Abstract
Background:
The aims of this study were to quantify and contrast patient preferences between second-line advanced renal cell carcinoma (RCC) medication profiles and their associated benefits and toxicities, and to help frame the doctor–patient discussion about selecting appropriate RCC therapies.
Research design and methods:
Adult residents of the US with a diagnosis of RCC completed a Web-enabled choice-format conjoint survey consisting of a series of 10 treatment-choice questions, each of which included a pair of hypothetical RCC medication profiles. Each profile was described by various medication attributes (features or outcomes) with varying levels. The attributes included efficacy (progression-free survival [PFS]), tolerability (fatigue, stomach problems, mucositis or stomatitis, hand–foot syndrome [HFS]), serious but rare adverse events (pneumonitis, hepatic impairment), and mode of administration. Treatment-choice questions were based on an experimental design with known statistical properties. Random-parameters logit regression was used to estimate relative preference weights for each attribute level. Benefit equivalent measures (additional months of PFS in exchange for toxicities) were also calculated.
Results:
Of the 272 patients who completed the survey, the majority were female (53%), white (92%), and had at least a college degree (66%). The mean age was 57 years (standard deviation: 10 years). Over the range of attributes and attribute levels included in the survey, PFS was the most important attribute, followed by fatigue, stomach problems, hepatic impairment, mucositis or stomatitis, HFS, pneumonitis, and mode of administration. To reduce severe fatigue to mild-to-moderate fatigue, patients on average would be willing to forego 4.4 months of PFS. To reduce hepatic impairment risk from 0.5% to 0.0%, patients on average would be willing to forego 1.0 month of PFS. The main study limitation was that patients answered hypothetical treatment-choice questions.
Conclusions:
This study provides information to physicians about patient priorities when reviewing and selecting RCC therapies with patients.
Introduction
The past 6 years have seen an unprecedented array of new therapies for advanced renal cell carcinoma (RCC). These new therapies were developed as a result of the findings that the vascular endothelial growth factor (VEGF) and mammalian target of rapamycin (mTOR) pathways are critical molecular mechanisms that drive RCCCitation1. Small molecules have been developed to specifically target these pathways and are known as VEGF tyrosine kinase inhibitors (TKIs) or mTOR inhibitors. A number of Phase III clinical trials have shown significant benefit in progression-free survival (PFS) when these drugs are used in patients with advanced RCCCitation2–6.
In addition to their dominant effects on the VEGF family of receptors, VEGF TKIs inhibit a wide spectrum of cellular kinasesCitation7. These so-called off-target effects likely account for the differences in their biologic activity and toxicities. The mTOR pathway serves as an intracellular regulator of cellular metabolism and energy homeostasis, and its inhibition affects cell translation, transcription, and protein synthesis across a large cross-section of cellular pathwaysCitation8. Although the toxicity profiles of these new therapies, as documented in clinical trials, are improved compared with cytotoxic chemotherapy regimen profiles, the toxicities nevertheless are common, pernicious, chronic, and affect multiple organ systems. A very small minority of these toxicities, such as hepatic impairment and pneumonitis, can be serious and life threatening.
Despite the beneficial impact of VEGF TKIs and mTOR inhibitors on PFS in advanced RCC patients, long-term durable complete responses are rare, necessitating the therapies’ continuous use, accompanied by their toxicities, throughout the entire course of illnessCitation7,Citation8. In essence, patients and their physicians are forced to choose between achieving the benefits of treatment and avoiding toxicities.
Multiple consensus treatment algorithms can help guide clinicians to select the most appropriate therapy in RCCCitation9. However, one of the knowledge gaps—and an area of active debate—concerns the best sequence of RCC therapies when using one therapy after the other. Therefore, there is currently an element of ‘art’ in the medical decision-making process. However, the published literature remains relatively silent on the elements of the new therapies’ toxicity profiles that could be important to patients when selecting sequential therapy. In other words, what toxicities are important to patients, and how would they trade off toxicity and benefit?
Mohamed et al.Citation10 estimated patient preferences for benefits, toxicities, and risks of RCC treatments by examining patients’ willingness to trade-off between PFS and toxicities and between PFS and serious adverse-event risks commonly associated with angiogenesis inhibitor agents (sunitinib, sorafenib, bevacizumab, and pazopanib) and other first-line RCC treatments. That study’s results indicated that, while increasing PFS was the most important attribute to patients, reducing severe fatigue and diarrhea also were very important. In contrast, the current study extends the existing knowledge by examining patient preferences for attributes associated with angiogenesis and mTOR inhibitor agents when used in second and subsequent treatment lines for advanced RCC. In addition, this study also involves a more heterogeneous cross-section of toxicities in a background of chronicity, more closely modeling ‘real-world’ RCC therapy.
The premises of this study were that toxicities were not equal for patients and that patients had distinct preferences. Our hypothesis was that patients considered PFS as the most desirable attribute but could be willing to trade-off PFS against a hierarchy of specific toxicities that affected quality-of-life. The hierarchy consisted of determining the threshold PFS that would trigger a given treatment switch. The goal of this study was to provide treating oncologists, drug developers, and cancer policy-makers with guidance as to patient preferences when considering drug sequencing in RCC treatment.
Patients and methods
Study sample
RCC patients were recruited from the Kidney Cancer Association (KCA) member panel that consisted of not only patients but also caregivers in various countries, including Australia, Canada, the UK, and the US. The KCA member panel was the best available option at the time of the study due to the low prevalence of RCC. Potential study patients received an e-mail invitation asking them to participate in an online survey. All participating patients were required to be a US resident of at least 18 years of age with a self-reported physician diagnosis of RCC. The 25-min online survey was administered in June 2011. As an incentive for patients to complete the survey, a $20 donation to support future research was made to the KCA for each completed survey.
Ethical consideration for this study was reviewed by RTI International’s Office of Research Protection and Ethics and approved by RTI International’s institutional review board. Patients were informed of the potential benefits and risks of participation and were required to provide informed consent prior to participating in the survey.
Survey instrument
Choice-format conjoint analysis is a systematic method of eliciting trade-offs to quantify the relative importance patients assign to various treatment attributes or outcomesCitation11,Citation12. Such an analysis is based on the premise that treatments are composed of a set of attributes and that the attractiveness of a particular treatment to an individual patient is a function of these attributesCitation13,Citation14.
After consultation with practicing oncologists who treat RCC patients on a daily basis (including the lead author), we identified the following eight attributes () that are common and likely of concern to RCC patients: PFS, fatigue, stomach problems, mucositis or stomatitis, hand-foot syndrome, chance of pneumonitis, chance of hepatic impairment, and mode of administration. The attributes were described in patient-friendly terms using clinical definitionsCitation15.
Table 1. Attributes and levels with descriptions for the choice questions.
Fifteen 1-h semi-structured interviews were conducted with RCC patients in order to test the clarity of the survey instrument and the appropriateness of the descriptive information, to confirm that the eight attributes included in the survey were of concern to patients, and to assess patients’ willingness to accept trade-offs among attributes in evaluating hypothetical treatments. Three of the 15 interviews were conducted in Philadelphia, PA. The three respondents were recruited by a focus group facility and completed the paper-and-pencil survey. The remaining 12 interviews were conducted over the telephone. For telephone interviews, respondents who were recruited from the KCA member panel completed the survey online. During the interviews, patients were asked to ‘think aloud’ as they completed the draft survey instrument. Patients also were asked a series of debriefing questions to determine whether they understood the definitions and instructions, accepted the hypothetical context of the survey, and successfully completed the choice questions in the survey instrument as instructedCitation16. As a result of these semi-structured interviews, text changes were made to the survey to improve readability and comprehension.
In each choice question, patients were asked to indicate which of two hypothetical treatments they would choose. Each hypothetical treatment was defined by various levels of the eight attributes (). In addition, the survey elicited a number of items about patients’ experiences with RCC (e.g., time since diagnosis, kidney surgery status, metastatic disease status, and current treatment status)Citation10, as well as standard demographic information (e.g., age, gender, race, marital status, education level).
Figure 1. Example of a choice question. Patients could click on the label to see the description for any medication feature. Prior to answering the choice questions, patients were asked to assume that (1) their RCC was metastatic, (2) they were starting a new medication, (3) this was the last medication they could take, and (4) all of their medical bills, including the cost of medications, were covered by health insurance. RCC = renal cell carcinoma.
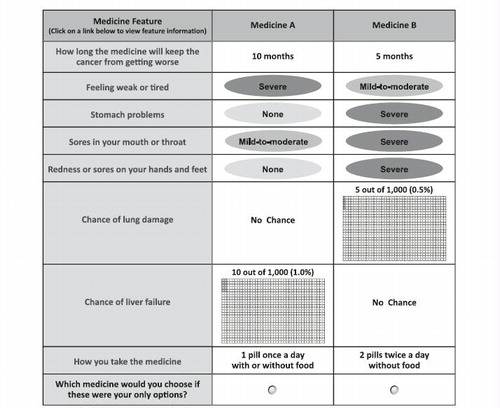
The experimental design used to construct the choice questions utilized the D-optimal main-effects criteria using SAS version 9.2 (SAS Institute, Inc., Cary, NC)Citation17,Citation18. The resulting experimental design consisted of 30 choice questions that were divided equally into three survey versions, each containing 10 choice questions. The order of the choice questions in each survey version was randomized for each patient. Each patient was randomly assigned to one of the three versions. Prior to answering the choice questions, patients were asked to assume that (1) their RCC was metastatic, (2) they were starting a new medication, (3) this was the last available medication they could take, and (4) all of their medical bills, including the cost of medications, were covered by health insurance.
Statistical analysis
The responses to the treatment-choice questions were analyzed using a random-parameters logit model, which controlled for the panel nature of the dataCitation19–21. The model was estimated using NLOGIT 4.0 (Econometric Software, Inc., Plainview, NY). In this model, the dependent variable was the patient’s choice, and the explanatory variables were the levels of the attributes shown in . For each attribute, we estimated the parameters of the omitted categories as the negative sum of the included categories as effects-coded variablesCitation21,Citation22. We estimated the standard errors for each omitted category using the variance–covariance matrix.
The resulting parameter estimates quantified the relative preference weight of each attribute levelCitation10,Citation16,Citation22. The vertical distance between the best and worst level (that is, difference in the model coefficients) of each attribute is a measure of the overall mean relative importance of that attribute (over the ranges presented in the survey) to RCC patients. Benefit equivalents, representing the amount of PFS patients would be willing to sacrifice to achieve a given reduction in toxicities, were estimated by dividing the difference in the preference weights of interest (e.g., for an improvement in fatigue from ‘mild-to-moderate’ to ‘none’) by the relevant preference weight for PFS. In addition, a series of sub-group analyses investigated the effects of patients’ demographic characteristics and health histories on their stated treatment preferences. Sub-groups of interest were defined by gender, treatment status, and metastases.
Results
Sample characteristics
E-mail invitations were sent to 4470 existing KCA panel members to ask potential respondents to participate in the online survey. Of the 363 panel members who responded by clicking on the link to the survey, 321 patients were eligible and consented to participate in the survey. Of these, 45 patients did not answer any choice questions and four had no variation in their responses to the choice questions (that is, they always chose Medicine A or Medicine B in each choice question); their answers were excluded from the survey. The number of patients in the final analysis was 272.
summarizes the demographic and baseline characteristics of the sample. The majority of the patients were female (53%), white (92%), married (74%), employed (56%), and had at least a college degree (66%). The mean age of the patients was 57 years (SD = 10 years). Also, 65% of the patients had been diagnosed with RCC at least 2 years ago, 98% had kidney surgery to treat RCC, 57% had non-metastatic disease, and 73% were currently not taking prescription medication.
Table 2. Demographics and baseline characteristics of the sample.
Preference weights
shows the preference weights (and 95% confidence intervals) for all the attribute levels. The estimated preference weights for seven of the eight attributes were consistent with the natural ordering of the levels—that is, better clinical outcomes were preferred to worse clinical outcomes. For example, 10 months of PFS had a higher preference weight than 5 months of PFS, and 5 months of PFS had a higher preference weight than 3 months of PFS. Fatigue was disordered between ‘none’ and ‘mild-to-moderate’ (i.e., the mean preference weight for no fatigue was slightly lower than that for mild-to-moderate fatigue); however, this disordering was not statistically significant (p > 0.05).
Figure 2. Preference weight graph. Preference weights for the eight attributes. Only relative differences matter when interpreting preference weights. The differences between adjacent weights indicate the relative importance of moving from one level of an attribute to an adjacent level of that attribute. The vertical distance between the best and worst level (that is, difference in the model coefficients) of each attribute is a measure of the overall mean relative importance of that attribute (over the ranges presented in the survey) to patients. The vertical bars surrounding each mean preference weight denote the 95% confidence intervals about the point estimate. If the confidence intervals do not overlap for adjacent levels in a particular attribute, the mean estimates are statistically different from each other at the 5% level of significance.
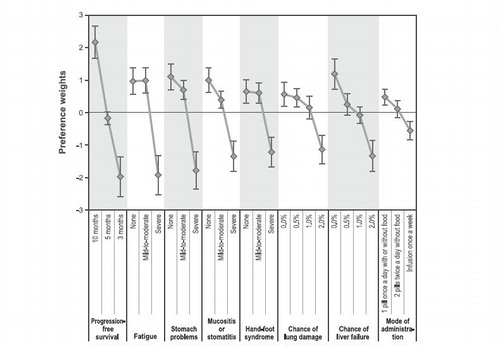
Within each attribute, the vertical distance between consecutive preference weights in indicates the relative importance of moving from one level of the attribute to an adjacent level of the same attribute. For example, the relative importance of an improvement in mucositis or stomatitis from ‘mild-to-moderate’ to ‘none’ was ∼ 0.6. Likewise, an improvement in the chance of pneumonitis (lung damage) from ‘1.0%’ to ‘0.0%’ had a relative importance of ∼ 0.5. Therefore, the improvement from ‘mild-to-moderate’ to ‘none’ on mucositis or stomatitis was ∼ 1.2-times as important as a 1.0 percentage-point decrease in the chance of pneumonitis. Similarly, an improvement in PFS from 5 months to 10 months (a relative importance of 2.4) was 4.0-times as important as an improvement from ‘mild-to-moderate’ to ‘none’ in mucositis or stomatitis.
Given the sample sizes for male and female patients, we found an overall statistically significant difference between male and female patients (p < 0.05). Over the 26 attribute levels, female patients were more averse to 3 months of PFS and severe stomach problems than male patients (p < 0.05), and male patients were more averse to infusion once a week than female patients (p < 0.05). However, no statistically significant differences were found between treatment-naïve and treatment-experienced patients (p > 0.05) and between metastatic and non-metastatic patients (p > 0.05).
Benefit equivalents
expresses the relative importance of changes in toxicities as benefit equivalents. Benefit equivalents represent the amount of benefit (in , months of PFS) that patients generally require to accept adverse events of varying severities. These measures also can be interpreted as the amount of benefit that patients would be willing to forego to avoid toxicities. For example, to reduce fatigue from ‘severe’ to ‘mild-to-moderate’, patients on average would be willing to forego 4.4 months of PFS. The benefit equivalents in are presented in descending order, from highest equivalent to lowest equivalent.
Figure 3. Benefit equivalents. Benefit equivalents, as measured in months of progression-free survival, are measures of the relative importance of changes in toxicities that patients generally were willing to accept in exchange for accepting adverse events of varying severities. The horizontal bars surrounding each benefit equivalent denote the 95% confidence intervals about the point estimate. If the confidence intervals do not overlap for two benefit equivalents, the mean estimates are statistically different from each other at the 5% level of significance. The confidence intervals were estimated using the Krinsky-Robb procedure of 10,000 draws by dividing the difference in the preference weights of interest (e.g., an improvement in fatigue from ‘mild-to-moderate’ to ‘none’) by the relevant preference weight interval for progression-free survival.
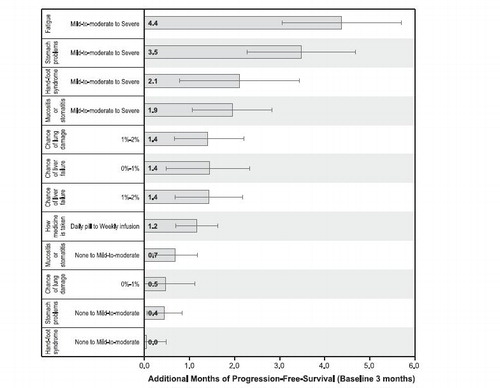
Discussion
This study of patient preferences for targeted drugs used in RCC in second and subsequent lines of therapies yielded several important results. This study showed that patients have distinct preferences and that some outcomes are more desirable than others. Using PFS as the reference or gold standard, the value of each toxicity can be expressed in a PFS ‘currency’ (much like the dollar has a gold value). Using this measure, changes in fatigue, stomach problems, and mucositis or stomatitis from ‘mild-to-moderate’ levels to ‘severe’ levels were the three most costly (i.e., least desirable) toxicities, in descending order of value.
Surprisingly, the toxicities that had lower incidence but were presumably more severe and potentially life-threatening (such as lung and liver toxicities) were uniformly ranked lower than the toxicities that were more prevalent but had a lower severity (such as fatigue, mucositis or stomatitis, and stomach problems). One hypothesis to explain this is that patients placed greater value on chronicity of those toxicities that occur and persist throughout the course of the illness: severe and episodic toxicities may be acceptable to patients as long as high quality-of-life exists in the interim. Patients also could have been influenced by the knowledge that these toxicities can be detected early (by imaging, for lung toxicity, or blood tests, for liver toxicity) and are potentially reversible. This study was not designed to account for such factors. Because of the potential seriousness of these toxicities and the need for medical intervention and resources, physicians typically place a high priority on these particular side-effects. The potential differences that may exist between physician and patient values on toxicities points to a gap in the therapeutic relationship and the need to carefully address this issue in future research.
This study had some similar findings to Mohamed et al.Citation10. First, PFS was the most important attribute (over the ranges of attributes and levels presented in the survey) to RCC patients in both studies, and fatigue and diarrhea (captured by stomach problems in this study) were rated as the most troublesome tolerability effects of RCC treatment. Thus, regardless of treatment line, PFS was very important to RCC patients. Second, patients were willing to accept significant treatment-related risks of serious adverse events to increase PFS. In contrast, RCC patients in this study were willing to accept higher risks of adverse events for an additional month of PFS than those in Mohamed et al.Citation10. This indicates that an additional month of PFS was more important to RCC patients on second or later lines of treatment than patients on first-line treatment.
The results of this study are best interpreted with several issues and qualifications in mind. First, despite the fact that health economists employ choice-format conjoint analysis to elicit patient preferences for a number of treatment outcomes, the method has inherent limiting factors. One such limitation is that the method asks patients to choose between two or more conceptual treatments. Health economists construct the treatments so as to elicit possible clinical decisions made by patients, but such decisions do not have the same impact, force, or consequences as decisions made in the real world. For example, we do not know whether real-world considerations (such as cost of treatment) will influence treatment decisions among these patients. Thus, differences can arise between stated and actual choices. Although we did not explicitly consider the interaction of patients and physicians when discussing RCC therapies in the real-world setting of an office visit, we have attempted to offer alternatives that not only mimic real-world trade-offs as closely as possible but also directly address our research question.
Additionally, patients were required to have a self-reported diagnosis of RCC, i.e., we did not require physician confirmation or chart review. There was a possibility that individuals who did not have RCC would participate in the survey, but this possibility was small because of the types of questions posed and because there was no monetary compensation given to the individuals themselves in exchange for time spent answering survey questions. Finally, we recruited patients through a patient group’s online member panel, which means that the sample used in this study is not necessarily representative of RCC patients in the US. It is possible that patients involved in an advocacy group may be more highly motivated to obtain treatment at any cost, which was not included in this study.
Results from the sub-group analysis should be interpreted as informative at best given that our study was not specifically powered to detect differences in preferences between sub-groups. In terms of gender, we found an overall statistically significant difference between male and female patients (p < 0.05). Over the 26 attribute levels, female patients were more averse to 3 months of PFS and severe stomach problems than male patients (p < 0.05), and male patients were more averse to infusion once a week than female patients (p < 0.05). Our sample had a low number of patients on prescription treatment (27%) and we found no statistically significant differences in the model results between patients on treatment and patients not on treatment (p > 0.05). Our sample was highly educated compared with the general population and may be a result of using an online format instead of mail or telephone formats. However, RCC is a rare form of kidney cancer, and an online survey with a nominal $20 donation for each completed survey was the best method available to collect data for this patient populationCitation23. Most patients in the sample were healthy (57% were non-metastatic, and only 27% were on active treatment), and we found no statistically significant differences in the model results between metastatic and non-metastatic patients (p > 0.05). Although more than half of the study sample did not have metastatic disease or might not experience severe side-effects of their RCC treatments, the healthier patients could be viewed as potential future decision-makers who may need targeted therapies in the future and therefore could be the appropriate patient population to determine which treatment attributes most influence treatment decisions. Our sample size of 272 was well within the range of other published choice-format conjoint studiesCitation11 and was adequately powered to estimate a preference model with acceptable confidence intervals for all parameters, while not specifically powered for detecting statistically significant differences in treatment preferences between patient sub-groups. Sample-size calculations represent a challenge in choice-format conjoint analysis. Minimum sample size depends on a number of criteria, including the question format, the complexity of the choice task, and the desired precision of the resultsCitation24.
In conclusion, patients placed the highest value on PFS, but were willing to make trade-offs in toxicities that appreciably affected their quality-of-life. Interestingly, patients placed significantly less value on therapies that differ in episodic, low-frequency, but potentially life-threatening toxicities. The results from this study can add the patient’s voice to the discussion about drug sequencing in RCC therapy.
Conclusion
The results from this study may inform treating oncologists about what patients value as important and about the trade-offs they are willing to consider during therapy. The results may add an extra dimension to the treatment algorithm. This information may be particularly useful in situations where the evidence is less robust (as in RCC drug sequencing) or in clinical equipoise (as in selecting between two VEGF TKIs in RCC). In addition, knowledge of patient preferences can help frame the difficult doctor–patient discussion about changing RCC therapy in the face of tumor progression or a limiting toxicity. Lastly, patient preferences are under-represented yet important considerations that should be incorporated into clinical treatment guidelines, drug development, and health policy-making.
Transparency
Declaration of funding
This study was funded by Novartis Pharmaceuticals Corporation, East Hanover, NJ.
Declaration of financial/other relationships
Dr Wong has teaching and speaking interests and membership on advisory committees or review panels for Pfizer, Genentech, Bristol-Myers Squibb, and Novartis. Dr Hauber, Ms Mohamed, and Ms Yang have disclosed that they are employees of RTI Health Solutions, a company that received funding from Novartis to conduct this study. Drs Liu, Rogerio, and Garay have stock ownership in Novartis.
Supplementary Material
Download PDF (1.1 MB)Acknowledgments
The authors would like to thank the Kidney Cancer Association for their assistance in locating RCC patients who were interested in participating in this study, and the patients who chose to participate in either the pilot study or the main study. The authors also would like to thank Paul Shannon and Stuart Yarr of RTI Health Solutions for programming the online survey and Vikram Kilambi and Ryan Ziemiecki of RTI Health Solutions for their assistance in analyzing the data.
References
- Furge KA, MacKeigan JP, Teh BT. Kinase targets in renal-cell carcinomas: reassessing the old and discovering the new. Lancet Oncol 2010;11:571-8
- Motzer RJ, Hutson TE, Tomczak P, et al. Sunitinib versus interferon alfa in metastatic renal-cell carcinoma. N Engl J Med 2007;356:115-24
- Escudier B, Eisen T, Stadler WM, et al. The TARGET Study Group. Sorafenib in advanced clear-cell renal-cell carcinoma. N Engl J Med 2007;356:125-34
- Escudier B, Pluzanska A, Koralewski P, et al. The AVOREN Trial Investigators. Bevacizumab plus interferon alfa-2a for treatment of metastatic renal cell carcinoma: a randomised, double-blind phase III trial. Lancet 2007;370:2103-11
- Hudes G, Carducci M, Tomczak P, et al. Global ARCC Trial. Temsirolimus, interferon alfa, or both for advanced renal-cell carcinoma. N Engl J Med 2007;356:2271-81
- Sternberg CN, Davis ID, Mardiak J, et al. Pazopanib in locally advanced or metastatic renal cell carcinoma: results of a randomized phase III trial. J Clin Oncol 2010;28:1061-8
- Fabian MA, Biggs WH, III, Treiber DK, et al. A small molecule-kinase interaction map for clinical kinase inhibitors. Nat Biotechnol 2005;23:329-36
- Agarwala SS, Case S. Everolimus (RAD001) in the treatment of advanced renal cell carcinoma: a review. Oncologist 2010;15:236-45
- NCCN. Clinical practice guidelines in oncology: kidney cancer. Fort Washington, NY: National Comprehensive Cancer Network, 2012. http://www.nccn.org/professionals/physician_gls/f_guidelines.asp. Accessed March 13, 2012
- Mohamed AF, Hauber AB, Neary MP. Patient benefit-risk preferences for targeted agents in the treatment of renal cell carcinoma. Pharmacoeconomics 2011;29:977-88
- Marshall D, Bridges JFP, Hauber AB, et al. Conjoint analysis applications in health–how are studies being designed and reported? An update on current practice in the published literature between 2005 and 2008. Patient 2010;3:249-56
- Bridges JFP, Kinter ET, Kidane L, et al. Things are looking up since we started listening to patients: trends in the application of conjoint analysis in health 1982-2007. Patient 2008;1:273-82
- Hauber AB, Mohamed AF, Beam C, et al. Patient preferences and assessment of likely adherence to hepatitis C virus treatments. J Viral Hepat 2011;18:619-27
- Hauber AB, Mohamed AF, Watson ME, et al. Benefits, risk, and uncertainty: preferences of antiretroviral-naïve African Americans for HIV treatments. AIDS Patient Care STDS 2009;23:29-34
- U.S. Department of Health and Human Services, National Institutes of Health, National Cancer Institute. The common terminology criteria for adverse events (CTCAE), version 4.0. Bethesda, MD: National Cancer Institute, 2009. http://www.acrin.org/Portals/0/Administration/Regulatory/CTCAE_4.02_2009-09-15_QuickReference_5x7.pdf. Accessed November 21, 2011.
- Bridges JFP, Hauber AB, Marshall D, et al. Conjoint analysis applications in health-a checklist: a report of the ISPOR Good Research Practices for Conjoint Analysis Task Force. Value Health 2011;14:403-11
- Kuhfeld W. Marketing research methods in SAS: experimental design, choice, conjoint, and graphical techniques. Cary, NC: SAS Institute Inc., 2010
- Kuhfeld W, Tobias F, Garratt M. Efficient experimental design with marketing research applications. J Mark Res 1994;31:545-57
- Train K. Mixed logit. In: Train K, ed. Discrete choice methods with simulation. Cambridge: Cambridge University Press, 2003
- Train K, Sonnier G. Mixed logit with bounded distributions of correlated partworths. In: Scarpa R, Alberini A, eds. Applications of simulation methods in environmental and resource economics. Dordrecht: Springer Publisher, 2005
- Hensher DA, Rose JM, Greene WH. Applied choice analysis. Cambridge: Cambridge University Press, 2005
- Bech M, Gyrd-Hansen D. Effects coding in discrete choice experiments. Health Econ 2005;14:1079-83
- Dillman DA, Smyth JD, Christian LM. Internet, mail, and mixed-mode surveys: the tailored design method. Hoboken, NJ: John Wiley & Sons, Inc., 2009
- Louviere JJ, Hensher DA, Swait JD. Stated choice methods: analysis and applications. New York: Cambridge University Press, 2000