HR, Dinger MK, Knehans AW, Thompson DM, Fields DA. Impact of maternal body mass index on neonate birthweight and body composition. Am J Obstet Gynecol 2008;198:416.e1–416.e6.
- Dietz PM, Callaghan WM, Smith R, Sharma AJ. Low pregnancy weight gain and small for gestational age: a comparison of the association using 3 different measures of small for gestational age. Am J Obstet Gynecol 2009;201:53.e1–53.e7.
- Dietz PM, Callaghan WM, Sharma AJ. High pregnancy weight gain and risk of excessive fetal growth. Am J Obstet Gynecol 2009;201:51.e1–51.e6.
- Valensise H, Larciprete G, Vasapollo B, Novelli G, Altomare F, Andreoli A, De Lorenzo A, Arduini D. Nifedipine-induced changes in body composition in hypertensive patients at term. Eur J Obstet Gynecol Reprod Biol 2003;106:139–143.
- Galan HL, Rigano S, Radaelli T, Cetin I, Bozzo M, Chyu J, Hobbins JC, Ferrazzi E. Reduction of subcutaneous mass, but not lean mass, in normal fetuses in Denver, Colorado. Am J Obstet Gynecol 2001;185:839–844.
- Padoan A, Rigano S, Ferrazzi E, Beaty BL, Battaglia FC, Galan HL. Differences in fat and lean mass proportions in normal and growth-restricted fetuses. Am J Obstet Gynecol 2004;191:1459–1464.
- Lee W, Deter RL, McNie B, Gonçalves LF, Espinoza J, Chaiworapongsa T, Romero R. Individualized growth assessment of fetal soft tissue using fractional thigh volume. Ultrasound Obstet Gynecol 2004;24:766–774.
- Lee W, Deter RL, McNie B, Goncalves LF, Espinoza J, Chaiworapongsa T, Balasubramaniam M, Romero R. The fetal arm: individualized growth assessment in normal pregnancies. J Ultrasound Med 2005;24:817–828.
- Lee W, Deter RL, Ebersole JD, Huang R, Blanckaert K, Romero R. Birth weight prediction by three-dimensional ultrasonography: fractional limb volume. J Ultrasound Med 2001;20:1283–1292.
- Lee W, Balasubramaniam M, Deter RL, Yeo L, Hassan SS, Gotsch F, Kusanovic JP et al. New fetal weight estimation models using fractional limb volume. Ultrasound Obstet Gynecol 2009;34:556–565.
- Khoury FR, Stetzer B, Myers SA, Mercer B. Comparison of estimated fetal weights using volume and 2-dimensional sonography and their relationship to neonatal markers of fat. J Ultrasound Med 2009;28:309–315.
- Srisantiroj N, Chanprapaph P, Komoltri C. Fractional thigh volume by three-dimensional ultrasonography for birth weight prediction. J Med Assoc Thai 2009;92:1580–1585.
- Yang F, Leung KY, Hou YW, Yuan Y, Tang MH. Birth-weight prediction using three-dimensional sonographic fractional thigh volume at term in a Chinese population. Ultrasound Obstet Gynecol 2011;38:425–433.