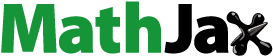
Abstract
Advances in the field of T cell immunology have contributed to the understanding that cross-reactivity is an intrinsic characteristic of the T cell receptor (TCR), and that each TCR can potentially interact with many different T cell epitopes. To better define the potential for TCR cross-reactivity between epitopes derived from the human genome, the human microbiome, and human pathogens, we developed a new immunoinformatics tool, JanusMatrix, that represents an extension of the validated T cell epitope mapping tool, EpiMatrix. Initial explorations, summarized in this synopsis, have uncovered what appear to be important differences in the TCR cross-reactivity of selected regulatory and effector T cell epitopes with other epitopes in the human genome, human microbiome, and selected human pathogens. In addition to exploring the T cell epitope relationships between human self, commensal and pathogen, JanusMatrix may also be useful to explore some aspects of heterologous immunity and to examine T cell epitope relatedness between pathogens to which humans are exposed (Dengue serotypes, or HCV and Influenza, for example). In Hand-Foot-Mouth disease (HFMD) for example, extensive enterovirus and human microbiome cross-reactivity (and limited cross-reactivity with the human genome) seemingly predicts immunodominance. In contrast, more extensive cross-reactivity with proteins contained in the human genome as compared to the human microbiome was observed for selected Treg epitopes. While it may be impossible to predict all immune response influences, the availability of sequence data from the human genome, the human microbiome, and an array of human pathogens and vaccines has made computationally–driven exploration of the effects of T cell epitope cross-reactivity now possible. This is the first description of JanusMatrix, an algorithm that assesses TCR cross-reactivity that may contribute to a means of predicting the phenotype of T cells responding to selected T cell epitopes. Whether used for explorations of T cell phenotype or for evaluating cross-conservation between related viral strains at the TCR face of viral epitopes, further JanusMatrix studies may contribute to developing safer, more effective vaccines.
Introduction
JanusMatrix is a new immunoinformatics tool that was developed to compare T cell epitope conservation between protein sequences from bacterial and viral organisms that make up the human gut microbiome (HM), autologous proteins from the human genome (HG), and human viral and bacterial pathogens (HP). We have used JanusMatrix to initiate the exploration of the relationships between conservation with self (and non-self), T cell phenotype (regulatory or effector), and immunodominance. For example, we postulated that regulatory T cells (Treg) might bear T cell receptors (TCRs) that are more likely to bind to T cell epitopes that are cross-conserved (at the TCR face) in many human (self) proteins, while, by comparison true effector T cells (Teff) might bear TCRs that recognize TCR-facing residues that are less cross-conserved with human genome proteins. We reasoned that such cross-conserved epitopes might be important to remove from vaccines, because of the potential for cross-reactive T cells to the vaccine epitopes to suppress, or alter, the desired immune response. The tool may also be used to uncover pathogen epitopes that are associated with autoimmune responses, and epitopes that are conserved between two similar pathogens (Dengue serotypes) or slightly dissimilar pathogens (West Nile Virus and Yellow Fever Virus, for example). The purpose of this review is to describe the use of this tool, as it is currently being implemented in our research programs, and to illustrate several potential applications of JanusMatrix to vaccine research and development.
The mechanics of T cell receptor cross-reactivity
T cells recognize linear peptides or “T cell epitopes” that form a complex with human (HLA) or other species’ MHC molecules. The T cell bears a surface receptor (T cell receptor, or TCR) that binds to the T cell epitope’s amino acid side chains facing upwards, out of the MHC binding cleft, while the MHC binds to the T cell epitope’s amino acid side chains facing into “pockets” located on the sides and bottom of the binding cleft. The T cell epitope face that binds into the MHC-binding cleft is known as the “agretope", while “epitope” refers to either the whole linear peptide or to the TCR face of the peptide ().
Figure 1.JanusMatrix separates the amino acid sequence of T cell epitopes into TCR-facing residues and HLA binding cleft-facing residues, and then compares the TCR face to other putative T cell epitopes. JanusMatrix defines cross-reactive T cell epitopes as those that have the same MHC allele restriction, the same or similar T cell-facing residues (epitope), and conserved binding of MHC-facing residues (agretope). The HLA-facing residues of the comparators are allowed to vary, as long as they still bind to the original HLA allele. Epitopes that are identical in terms of their TCR face and are equally able to bind to the identical HLA, but differ in sequence, are rapidly identified from a given database of genomic sequences. This enables large-scale comparisons between TCR-homologous T cell epitopes from the HG, the HM, and the HP.
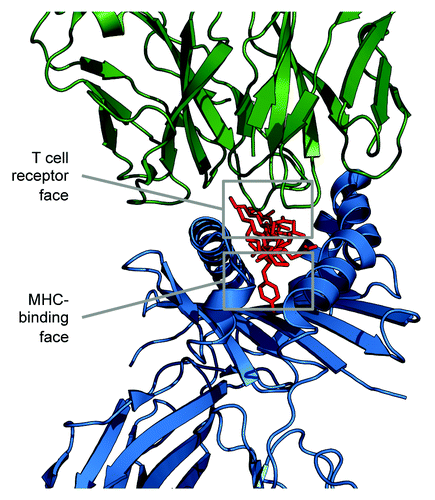
Effective T cell immunity requires that the host be able to generate a specific response to antigenic epitopes without any prior knowledge of their composition. In this regard, the immune system faces a fundamental dilemma: how to cover the vastly higher number of potential epitopes (estimated at >1012) with available TCRs (estimated maximum of <108 in humans). One hypothesis for resolving this dilemma is that TCRs may recognize more than one peptide epitope. This theory has since been validated: indeed, each TCR has the potential to recognize as many as one million peptides. The possibility for cross-reactivity may seem high and even potentially dangerous, but given the number of potential T cell epitopes represented by modifying the 20 amino acids at each of the TCR-facing positions, it turns out that this reflects a minimum cross-specificity of 1:100,000 peptides.Citation1 Thus, there is a relatively limited probability of cross-reactivity with self as well as foreign antigens. While it is true that altering MHC binding residues can modify the affinity of the peptide and change the shape of the TCR face, the TCR has been shown to adapt to minor changes, enabling a single TCR to bind to more than one epitope containing the same TCR-facing but different MHC-binding-facing residues.Citation2,Citation3
Rules of contact enable the generation of TCR cross-reactivity predictions
Based on crystal structures of ternary MHC:peptide:TCR structures, general patterns of contact have been observed, with some peptide residues frequently in contact with the MHC and others frequently in contact with the TCR.Citation4 These TCR-facing and MHC-facing amino acid residue rules can be adapted for use with T cell epitope prediction tools such as EpiMatrixCitation5 to define cross-conserved TCR-facing residues across large sequence databases (see JanusMatrix algorithm, below). Once a single T cell epitope is defined, by holding its TCR-facing residues constant and allowing its MHC-binding residues to vary, it becomes possible to search protein sequence databases for epitopes that appear “homologous” to a given TCR, despite minor variations in MHC binding residues, that will still be predicted to bind to the same MHC. As a first approximation, this approach reduces the number of potential cross-reactive sequences identified and makes experimental validation feasible. In reality, however, TCRs are not required to recognize HLA-bound peptides by sequence similarity but rather by peptide side chain accessibility.Citation6,Citation7 Consequently, the set of potential cross-reactive sequences is very likely dramatically greater and would be unwieldy in initial validation experiments. We believe experimental confirmation in the simplest case (identical TCR-facing residues) is a reasonable first step to be followed by computational screening of an expanded sequence/structure space to capture additional cross-reactive epitopes.
TCR cross-reactivity between self and non-self
Cross-reactivity is an intrinsic characteristic of the TCR, i.e., each single TCR can potentially interact with different peptide-MHC complexes. In fact, T cell epitope cross-reactivity is critical to many aspects of T cell biology, including positive and negative selection. Cross-reactive immunity can have either advantageous or negative (e.g., leading to pathology) consequences. Deleterious autoimmune cross-reactivity can occur following infection, a response that has previously been described as molecular mimicry. For example, T cell epitope cross-reactive immune responses may contribute to the pathogenesis of systemic lupus erythematosus,Citation8 tropical spastic paraparesis,Citation9 and diabetes,Citation10 among other conditions. While we have not yet used JanusMatrix to define cross-reactivity between human pathogens and T cell epitopes associated with auto-immune disease, this is an area of great interest that we are likely to pursue in the future.
Heterologous immunity: TCR cross-reactivity between pathogens
Heterologous immunity is a term coined by Welsh and Selin to describe the partial immunity (or altered immunopathology) that occurs in response to a pathogen if the host has been previously infected or immunized with a particular unrelated pathogen. Both human and murine immune responses to antigens can be modified as a consequence of T cell cross-reactivity.Citation11,Citation12,Citation13 In some cases, heterologous immunity is attributed to T cells that cross-react with both pathogens. CD8+ T cell cross-reactivity has been documented between two epitopes on the same viral protein, between epitopes of different proteins from the same virus and, more importantly, between epitopes of proteins from different viruses.Citation14
Heterologous immunity research focused initially on specific viral infections (e.g., vaccinia, EBV, influenza).Citation15,Citation16 It is now clear that T cells stimulated by epitopes from a given pathogen may subsequently respond differently to cross-reactive similar epitopes with later infection by a different virus.Citation17 T cells that have been previously activated due to a cross-reactive infection are capable of restricting pathogen growth and reducing the severity of the second infection, which can be viewed as a positive effect. In the context of some infections caused by different viruses, heterologous immunity may contribute to the immunodominance of low-avidity memory responses over higher-avidity naïve responses. However, in other circumstances, the result of cross-reactive immunity is enhanced pathogen growth and/or immunopathology.Citation18 Proposed mechanisms underlying these negative effects may include induction of Treg or, in contrast, increased production of inflammatory cytokines.Citation11,Citation19,Citation20,Citation21 JanusMatrix is currently being used to search for epitopes that may contribute to heterologous immunity.
The relevance of the HM to adaptive T cell responses
Evidence linking gut microbes, adaptive immunity, metabolic syndrome, allergy, and autoimmune disorders has emerged.Citation22,Citation23 For example, linkages between immune responses to the HM and multiple sclerosis have been defined,Citation23 as have links between microbiota and autoimmune diseases of the gut.Citation24,Citation25 Exposure to a diverse array of gut microbes may reduce susceptibility to autoimmune diseases and allergy (the so-called “hygiene hypothesis”).Citation22,Citation23,Citation26 This may be attributable to direct interactions between the microbes and the human immune system (due to not-yet-defined factors) or to modulation of immune responses to self and other potential pathogens.Citation24,Citation27-Citation29
In addition, there is a growing body of evidence that the gut microbiome shapes adaptive immune responses.Citation27,Citation30,Citation31 Notably, post-thymic educated gut CD4+ T cells differentiate to become inducible Treg by training on commensal antigens.Citation32 This finding is significant because it establishes the existence of T cells with specificities for commensals, which may cross-react with pathogen- or vaccine-derived sequences. Since the gut microbiome is both vast and highly variable, it is likely that commensal-shaped immune responses are highly diverse and vary from person to person due to individual variations in the microbiome (related to diet or geography) and to genetic differences in HLA. In publishedCitation33 and yet-to-be-publishedCitation34 work, Sztein and VerBerkmoes have established the uniqueness and commonalities between individual microbial and human proteomes.
The availability of fast and accurate tools to analyze a large number of sequences for common TCR-facing residues now makes it both feasible and exciting to examine the role that cross-reactive T cell epitopes play in the generation of autoimmune and the modulation of adaptive immune responses. In the next section, we provide a brief description of the JanusMatrix tool and its application to: (1) evaluations of common “positive control” T cell epitopes for cross-reactivity between the HG and HM; (2) understanding the pathogenesis of hepatitis C virus (HCV); and (3) the discovery of T effector epitopes in EV71, the etiologic agent of Hand-Foot-Mouth disease (HFMD). For these initial studies, we applied JanusMatrix retrospectively to explore cross-reactive patterns of previously studied epitopes that induced various T cell responses, as well as prospectively to identify and experimentally evaluate new cross-reactive epitopes predicted to induce various responses.
Results
Developing epitope “silos”
Using EpiMatrix and JanusMatrix, we mapped groups of peptides that were experimentally shown to induce a defined T cell response (Teff, Treg, or no response) and searched for trends in the number of cross-reactive TCR-facing epitopes across the HG, HM, and HP databases supported by JanusMatrix. A set of 10,000 random nine-mers was also evaluated for comparison.
Based on cross-reactivity patterns, T cell epitopes examined thus far appear to fall into one of three categories, or “silos”: (1) patterns that are characteristic of Treg epitopes; (2) patterns that are characteristic of Teff epitopes; and (3) patterns associated with epitopes that are “null” (neither effector nor regulatory but are predicted to bind to HLA). We find these distinctive cross-reactivity patterns are generally correlated with the types of T cell epitopes defined in the literature. Specifically, T cell epitopes that appeared to be immunodominant were associated with Treg responses, as defined by upregulation of FoxP3 or expansion of CD4+CD25hi Treg, and had higher levels of cross-reactivity with the HG.
describes the emerging JanusMatrix patterns associated with T cell epitope categories (Treg, Teff) and provides an example for each category (a defined Treg epitope for HCVCitation35,Citation36,Citation37 and a defined Teff epitope in influenzaCitation38) in terms of their TCR cross-reactivity with the HG, HM, and selected human viral and bacterial pathogens (HP). “CEFT” epitopes that are commonly used as positive controls in human T cell assays to elicit a measurable antigen-specific memory response (see list of these epitopes in ref. Citation39) and Tregitopes (see ref. Citation40) were tested as representative sets of T effector and T regulatory epitopes respectively. Medians and ratios of cross-reactive hits are shown. We also describe cross-reactivity that could be expected in a random protein: 10,000 random nine-mers predicted to be HLA ligands show the lowest median of cross-reactive hits for each database analyzed. Number of genomes, proteins, and amino acids per database is also shown. Results of the statistical analysis (P-values) of the comparison between the distributions of ratios of cross-reactive hits between databases and types of epitopes are also shown in sub-tables. Comparisons between type of epitopes by database (e.g. CEFT vs. Treg for HG; see sub-table A) show that (1) Treg and CEFT are only different for HG; and (2) for the three databases, CEFT and Tregs are different from random; the only exception is the comparison of HP CEFT vs. Random. Comparisons between databases by type of epitope (e.g. HG vs. HM for CEFT; see sub-table B) we observe that (1) for CEFT, the distributions of the ratios of cross-reactive hits of the three databases are not different; (2) for Tregs, HG is different from both HM and HP and HM is not different from HP; and (3) for random peptides, HG, HM, and HP are all different.
Table 1. JanusMatrix TCR-cross reactivity frequencies for three types of epitopes
In summary, these results indicate that validated Treg epitopes have statistically greater TCR cross-reactivity with the human genome, based on JanusMatrix. Teff and Treg cross-reactivities with HG, HM, and HP are different from random nine-mers (Teff vs. Random for HP is the exception). Teff distributions of the ratios of cross-reactive hits are not different between databases. Some of the overlap between HP and HM and HG might be due to co-opting of HG cross-reactivity by human pathogens to escape immune response. Cytoscape-based TCR-epitope network patterns for the HCV Treg epitope and influenza Teff epitope are shown in .
Figure 2. TCR-Epitope Networks (developed using Cytoscape) for regulatory T cell epitopes (A) and effector T cell epitopes (B). Epitopes with TCR-facing residues similar to each of the test epitopes were identified in protein sequences from the human genome (HG; left), human microbiome (HM; center), and human viral and bacterial genomes (HP; right) databases. Green diamonds represent source peptides; gray squares are predicted nine-mer epitopes derived from the source peptide (predicted using EpiMatrix); blue triangles are nine-mers that are 100% identical to the TCR face of the source epitope and that are predicted to bind to the identical HLA; and light purple circles are proteins containing the cross-reactive epitope.
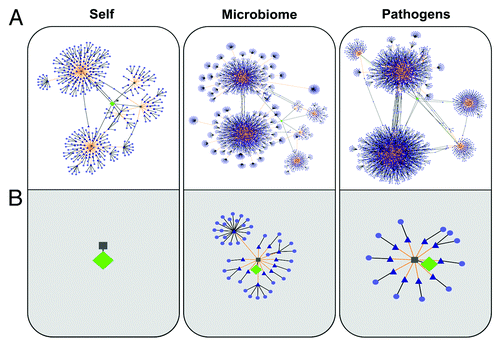
Illustration of JanusMatrix patterns
Example 1. “Positive control” T cell epitopes ().
Table 2. JanusMatrix analysis of the CEFT peptide pool
We had previously evaluated these 15 CEFT epitopes for MHC binding affinity, using EpiMatrix and found that their reported MHC restriction was highly correlated with EpiMatrix predictions, and that most epitopes were also predicted to bind to a number of additional MHC class II alleles with high affinity.Citation39 Although the median number of cross-reactive hits between these T cell epitopes and the HM (Median=29, SD=68.03) is different from the median number of hits with the HG (Median = 2, SD = 5.96), no significant difference is found when the ratios of cross-reactive hits are compared (p = 0.1; ). However, the HLA allele for which the predicted nine-mer ligand with the highest number of cross-reactive hits with HG, HM, and HP is not the same allele for which immunogenic response to CEFT 09 is reported. This may suggest that the peptide contained within the larger epitope induces a null (tolerant) or Treg response for individuals possessing the predicted cross-reactive HLA.
Example 2. Viral HP
We recently mapped T cell epitopes for EV71, the enterovirus that has been responsible for several outbreaks of HFMD in Asia.Citation41 We found that extensive cross-reactivity with HM seemed to be associated with immunodominance (). Shown in the figure are the T cell epitopes that were tested in HFMD patients. One of the two immunodominant epitopes (NTAYIIALAAAQKNFTMKL) was highly cross-conserved with enteroviruses such as polio virus (not shown) and with the human microbiome and pathogen sequences (). As is described in greater detail in the original paper, cross-reactivity between EV71 and poliovirus, appeared to be linked to protection from severe HFMD and thus may be an example of beneficial cross-reactive T cell responses.
Figure 3. Hand-Foot-Mouth Disease (HFMD) epitope conservation in human microbiome/human pathogen genome sequence and immunodominance. The figure shows the potential importance of cross-reactivity between microbial genomes to the immunodominance of a particular epitope. In HFMD, extensive cross-reactivity with the HM seems to predict immunodominance. Y axis: number of cross-reactive hits in the database; x axis, individual epitopes (and nine-mers within those epitopes).
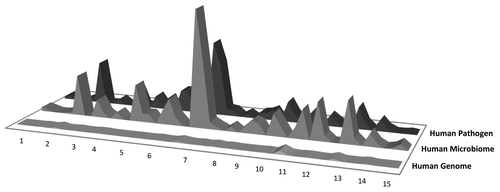
Example 3. Human Treg epitopes from Hepatitis C
For a set of Treg epitopes that were previously defined in HCV disease, HG cross-reactivity appears to be much more extensive than HM. Overall, greater cross-reactivity with HG seems to distinguish published Treg and Teff epitopes from the same viral pathogen ().
Figure 4.Hepatitis C virus (HCV) epitope conservation in human microbiome/human pathogen genome sequence and immunodominance. For regulatory T cell epitopes defined in HCV disease, HG cross-reactivity is more extensive. Overall, greater cross-reactivity with HG, HM, and HP seems to distinguish published Treg and T effector epitopes. The exact parameters defining “greater” and “lesser” cross reactivity remain to be defined following the evaluation of a number of well-defined Treg and T effector epitope examples. Y axis: number of cross-reactive hits in the database; x axis, individual epitopes (and nine-mers within those epitopes).
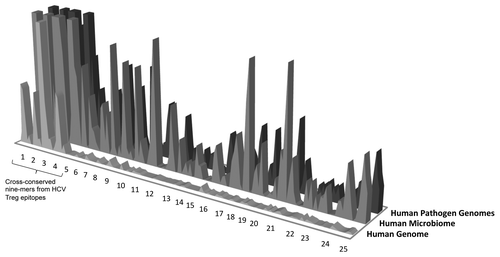
Discussion
The future: two-faced epitopes
Using the newly developed algorithm JanusMatrix, we have begun to expand the information available on the role of T cell epitopes conserved between the HM, HG, and HP, and to understand how these epitopes may influence the immune response. We are applying the tool to examine whether exposure to human commensals alters subsequent T cell responses to common human pathogens and to re-examine prevailing paradigms related to autoreactive T cells. This approach, in which we define a T cell epitope based on a well-established T cell epitope-mapping algorithm,Citation42,Citation43 is significantly different from previous analyses.Citation44 JanusMatrix compares putative T cell epitopes and their TCR-facing residues across genome sequences rather than linear peptide fragments.
JanusMatrix may enable the definition of microbial T cell epitopes that contribute to host-microbial homeostasis. As disruption of microbial homeostasis may contribute to autoimmunity, and other pathological processes, the availability of the JanusMatrix tool could significantly advance the understanding of and treatment for autoimmune and other disorders. The extensive cross-reactivity of TCR-facing residues across genomic sequences and the distinct patterns of cross-reactivity associated with T cell phenotype may have important implications for the development of vaccines and for vaccine safety and efficacy, as T cells activated by cross-reactive HM epitopes can exhibit differential ability(ies) to respond to vaccine antigens.
Cross-reactive T cells such as those described for HFMD and other enteroviruses may enhance immune responses following exposure to a new pathogen or to a vaccine containing the conserved T cell epitope. In contrast, cross-reactivity between pathogens (or commensals) might redirect the immune response towards suboptimal epitopes or enhance cytokine responses to vaccine antigens, contributing to vaccine-related adverse events. T cells activated by vaccination can also have cross-reactivity with self-antigens.Citation45,Citation46 This can lead to the breakdown of tolerance mechanisms and subsequent autoimmune reactions. Autoimmune reactions such as myocarditis, Guillain-Barré syndrome, and vasculitis have been described after administration of several vaccines,Citation47-Citation52 but their cause is not fully understood. The JanusMatrix tool may be particularly valuable for future explorations of immune-mediated adverse responses to vaccination.
JanusMatrix may also be useful for defining homologous T cell epitopes among variant strains or serotypes of the same pathogen. For example, use of these defined rules to search for conserved TCR-facing residues in large Dengue sequence databases may contribute to understanding the immunopathogenesis of Dengue Hemmorhagic Fever, a severe manifestation of Dengue infection. In addition, the use of the tool may uncover important similarities between HIV and the human genome, that have long been surmised but not yet validated.
Aspects of JanusMatrix analysis that may lead to inaccurate predictions
The current analysis relies on the accuracy of the EpiMatrix epitope prediction tool, as well as the careful curation of protein sequences in each of the analyzed databases. While each of these sources is most certainly subject to some error, the large deviations in the frequencies (see ) that we are observing seem to suggest that cross-reactivity at the TCR level may indeed influence T cell phenotype. We also note that the sheer volume of genomic data that is available in the HP and HM databases, compared to that of the HG may obscure important pattern differences, and that redundancies in epitopes between pathogens may further obscure differences; future iterations of this tool will allow a slight discount or ‘weighting’ of redundant epitopes. We also plan to normalize the comparisons of cross-reactive hits for each genome by defining the median number of cross-reactive hits for 1 million random nine-mer sequences. This would create a norm and standard deviations against which all other epitopes could be compared, and also make it possible to report deviations from the norm for each genome as a Z-score.
Population vs. individual specificity
We also note that the JanusMatrix predictions are performed at the population-wide level and are not based on any individual HLA type. Even if the general observation of the relationship between cross-reactivity and T cell phenotype holds true, these findings may vary from individual to individual based on their HLA, their history of exposures to vaccines and human pathogens, and their gut microbiome.
Furthermore, it is likely that cross-reactive immune responses are due to a diverse set of TCR-peptide-MHC interactions. Rather than being defined by a single cross-reactive T cell clone, it is likely that many different T cells, each with a unique TCR, respond to cross-reactive epitopes. Each clonal TCR that interacts with a set of peptide-MHC complexes (pMHC) may respond with a different set of effector functions. Consequently, specific patterns of cross-reactive immunity may occur only in certain people due to individual variation in the pMHC-specific T cell repertoire (“private specificity”).Citation53 Nonetheless, detailed understanding of these cross-reactive immune responses to prior exposures, and the relatedness of these responses to HLA, may lead to better vaccine designs and significant improvements in vaccine efficacy.
The future
Due to advances in computational power, the availability of vast genome sequences from commensals and pathogens to which humans are exposed, and the development of algorithms such as JanusMatrix, it is now possible to begin exploring the HM-HG and HP-HG intersections at the level of the T cell epitope. No comprehensive effort to uncover the conservation of HM epitopes across different pathogens and self proteins has been published to date, nor has the available literature defined a set of generalizable principles underlying T cell cross-reactivity that would permit predictions to be experimentally tested in a prospective manner. We expect that the exploration of the two-faced T cell epitope using JanusMatrix will significantly advance human immunology in this respect.
Methods
JanusMatrix tool development
As implied by its name,a JanusMatrix examines predicted T cell epitopes from both the HLA-binding and TCR-facing perspectives, identifying as potentially cross-reactive those that are predicted to bind the same HLA (though perhaps with different amino acid composition on the HLA-facing side) while presenting the same amino acids to the TCR (). Given a starting protein sequence, putative T cell epitopes are first identified using the EpiMatrix epitope-prediction tool. The JanusMatrix tool parses the epitopes into nine-mer frames and divides each nine-mer into T cell receptor-facing residues and MHC-binding residues, based on defined rules from the literature.Citation54 Specifically, for Class I: TCR facing residues are considered to be residues 4, 5, 6, 7, 8, and for Class II: 2, 3, 5, 7, 8. JanusMatrix then searches for potentially cross-reactive TCR-facing epitopes across any number of large sequence databases that have been pre-loaded into the tool, including the HM, the HG, and HP. JanusMatrix focuses in 9-mer searches because although peptides of different lengths interact with the MHC, most T cell epitopes can be mapped to a minimum of nine or 10 amino acids in any given peptide, even if the peptide is longer.Citation55,Citation56 Future versions of the algorithm will include 10-mer as well as 9-mer searches.
Sequence databases
We have integrated available sequence datasets into our JanusMatrix toolkit so as to uncover potentially cross-reactive epitopes between self and microbes, including the HG,Citation57 HM,Citation58 and bacterialCitation59 and viralCitation60 proteomes that we have compiled into one HP database. The number of genomes, proteins, and amino acids per database was determined. In addition, we have access to unpublished databases comprising “frequently expressed proteins” of the HM, courtesy of Sztein and VerBerkmoes (method described in ref. Citation34). The HM database of expressed peptide sequences was created from the most extensive healthy human-microbial distal gut metaproteome available to date.Citation33 In order to obtain the metaproteome, cells are enriched from fecal samples via differential centrifugation. The samples are then enriched in microbial cells and thus had a higher abundance of microbial proteins while still containing large amounts of interacting human proteins. The mixed human-microbial proteome was lysed, denatured, and reduced with 6M Guanidine/10mM DTT, and subsequently digested into peptides with sequencing grade trypsin (Promega). The complex peptide mixture was analyzed via shotgun proteomics on a 2d-LC-MS/MS system (Linear Ion trap Orbitrap). The resultant MS/MS spectra were searched against a combined human (human ref seq) and microbial isolates database (JGI-IMG HM database). Proteins were computationally assembled from identified peptides (strictly filtered at ~1% false positive rate). The entire dataset from both subjects was extracted for all identified peptides and proteins and was provided as an annotated database for upload into Janus Matrix.
Computational methods
JanusMatrix builds upon the EpiMatrix epitope predictor, processing each genome to identify TCR-facing cross-conserved HLA-binding nine-mers.Citation37,Citation41,Citation43 In addition to identifying cross-reactive epitopes and describing these in tabular format, we have found it useful to visualize the patterns with “epitope networks” using Cytoscape. An epitope network has nodes for the epitopes and for the proteins that contain them, with edges between cross-reactive pairs of epitopes and between the proteins and their constituent epitopes. We have incorporated CytoscapeCitation61 into the JanusMatrix toolkit so as to interactively visualize relatedness between selected T cell epitopes and target genomes (HG, HM, HP) and to describe the resulting epitope networks. In the examples provided in Results, we used JanusMatrix, EpiMatrix v1.2,Citation62 and the genome databases described above. Ratios of the cross-reactive hits per amino acids in the comparison database (HG, HM, or HP) were calculated for comparison purposes. Since the distributions of the ratios of cross-reactive hits were not normally distributed, non-parametric tests (Wilcoxon-Mann-Whitney test and Wilcoxon rank sum test) were used to evaluate differences in the distributions of ratios of cross-reactive hits between databases. Two type of comparisons were performed: (1) Comparisons between ratios across types of epitopes (Treg vs. Teff, Teff vs. Random, and Treg vs. Random) by database, and (2) comparisons between ratios across databases (HG vs. HM, HG vs. HP, and HM vs. HP) by type of epitope. All the analyses were performed with a 95% confidence level.
Disclosure of potential conflicts of interest
A.D.G. and W.D.M. are senior officers and majority shareholders at EpiVax, Inc., a privately owned immunoinformatics and vaccine design company located in Providence, RI, USA. L.M. and F.T. are employees at EpiVax, in which L.M. holds stock options. These authors acknowledge that there is a potential conflict of interest related to their relationship with EpiVax and attest that the work contained in this research report is free of any bias that might be associated with the commercial goals of the company.
Acknowledgments
Funding to support the discussions leading to the development of the JanusMatrix tool can be attributed to the NIH U19 grant (AI082642) (to A.D.G.) and the generation of healthy human-microbial distal gut metaproteome data was supported by grants R01-AI036525 and U19 AI082655 (to M.B.S). We are grateful to Lauren Levitz and Ryan Tassone for a close reading of the draft manuscript. The content is solely the responsibility of the authors and does not necessarily represent the official views of the National Institute of Allergy and Infectious Diseases or the National Institutes of Health.
Endnote
a In Roman mythology, Janus is “god of beginnings and transitions”, of gates, doors, doorways, endings and time. He is usually portrayed as a two-faced god since he looks to the future and the past.
References
- Wooldridge L, Ekeruche-Makinde J, van den Berg HA, Skowera A, Miles JJ, Tan MP, et al. A single autoimmune T cell receptor recognizes more than a million different peptides. J Biol Chem 2012; 287:1168 - 77; http://dx.doi.org/10.1074/jbc.M111.289488; PMID: 22102287
- Kersh GJ, Allen PM. Structural basis for T cell recognition of altered peptide ligands: a single T cell receptor can productively recognize a large continuum of related ligands. J Exp Med 1996; 184:1259 - 68; http://dx.doi.org/10.1084/jem.184.4.1259; PMID: 8879197
- Donermeyer DL, Weber KS, Kranz DM, Allen PM. The study of high-affinity TCRs reveals duality in T cell recognition of antigen: specificity and degeneracy. J Immunol 2006; 177:6911 - 9; PMID: 17082606
- Rudolph MG, Stanfield RL, Wilson IA. How TCRs bind MHCs, peptides, and coreceptors. Annu Rev Immunol 2006; 24:419 - 66; http://dx.doi.org/10.1146/annurev.immunol.23.021704.115658; PMID: 16551255
- De Groot AS, Bosma A, Chinai N, Frost J, Jesdale BM, Gonzalez MA, et al. From genome to vaccine: in silico predictions, ex vivo verification. Vaccine 2001; 19:4385 - 95; http://dx.doi.org/10.1016/S0264-410X(01)00145-1; PMID: 11483263
- Mason D. A very high level of crossreactivity is an essential feature of the T-cell receptor. Immunol Today 1998; 19:395 - 404; http://dx.doi.org/10.1016/S0167-5699(98)01299-7; PMID: 9745202
- Colf LA, Bankovich AJ, Hanick NA, Bowerman NA, Jones LL, Kranz DM, et al. How a single T cell receptor recognizes both self and foreign MHC. Cell 2007; 129:135 - 46; http://dx.doi.org/10.1016/j.cell.2007.01.048; PMID: 17418792
- Deshmukh US, Sim DL, Dai C, Kannapell CJ, Gaskin F, Rajagopalan G, et al. HLA-DR3 restricted T cell epitope mimicry in induction of autoimmune response to lupus-associated antigen SmD. J Autoimmun 2011; 37:254 - 62; http://dx.doi.org/10.1016/j.jaut.2011.07.002; PMID: 21868195
- Borbulevych OY, Piepenbrink KH, Baker BM. Conformational melding permits a conserved binding geometry in TCR recognition of foreign and self molecular mimics. J Immunol 2011; 186:2950 - 8; http://dx.doi.org/10.4049/jimmunol.1003150; PMID: 21282516
- Honeyman MC, Stone NL, Falk BA, Nepom G, Harrison LC. Evidence for molecular mimicry between human T cell epitopes in rotavirus and pancreatic islet autoantigens. J Immunol 2010; 184:2204 - 10; http://dx.doi.org/10.4049/jimmunol.0900709; PMID: 20083660
- Clute SC, Watkin LB, Cornberg M, Naumov YN, Sullivan JL, Luzuriaga K, et al. Cross-reactive influenza virus-specific CD8+ T cells contribute to lymphoproliferation in Epstein-Barr virus-associated infectious mononucleosis. J Clin Invest 2005; 115:3602 - 12; http://dx.doi.org/10.1172/JCI25078; PMID: 16308574
- Bashyam HS, Green S, Rothman AL. Dengue virus-reactive CD8+ T cells display quantitative and qualitative differences in their response to variant epitopes of heterologous viral serotypes. J Immunol 2006; 176:2817 - 24; PMID: 16493038
- Chen HD, Fraire AE, Joris I, Brehm MA, Welsh RM, Selin LK. Memory CD8+ T cells in heterologous antiviral immunity and immunopathology in the lung. Nat Immunol 2001; 2:1067 - 76; http://dx.doi.org/10.1038/ni727; PMID: 11668342
- Welsh RM, Fujinami RS. Pathogenic epitopes, heterologous immunity and vaccine design. Nat Rev Microbiol 2007; 5:555 - 63; http://dx.doi.org/10.1038/nrmicro1709; PMID: 17558423
- Selin LK, Wlodarczyk MF, Kraft AR, Nie S, Kenney LL, Puzone R, et al. Heterologous immunity: immunopathology, autoimmunity and protection during viral infections. Autoimmunity 2011; 44:328 - 47; http://dx.doi.org/10.3109/08916934.2011.523277; PMID: 21250837
- Welsh RM, Che JW, Brehm MA, Selin LK. Heterologous immunity between viruses. Immunol Rev 2010; 235:244 - 66; PMID: 20536568
- Page KR, Scott AL, Manabe YC. The expanding realm of heterologous immunity: friend or foe?. Cell Microbiol 2006; 8:185 - 96; http://dx.doi.org/10.1111/j.1462-5822.2005.00653.x; PMID: 16441430
- Santiago HC, LeeVan E, Bennuru S, Ribeiro-Gomes F, Mueller E, Wilson M, et al. Molecular mimicry between cockroach and helminth glutathione S-transferases promotes cross-reactivity and cross-sensitization. J Allergy Clin Immunol 2012; 130:248-56.e9.
- Mongkolsapaya J, Dejnirattisai W, Xu XN, Vasanawathana S, Tangthawornchaikul N, Chairunsri A, et al. Original antigenic sin and apoptosis in the pathogenesis of dengue hemorrhagic fever. Nat Med 2003; 9:921 - 7; http://dx.doi.org/10.1038/nm887; PMID: 12808447
- Mongkolsapaya J, Duangchinda T, Dejnirattisai W, Vasanawathana S, Avirutnan P, Jairungsri A, et al. T cell responses in dengue hemorrhagic fever: are cross-reactive T cells suboptimal?. J Immunol 2006; 176:3821 - 9; PMID: 16517753
- Nie S, Lin SJ, Kim SK, Welsh RM, Selin LK. Pathological features of heterologous immunity are regulated by the private specificities of the immune repertoire. Am J Pathol 2010; 176:2107 - 12; http://dx.doi.org/10.2353/ajpath.2010.090656; PMID: 20348239
- de St Groth BF. Regulatory T-cell abnormalities and the global epidemic of immuno-inflammatory disease. Immunol Cell Biol 2012; 90:256 - 9; http://dx.doi.org/10.1038/icb.2011.113; PMID: 22310378
- Berer K, Mues M, Koutrolos M, Rasbi ZA, Boziki M, Johner C, et al. Commensal microbiota and myelin autoantigen cooperate to trigger autoimmune demyelination. Nature 2011; 479:538 - 41; http://dx.doi.org/10.1038/nature10554; PMID: 22031325
- Ng SC, Benjamin JL, McCarthy NE, Hedin CR, Koutsoumpas A, Plamondon S, et al. Relationship between human intestinal dendritic cells, gut microbiota, and disease activity in Crohn’s disease. Inflamm Bowel Dis 2011; 17:2027 - 37; http://dx.doi.org/10.1002/ibd.21590; PMID: 21910165
- Erickson AR, Cantarel BL, Lamendella R, Darzi Y, Mongodin EF, Pan C, et al. Integrated metagenomics/metaproteomics reveals human host-microbiota signatures of Crohn’s disease. PLoS One 2012; 7:e49138; http://dx.doi.org/10.1371/journal.pone.0049138; PMID: 23209564
- McLoughlin RM, Mills KH. Influence of gastrointestinal commensal bacteria on the immune responses that mediate allergy and asthma. J Allergy Clin Immunol 2011; 127:1097 - 107, quiz 1108-9; http://dx.doi.org/10.1016/j.jaci.2011.02.012; PMID: 21420159
- Gaboriau-Routhiau V, Lécuyer E, Cerf-Bensussan N. Role of microbiota in postnatal maturation of intestinal T-cell responses. Curr Opin Gastroenterol 2011; 27:502 - 8; http://dx.doi.org/10.1097/MOG.0b013e32834bb82b; PMID: 21946030
- Pils MC, Bleich A, Prinz I, Fasnacht N, Bollati-Fogolin M, Schippers A, et al. Commensal gut flora reduces susceptibility to experimentally induced colitis via T-cell-derived interleukin-10. Inflamm Bowel Dis 2011; 17:2038 - 46; http://dx.doi.org/10.1002/ibd.21587; PMID: 21182023
- Dahlqvist G, Piessevaux H. Irritable bowel syndrome: the role of the intestinal microbiota, pathogenesis and therapeutic targets. Acta Gastroenterol Belg 2011; 74:375 - 80; PMID: 22103040
- Chung H, Pamp SJ, Hill JA, Surana NK, Edelman SM, Troy EB, et al. Gut immune maturation depends on colonization with a host-specific microbiota. Cell 2012; 149:1578 - 93; http://dx.doi.org/10.1016/j.cell.2012.04.037; PMID: 22726443
- Lee YK, Mazmanian SK. Has the microbiota played a critical role in the evolution of the adaptive immune system?. Science 2010; 330:1768 - 73; http://dx.doi.org/10.1126/science.1195568; PMID: 21205662
- Lathrop SK, Bloom SM, Rao SM, Nutsch K, Lio CW, Santacruz N, et al. Peripheral education of the immune system by colonic commensal microbiota. Nature 2011; 478:250 - 4; http://dx.doi.org/10.1038/nature10434; PMID: 21937990
- Verberkmoes NC, Russell AL, Shah M, Godzik A, Rosenquist M, Halfvarson J, et al. Shotgun metaproteomics of the human distal gut microbiota. ISME J 2009; 3:179 - 89; http://dx.doi.org/10.1038/ismej.2008.108; PMID: 18971961
- Lee LH, Corrier K, Dill B, Shah M, Hettich R, Jansson J, et al. Advanced deep metaproteomics methods provide unique insights into the diversity of the human proteome and gut microbiota in healthy adults. In: 61st American Society for Mass Spectrometry (ASMS) Conference, June 9-13, 2013, Minneapolis, MN.
- Losikoff PT, Self AA, Gregory SH. Dendritic cells, regulatory T cells and the pathogenesis of chronic hepatitis C. Virulence 2012; 3:610 - 20; http://dx.doi.org/10.4161/viru.21823; PMID: 23076334
- Self AA, Losikoff PT, Gregory SH, Regulatory T. Cells Promote the Pathogenesis of Chronic Hepatitis C. Hum Vaccin Immunother Manuscript submitted
- Li S, Floess S, Hamann A, Gaudieri S, Lucas A, Hellard M, et al. Analysis of FOXP3+ regulatory T cells that display apparent viral antigen specificity during chronic hepatitis C virus infection. PLoS Pathog 2009; 5:e1000707; http://dx.doi.org/10.1371/journal.ppat.1000707; PMID: 20041222
- Newell EW, Klein LO, Yu W, Davis MM. Simultaneous detection of many T-cell specificities using combinatorial tetramer staining. Nat Methods 2009; 6:497 - 9; http://dx.doi.org/10.1038/nmeth.1344; PMID: 19543286
- Weber CA, Mehta PJ, Ardito M, Moise L, Martin B, De Groot AS. T cell epitope: friend or foe? Immunogenicity of biologics in context. Adv Drug Deliv Rev 2009; 61:965 - 76; http://dx.doi.org/10.1016/j.addr.2009.07.001; PMID: 19619593
- De Groot AS, Moise L, McMurry JA, Wambre E, Van Overtvelt L, Moingeon P, et al. Activation of natural regulatory T cells by IgG Fc-derived peptide “Tregitopes”. Blood 2008; 112:3303 - 11; http://dx.doi.org/10.1182/blood-2008-02-138073; PMID: 18660382
- Wei R, Yang C, Zeng M, Terry F, Zhu K, Yang C, et al. A dominant EV71-specific CD4+ T cell epitope is highly conserved among human enteroviruses. PLoS One 2012; 7:e51957; http://dx.doi.org/10.1371/journal.pone.0051957; PMID: 23251663
- Moss SF, Moise L, Lee DS, Kim W, Zhang S, Lee J, et al. HelicoVax: Epitope-based therapeutic H. pylori vaccination in a mouse model of gastric cancer. Vaccine 2011; 29:2085 - 91; http://dx.doi.org/10.1016/j.vaccine.2010.12.130; PMID: 21236233
- Schanen BC, De Groot AS, Moise L, Ardito M, McClaine E, Martin WB, et al. Coupling sensitive in vitro and in silico techniques to assess cross-reactive CD4(+) T cells against the swine-origin H1N1 influenza virus. Vaccine 2011; 29:3299 - 309; http://dx.doi.org/10.1016/j.vaccine.2011.02.019; PMID: 21349362
- Trost B, Kusalik A, Lucchese G, Kanduc D. Bacterial peptides are intensively present throughout the human proteome. Self Nonself 2010; 1:71 - 4; http://dx.doi.org/10.4161/self.1.1.9588; PMID: 21559180
- Rist M, Smith C, Bell MJ, Burrows SR, Khanna R. Cross-recognition of HLA DR4 alloantigen by virus-specific CD8+ T cells: a new paradigm for self-/nonself-recognition. Blood 2009; 114:2244 - 53; http://dx.doi.org/10.1182/blood-2009-05-222596; PMID: 19617574
- Takahashi Y, Matsuda K, Kubota Y, Shimomura J, Yamasaki E, Kudo T, et al. Vaccination and infection as causative factors in Japanese patients with Rasmussen syndrome: molecular mimicry and HLA class I. Clin Dev Immunol 2006; 13:381 - 7; http://dx.doi.org/10.1080/17402520600589522; PMID: 17162382
- de Carvalho JF, Shoenfeld Y. Status epilepticus and lymphocytic pneumonitis following hepatitis B vaccination. Eur J Intern Med 2008; 19:383 - 5; http://dx.doi.org/10.1016/j.ejim.2007.10.005; PMID: 18549949
- Israeli E, Agmon-Levin N, Blank M, Chapman J, Shoenfeld Y. Guillain-Barré syndrome--a classical autoimmune disease triggered by infection or vaccination. Clin Rev Allergy Immunol 2012; 42:121 - 30; http://dx.doi.org/10.1007/s12016-010-8213-3; PMID: 20890797
- Kennedy JS, Co M, Green S, Longtine K, Longtine J, O’Neill MA, et al. The safety and tolerability of an HIV-1 DNA prime-protein boost vaccine (DP6-001) in healthy adult volunteers. Vaccine 2008; 26:4420 - 4; http://dx.doi.org/10.1016/j.vaccine.2008.05.090; PMID: 18588934
- Morgan J, Roper MH, Sperling L, Schieber RA, Heffelfinger JD, Casey CG, et al. Myocarditis, pericarditis, and dilated cardiomyopathy after smallpox vaccination among civilians in the United States, January-October 2003. Clin Infect Dis 2008; 46:Suppl 3 S242 - 50; http://dx.doi.org/10.1086/524747; PMID: 18284365
- Orbach H, Agmon-Levin N, Zandman-Goddard G. Vaccines and autoimmune diseases of the adult. Discov Med 2010; 9:90 - 7; PMID: 20193633
- Souayah N, Michas-Martin PA, Nasar A, Krivitskaya N, Yacoub HA, Khan H, et al. Guillain-Barré syndrome after Gardasil vaccination: data from Vaccine Adverse Event Reporting System 2006-2009. Vaccine 2011; 29:886 - 9; http://dx.doi.org/10.1016/j.vaccine.2010.09.020; PMID: 20869467
- Kim SK, Cornberg M, Wang XZ, Chen HD, Selin LK, Welsh RM. Private specificities of CD8 T cell responses control patterns of heterologous immunity. J Exp Med 2005; 201:523 - 33; http://dx.doi.org/10.1084/jem.20041337; PMID: 15710651
- Rudolph MG, Stanfield RL, Wilson IA. How TCRs bind MHCs, peptides, and coreceptors. Annu Rev Immunol 2006; 24:419 - 66; http://dx.doi.org/10.1146/annurev.immunol.23.021704.115658; PMID: 16551255
- Rammensee HG. Chemistry of peptides associated with MHC class I and class II molecules. Curr Opin Immunol 1995; 7:85 - 96; http://dx.doi.org/10.1016/0952-7915(95)80033-6; PMID: 7772286
- Madden DR. The three-dimensional structure of peptide-MHC complexes. Annu Rev Immunol 1995; 13:587 - 622; http://dx.doi.org/10.1146/annurev.iy.13.040195.003103; PMID: 7612235
- UniProt Consortium. Reorganizing the protein space at the Universal Protein Resource (UniProt). Nucleic Acids Res 2012; 40:Database issue D71 - 5; http://dx.doi.org/10.1093/nar/gkr981; PMID: 22102590
- Gevers D, Knight R, Petrosino JF, Huang K, McGuire AL, Birren BW, et al. The Human Microbiome Project: a community resource for the healthy human microbiome. PLoS Biol 2012; 10:e1001377; http://dx.doi.org/10.1371/journal.pbio.1001377; PMID: 22904687
- Calis JJ, de Boer RJ, Keşmir C. Degenerate T-cell recognition of peptides on MHC molecules creates large holes in the T-cell repertoire. PLoS Comput Biol 2012; 8:e1002412; http://dx.doi.org/10.1371/journal.pcbi.1002412; PMID: 22396638
- Hertz T, Nolan D, James I, John M, Gaudieri S, Phillips E, et al. Mapping the landscape of host-pathogen coevolution: HLA class I binding and its relationship with evolutionary conservation in human and viral proteins. J Virol 2011; 85:1310 - 21; http://dx.doi.org/10.1128/JVI.01966-10; PMID: 21084470
- Shannon P, Markiel A, Ozier O, Baliga NS, Wang JT, Ramage D, et al. Cytoscape: a software environment for integrated models of biomolecular interaction networks. Genome Res 2003; 13:2498 - 504; http://dx.doi.org/10.1101/gr.1239303; PMID: 14597658
- De Groot AS, Martin W. Reducing risk, improving outcomes: bioengineering less immunogenic protein therapeutics. Clin Immunol 2009; 131:189 - 201; http://dx.doi.org/10.1016/j.clim.2009.01.009; PMID: 19269256