Abstract
Background:
Economic evaluations are increasingly utilized to inform decisions in healthcare; however, decisions remain uncertain when they are not based on adequate evidence. Value of information (VOI) analysis has been proposed as a systematic approach to measure decision uncertainty and assess whether there is sufficient evidence to support new technologies.
Scope:
The objective of this paper is to review the principles and applications of VOI analysis in healthcare. Relevant databases were systematically searched to identify VOI articles. The findings from the selected articles were summarized and narratively presented.
Findings:
Various VOI methods have been developed and applied to inform decision-making, optimally designing research studies and setting research priorities. However, the application of this approach in healthcare remains limited due to technical and policy challenges.
Conclusion:
There is a need to create more awareness about VOI analysis, simplify its current methods, and align them with the needs of decision-making organizations.
Introduction
Comparative effectiveness research has been proposed as a potential avenue to identify, evaluate, and provide effective, safe, and cost-effective healthcare on the basis of informed and evidence-based decisionsCitation1. When comparing alternative healthcare options, it is essential to identify and combine the best available evidence on treatment effects, health-related preferences (utilities), resource use, and costsCitation2. Nevertheless, the evidence could be absent or uncertain due to the limitations and weaknesses of the available studies. A cost-effectiveness analysis that is based on such evidence is uncertain and, thus, any decision based on this analysis will also be uncertainCitation3. Decision uncertainty is associated with risk, because making the wrong decision could lead to costly consequences for the healthcare system (e.g. adopting sub-optimal treatment). Acquiring additional information could reduce uncertainty and better inform decisions; however, there is a cost for obtaining further evidence in terms of the direct costs of conducting research and the opportunity cost of delaying the decision awaiting research resultsCitation3,Citation4. In addition, under limited budgets, the money spent on a specific research study could be spent on healthcare or on other competing research proposals. Therefore, it is recommended to assess the need and value of additional research before making decisionsCitation5,Citation6.
Value of information analysis has been proposed to aid decision-makers decide simultaneously on the adoption of new technologies and the need for further research. Various value of information methods have been developed and successfully applied to inform whether there is sufficient evidence to support new technologies, optimally designing research studies and setting research prioritiesCitation7,Citation8.
The majority of the published papers on value of information analysis are methodologicalCitation7. Even in the applied papers, the topic is often presented with complexity, rendering this approach difficult to grasp by non-specialists. Thus, there is a need to present the principles and advantages of value of information analysis to decision-makers, researchers, and practitioners in a succinct but comprehensive manner. The objective of this paper is to review value of information principles and applications in healthcare.
Scope
The first section of this paper describes the principles of value of information analysis, and the second section reviews the applications of value of information in healthcare. The general approach is to identify the relevant literature to inform this review, searching various databases including PubMed, Medline, CINAHL, and the National Health Service Economic Evaluation Database for value of information articles published from January 2003 to January 2013. Search terms included: ‘value of information’, ‘value of perfect information’, ‘value of sampling information’, and ‘value of perfect parameter information’. These terms were searched in combination with the terms ‘decision-making’, ‘trial design’, and ‘research prioritization’. A narrative approach is used to summarize and present the principles and applications of value of information from the reviewed articles.
Principles of value of information analysis
Value of information analysis provides an analytic framework to quantitatively estimate the value of acquiring additional evidence to inform a decision problem. It is based on the notion that information is valuable because it reduces the expected cost of making the wrong decisions under uncertaintyCitation5,Citation6. By measuring the expected benefits of additional evidence and comparing this with the expected costs of further research, the value of information approach helps decision-makers answer the following five related questionsCitation8,Citation9:
Is additional research required? And, if yes,
What type of research?
Do the benefits of research exceed the costs?
What is the optimal research study design?
What priority should this research study take?
Is additional research required?
To know whether additional research is required, it is essential to consider the expected cost of the consequences of making a wrong decision (i.e. the cost of uncertainty)Citation3. High expected cost of uncertainty indicates a need for acquiring further information before making a decision. The expected cost of uncertainty is determined by two factors: (1) the probability that a decision is wrong, and (2) the consequences of this potentially wrong decisionCitation9.
To explain how the cost of uncertainty is estimated, a simplified hypothetical example is presented for two treatment interventions (A and B) modeled in a cost-effectiveness analysis. The uncertainty in the results of the cost-effectiveness analysis is characterized by presenting the expected net benefit estimates (i.e. effects measured in monetary terms minus costs) for each intervention. In this example, the model is calculated five times to reflect various possible values of the model parameters (). Because the expected average net benefit for intervention B ($1200) is higher than for A ($1000), selecting intervention B would be the best decision. However, this decision is imperfect as there is a 40% probability that a wrong decision is made; in two out of five scenarios treatment A is cost effective. The consequence of this wrong decision is the opportunity loss (i.e. benefit forgone) from choosing treatment B when treatment A was the preferred intervention. This opportunity loss is calculated by taking the difference between the net benefits of the two interventions in each scenario when A was preferred. The average opportunity loss across all scenarios is the expected cost of uncertainty of the decision for adopting treatment B, which is $40 per patient in this example. Equivalently, if we knew all parameters with absolute certainty (i.e. we have perfect information), we would choose the intervention with the maximum net benefit in each scenario. Averaging the maximum values across all scenarios gives the expected benefit of a decision made with perfect information, which is $1240. The difference between the expected benefit of a decision made with perfect information and a decision made without perfect information ($1240 − $1200 = $40 per patient) is the expected value of perfect information (EVPI) which is also the expected cost of uncertaintyCitation10.
Table 1. Illustrative example of the expected value of perfect information.
The EVPI calculated above is an average estimate (i.e. per patient EVPI). Multiplying per-patient EVPI by the population of patients expected to benefit from the evaluated intervention over a period of time gives the population EVPI which represents the maximum potential value (i.e. upper bound) of additional researchCitation8,Citation9. If the population EVPI appears to exceed the cost of additional research study, then this study is potentially worthwhile and further assessment is required to inform its optimal designCitation9,Citation11. Nevertheless, it has been argued that population EVPI is neither necessary nor sufficient to inform whether additional research is worthwhile, because it is impossible to estimate the expected cost of research without knowing the specific research study design (e.g. sample size, follow-up time)Citation8. However, calculating population EVPI is relatively simple and considered a continuation step to uncertainty assessment in cost-effectiveness analyses. When the population EVPI approaches zero it is unlikely that the value of additional research will exceed its cost and there will be no need to undertake further value of information analysesCitation12.
What type of research?
If further research appears potentially worthwhile based on the population EVPI, it would be useful to identify the particular aspects of a decision problem that are worth studying to resolve the uncertainty surrounding themCitation11. This could be achieved by estimating the expected value of information for certain input parameters in a given economic evaluation, often referred to as the partial EVPI or the expected value of perfect parameter information (EVPPI)Citation3. EVPPI is defined as the difference between the expected value of a decision made with perfect information on the selected parameters and the decision made based on current informationCitation11. EVPPI serves as a measure of the sensitivity of the economic evaluation to the uncertainty in its different input parametersCitation3,Citation11. A parameter with a higher EVPPI is more uncertain and further research can be designed and focused to get more precise estimate of its value. Importantly, the nature of the uncertain parameter(s) would inform the type and possibly the cost of the additional research study needed (e.g. randomized controlled or observational)Citation3,Citation11.
Do the benefits of research exceed the costs?
When the benefits of additional research study in reducing decision uncertainty exceeds its total cost, then this study is worthwhile. EVPI and EVPPI measure the expected value of additional research providing perfect information to resolve uncertainty of all parameters or specific parametersCitation10. However, acquiring perfect information requires a very large research sample (i.e. infinite sample size) which is not practical. In reality, it is only possible to reduce uncertainty with additional information from a research study of a finite sample sizeCitation5. The expected value of sample information (EVSI) estimates the expected value of reducing the uncertainty by a given research study with a specific sample size within a particular study designCitation8. This can be calculated for all effect and cost parameters (i.e. total EVSI) or for the parameter(s) of interest (i.e. partial EVSI)Citation13. Population EVSI is calculated by multiplying the per-patient EVSI by the size of the population to whom information from the trial is valuableCitation8.
The expected total cost of a research study includes three components: (1) fixed cost (e.g. set-up cost, salaries), (2) variable cost per patient, and (3) an opportunity cost for those patients who receive the inferior intervention while the study is underwayCitation6. The total cost commonly takes a societal perspective; however, this cost may also be from the perspective of the sponsor of the study. The difference between the population EVSI for a specific study design and its expected total cost is the expected net benefit of sampling (ENBS)Citation8. A positive ENBS indicates that the research study is worthwhile. Conversely, when the ENBS is negative, it would be irrational to conduct further research because the expected costs of the study exceed its expected benefits, and, in this case, the current available evidence is sufficient for decision-makingCitation14. The EVSI and the ENBS are the preferred measures of value of information because they are sufficient to inform whether a specific research study is potentially worthwhileCitation8.
What is the optimal research study design?
The sample sizes of clinical trials are usually calculated based on type I and II error, and the minimum clinically important differenceCitation15. The value of information framework provides an alternative to the standard hypothesis testing approach which relies on arbitrary chosen error probabilities where type I and type II errors receive the same weight (e.g. 5% and 20%, respectively) regardless of the consequences of making an errorCitation10,Citation16. shows the population EVSI across a number of sample sizes for a future research study. As the sample size increases and more uncertainty resolved the calculated population EVSI converges to the population EVPI (i.e. upper bound). Deducting the expected total cost from the EVSI results in the ENBS curve which, in this example, is positive for a wide range of sample sizes; however, the ENBS is at maximum when the sample size is 250 patients in each arm, which represents the optimal sample size.
Figure 1. Optimal sample size determination using value of information methods. EVSI, expected value of sample information; ENBS, expected net benefit of sampling.
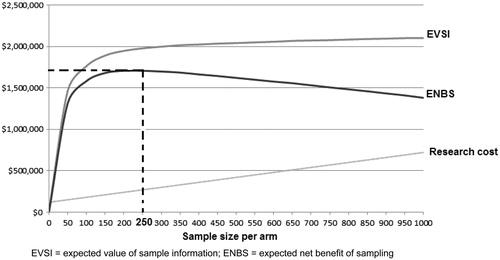
Beyond sample size determination, value of information analysis can optimize additional aspects of research design such as possible comparator arms and alternative follow-up periodsCitation8,Citation17. More uncertainty is expected to resolve with longer follow-up and more comparator arms, albeit with additional research costs. The preferred trial design would be the one that maximizes the ENBSCitation8.
What priority should this research study take?
Typically, decisions to fund and prioritize research proposals have been subjectively made based on the opinions, judgments, and consensus among experts on a research panel evaluating the scientific merit and relevance of the proposalsCitation18. However, different objective approaches have been proposed and implemented to prioritize research projects such as the burden of disease and the ‘payback’ approachCitation18,Citation19. In the burden of the disease, the higher the cost of a disease the greater the need for research; however, this does not take into consideration the expected incremental costs and returns from the additional researchCitation8,Citation18. Moreover, the burden of the disease approach might undermine investment in rare diseases as it focuses the decision-maker’s attention on common diseases where there is usually a high illness cost. In the ‘payback’ approach, however, the costs and benefits from conducting and implementing research are evaluated and comparedCitation8,Citation18. Under this approach, a research project is worthwhile if its benefits outweigh the expected costsCitation18. Nevertheless, the ‘payback’ requires the comparison of the costs and benefits of undertaking a pre-designed research project, implicitly assuming that the proposed research has been optimally designedCitation20. The value of information analysis has been proposed as an alternative quantitative approach to prioritize research studiesCitation8,Citation21. Under the value of information approach, competing research proposals are ranked according to their expected values, whereby priority is given to the studies with the highest ENBSCitation8. This is illustrated in a hypothetical example in where five research proposals are being compared. Nevertheless, it has been argued that the proposal with the highest ENBS may not necessarily provide the highest return on investment (i.e. ENBS divided by the expected total cost of research)Citation8.
Table 2. Prioritizing alternative research proposals using the value of information approach.
Value of information applications in healthcare
Value of information analysis is increasingly applied in healthcare to inform decisions, optimize trial design, and prioritize researchCitation8. In a systematic review on the application of value of information in health technology assessment a total of 118 papers were identified, of which 59 were appliedCitation7. The authors of this review observed a rapidly accumulating literature base on value of information from 1999 onwards for methodological papers and from 2005 onwards for applied papersCitation7. Most of the identified applied articles estimated the EVPI and the EVPPI, indicating that the majority of the studies used the value of information approach to estimate the maximum value of additional information to assess whether further research is warrantedCitation7. However, a limited number of applied papers reported the preferred value of information measures of EVSI (six articles) and ENBS (four articles)Citation7. Similar results were reported in a recent systematic review on value of information application in oncology, where less than 10% of the identified articles reported the use of this approach to inform optimal trial design and research prioritizationCitation22.
Informing decisions
The most explicit use of value of information methods to inform decisions is by the National Institute for Health and Clinical Excellence (NICE) in England. Claxton et al.Citation9 have developed a value of information based framework for NICE to inform the following decision options:
Approve based on existing information.
Approval with research (i.e. Approve and ask for additional research).
Only in research (i.e. Delay approval and ask for additional research).
Reject based on existing information.
Generally, when the technology is cost-effective, ‘approval with research’ would be appropriate if additional research is possible and worthwhileCitation9. Conversely, if the technology is not cost-effective but additional research is worthwhile, ‘only in research’ would be the preferred option. Nevertheless, exceptions from this general rule would be appropriate depending on the presence of irrecoverable cost associated with the adoption of the new intervention (e.g. cost of training)Citation9. Thus, ‘only in research’ or even ‘reject based on existing information’ rather than ‘approval with research’ or ‘approve based on existing information’ may be appropriate even if research is possible when there are significant irrecoverable adoption costsCitation9.
A recent study reviewed NICE technology appraisals with ‘only in research’ or ‘approval with research’ recommendations and examined the key considerations that led to those decisionsCitation23. In total, 29 final and 31 draft guidance documents included ‘only in research’ or ‘approval with research’ recommendations up to 2010. Overall, 86% of final guidance included ‘only in research’ recommendations. Of these, the majority was for technologies considered to be cost ineffective (83%), while 66% of the final guidance specified the need for further evidence on relative effectivenessCitation23.
From the industry perspective, any change in the price of the intervention, such as through patient access schemes or price negotiations, will affect the key assessments, possibly leading to a different decisionCitation9,Citation24. Subsequently, once the need for additional information and the size of irrecoverable costs are recognized, the threshold price that would lead to ‘adopt based on existing evidence’ rather than ‘only in research’ will always be lower than a single value-based price based on expected cost effectiveness aloneCitation9. Willan and EckermannCitation25 have proposed a framework to bring together the societal and industry perspectives, allowing for trade-offs between the value and cost of research and the price of the new intervention. Under this framework, if the decision-maker’s threshold price exceeds the sponsor’s (industry), then current evidence is sufficient since any price between the thresholds is acceptable to both. However, if the decision-maker’s threshold price is lower than the company’s, then no price is acceptable to both and the company’s optimal strategy is to conduct additional researchCitation25.
Optimizing trial design
The use of the value of information methods in optimizing trial design remains limited and most applications have been restricted to the estimation of optimal sample size, and mainly in two-arm randomized trialsCitation14,Citation26,Citation27. For example, Koerkamp et al.Citation26,Citation28 applied value of information analysis to patient-level data from two randomized trials on intermittent claudication and magnetic resonance imaging (MRI) in acute knee trauma. The optimal study design for the treatment of intermittent claudication would involve a randomized controlled trial collecting data on the quality-adjusted life expectancy and additional admission costs for 525 patients per treatment armCitation26. For the MRI in acute knee trauma, three parameters were found responsible for most of the decision uncertainty: number of quality-adjusted life-years, cost of an overnight hospital stay, and friction costsCitation28. A study in which data on these three parameters are gathered would have an optimal sample size of 3500 patients per armCitation28. Soares et al.Citation29 showed how value of information analysis informed the optimal future trial design on negative-pressure wound therapy for severe pressure ulcers. In their study, a three-arm trial with 1-year follow-up and a sample size of 497 patients (in each arm) was estimated to be the most efficientCitation29.
Prioritizing research
In a survey prepared for the Agency for Healthcare Research and Quality (AHRQ), research prioritization approaches for 48 research-sponsoring organizations from the US, UK, Australia, Germany, and Canada were identified and comparedCitation30. The results showed that only 31 (65%) organizations utilized specific priority-setting methods. The most explicit use of value of information and other quantitative methods was by NICE, where the assessment is usually performed by a network of academic centers under the umbrella of the National Institute for Health Research (NIHR) Health Technology Assessment programCitation30. This is expected, because in the UK, where research is often commissioned on a tender basis, the application of value of information methods in identifying areas of value for funding bodies may be useful. On the other side, in settings where grant applicants have a more active role in defining research questions (e.g. US and Australia), it is suggested that more emphasis be placed on application of value of information methods by applicants in showing the connection of proposed trial designs to value of research and decision-makingCitation8.
Recently, Carlson et al.Citation31 have evaluated the feasibility and outcomes of incorporating value of information analysis into a stakeholder-driven research prioritization process within a program to prioritize comparative effectiveness research in cancer genomics. The authors described how they convened an external group of stakeholders to identify three high-priority cancer genomics tests for further research and to rank these in order of priority for conducting further researchCitation31. These test included expression testing for platinum-based adjuvant therapy (ERCC) in resectable non-small cell lung cancer (NSCLC), epidermal growth factor receptor (EGFR) mutation testing for erlotinib maintenance therapy in advanced NSCLC and breast cancer tumor markers (BC markers) for detection of recurrence after primary breast cancer therapyCitation31. The study demonstrated how providing the stakeholders with value of information estimates about the three tests resulted in participants changing their ranking of the tests from (1) ERCC1, (2) EGFR, and (3) BC markers to (1) ERCC1, (2) BC markers, and (3) EGFRCitation31.
Challenges for value of information application
The wide adoption of value of information methods in healthcare faces technical and policy challenges. From a technical perspective, conducting value of information analyses, especially calculating the EVSI and the EVPPI in non-linear models, requires sophisticated computations together with advanced expertise in economic evaluation and simulation techniquesCitation7; nevertheless, recent years have witnessed a progressive evolution and simplification of methods as well as advanced computing tools to reduce computational challengesCitation8,Citation32–35. Another technical challenge is that certain assumptions are necessary when estimating value of information measures. These include the population expected to benefit from the technology, the lifetime of the technology, and the level of its implementation since the value of research is reduced if the results were not fully implementedCitation4,Citation36–38. Several papers have addressed these assumptions and provided guidance to handle the uncertainty surrounding their estimatesCitation4,Citation36–38.
For the policy aspect, the main issue is that the decisions to adopt technologies and to conduct research are usually separateCitation11. Claxton and SculpherCitation11 noted this point in their first pilot study on value of information: ‘The key problem seems to be the policy environment where accountability and transparency for research prioritization and commissioning lags behind adoption and reimbursement decisions, and where there appears to be a separate remit for reimbursement and research decisions’ (p. 1067). Furthermore, the approach is relatively new and it will be some time before its value is realized by decision-makers. Therefore, for the value of information analysis to be more incorporated into decision-making frameworks, there is a need to create more awareness about the value of this approach and to align its methods with the needs of the decision-making organizationsCitation7.
Conclusion
Value of information analysis is a systematic framework to measure decision uncertainty and assess whether there is sufficient evidence to support new technologies. Various value of information methods have been developed to inform decision-making, optimally designing research studies and setting research priorities. The application of value of information analysis in healthcare is increasing but remains limited due to conceptual, technical and policy challenges. Therefore, there is a need to create more awareness about this approach, simplify its current methods, and align them with the needs of the different jurisdictions in order for this approach to be incorporated into decision frameworks.
Transparency
Declaration of funding
This review was not funded.
Declaration of financial/other interests
The authors have no relevant financial relationships to disclose. HT is supported by an NHMRC PhD scholarship. PS is a member of the JME Editorial Board. JME peer reviewers on this manuscript have no relevant financial or other relationships to disclose.
References
- Lyman GH, Levine M. Comparative effectiveness research in oncology: an overview. J Clin Oncol Official J Am Soc Clin Oncol 2012;30:4181-4
- Sculpher M, Claxton K. Establishing the cost-effectiveness of new pharmaceuticals under conditions of uncertainty–when is there sufficient evidence? Value Health 2005;8:433-46
- Claxton K. Exploring uncertainty in cost-effectiveness analysis. Pharmacoeconomics 2008;26:781-98
- Eckermann S, Willan AR. Time and expected value of sample information wait for no patient. Value Health 2008;11:522-6
- Claxton K. The irrelevance of inference: a decision-making approach to the stochastic evaluation of health care technologies. J Health Econ 1999;18:341-64
- Eckermann S, Willan AR. Expected value of information and decision making in HTA. Health Econ 2007;16:195-209
- Steuten L, van de Wetering G, Groothuis-Oudshoorn K, et al. A systematic and critical review of the evolving methods and applications of value of information in academia and practice. Pharmacoeconomics 2013;31:25-48
- Eckermann S, Karnon J, Willan AR. The value of value of information best informing research design and prioritization using current methods. Pharmacoeconomics 2010;28:699-709
- Claxton K, Palmer S, Longworth L, et al. Informing a decision framework for when NICE should recommend the use of health technologies only in the context of an appropriately designed programme of evidence development. Health Technol Assess 2012;16:1-323
- Claxton K, Posnett J. An economic approach to clinical trial design and research priority-setting. Health Econ 1996;5:513-24
- Claxton KP, Sculpher MJ. Using value of information analysis to prioritise health research: some lessons from recent UK experience. Pharmacoeconomics 2006;24:1055-68
- Tuffaha HW, Rickard CM, Webster J, et al. Cost-effectiveness analysis of clinically indicated versus routine replacement of peripheral intravenous catheters. Appl Health Econ Health Policy 2014;12:51-8
- Ades AE, Lu G, Claxton K. Expected value of sample information calculations in medical decision modeling. Med Decis Mak 2004;24:207-27
- Willan AR, Goeree R, Boutis K. Value of information methods for planning and analyzing clinical studies optimize decision making and research planning. J Clin Epidemiol 2012;65:870-6
- Willan AR. Sample size determination for cost-effectiveness trials. Pharmacoeconomics 2011;29:933-49
- Willan AR, Pinto EM. The value of information and optimal clinical trial design. Stat Med 2005;24:1791-806
- McKenna C, Claxton K. Addressing adoption and research design decisions simultaneously: the role of value of sample information analysis. Med Decis Mak 2011;31:853-65
- Fleurence RL. Setting priorities for research: a practical application of ‘payback' and expected value of information. Health Econ 2007;16:1345-57
- Davies L, Drummond M, Papanikolaou P. Prioritizing investments in health technology assessment. Can we assess potential value for money? Int J Technol Assess Health Care 2000;16:73-91
- Chilcott J, Brennan A, Booth A, et al. The role of modelling in prioritising and planning clinical trials. Health Technol Assess 2003;7:1-138
- Fleurence RL, Meltzer DO. Toward a science of research prioritization? The use of value of information by multidisciplinary stakeholder groups. Med Decis Making 2013;33:460-2
- Tuffaha HW, Gordon LG, Scuffham PA. Value of information analysis in oncology: the value of evidence and evidence of value. J Oncol Pract 2014;10(2):e55-e62
- Longworth L, Youn J, Bojke L, et al. When does NICE recommend the use of health technologies within a programme of evidence development?: a systematic review of NICE guidance. Pharmacoeconomics 2013;31:137-49
- Walker S, Sculpher M, Claxton K, et al. Coverage with evidence development, only in research, risk sharing, or patient access scheme? A framework for coverage decisions. Value Health 2012;15:570-9
- Willan AR, Eckermann S. Value of information and pricing new healthcare interventions. Pharmacoeconomics 2012;30:447-59
- Koerkamp BG, Spronk S, Stijnen T, et al. Value of information analyses of economic randomized controlled trials: the treatment of intermittent claudication. Value Health 2010;13:242-50
- Stevenson MD, Jones ML. The cost effectiveness of a randomized controlled trial to establish the relative efficacy of vitamin K1 compared with alendronate. Med Decis Mak 2011;31:43-52
- Koerkamp BG, Nikken JJ, Oei EH, et al. Value of information analysis used to determine the necessity of additional research: MR imaging in acute knee trauma as an example. Radiology 2008;246:420-5
- Soares MO, Dumville JC, Ashby RL, et al. Methods to assess cost-effectiveness and value of further research when data are sparse: negative-pressure wound therapy for severe pressure ulcers. Med Decis Mak 2013;33:415-36
- Myers E, Sanders GD, Ravi D, et al. Evaluating the potential use of modeling and value-of-information analysis for future research prioritization within the evidence-based practice center program. Rockville, MD: Agency for Healthcare Research and Quality, 2011. Available from: http://www.ncbi.nlm.nih.gov/books/NBK62134 [Last accessed January 2014]
- Carlson JJ, Thariani R, Roth J, et al. Value-of-information analysis within a stakeholder-driven research prioritization process in a US setting: an application in cancer genomics. Med Decis Mak 2013;33:463-71
- Sadatsafavi M, Bansback N, Zafari Z, et al. Need for Speed: an efficient algorithm for calculation of single-parameter expected value of partial perfect information. Value Health 2013;16:438-48
- Sadatsafavi M, Marra C, Bryan S. Two-level resampling as a novel method for the calculation of the expected value of sample information in economic trials. Health Econ 2013;22:877-88
- Brennan A, Kharroubi SA. Efficient computation of partial expected value of sample information using Bayesian approximation. J Health Econ 2007;26:122-48
- Brennan A, Kharroubi S, O'Hagan A, et al. Calculating partial expected value of perfect information via Monte Carlo sampling algorithms. Med Decis Mak 2007;27:448-70
- Fenwick E, Claxton K, Sculpher M. The value of implementation and the value of information: combined and uneven development. Med Decis Mak 2008;28:21-32
- Philips Z, Claxton K, Palmer S. The half-life of truth: what are appropriate time horizons for research decisions? Med Decis Mak 2008;28:287-99
- Willan AR, Eckermann S. Optimal clinical trial design using value of information methods with imperfect implementation. Health Econ 2010;19:549-61