Abstract
Background
Inflammation plays a pivotal role in the pathogenesis of heart failure (HF). This study was aimed to the potential association between complete blood cell count (CBC)-derived inflammatory biomarkers and HF.
Methods
Data from the National Health and Nutrition Examination Survey (NHANES) 2009-2018 were utilised. We evaluated the associations between HF and five systemic inflammation markers derived from CBC: systemic immune-inflammation index (SII), systemic inflammatory response index (SIRI), neutrophil-to-lymphocyte ratio (NLR), platelet-to-lymphocyte ratio (PLR), and monocyte-to-lymphocyte ratio (MLR). Demographic characteristics, physical examinations, and laboratory data were systematically collected for comparative analysis between HF and non-HF individuals. Fitted smoothing curves and threshold effect analysis delineated the relationship. In addition, Spearman correlation and subgroup analyses were further conducted.
Results
A total of 26,021 participants were categorised into HF (n = 858) and non-HF (n = 25,163) groups. After adjusting for confounding variables, SIRI, NLR, and MLR had significant positive correlations with the risk of HF. Participants in the highest quarter groups of SIRI, NLR, and MLR showed a increased risk of developing HF compared to those in the lowest quarter group. Furthermore, subgroup and sensitivity analyses indicated that SIRI, NLR, and MLR had a stronger correlation to HF (all p < 0.05). Smoothing curve fitting highlighted a nonlinear relationship between CBC-derived inflammatory biomarkers and HF.
Conclusions
Our results illustrated a significant association between elevated levels of SIRI, NLR, and MLR and an increased risk of HF. SIRI, NLR, and MLR could potentially serve as systemic inflammation hazard markers for HF.
1. Introduction
Heart failure (HF) is a complex clinical syndrome most commonly observed in the terminal stage of various cardiovascular diseases (CVDs). It is mainly characterised by clinical manifestations such as breathlessness, fluid retention, fatigue, and diminished activity tolerance, resulting from structural and functional abnormalities of the heart [Citation1,Citation2]. In the United States, approximately 6.5 million adults suffer from HF, with nearly 1 million newly cases are diagnosed each year, highlighting its increasing prevalence rate. Globally, the number of individuals afflicted by HF is estimated at 64.3 million [Citation3,Citation4]. Although advanced therapies in treatments and devices for HF including neurohormonal blockade, and implantable defibrillators have substantially improved the survival and quality of life in patients over the past decades, it remains a widespread disease with significant morbidity and mortality rates, with only a 25% survival rate over ten yeas [Citation5,Citation6]. Given the high prevalence and cost of HF, there is an increasing focus on uncovering its underlying factors. A deeper understanding of risk factors and their interconnection with HF is crucial for developing new preventive strategies.
Although the specific pathogenesis of HF remains unclear, it appears that abnormal immune activation and chronic inflammation are essential to its progression [Citation7,Citation8]. Myocardial damage, repair, and remodelling are the important links in the occurrence and development of HF, in which immune/inflammatory cells (neutrophils, monocytes, lymphocytes, etc.) and the inflammatory factors (tumor necrosis factor, interleukin-6, etc.) released by them are involved [Citation9]. Inflammatory cells and their functional inflammatory factors can induce cardiomyocyte hypertrophy, apoptosis, and fibrosis, resulting in adverse cardiac remodelling and progressive left ventricular dysfunction [Citation10]. Clinically, white blood cell counts and subset counts from blood routine tests are used as surrogates for systemic inflammation. Numerous inflammation markers have been reported to correlate with an increased risk of cardiovascular disease, enhancing predictive power for cardiovascular events [Citation11]. Leukocyte is a vital component of the immune system, and denote a class of cells capable of eliciting specific immune reactions. Neutrophils, the most populous type of white blood cells, play a pivotal role in initiating innate immune responses, deeply involved in acute injury and repair, chronic inflammatory processes, cancer, and autoimmunity [Citation12]. Monocytes are part of the immune system and can migrate to tissues where they differentiate into macrophages or dendritic cells. Monocytes are closely associated with inflammation as they increase in number during infections or inflammatory conditions and participate in clearing damaged cells and pathogens, thereby alleviating inflammatory responses [Citation13]. Lymphocytes are indicators of allergic inflammation regulators. They are also instrumental in mediating both cellular and humoral immunity [Citation14]. Platelets, commonly associated with clotting, also have significant roles in mediating immune and inflammatory responses [Citation15]. The interactions of immune and inflammatory cells are responsible for immune surveillance, immune defense, and immune homeostasis, which have significant implications for human health. The research for biomarkers to evaluate inflammation has garnered increasing attentions, with new complete blood count (CBC)-derived inflammatory biomarkers inflammatory biomarkers, including but not limited to systemic immune-inflammation index (SII), systemic inflammatory response index (SIRI), neutrophil-to-lymphocyte ratio (NLR), platelet-to-lymphocyte ratio (PLR), and monocyte-to-lymphocyte ratio (MLR) being focused. Evidence has shown that platelet, PLR, NLR, SIRI, and SII are associated with cardiovascular diseases [Citation16–20]. The blood inflammation indicators analysed in this study were collected from the National Health and Nutrition Examination Survey (NHANES) database, a national population-based cross-sectional survey designed and conducted by the National Centre for Health Statistics (NCHS) to collect information on potential health risk factors and nutritional status of non-institutionalized civilians in the United States (https://www.cdc.gov/nchs/nhanes/) [Citation21]. However, there is little comprehensive evidence delineating the relationships between HF and the CBC-derived inflammatory biomarkers. This study investigates the correlation between CBC-derived inflammatory biomarkers levels and the incidence of HF at a cross-sectional level, aiming to clarify the potential link between inflammation levels and the onset of HF, providing a reference for clinical prevention and guiding the treatment of HF.
2. Materials and methods
2.1. Study population and design
We sourced our data from the NHANES database. In our analysis, we aggregated data from five NHANES cycles (2009-2010, 2011-2012, 2013-2014, 2015-2016, and 2017-2018), and these cycles were all listed before exclusion. To ensure a representative sample of US civilians, a complex multistage probability sampling method was employed in the NHANES. The NHANES study protocol was approved by the NCHS Research Ethics Review Committee, and written informed consent was obtained from all survey participants. Participants completed a household interview to provide demographic, socioeconomic, and health-related information. Additionally, a physical examination and laboratory tests at a mobile examination centre (MEC) were performed to evaluate their medical and physiological status. All detailed NHANES study designs and data are publicly available at https://www.cdc.gov/nchs/nhanes/.
2.2. Inclusion and exclusion criteria
Initially, the dataset comprised 49,693 participants. However, we applied the following exclusion criteria: (1) missing data for inflammatory immune indexes (n = 8,805), (2) missing data for heart failure (n = 14,585), and (3) participants below 20 years of age or those who were pregnant (n = 282). After these considerations, a final cohort of 26,021 participants were initially recruited as depicted in .
2.3. Assessment of CBC-derived inflammatory biomarkers
A hematology-analyzing device (Coulter®DxH 800 Analyzer) was used to determine the complete blood count (CBC), which was subsequently expressed as × 103 cells/μl. Based on the obtained peripheral blood cell counts, we calculated five systemic inflammation markers: SII, SIRI, NLR, PLR, and MLR. The calculations were defined as: SII = platelet count × neutrophil count/lymphocyte count, SIRI = neutrophil counts × monocyte counts/lymphocyte counts, NLR = neutrophil counts/lymphocyte counts, PLR = platelet counts/lymphocyte counts, MLR = monocyte counts/lymphocyte counts.
2.4. Definition of Heart failure
Heart failure was defined based on a standardised medical condition questionnaire, which was administered during self- and proxy-reported personal interviews. Within the health questionnaire, participants were asked “Has a doctor or other health professional ever told you that you had congestive heart failure?”. Those responding with “yes” were classified as having HF.
2.5. Covariates
In this study, the following covariates were employed: age (years), gender (male/female), race (Mexican American/Other Hispanic/Non-Hispanic White/Non-Hispanic Black/Other race), body mass index (BMI) was classified as < 25, 25-29.9, and >29.9 kg/m2, corresponding to normal weight, overweight, and obesity, respectively [Citation22]. Educational status was divided into less than high school, high school or equivalent, and above high school. Smoking status was categorised as current smoker (>100 cigarettes/lifetime and currently smoking on certain days or every day) or never smoker (including never [<100 cigarettes/lifetime] or former smoker [100 cigarettes/lifetime and absolutely no smoking now]) [Citation23]. Alcohol use was classified as: never alcohol user (<12 drinks/lifetime), mild (1 drink/day for women or 1-2 drinks/day for men), moderate (2-3 drinks/day for women or 3-4drinks/day for men) or heavy (≥4 drinks/day for women and ≥5 drinks/day for men) [Citation24]. Hypertension was defined as either having a systolic blood pressure (SBP) of ≥140 or/and a diastolic blood pressure (DBP) of ≥90 mmHg, or having a self-reported history of hypertension [Citation25,Citation26]. Diabetes was diagnosed based on self-reported diabetes, fasting plasma glucose ≥7.0 mmol/L, 2-h OGTT ≥ 11.1 mmol/L, or haemoglobin A1c (HbA1c) level ≥6.5% [Citation27]. Hyperlipidaemia was defined by total cholesterol ≥240 mg/dL, triglycerides ≥200 mg/dL, LDL-C ≥ 160 mg/dL, and HDL-C < 40 mg/dL or a physician’s previous diagnosis [Citation28]. Poverty was assessed based on the poverty-to-income ratio (PIR) was classified as low (<1.3), middle (1.3-3.5), and high (>3.5). Sleep disturbance was assessed by the questionnaire: “Have you ever told a doctor or other health professional that you have trouble sleeping?”, with a “yes” response. A participant was recorded as having coronary heart disease or stroke if she/he answered “yes” to the following question: “Has a doctor or other health professional ever told you that you had congestive coronary heart disease/stroke?”. Cases of non-congestive coronary artery disease were not included.
2.6. Statistical analyses
As part of the NHANES complex sampling design, we utilised appropriate weights to achieve a representative sample of the US national population. The statistical study was analysed using the R software (version 4.3.1), StataMP (version 16.0), and EmpowerStats (version 4.0). First, only participants with complete data were included, and those with missing covariate data were subsequently excluded. Second, the weighted student’s t-test (continuous variables) or weighted chi-square test (categoric variables) were utilised to compare differences in baseline characteristics between non-HF and HF groups in patients. Third, the sample weight used in the final analysis was equal to one-fifth the value of “WTMEC2YR”, because we combined five NHANES survey cycles. Fourthly, the Spearman rank correlation coefficient was employed to assess the association between CBC-derived inflammatory biomarkers and baseline characteristics. Lastly, we performed weighted univariate and multivariate logistic regression to evaluate the correlation between CBC-derived inflammatory indices and HF, with 95% confidence intervals (CI) and odds ratio (OR) calculated.
Four statistical models were established: Unadjusted Model: no covariates were adjusted; Model 1: age, gender, race, education level, and PIR were adjusted; Model 2: age, gender, race, education level, PIR, BMI, sleep disturbance, alcohol use, and smoking status were adjusted; Model 3: adjusted for all covariates (age, gender, race, education level, PIR, BMI, sleep disturbance, alcohol use, smoking status, hypertension, diabetics, hyperlipidaemia, and congestive coronary heart disease/stroke). Smoothed curve fits were done simultaneously. Subgroup analysis was conducted with stratified factors, such as age, gender, race, BMI, education level, alcohol use, smoking status, sleep disturbance, stroke, diabetes, hypertension, hyperlipidaemia, coronary heart disease, and stroke, to determine the potential effect moderators. To analyse the heterogeneity of correlations among different subgroups, interaction analyses were included as well. A P-value <0.05 denoted statistical significance.
3. Results
3.1. Baseline characteristics of participants
From the NHANES 2009-2018 dataset, a total of 26,021 participants were involved, representing an estimated 217.19 million non-institutionalized U.S. civilians. Among these participants, the average age was 47.93 ± 16.99 years, with a gender distribution of 48.58% men and 51.42% women. Subsequently, participants were categorised into two groups: those with HF(n = 858) and those without (n = 25,163). Statistically significant differences between the HF and non-HF groups were observed in age, race, PIR, education level, BMI, diabetes, smoking status, sleep disturbance, alcohol use, hypertension, hyperlipidaemia, coronary heart disease, CBC count, and CBC-derived indicators (all p < 0.05). However, gender distribution remained consistent between the two groups. Compared to participants without HF, those with HF were more likely to be older, predominantly non-Hispanic White, had a higher education level, and with higher levels of BMI. Moreover, participants with HF presented with a greater incidence of comorbidities, including hypertension, stroke, diabetes, and coronary heart disease ().
Table 1. Basic characteristics of participants with CBC-derived inflammatory biomarkers in the NHANES 2009-2018.
3.2. Univariate and multivariate logistic regression models
The weighted multivariate logistic regression analysis between CBC-derived indicators and HF is delineated in . Without adjusting for covariates, SIRI, NLR, and MLR were positively associated with HF [odds ratio (OR): 1.481, 95% confidence interval (CI): 1.368-1.582, p < 0.001; 1.311, 1.239-1.386, p < 0.001; 27.684, 27.684-54.576, p < 0.001, respectively]. Conversely, SII and PLR showed only weak associations with HF (OR: 1.001, 95% CI: 1.000-1.001, p < 0.001; 1.002, 1.000-1.004, p = 0.03, respectively). Likewise, when treated as categorical variables, the higher levels of SIRI, NLR, and MLR were linked to a higher likelihood of HF, which were consistent with the trends in the continuous analyses. After controlling for of several confounding variables or fully adjusting for all covariates, SIRI, NLR, and MLR were positively correlated with HF (OR: 1.196, 1.143, and 5.185, respectively; all p < 0.001), indicating that a one-unit increase in SIRI, NLR, and MLR scores was associated with a 1.196-, 1.143-, and 5.185-fold increase in the risk of HF, respectively. In the sensitivity analysis, CBC-derived inflammatory markers were converted from a continuous variable to a categorical variable (Q1-Q4). The results showed that the associations between SIRI, NLR, and MLR with HF risk were consistent with the trends observed in the continuous analysis. Specifically, the highest quarter groups (Q4) of SIRI, NLR, and MLR had increased odds of developing HF by factors of 1.802, 1.852, and 1.928, respectively, compared to the lowest quarter group (OR: 1.802, 1.852, 1.928, respectively; all p < 0.001).
Table 2. Association between CBC-derived indicators and HF.
3.3. Subgroup analysis
Our analysis revealed significant associations between HF and CBC-derived indicators, namely SIRI, NLR, and MLR, across subgroups stratified by factors including age, gender, BMI, smoking status, alcohol use, sleep disturbance, hypertension, hyperlipidaemia, stroke, diabetes, and coronary heart disease (all p < 0.05), as summarised in . Additively, we found that SII and PLR were not consistently associated with HF in some subgroups. Meanwhile, the interaction test showed that there was no significant difference among each stratification in the association between CBC-derived indicators and HF, indicating that there was no significant dependence on age, gender, BMI, smoking status, alcohol use, sleep disturbance, hypertension, hyperlipidaemia, and coronary heart disease on this connection (all p for the interaction > 0.05). However, it is crucial to note that stroke and diabetes might influence the positive association between CBC-derived indicators and HF (all p for interaction <0.05).
Table 3. Subgroup analysis for the association between CBC-derived indicators and HF.
3.4. Nonlinear relationships between CBC-derived inflammatory biomarkers and HF
We performed a smooth curve fitting to elucidate the nonlinear relationship between CBC-derived inflammatory biomarkers and HF after adjusting all confounders, the results are shown in . Notably, the distinct CBC-derived indicators-SIRI, NLR, and MLR-stand out with their pronounced associations with HF, detailed in .
Figure 2. The Nonlinear relationship between complete blood cell count (CBC)-derived indicators and HF. (A) SII; (B) SIRI; (C) PLR; (D) NLR; (E) MLR. A smooth curve fitting between variables is represented by the solid red line. The 95% confidence interval of the fit is indicated by the blue line. The solid red line represents the smooth curve fit between variables. Blue bands represent the 95% confidence interval from the fit. The values were adjusted for all covariates.
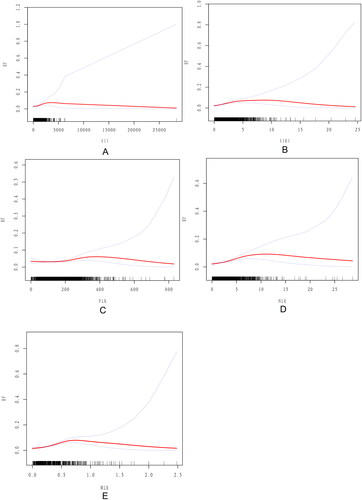
Table 4. Threshold effect and saturation effect analysis of CBC-derived indicators and HF.
3.5. Relationship between CBC-derived inflammatory biomarkers and covariates related to HF
According to Spearman rank correlation analysis, SII was positively correlated with age, gender, smoking status, alcohol use, BMI, hypertension, and diabetes (r = 0.0332, 0.0678, 0.0562, 0.0328, 0.0692, 0.0571, and 0.0512, respectively), and negatively correlated with race, sleep disturbance, and stroke (r = −0.1121, −0.0488 and −0.0430, respectively). However, a marginal negative correlation was observed with between SII and PIR (r= −0.0134). Similarly, SIRI revealed positive associations with age, smoking status, alcohol use, BMI, hypertension, and diabetes (r = 0.1418, 0.1312, 0.0533, 0.0807, 0.1239, 0.0571, and 0.0979, respectively), but negative associations with gender, PIR, race, education level, sleep disturbance, hyperlipidaemia, CHD, and stroke (r = −0.1244, −0.0276, −0.1409, −0.0315, −0.0531, −0.0503, −0.0892, and −0.0725, respectively). Additionally, NLR was positively correlated with age, smoking status, alcohol use, BMI, hypertension, and diabetes (r = 0.1366, 0.075, 0.0304, 0.028, 0.0897, 0.0571, and 0.0886, respectively), and negatively with gender, race, sleep disturbance, hyperlipidaemia, CHD, and stroke (r = −0.0556, −0.1267, −0.0438, −0.04, −0.0681 and −0.0647, respectively). Moreover, PLR was positively correlated with age, gender, PIR, race, education level, hypertension, hyperlipidaemia, and CHD (r = 0.0655, 0.082, 0.0608, 0.1571, 0.0664, 0.0128, 0.0169, and 0.0186, respectively), while was negatively correlated with smoking status, alcohol use, BMI, sleep disturbance, stroke, and diabetes (r = −0.0867, −0.0269, −0.0673, −0.0136, −0.0193 and −0.0305, respectively). Lastly, MLR was positively correlated with age, PIR, smoking status, alcohol use, hypertension, and diabetes (r = 0.228, 0.0311, 0.0552, 0.0142, 0.1304, and 0.0495, respectively), while negatively correlated with gender, race, BMI, sleep disturbance, hyperlipidaemia, CHD, and stroke, (r = −0.2129, −0.0577, −0.0311, −0.0265, −0.0721, −0.1052 and −0.0693, respectively), as detailed in .
Table 5. Spearman correlation analysis of CBC-derived inflammatory biomarkers and baseline characteristics.
4. Discussion
In our cross-sectional study of 26,021 eligible participants from NHANES, we analysed 858 individuals with HF and 25,163 without HF. We utilised various independent variables, including continuous and categorical types, to robust multiple regression models for more accurate results. After adjusting for multiple variables, we found significant associations between high levels of SIRI, NLR, and MLR and a increased risk of HF. Moreover, our analyses included adjustments for relevant covariates, allowing us to reveal potential confounding factors linked to HF. Notably, CBC-derived indicators were associated with HF after adjustment for all covariates, exhibiting a non-linear relationship. However, SII and PLR showed no association with HF. Through interaction testing based on some clinical parameters and comorbidities, we found the direction of relationships between the CBC-derived indicators and HF in different subgroups was consistent with that in the total study population. Furthermore, we observed synergisic and additive interactions between stroke and diabetes on the occurrence of HF, further lending crediblity to our findings.
HF is a complex systemic disease influenced not only by cardiac factors but also by the immune system activation and inflammation. Inflammatory responses are crucial to HF, involving the infiltration of inflammatory cells, the secretion and regulation of cytokines, and the process of myocardial extracellular matrix remodelling [Citation10,Citation29–31]. Neutrophils, the most abundant white blood cells, are related to the initiation and modulation of the immune process. In patients with HF, neutrophils can be activated and release large amounts of pro-inflammatory cytokines and oxidative stress substances. Thus, neutrophilia has been independently associated with the severity and prognosis of HF [Citation12,Citation32,Citation33]. Lymphocytes are involved in the regulation of adaptive immunity and innate immunity and possess protective or regulatory properties. Lymphocytes can regulate the inflammatory response by reducing the release of inflammatory cytokines and inhibiting the activation of neutrophils and monocytes, thereby protecting the heart from injury [Citation34–36]. Leukocytosis, mainly due to neutrophilia, is associated with HF, and strongly correlated to the pro-inflammatory roles of lymphocytes and monocytes, leading to adverse effects like myocardial remodelling or negative inotropy [Citation37]. Monocytes, crucial in atherosclerosis, are predictors of cardiovascular and all-cause mortality in cardiovascular disease patients. Circulating monocytes infiltrate the heart early after myocardial injury and participate in inflammation and repair processes, influencing left ventricular remodelling [Citation38,Citation39]. Platelets also play an important role in the development and progression of HF, with their activation and coagulation associated with an increased incidence of cardiovascular events. Activated platelets can contribute to the inflammatory response through the release of pro-inflammatory cytokines and interactions with other cell types such as neutrophils, monocytes, and endothelial cells. Meanwhile, platelets can affect neutrophil functions, which causes an exacerbation of cardiac damage by damaging surviving myocytes [Citation15,Citation40–43]. In summary, monocytes, neutrophils, lymphocytes, and platelets play different roles in either myocardial fibrosis progression, sustained systemic inflammation, endothelial injury, or impaired heart function, leading to worsening HF. However, the mechanism of the relationship between CBC-derived indicators and HF remains incompletely understood, which is potentially linked to the inflammatory response and platelet activity.
Systemic inflammation, stemming from metabolic or immunological conditions, is strongly linked to cardiovascular disease. CBC-derived indicators provide insights into the immune and chronic inflammatory states of the body, which have been studied in recent years. Among these, SIRI is a comprehensive indicator of chronic low-grade inflammation, based on neutrophils, monocytes, and lymphocytes. In particular, SIRI could better reflect the state of long-term inflammation of HF [Citation44,Citation45]. Meanwhile, MLR is associated with adverse long-term outcomes in patients with HF [Citation17]. Angkananard et al. concluded that an elevated NLR on admission in patients with acute HF was independently associated with worse cardiovascular events, HF-related rehospitalization, and in-hospital fatalities [Citation46]. Intriguingly, SIRI, as the combination of NLR and MLR, has been shown to be a potent biomarker for assessing the inflammatory status and predicting outcomes in patients with HF. Our sutdy aligns with previous research showing significant associations betwwen SIRI, NLR, and MLR with increased HF risk. In contrast, PLR’s prognostic role in the outcomes of HF is less clear, with mixed findings in the literature. However, some retrospective studies have reported that a higher PLR was associated with poor clinical outcomes in patients with acute HF [Citation47,Citation48]. SII was robust in predicting the prognosis of patients with HF, and elevated SII levels have been linked to worse survival outcomes in patients with chronic HF [Citation16,Citation44,Citation49]. Different from the above-cited studies, our results suggested that SII and PLR appeared to have a lack significant association with HF. Interestingly, CBC-derived indicators were more closely related to the occurrence of HF in patients with stroke and diabetes. This finding was consistent with our previous understanding that patients with stroke and diabetes were often associated with inflammation [Citation50–52].
In our analysis of the NHANES database, which is composed of multi-stage complex sampling data, we employed a weighted logistic regression model for analysis and adjusted other covariates, enhancing the accuracy and reliability of our conclusions. Additionally, by using restrictive cubic spline and smooth curve fitting, we delved into potential non-linear relationships and further calculated the inflection points. The findings of this study clarify the impact of CBC-derived indicators on the occurrence of HF, aiding clinicians in making appropriate interventions.
Nevertheless, it is imperative to acknowledge the limitations of our study. First, the cross-sectional design of the NHANES dataset inhibits the establishment of causal relationships, necessitating prospective investigations for causal determination. Second, bias from the methodology should be considered, for example, selection bias may occur during NHANES data selection. If the chosen sample is not representative of the general population, the study results may lack universality. Then, different statistical methods might lead to different results. For example, when using multiple linear regression, if the sample weights are not correctly handled, it could affect the accuracy of the results. After that, our results were adjusted for age, sex, race, and other confounding factors, but there may be unknown confounding factors affecting the analyses such as sports level and dietary patterns.
Before applying these findings to a broader population, we must consider several key factors to ensure the reasonableness and accuracy of extrapolation. Since NHANES is a database based in the United States, our findings may not necessarily apply to other countries or regions, especially those with different health conditions, cultural backgrounds dietary pattern, or medical practices. Secondly, the study considered a variety of potential confounding factors, such as age, gender, BMI, diabetes, hypertension et al. to reduce the interference of these variables on the results. However, there may still be unmeasured or uncontrolled confounding factors that could affect the results extrapolation. More studies are needed to confirm the stability and applicability of these results before they can be generalised to clinical practice or public health policy. Especially among populations of different geographical regions, ethnic groups, and health statuses, these results may require further validation and adjustment.
5. Conclusion
In conclusion, our findings augment the existing body of evidence supporting the clinical significance of CBC-derived indicators as factors which correlate to HF and disease outcomes. We observed that elevated levels of SIRI, NLR, and MLR are strongly associated with the risk of HF, with a nonlinear pattern. Furthermore, we delve into the turning points and subgroup differences. Future larger prospective studies are needed to establish causality and explore the potential clinical applications of CBC-derived indicators in HF.
Authors’ contributions
Y. Z. performed the data analysis and wrote the manuscript. L.F., B.H. and Z.Z. contributed to data collection and the literature search. X.L. oversighted and managed responsibility for the research activity. All authors contributed to the design of the study protocol and reviewed the manuscript. The authors read and approved the final manuscript.
Acknowledgments
We appreciate the personnel at the National Health and Nutrition Examination Survey for planning, collecting, and compiling NHANES data and establishing the public database. In addition, we want to acknowledge FigDraw (www.figdraw.com) for providing the graphic elements used in Graphical Abstract.
Disclosure statement
The authors report there are no competing interests to declare.
Additional information
Funding
References
- Chen J, Aronowitz P. Congestive heart failure. Med Clin North Am. 2022;106(3):447–458. doi: 10.1016/j.mcna.2021.12.002.
- Figueroa MS, Peters JI. Congestive heart failure: diagnosis, pathophysiology, therapy, and implications for respiratory care. Respir Care. 2006;51(4):403–412.
- Zannad F, Adamopoulos C, Mebazaa A, et al. The challenge of acute decompensated heart failure. Heart Fail Rev. 2006;11(2):135–139. doi: 10.1007/s10741-006-9484-x.
- Jung M, Apostolova LG, Moser DK, et al. Virtual reality cognitive intervention for heart failure: CORE study protocol. Alzheimers Dement (N Y). 2022;8(1):e12230. doi: 10.1002/trc2.12230.
- Sierpiński R, Sokolska JM, Suchocki T, et al. 10 Year trends in hospitalization rates due to heart failure and related in-Hospital mortality in Poland (2010-2019). ESC Heart Fail. 2020;7(6):3365–3373. doi: 10.1002/ehf2.13060.
- Andrews RE, Fenton MJ, Dominguez T, et al. British congenital cardiac association. Heart failure from heart muscle disease in childhood: a 5-10 year follow-up study in the UK and Ireland. ESC Heart Fail. 2016;3(2):107–114. doi: 10.1002/ehf2.12082.
- Mehmood M. Inflammation, heart failure, and the path forward. JACC Heart Fail. 2020;8(2):153–154. doi: 10.1016/j.jchf.2019.10.010.
- Wang H-W, Ahmad M, Jadayel R, et al. Inhibition of inflammation by minocycline improves heart failure and depression-like behaviour in rats after myocardial infarction. PLoS One. 2019;14(6):e0217437. doi: 10.1371/journal.pone.0217437.
- Kologrivova I, Shtatolkina M, Suslova T, et al. Cells of the immune system in cardiac remodeling: main players in resolution of inflammation and repair after myocardial infarction. Front Immunol. 2021;12:664457. doi: 10.3389/fimmu.2021.664457.
- Hanna A, Frangogiannis NG. Inflammatory cytokines and chemokines as therapeutic targets in heart failure. Cardiovasc Drugs Ther. 2020;34(6):849–863. doi: 10.1007/s10557-020-07071-0.
- Ferrucci L, Fabbri E. Inflammageing: Chronic inflammation in ageing, cardiovascular disease, and frailty. Nat Rev Cardiol. 2018;15(9):505–522. doi: 10.1038/s41569-018-0064-2.
- Silvestre-Roig C, Braster Q, Ortega-Gomez A, et al. Neutrophils as regulators of cardiovascular inflammation. Nat Rev Cardiol. 2020;17(6):327–340. doi: 10.1038/s41569-019-0326-7.
- Shi C, Pamer EG. Monocyte recruitment during infection and inflammation. Nat Rev Immunol. 2011;11(11):762–774. doi: 10.1038/nri3070.
- Rao M, Wang X, Guo G, et al. Resolving the intertwining of inflammation and fibrosis in human heart failure at Single-Cell level. Basic Res Cardiol. 2021;116(1):55. doi: 10.1007/s00395-021-00897-1.
- Chung I, Lip GYH. Platelets and heart failure. Eur Heart J. 2006;27(22):2623–2631. doi: 10.1093/eurheartj/ehl305.
- Tang Y, Zeng X, Feng Y, et al. Association of systemic immune-inflammation index with Short-Term mortality of congestive heart failure: a retrospective cohort study. Front Cardiovasc Med. 2021;8:753133. doi: 10.3389/fcvm.2021.753133.
- Gijsberts CM, Ellenbroek GHJM, Ten Berg MJ, et al. Effect of monocyte-to-lymphocyte ratio on heart failure characteristics and hospitalizations in a coronary angiography cohort. Am J Cardiol. 2017;120(6):911–916. doi: 10.1016/j.amjcard.2017.06.020.
- Afari ME, Bhat T. Neutrophil to lymphocyte ratio (NLR) and cardiovascular diseases: an update. Expert Rev Cardiovasc Ther. 2016;14(5):573–577. doi: 10.1586/14779072.2016.1154788.
- Djordjevic D, Rondovic G, Surbatovic M, et al. Neutrophil-to-lymphocyte ratio, monocyte-to-lymphocyte ratio, platelet-to-lymphocyte ratio, and mean platelet volume-to-platelet count ratio as biomarkers in critically ill and injured patients: which ratio to choose to predict outcome and nature of bacteremia? Mediators Inflamm. 2018;2018:3758068. doi: 10.1155/2018/3758068.
- Xia Y, Xia C, Wu L, et al. Systemic immune inflammation index (SII), system inflammation response index (SIRI) and risk of all-cause mortality and cardiovascular mortality: a 20-year follow-up cohort study of 42,875 US adults. J Clin Med. 2023;12(3):1128. doi: 10.3390/jcm12031128.
- Fain JA. NHANES: use of a free public data set. Diabetes Educ. 2017;43(2):151–151. doi: 10.1177/0145721717698651.
- WHO Expert Consultation. Appropriate body-Mass index for Asian populations and its implications for policy and intervention strategies. Lancet. 2004;363(9403):157–163. doi: 10.1016/S0140-6736(03)15268-3.
- Wu Z, Yue Q, Zhao Z, et al. A cross-sectional study of smoking and depression among US adults: NHANES (2005-2018). Front Public Health. 2023;11:1081706. doi: 10.3389/fpubh.2023.1081706.
- Asowata OJ, Okekunle AP, Adedokun B, et al. Alcohol use patterns and hypertension among adults in the United States: findings from the 2015-2016 NHANES data. Public Health. 2023;225:327–335. doi: 10.1016/j.puhe.2023.10.016.
- Crim MT, Yoon SSS, Ortiz E, et al. National surveillance definitions for hypertension prevalence and control among adults. Circ Cardiovasc Qual Outcomes. 2012;5(3):343–351. doi: 10.1161/CIRCOUTCOMES.111.963439.
- Bakris G, Ali W, Parati G. ACC/AHA versus ESC/ESH on hypertension guidelines: JACC guideline comparison. J Am Coll Cardiol. 2019;73(23):3018–3026. doi: 10.1016/j.jacc.2019.03.507.
- ElSayed, Nuha A, Aleppo, Grazia, Aroda, Vanita R, ; et al. 2. Classification and diagnosis of diabetes: standards of care in diabetes-2023. Diabetes Care, 2023, 46 (Suppl 1), S19–S40. doi: 10.2337/dc23-S002.
- Grundy SM, Stone NJ, Bailey AL, et al. 2018 AHA/ACC/AACVPR/AAPA/ABC/ACPM/ADA/AGS/APhA/ASPC/NLA/PCNA guideline on the management of blood cholesterol: a report of the American College of Cardiology/American Heart Association task force on clinical practice guidelines. Circulation. 2019;139(25):e1082–e1143. doi: 10.1161/CIR.0000000000000625.
- Dick SA, Epelman S. Chronic heart failure and inflammation: what do we really know? Circ Res. 2016;119(1):159–176. doi: 10.1161/CIRCRESAHA.116.308030.
- Lafuse WP, Wozniak DJ, Rajaram MVS. Role of cardiac macrophages on cardiac inflammation, fibrosis and tissue repair. Cells. 2020;10(1):51. doi: 10.3390/cells10010051.
- Dutka M, Bobiński R, Ulman-Włodarz I, et al. Various aspects of inflammation in heart failure. Heart Fail Rev. 2020;25(3):537–548. doi: 10.1007/s10741-019-09875-1.
- Chaar D, Dumont BL, Vulesevic B, et al. Neutrophils and circulating inflammatory biomarkers in diabetes mellitus and heart failure with preserved ejection fraction. Am J Cardiol. 2022;178:80–88. doi: 10.1016/j.amjcard.2022.05.026.
- Kain V, Halade GV. Role of neutrophils in ischemic heart failure. Pharmacol Ther. 2020;205:107424. doi: 10.1016/j.pharmthera.2019.107424.
- Lu Y, Xia N, Cheng X. Regulatory T cells in chronic heart failure. Front Immunol. 2021;12:732794. doi: 10.3389/fimmu.2021.732794.
- Bermea K, Bhalodia A, Huff A, et al. The role of B cells in cardiomyopathy and heart failure. Curr Cardiol Rep. 2022;24(8):935–946. doi: 10.1007/s11886-022-01722-4.
- Song B, Li T, Chen S, et al. Correlations between MTP and ROS levels of peripheral blood lymphocytes and readmission in patients with chronic heart failure. Heart Lung Circ. 2016;25(3):296–302. doi: 10.1016/j.hlc.2015.09.004.
- Arruda-Olson AM, Reeder GS, Bell MR, et al. Neutrophilia predicts death and heart failure after myocardial infarction: a community-based study. Circ Cardiovasc Qual Outcomes. 2009;2(6):656–662. doi: 10.1161/CIRCOUTCOMES.108.831024.
- Shahid F, Lip GYH, Shantsila E. Role of monocytes in heart failure and atrial fibrillation. J Am Heart Assoc. 2018;7(3):e007849. doi: 10.1161/JAHA.117.007849.
- Apostolakis S, Lip GYH, Shantsila E. Monocytes in heart failure: relationship to a deteriorating immune overreaction or a desperate attempt for tissue repair? Cardiovasc Res. 2010;85(4):649–660. doi: 10.1093/cvr/cvp327.
- Gurbel PA, Gattis WA, Fuzaylov SF, et al. Evaluation of platelets in heart failure: is platelet activity related to etiology, functional class, or clinical outcomes? Am Heart J. 2002;143(6):1068–1075. doi: 10.1067/mhj.2002.121261.
- Jafri SM. Hypercoagulability in heart failure. Semin Thromb Hemost. 1997;23(6):543–545. doi: 10.1055/s-2007-996133.
- Jacob J, Martin-Sanchez FJ, Gil V, et al. The prognostic usefulness of platelets in acute heart failure. Emerg Med J. 2011;28(12):1079. doi: 10.1136/emermed-2011-200591.
- Ijsselmuiden AJJ, Musters RJP, de Ruiter G, et al. Circulating white blood cells and platelets amplify oxidative stress in heart failure. Nat Clin Pract Cardiovasc Med. 2008;5(12):811–820. doi: 10.1038/ncpcardio1364.
- Wang X, Ni Q, Wang J, et al. Systemic inflammation response index is a promising prognostic marker in elderly patients with heart failure: a retrospective cohort study. Front Cardiovasc Med. 2022;9:871031. doi: 10.3389/fcvm.2022.871031.
- Liu Z, Zhou X. A nomogram based on systemic inflammation markers can predict adverse outcomes in patients with heart failure. Nan Fang Yi Ke Da Xue Xue Bao. 2022;42(8):1149–1158. doi: 10.12122/j.issn.1673-4254.2022.08.06.
- Angkananard T, Inthanoo T, Sricholwattana S, et al. The predictive role of neutrophil-to-lymphocyte ratio (NLR) and mean platelet volume-to-lymphocyte ratio (MPVLR) for cardiovascular events in adult patients with acute heart failure. Mediators Inflamm. 2021;2021:6889733. doi: 10.1155/2021/6889733.
- Delcea C, Buzea CA, Vîjan AE, et al. The platelet to lymphocyte ratio in heart failure: a comprehensive review. Rom J Intern Med. 2023;61(2):84–97. doi: 10.2478/rjim-2023-0006.
- Heidarpour M, Bashiri S, Vakhshoori M, et al. The association between platelet-to-lymphocyte ratio with mortality among patients suffering from acute decompensated heart failure. BMC Cardiovasc Disord. 2021;21(1):454. doi: 10.1186/s12872-021-02260-7.
- Wang Z, Qin Z, Yuan R, et al. Systemic immune-inflammation index as a prognostic marker for advanced chronic heart failure with renal dysfunction. ESC Heart Fail. 2023;10(1):478–491. doi: 10.1002/ehf2.14217.
- Muriach M, Flores-Bellver M, Romero FJ, et al. Diabetes and the brain: oxidative stress, inflammation, and autophagy. Oxid Med Cell Longev. 2014;2014:102158–102159. doi: 10.1155/2014/102158.
- Lontchi-Yimagou E, Sobngwi E, Matsha TE, et al. Diabetes mellitus and inflammation. Curr Diab Rep. 2013;13(3):435–444. doi: 10.1007/s11892-013-0375-y.
- Levard D, Buendia I, Lanquetin A, et al. Filling the gaps on stroke research: focus on inflammation and immunity. Brain Behav Immun. 2021;91:649–667. doi: 10.1016/j.bbi.2020.09.025.