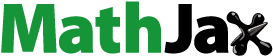
ABSTRACT
Customer geographical proximity is a factor influencing supplier firms’ trade-offs when granting customers trade credits. However, the extant literature offers scant empirical evidence on the effect of customer location on supplier firm’s trade credit. This paper investigates the impact of major customers’ geographical proximity on a supplier firm’s trade credit. Using a sample of 5,587 observations of publicly listed Chinese firms between 2007 and 2017, we document a positive relationship between major customers’ geographical distance and the supplier firm’s trade credit. Moreover, the main results remain robust when changing samples and considering endogeneity problems. This paper also tests two potential channels and identifies the exploring market channel that exists in our sample. Furthermore, the main relation is more pronounced in supplier firms with low financial risk, operating in areas of high marketization, high product development, strong legal environments, and low economic policy uncertainty. This paper extends the existing literature on the economic consequences of customer characteristics on supplier firms to include customer geographic proximity. In addition, this paper sheds light on supply chain risk management.
I. Introduction
Firms have a love-hate relationship with their customers. While firms rely on customers to buy their products to earn revenue, the integration and optimization of supply chains have concentrated the buying power of customers, bringing risk to firms’ operations (McKinsey Citation2010). Once major customers give up buying a supplier’s product, it might cause a significant loss to the supplier firm’s operation. Thus, firms always consider the above benefits and risks when dealing with their customers (Petersen and Rajan Citation1997).Footnote1 Existing studies examine the supplier-customer relationship in terms of, for example, the impact of major customers’ network structure (Jhang, Su, and Kao Citation2021), concentration (Huang et al. Citation2016; Wen, Ke, and Liu Citation2021), stability (Wen, Xia, and Ye Citation2020), and closeness of the relationship (Cen et al. Citation2017) on supplier firms’ corporate decisions. According to theories of information asymmetry and transaction costs, customers’ geographical location also plays a key role in the cost and benefit assessment of the firm (Choi and Krause Citation2006; North and North Citation1992; Petersen and Rajan Citation1997). This receives less attention in existing studies (Chu, Tian, and Wang Citation2019). In this paper, we investigate the impact of the geographic proximity of major customers on supplier firms’ willingness to provide those customers with trade credit.
Trade credit is an important financial flow in the supply chain. It affects the supplier-customer relationship and supports the firm in increasing its production activities and product quality (Jhang, Su, and Kao Citation2021; Rui and Lai Citation2015). Moreover, it requires that the firm consider potential benefits and risks (Fabbri and Klapper Citation2016). Existing empirical studies examine the determinants of trade credit, mainly focusing on firm characteristics such as cash flow and profitability (Dass, Kale, and Nanda Citation2014; Yang and Birge Citation2018). Jhang, Su, and Kao (Citation2021) extend this strand of the literature to investigate the impact of major customers’ network structures on supplier trade credit. They find that network interconnectedness is negatively associated with supplier trade credit, and network integration is positively associated with supplier trade credit. Inspired by their study, we further explore the impact of another factor in the supplier-customer relationship, the customer’s geographic proximity, on supplier trade credit.
Customer proximity could affect a supplier’s trade credit in two ways. First, customer proximity may increase the trade credit extended. Existing studies show that a short distance facilitates soft information exchange and reduces transaction costs (Ferris Citation1981; Wilson and Summers Citation2002). The low information cost enhances cooperation between suppliers and customers in advertising, marketing, and joint investment (Chu, Tian, and Wang Citation2019). A good relationship increases a supplier firm’s willingness and ability to provide trade credit to its major customers. Moreover, a short distance increases the possibility of both parties being under the jurisdiction of the same local government. Local governments may also enhance the stability of the relationship between firms and their major local customers to increase employment and economic growth (Jia and Mayer Citation2017). The efforts and pressure exerted by the local government may make firms more likely to provide trade credit to their close geographic customers.
However, customer proximity may also decrease the extent of trade credit. The strong bargaining power of close geographic customers may imply that the firm relies on its customers, which makes the conversion cost much higher and gives the close customer more bargaining power. The potential operating risk arising from close geographic customers’ strong bargaining power (e.g. customers require a lower price and more inventory) or the potentially high conversion cost may be mitigated by the supplier firm decreasing trade credit to close geographic customers. Firms might also use the guarantee function of trade credit to gain a competitive advantage in the industry (Fisman and Raturi Citation2004). This may facilitate their providing more trade credit to faraway customers to encourage product sales and maintain customer relations. In sum, it is unclear ex-ante how customer geographic proximity might affect supplier trade credit. This study focuses on 5,587 firm-year observations of Chinese-listed firms from 2007 to 2017 to investigate this empirical question.
Our findings make several contributions. First, we extend the literature on the impact of customer characteristics on firm behaviorbehaviour to investigate the impact of the customer’s geographic proximity. Prior studies on the economic consequences of customer characteristics mainly focus on customer concentration. For example, they examine the impact of customer concentration on a firm’s earnings management (Raman and Shahrur Citation2008), accounting conservatism (Hui, Klasa, and Yeung Citation2012), bank loans (Campello and Gao Citation2017), tax avoidance (Huang et al. Citation2016), CSR (Wen, Ke, and Liu Citation2021), analyst forecasting (Luo and Nagarajan Citation2015), auditor selection, and audit expenses (Krishnan et al. Citation2019). Few studies examine the effect of customers’ geographical distance. Compared to customer concentration, geographical distance can capture a firm’s potential costs in a more straightforward way by considering the information and transaction costs. This paper examines the influence of customer geographical distance on the provision of trade credit, extending the existing literature on the economic consequences of customer concentration to consider the impact of customers’ geographical location and distance.
Second, we extend the literature on the factors influencing trade credit to include customer characteristics and geographical distance. Existing studies on trade credit are mainly at the firm level and on issues such as ownership, bank loans, and product competition (Biais and Gollier Citation1997; Cull et al. Citation2017; Love, Preve, and Sarria-Allende Citation2007). At the institutional level, existing studies examine firm financial development, trust level, and marketization (Ayyagari, Demirgüç-Kunt, and Maksimovic Citation2010; Fisman and Love Citation2003; Oh and Kim Citation2016; Tosun and Yildiz Citation2022). However, very few studies consider the relationship between customers and suppliers (Jhang, Su, and Kao Citation2021). Compared to the relationship factors considered in existing studies, customer characteristics directly correlate with trade credit. This study offers related empirical evidence.
Finally, our study contributes to the literature on the positive relationship between customer geographic distance and trade credit. These findings provide empirical evidence concerning the relationship between firms and customers and are relevant to supply chain risk management. On the one hand, in respect of integrating and optimizing the supply chain, the firm should adequately manage the risk of providing trade credit when it chooses customers that are far away. On the other hand, regulators should pay more attention to the potential risk to the market order of firms with customers who are located far away.
The remainder of this paper is organized as follows. Section II develops the hypotheses. Section III describes the data and empirical methodology. Section IV presents the empirical results. Section V concludes.
II. Literature review and hypotheses development
Customer geographical distance refers to the distance between the firm and its major customers. Baldwin (Citation2012) demonstrates that geographical location is one of a firm’s main considerations when choosing customers. This is because location affects transaction costs, information exchange, and knowledge spillover between the firm and its customers. The existing financial and accounting literature investigates the impact of the geographical location of a bank or factory on firm decisions, mainly from the perspective of information exchange and supervision. For example, Degryse and Ongena (Citation2005) find that geographical distance between commercial borrowers and the lending bank positively impacts bank loan rates through transportation costs. Agarwal and Hauswald (Citation2010) show that borrower proximity gives nearby firms more readily access to credit from a bank because that proximity facilitates the collection of soft information. Knyazeva and Knyazeva (Citation2012) also find that banks require covenant provisions or collateral when lending to borrowers located far away. Giroud (Citation2013) finds that proximity to plants increases plant-level investment and productivity since it is easier for headquarters to monitor and acquire information on those plants.
Recently, there has been increased attention from academics to investigate the impact of customer geographical distance on supplier firms’ corporate decisions. Chu, Tian, and Wang (Citation2019) find that the knowledge spillover effect of customer geographical proximity increases firm innovation. Cao, Zhang, and Yuan (Citation2022) find that supplier−customer proximity can mitigate supplier crash risk. Huang and Fan (Citation2022) suggest that supplier-customer geographic proximity reduces supplier risk-taking. Customer proximity thus potentially influences the costs and benefits assessment in corporate decisions, and the extent of trade credit is an important result of the trade-off. Following this pattern, our paper investigates the influence of customer geographical distance on trade credit.
Customer geographical distance and trade credit
Theoretically, the geographical distance of customers may have positive or negative impacts on trade credit. A firm might be more likely to provide trade credit to customers at a close distance. When the customer is close to the firm, it is easy to reduce transaction costs and obtain local information on customers. This is beneficial to fostering long-term relations with customers (Ferris Citation1981; Wilson and Summers Citation2002). For example, firms and their customers could cooperate more in advertising and marketing or engage in joint investment. These efforts could, in turn, help improve their firm performance and market value (Gosman and Kohlbeck Citation2009; Huo, Zhao, and Zhou Citation2014; Kalwani and Narayandas Citation1995; Patatoukas Citation2012). Good performance enhances the trade credit to geographically close customers.
Local governments also have incentives to promote stable and reliable customer relations between firms and their major local customers to increase employment and economic growth (Jia and Mayer Citation2017). After all, stable and reliable customer relations will not only help alleviate moral hazards and adverse selection problems between the firm and its major customers in the process of trading but also improve the firm’s willingness to offer trade credit. Moreover, with improved performance, the firm can also have the ability to provide most customers (including their main local customers) with additional trade credit.
A firm may, however, be more likely to provide trade credit to geographically remote customers. One reason for this is that they may not be able to provide trade credit to close customers because of the strong bargaining power of those customers. Being a close customer implies the firm’s increased reliance on the relationship, making the conversion cost much higher and giving the close customer more bargaining power. Under the pressure of customers’ bargaining power, the firm may need to compromise and reduce selling prices or reserve excess inventory, which will harm its performance (Gosman et al. Citation2004; Piercy and Lane Citation2006; Porter Citation1974). The decline in performance might reduce the firm’s ability to provide trade credit to customers and its ability to improve trade credit to close customers.
The close geographical proximity of customers also means higher operational risks for firms. Any geographically close natural disasters or accidents might interrupt the trading relationship between these major customers and supplier firms and cause high conversion costs (Bode and Wagner Citation2015; Choi and Krause Citation2006; Maksimovic and Titman Citation1991).Footnote2 A firm may reduce this kind of risk by reaching out to customers far away, thereby diversifying its customers and its risk. According to transaction theory, trade credit provides a warranty for quality when customers are too far away to directly observe product characteristics (Long, Malitz, and Ravid Citation1993). Thus, firms are motivated to use the guarantee function of trade credit to gain a competitive advantage in the industry (Fisman and Raturi Citation2004). Firms might provide trade credit to faraway customers to expand and maintain customers with whom they have extensive information asymmetry because of geographical distance and to provide them with product quality assurance (Janet Citation1987); they provide this credit in exchange for product sales and to maintain a good relationship with customers to support for their products (Bougheas, Mateut, and Mizen Citation2009; Brennan, Miksimovic, and Zechner Citation1988; Klapper, Laeven, and Rajan Citation2010).
The aforementioned discussion leads to the competitive baseline hypothesis:
Hypothesis 1a
There is a negative relationship between major-customer geographical distance and trade credit.
Hypothesis 1b:
There is a positive relationship between major-customer geographical distance and trade credit.
III. Research design
Data
We focus on firms listed on the Shanghai Stock Exchange or Shenzhen Stock Exchange from 2007 to 2017.Footnote3 We obtain the customer geographical distance from the Supply Chain database from the China Stock Market & Accounting Research Database (CSMAR), which provides great-circle distance data between a listed firm and its top-five customers. This data is calculated based on the latitudes and longitudes of each party, using the Haversine formula as described by Kang and Kim (Citation2008).
Where and
are the latitudes and longitudes of a supplier firm s and its customer c, respectively. r is the radius of the earth ( = 6,371 km). The trade-credit data are from the Chinese Research Data Services. All financial data are from the CSMAR database.
We exclude financial firms because of the specific regulations (You, Zhang, and Zhang Citation2017). We also exclude firms whose financial information is missing. The above sample-selection criteria yield a sample of 5,587 firm-year observations.
Variable definition
Dependent variables
Our dependent variable AR denotes the customer’s trade credit, which is accounts receivable. We measure AR1 as the accounts receivable in the current year minus accounts receivable in the prior year, divided by total revenue. Following the prior literature (Fabbri and Klapper Citation2016), we also use an alternative measurement of the dependent variable, AR2, which is measured as the sum of accounts receivable and notes receivable in the current year minus the sum of accounts receivable and notes receivable in the prior year, divided by total revenue.
Independent variables
Consistent with previous studies (Giroud Citation2013; Petersen and Rajan Citation2002), we construct the customer-weighted geographical distance indicator, Distance, to measure the distance of the firm’s top-five customers:
where is the weighted distance of firm i of the top-five customers in t year.
represents the absolute distance between firm i and one of its top-five customers, j, in year t.
represents the ratio of the sales of each top-five customer j corresponding to the total sales of firm i in year t.
Control variables
Following the existing literature (Cull, Xu, and Zhu Citation2009; Lawrenz and Oberndorfer Citation2018; Love, Preve, and Sarria-Allende Citation2007; Petersen and Rajan Citation1997), we include a set of control variables. They are as follows: customer concentration (CC), management shareholdings (Msh), top shareholder share (Top1), the growth of firm revenue (Growth), return on assets (ROA), the ratio of total liabilities to total assets (Leverage), the ratio of cash holding to total assets (Cashhold), and the natural logarithm of total assets (Size). Gdpper, Loan, and Core are annual per capita GDP of each city, annual loan of each city, and whether city is a first-tier, provincial or capital city. Appendix 1 provides detailed definitions and data sources for all variables.
Empirical model
Following the existing literature (Petersen and Rajan Citation1997; Cull, Xu, and Zhu Citation2009; Wen, Xia, and Ye Citation2020; Wen, Ke, and Liu Citation2021), we use the following regression to test the main hypothesis:
We include industry-, city-, and year-fixed effects to control unobservable industry and city characteristics and time trends. The standard errors are clustered at the firm-year level. All the continuous variables are winsorized at the 1% and 99% levels to reduce the influence of outliers.
Descriptive statistics
Panel A of shows the full sample descriptive statistics for the primary analyses in this study. We find that the mean of AR1 is 0.0392, which denotes that, on average, 3.92% of revenue is accounts receivable increase. Regarding the effect of a change in notes receivable, the mean of AR2 is 0.0453, which indicates that 4.53% of the revenue is the increase in accounts receivable and notes receivable, on average. The average customer geographical distance is 3.3945 (28.7997 km), and the standard deviation is 1.4475. The mean and median of CC are 0.0683 and 0.0226 in our sample. Nearly 54.29% of the sample is located in a first-tier, provincial, or capital city. The results for the control variables are consistent with existing studies (Wen, Ke, and Liu Citation2021; Wen, Xia, and Ye Citation2020).
Table 1. Summary statistics and correlations.
Correlation analysis
Panel B of provides Pearson (below the diagonal) and Spearman (above the diagonal) correlations. Both sets of coefficients show that Distance is significantly positively correlated with AR1 and AR2. This provides preliminary evidence for Hypothesis 1b that customer geographic distance positively impacts corporate trade credit. In addition, all correlation coefficients are relatively low, indicating that multicollinearity is not a serious concern in our regression model.
IV. Empirical results
Main results
shows the empirical results for Hypothesis 1. We find a significant positive association between Distance and AR1(columns 1,2,4)/AR2 (columns 5,6,8); the coefficients are 0.0036 and 0.0034, and both are significant at the 1% level. This result supports Hypothesis 1b. Our results are also economically significant. For example, an increase of 1 standard deviation in customer distance is associated with an increase of 13.29% (10.86% for AR2) in supplier trade credit relative to the sample mean. Footnote4
Table 2. Customer geographical distance and trade credit.
Customer concentration may be a vital factor affecting trade credit due to the stronger bargaining power of major customers. We show the results only include the CC in the baseline model and remove the key variable Distance. Columns 3 and 7 of show that customer concentration significantly increases trade credit. Furthermore, columns 4 and 8 show customer geographical distance has an additional impact on trade credit when controlling the effect of customer concentration.Footnote5
Among the control variables, the coefficients of Msh, Growth, and ROA are positive and significant, suggesting that the percentage of management shareholdings, firm growth, and performance may have an impact on the ability of the supplier firm to provide trade credit, which is consistent with the argument of existing studies (Afrifa and Gyapong Citation2017; Paul and Boden Citation2008). The coefficient of Top1 is negative and significant, indicating that Top1 shareholders may decrease the firm’s intention to provide trade credit. We interpret this result as the largest shareholder being risk-averse and preferring to have the benefit of more cash flow rather than offering that to customers. The coefficient of Leverage is negative and significant, suggesting that the firm with more leverage is less able and less likely to provide trade credit (Afrifa and Gyapong Citation2017).
Robustness checks
We conduct a variety of robustness tests of our main results. First, we eliminate the sample of related-party transactions to ensure that our main results are not driven by these factors (Chung, Choi, and Jung Citation2019). We identify the related-party transactions as follows: 1) We check the address of the customer and the address of the supplier firm. 2) If they are the same, we manually check to see if they are related (e.g. if the customer firms are the supplier firm’s subsidiaries). 3) We drop from the sample the related-party transactions. We reestimate the model based on new sample of 5,446 observations. As shown in , the results still hold.
Table 3. Robustness tests: deleting the sample of related party transactions.
Second, we keep the sample in which the top-five customers account for at least 80% of the supplier’s revenue. The high ratio of revenue from the top-five customers indicates their importance and bargaining power. We are left with 723 observations, and the results still hold in .
Table 4. Robustness tests: keep the sample of top five customers account for at least 80% of the supplier’s revenue.
Third, we identify accounts receivable from the top-five customers by using the fuzzy matching method to match the information of the top-five customers to the accounts receivable details disclosed in Notes to Consolidated Financial Statements and try to obtain the corresponding accounts receivable information of the top-five customers for that year. AR3 is measured as accounts receivable from five major customers in the current year minus accounts receivable from five major customers in the prior year, then divided by total revenue. Based on 4,132 observations from the above method, the results still hold in .
Table 5. Robustness tests: Focusing on the trade credit from the top five major customers.
Forth, we separately present the results of the effect of the top-five customers’ geographic distances on trade credit. We measure the major customers’ geographic distance as a weighted distance for the top-five customers in the main results. We further explore the specific characteristics of each customer by including five customers’ geographic distances in the model to see the effect of each on the supplier’s trade credit. The results are shown in Panel A and B of . The Distance1st to Distance5th in Panel A is calculated by the distance of each top-five customer with equal weight, and the Distance1st to Distance5th in Panel B is calculated by the weighted distance of each top-five customer according to each customer’s sales as a percentage of the supplier’s total revenue. We address the impact of related-party transactions on trade credit by deleting the sample of related parties. We are left with a sample of 4,339 observations. The results show that Distance2nd and Distance3rd are positive and significant, indicating that the main results might be driven mainly by the second and third-top customers.
Table 6. Robustness tests: Detailed each customer distance.
Finally, firms located in a first-tier, provincial, or capital city may be better able to provide trade credit and may be better supported by the government. This may affect the relationship between major customer distance proximity and trade credit, and we thus drop the sample of firms located in such cities. shows that the main results exist based on 2,554 remaining observations.
Table 7. Robustness tests: Dropping the sample of firms located in a first-tier, provincial or capital city.
Endogeneity problems
An instrumental variable approach
There may be an endogeneity problem in our main results since these could be jointly driven by unobserved factors that affect trade credit and are correlated with the geographic proximity of customers to the supplier firm. We mitigate the effect of omitted variable bias on our main results by employing the two-stage least squares (2SLS) approach using two instrumental variables (IV).
The first exogenous instrumental variable, Diver, represents the local linguistic diversity as an indicator of cultural diversity in each province (data sourced from the Language Atlas of China, a collaborative work by the Australian Academy of the Humanities and the Chinese Academy of Social Sciences).Footnote6 A higher Diver value indicates the supplier firm’s location is more culturally diverse. Firms in culturally diverse areas may be more likely to seek remote customers. The second exogenous instrumental variable, Temple, represents the characteristics of local traditional culture, the number of religious places per 10,000 square kilometerskilometres in each province (data from CSMAR). A higher value for Temple indicates that predetermined traditional cultural symbols, including those of Buddhism and Taoism, can induce a relative conservatism in the corporate business model. The firms in these places might be more accepting of local forms of social communication and might also be more risk-averse (Meyners et al. Citation2017). This might lead them to prefer close customers and be less likely to choose those who are remote. In sum, these two instrumental variables could directly influence the supplier firms’ choices concerning customer geographic proximity but not influence the supplier’s trade credit; this allows us to alleviate the problem of correlated omitted variable bias.
presents the results of the 2SLS regression. As expected, Diver has a statistically significant positive correlation with Distance, while Temple has a statistically significant negative correlation with Distance. Columns 2 and 3 present the results from the second-stage regression estimation and the coefficients of are still positive and statistically significant at the 1% level for AR1 (AR2). Moreover, the Wu-Hausman test strongly rejects the null hypothesis of the endogeneity problem (p-value of 0.0000 (0.0004) for AR1 (AR2)). The weak-instrument test and overidentification test also strongly support the appropriateness of the instrumental variables, suggesting that the instrumental variables selected are valid.
Table 8. Instrumental variable approach.
Two-stage regression analysis
Our main results might also face the potential endogeneity problem of spurious correlation. Following Patatoukas (Citation2012), we use the two-stage regression analysis to allay concerns of spurious correlations. In the first stage, we estimate the residuals from annual cross-sectional regressions of the raw values of customer geographical distance on a vector of variables. We use factors such as UncertaintyFootnote7, Size, and RegindFootnote8 (Elmaghraby Citation2000; Kekre, Murthi, and Srinivasan Citation1995) to explain the customer distance to obtain the Distance residual term (Distan_Resid). In the second-stage estimation, we use the ‘residual changes’ in lieu of the ‘observed changes’ in customer geographical distance, and the Distan_Resid estimated in the first stage in place of Distance for the regression in the second stage.
presents the second-stage regression results focusing on the association between subsequent efficiency gains and contemporaneous residual changes in customer geographical distance.Footnote9
Additional tests
Financial risk
Supplier firms’ willingness and ability to provide trade credit are affected by their financial situation (Love, Preve, and Sarria-Allende Citation2007). The degree of financial risk that firms face may affect their ability to provide trade credit to downstream customers. When the firm is at high financial risk, it might face a higher risk of decreasing cash flow, operational trouble, or even potential bankruptcy if it continues to provide trade credit to customers. For example, Love, Preve, and Sarria-Allende (Citation2007) argue that firms that are financially more vulnerable to crises extend less trade credit to their customers. In this regard, Shenoy and Williams (Citation2017) investigate trade credit in the supplier-customer relationships of US-listed firms. They find that supplier firms with greater banking liquidity and lower financial risk offer more trade credit to their customers. Therefore, financial risk may weaken the relationship between major-customer geographic distance and the supplier firm’s trade credit.
We empirically test the above prediction by including the variable Risk and the interaction of Risk and Distance in EquationEquation (3)(3)
(3) . Risk is an indicator variable measured by the natural logarithm of one plus the total debt divided by total assets. The coefficients in on the Risk*Distance interaction are significantly negative at the 10% level. These results suggest that suppliers having high financial risk negatively moderates the positive association between major-customer geographic distance and the supplier firm’s trade credit.
Table 10. Moderating variable: Financial risk.
Market characteristics
Supplier firms’ incentives and ability to extend trade credit may also vary with the market characteristics. First, marketization is vital for enhancing economic growth in China (Fan, Wang, and Zhu Citation2011). China’s provinces experience different levels of marketization. A high degree of marketization usually indicates the increased role of the market in resource allocation and the presence of firms with an abundance of conveniences, such as lower transaction costs, more workers, and better legal protection. In areas with high marketization, close customers have easier access to suppliers’ private information on operation, production, and management and can supervise their behaviorsbehaviours, giving those customers greater bargaining power (Diamond Citation1984, Citation1991). Suppliers wishing to diversify their operational risk might be more willing to provide trade credit to long-distance customers than to those nearby. In addition, since a competitive market could enhance a firm’s operating efficiency, suppliers in areas with high marketization are more motivated to provide trade credit to attract major long-distance customers. Moreover, it is easier for firms in high marketization areas to achieve good performance, making them more able to provide trade credit. In sum, the marketization level may positively moderate the relationship between major customers’ geographic distance and the supplier firm’s trade credit.
We empirically test the above prediction by including the variable Market and the interaction of Market and Distance in EquationEquation (3)(3)
(3) . Market is the provincial-level marketization index from the NERI (Fan, Wang, and Zhu Citation2011). A higher marketization index indicates a higher marketization process. The coefficients in on interaction Market*Distance are significantly positive at the 5% level. These results suggest that the positive association between major-customer geographic distance and the supplier firm’s trade credit is more pronounced for suppliers operating in areas of high marketization.Footnote10
Table 11. Moderating variable: Market development.
Similarly, product-market development has an important impact on corporate behaviorsbehaviours (Dass, Kale, and Nanda Citation2014). A good product market can provide firms with opportunities to perform and an environment in which they can cooperate and explore remote supplier-customer relationships, thereby putting them in a position to extend trade credit. As with marketization, we expect the level of product-market development may positively moderate the relationship between major customers’ geographic distance and the supplier firm’s trade credit. We also empirically test the prediction by including the variable Product and the interaction of Product and Distance in EquationEquation (3)(3)
(3) . Product is the provincial-level product development index from the NERI (Fan, Wang, and Zhu Citation2011). A higher product index indicates a higher product development level. In columns 1 and 2 of , the coefficients on interaction Product*Distance are significantly positive at the 5% level. These results suggest that the positive association between major-customer geographic distance and the supplier firm’s trade credit is more pronounced for suppliers operating in areas with high levels of product development.
Table 12. Moderating variable: Product development.
The final market characteristic we explore is the legal environment. A strong legal environment and effective law enforcement increase the likelihood of firms cooperating along the supply chain and setting up good relationships. Hence, firms operating in stronger legal environments are more able and more motivated to provide trade credit to geographically remote customers than those in poorer legal environments. We thus predict that the positive association between major customers’ geographic distance and the supplier firm’s trade credit is more pronounced for firms operating in a strong legal environment.
We also empirically test the prediction by including the variable Law and the interaction of Law and Distance in EquationEquation (3)(3)
(3) . Law is the provincial-level law enforcement effectiveness index from the NERI (Fan, Wang, and Zhu Citation2011). A higher product index indicates a higher law enforcement effectiveness. In columns 1 and 2 of , the coefficients on interaction Law*Distance are significantly positive at the 1% level. These results suggest that the positive association between major-customer geographic distance and the supplier firm’s trade credit is more pronounced for suppliers operating in areas with highly effective law enforcement.
Table 13. Moderating variable: Law.
Economic policy uncertainty
Theoretical work points out that high levels of EPU puts upward pressure on firms’ cost of finance and decrease their incentives to invest (Nodari Citation2014; Pastor and Veronesi Citation2012). Jory et al. (Citation2020) find that firms tend to tighten their trade credit during periods of high EPU, for example, curtailing their receivables periods and facing shorter payables periods from suppliers. Overly reducing trade credit can lead to losing customers to competitors and may decrease the firm’s value in the long term. We expect the impact of major-customer geographic distance on trade credit may be different for supplier firms depending on the levels of uncertainty. Supplier firms in high-EPU contexts may be more likely to be risk-averse, which decreases their willingness to provide trade credit. Thus, we predict EPU negatively moderates the positive association between major-customer geographic distance and supplier firm trade credit.
We empirically test the above prediction by including the variable Epu and the interaction of Epu and Distance in EquationEquation (3)(3)
(3) . Epu measures policy uncertainty using the BBD news-based index developed by Baker, Bloom, and Davis (Citation2016). A higher Epu index value indicates a higher level of policy uncertainty. In columns 1 and 2 of , the coefficients on interaction Epu*Distance are significantly negative at the 5% level at least, suggesting that EPU negatively moderates the positive relationship between major-customer geographic distance and the supplier firm’s trade credit.
Table 14. Moderating variable: EPU.
Potential channels
In Section II, we argue that supplier firms may have two main intentions. First, supply firms offer trade credit to geographically remote customers to diversify the potential operating risk from those relationships with customers in close proximity. Once the relationship is suspended, the supplier firm may face a high conversion cost. The second intention of supplier firms may be related to the guarantee function of trade credit that allows them to gain a competitive advantage in the industry (Fisman and Raturi Citation2004). As a result, the firm may advance additional trade credit to customers at a distance in return for their purchase of products and to maintain the relationship.
Diversifying risk channel
We test the supply firms’ intention to diversify operating risk related to customers’ geographic proximity by exploring the moderating effect of areas being prone to natural disasters. We assume that the firms that operate in such areas are more concerned with the supply chain operating risk. Therefore, they have a greater incentive to seek geographically remote customers and keep a good relationship with them, thereby diversifying the potential operating risks from geographically close customers.
To test this channel, we construct a variable Disaster, which equals the natural logarithm of one plus the number of environmental disasters each year in the city (data from RESSET/NATD database). We include the variable Disaster and the interaction of Disaster and Distance in EquationEquation (3)(3)
(3) . Columns 1 and 2 of show that the coefficients of Disaster*Distance for both AR1 and AR2 are insignificant. These results indicate that the risk diversification channel is not statistically significant in our sample.
Table 15. Channel 1: Diversify risk channel: Firms operate in areas prone to natural disasters.
Exploring market channel
We test the supply firms’ intention to explore market channels by investigating the moderating effect of customer location. First, we construct a variable, Different city, which is equal to one if more than half of the firm’s top-five customers are located in different cities from the supplier firm, and zero otherwise. We include this variable and the interaction of Different city and Distance in EquationEquation (3)(3)
(3) . We find positive and significant coefficients of Different city*Distance in . These results show that the positive association between major customers’ geographic distance and supplier firms’ trade credit is more pronounced where supplier firms and customers are from different cities. This supports the assumption that supplier firms use the guarantee function of trade credit to gain a competitive advantage in the industry and cultivate good supplier-customer relationships.
Table 16. Channel 2: Explore market channel: Customers from different cities.
V. Conclusion
The existing research on the factors influencing the supplier-customers trade-offs focuses on the impact of supplier’s corporate decisions on aspects of the supplier-customer relationships: major customers’ network structure (Jhang, Su, and Kao Citation2021), concentration (Wen, Ke, and Liu Citation2021; Huang et al. Citation2016), stability (Wen, Xia, and Ye Citation2020), and closeness of the relationship (Cen et al. Citation2017). This study focuses on customers’ geographical location and shows that the supplier firm may choose to provide more trade credit to geographically remote customers to explore new markets. In addition, the main results remain robust when using different samples and considering endogeneity. Moreover, we find that the positive relation between major customers’ geographical distance and trade credit is more pronounced in supplier firms with low financial risk, firms operating in an area of high marketization, high product development, strong legal environment, and low EPU. These findings are interesting and provide a better understanding of a supplier firm’s trade credit decisions that, when facing geographically remote customers, vary according to different internal and external environments. Finally, we explore the potential channels and find the exploring market channel in our sample.
The findings have implications for a supplier firm when they choose and deal with customer relationships and supply chain risk management. For example, we recommend that the company should rigorously evaluate its cash flow risk when considering the trade-off of extending trade credit to major customers far away for exploring the market. These findings also remind related regulators to pay more attention to the potential risk among firms whose customers are far away to maintain steady market order.
The limitation of this study is as follows: First, we can only capture the major customer geographical location rather than other customer characteristics that also play an important role in influencing suppliers’ incentives to provide trade credit. Second, our evidence is only based on the sample of listed Chinese firms and calls for further study of global supply chain relationships.
Disclosure statement
No potential conflict of interest was reported by the author(s).
Notes
1 Explanations of this, such as the financing comparative advantage theory, price discrimination theory, transaction cost theory are discussed in detail in Petersen and Rajan (Citation1997). We briefly discuss the theoretical basis in the second section.
2 For example, if the supplier chooses customers who are geographically close, once the relationship is suspended, the supplier firm may face additional costs to find geographically remote customers. However, on the contrary, if the supplier firm chooses geographically remote customers, once the relationship is suspended, the cost of transferring to geographically close customers is lower. There are two reasons for this result: 1) transferring from geographically remote to close customers can save time and search costs for supplier firms, and 2) special investments, such as a complete long-distance relationship network, with geographically remote customers can be applied to geographically close customers and search costs for new customers is lower.
3 On 15 February 2006, the Ministry of Finance of the People’s Republic of China (the ‘MoF’) formally announced the issuance of the Accounting Standard for Business Enterprises (‘ASBE’), which covers nearly all topics under the current IFRS literature. These standards, which were effective from 1 January 2007. Therefore, we start the sample from 2007.
4 The economic significance is calculated as follows: (0.0036 × 1.4475)/0.0392 = 13.29% and (0.0034 × 1.4475)/0.0453 = 10.86%. As shown in Panel A of , the standard deviation of Distance is 1.4475 and the mean of AR1 (AR2) is 0.0392 (0.0453).
5 We conducted an additional test by adding an interaction term between CC and Distance to the baseline model. We find that customer geographical distance strengthens the impact of customer concentration on trade credit. These results are not reported for the sake of brevity.
6 Chang et al. (Citation2015) provide a detailed discussion about this database and the variable identification.
7 Here we use the standard deviation of the revenue growth in the last three years to measure the uncertainty of demand. As Uncertainty requires firms to observe values for three consecutive years, which reduce the number of samples in to 5,045. Since Size is included in the first stage of the regression, we exclude this control variable in the second stage.
Table 9. Two-Stage Regression Analysis.
8 We follow Bradshaw, Liao, and Ma (Citation2019) and Chang, Chen, and Liao (Citation2014) to measure regulated industries, including mining, railroads, trucking, airlines, telecommunications, energy supply, and media.
9 We also adopted the Heckman two-stage regression method to alleviate another potential concern of sample self-selection due to firms’ voluntary disclosure of top-5 customer information. The results show that the inverse Mills ratio (IMR) is not significant, indicating that there is no serious problem of sample self-selection. We did not include these results in the paper for the sake of brevity.
10 As we use leverage to measure risk at the firm level, there is collinearity between risk and leverage. So, we exclude leverage of the vector of control variables.
References
- Afrifa, G. A., and E. Gyapong. 2017. “Net Trade Credit: What are the Determinants?” International Journal of Managerial Finance 13 (3): 246–266. doi:10.1108/IJMF-12-2015-0222.
- Agarwal, S., and R. Hauswald. 2010. “Distance and Private Information in Lending.” The Review of Financial Studies 23 (7): 2757–2788. doi:10.1093/rfs/hhq001.
- Ayyagari, M., A. Demirgüç-Kunt, and V. Maksimovic. 2010. “Formal versus Informal Finance: Evidence from China.” The Review of Financial Studies 23 (8): 3048–3097. doi:10.1093/rfs/hhq030.
- Baker, S. R., N. Bloom, and S. J. Davis. 2016. “Measuring Economic Policy Uncertainty.” The Quarterly Journal of Economics 131 (4): 1593–1636. doi:10.1093/qje/qjw024.
- Baldwin, R. 2012. Global Supply Chains: Why They Emerged, Why They Matter, and Where They are Going. CEPR Discussion Papers.
- Biais, B., and C. Gollier. 1997. “Trade Credit and Credit Rationing.” The Review of Financial Studies 10 (4): 903–937. doi:10.1093/rfs/10.4.903.
- Bode, C., and S. M. Wagner. 2015. “Structural Drivers of Upstream Supply Chain Complexity and the Frequency of Supply Chain Disruptions.” Journal of Operations Management 36: 215–228. doi:10.1016/j.jom.2014.12.004.
- Bougheas, S., S. Mateut, and P. Mizen. 2009. “Corporate Trade Credit and Inventories: New Evidence of a Trade-Off from Accounts Payable and Receivable.” Journal of Banking & Finance 33 (2): 300–307. doi:10.1016/j.jbankfin.2008.07.019.
- Bradshaw, M., G. Liao, and M. S. Ma. 2019. “Agency Costs and Tax Planning When the Government is a Major Shareholder.” Journal of Accounting and Economics 67 (2–3): 255–277. doi:10.1016/j.jacceco.2018.10.002.
- Brennan, M. J., V. Miksimovic, and J. Zechner. 1988. “Vendor Financing.” The Journal of Finance 43 (5): 1127–1141. doi:10.1111/j.1540-6261.1988.tb03960.x.
- Campello, M., and J. Gao. 2017. “Customer Concentration and Loan Contract Terms.” Journal of Financial Economics 115 (1): 168–188. doi:10.1016/j.jfineco.2016.03.010.
- Cao, F., X. Zhang, and R. Yuan. 2022. “Do Geographically Nearby Major Customers Mitigate suppliers’ Stock Price Crash Risk?” The British Accounting Review, Forthcoming 54 (6): 101118. doi:10.1016/j.bar.2022.101118.
- Cen, L., E. L. Maydew, L. Zhang, and L. Zuo. 2017. “Customer–Supplier Relationships and Corporate Tax Avoidance.” Journal of Financial Economics 123 (2): 377–394. doi:10.1016/j.jfineco.2016.09.009.
- Chang, C., X. Chen, and G. Liao. 2014. “What are the Reliably Important Determinants of Capital Structure in China?” Pacific-Basin Finance Journal 30: 87–113. doi:10.1016/j.pacfin.2014.06.001.
- Chang, Y. -C., H. G. Hong, L. Tiedens, N. Wang, and B. Zhao 2015. Does Diversity Lead to Diverse Opinions? Evidence from Languages and Stock Markets. Rock Center for Corporate Governance at Stanford University Working Paper(168), 13–16.
- Choi, T. Y., and D. R. Krause. 2006. “The Supply Base and Its Complexity: Implications for Transaction Costs, Risks, Responsiveness, and Innovation.” Journal of Operations Management 24 (5): 637–652. doi:10.1016/j.jom.2005.07.002.
- Chung, H., S. Choi, and W. -O. Jung. 2019. “Controlling shareholders’ Tax Incentives and Related Party Transactions.” Pacific-Basin Finance Journal 57: 101181. doi:10.1016/j.pacfin.2019.101181.
- Chu, Y., X. Tian, and W. Wang. 2019. “Corporate Innovation Along the Supply Chain.” Management Science 65 (6): 2445–2945. doi:10.1287/mnsc.2017.2924.
- Cull, R., L. C. Xu, X. Yang, L. A. Zhou, and T. Zhu. 2017. “Market Facilitation by Local Government and Firm Efficiency: Evidence from China.” Journal of Corporate Finance 42: 460–480.
- Cull, R., L. C. Xu, and T. Zhu. 2009. “Formal Finance and Trade Credit During China’s Transition.” Journal of Financial Intermediation 18 (2): 173–192. doi:10.1016/j.jfi.2008.08.004.
- Dass, N., J. R. Kale, and V. Nanda. 2014. “Trade Credit, Relationship-Specific Investment, and Product Market Power.” Review of Finance 19 (5): 1867–1923. doi:10.1093/rof/rfu038.
- Degryse, H., and S. Ongena. 2005. “Distance, Lending Relationships, and Competition.” The Journal of Finance 60 (1): 231–266. doi:10.1111/j.1540-6261.2005.00729.x.
- Diamond, D. W. 1984. “Financial Intermediation and Delegated Monitoring.” The Review of Economic Studies 51 (3): 393–414. doi:10.2307/2297430.
- Diamond, D. W. 1991. “Debt Maturity Structure and Liquidity Risk.” The Quarterly Journal of Economics 106 (3): 709–737. doi:10.2307/2937924.
- Elmaghraby, W. J. 2000. “Supply Contract Competition and Sourcing Policies.” Manufacturing & Service Operations Management 2 (4): 350–371. doi:10.1287/msom.2.4.350.12340.
- Fabbri, D., and L. F. Klapper. 2016. “Bargaining Power and Trade Credit.” Journal of Corporate Finance 41: 66–80. doi:10.1016/j.jcorpfin.2016.07.001.
- Fan, G., X. Wang, and H. Zhu 2011. The 2011 Report on the Relative Process of Marketization of Each Region in China. The Economic Science Press Beijing, China.
- Ferris, J. S. 1981. “A Transactions Theory of Trade Credit Use.” The Quarterly Journal of Economics 96 (2): 243–270. doi:10.2307/1882390.
- Fisman, R., and I. Love. 2003. “Trade Credit, Financial Intermediary Development, and Industry Growth.” The Journal of Finance 58 (1): 353–374. doi:10.1111/1540-6261.00527.
- Fisman, R., and M. Raturi. 2004. “Does Competition Encourage Credit Provision? Evidence from African Trade Credit Relationships.” Review of Economics & Statistics 86 (1): 345–352. doi:10.1162/003465304323023859.
- Giroud, X. 2013. “Proximity and Investment: Evidence from Plant-Level Data.” The Quarterly Journal of Economics 128 (2): 861–915. doi:10.1093/qje/qjs073.
- Gosman, M., T. Kelly, P. Olsson, and T. Warfield. 2004. “The Profitability and Pricing of Major Customers.” Review of Accounting Studies 9 (1): 117–139. doi:10.1023/B:RAST.0000013631.48714.c1.
- Gosman, M. L., and M. J. Kohlbeck. 2009. “Effects of the Existence and Identity of Major Customers on Supplier Profitability: Is Wal-Mart Different?” Journal of Management Accounting Research 21 (1): 179–201. doi:10.2308/jmar.2009.21.1.179.
- Huang, Y., and Y. Fan. 2022. “Risk Along the Supply Chain: Geographic Proximity and Corporate Risk Taking.” Finance Research Letters 50: 103150. doi:10.1016/j.frl.2022.103150.
- Huang, H. H., G. J. Lobo, C. Wang, and H. Xie. 2016. “Customer Concentration and Corporate Tax Avoidance.” Journal of Banking & Finance 72: 184–200. doi:10.1016/j.jbankfin.2016.07.018.
- Hui, K. W., S. Klasa, and P. E. Yeung. 2012. “Corporate Suppliers and Customers and Accounting Conservatism.” Journal of Accounting & Economics 53 (1–2): 115–135. doi:10.1016/j.jacceco.2011.11.007.
- Huo, B. F., X. D. Zhao, and H. G. Zhou. 2014. “The Effects of Competitive Environment on Supply Chain Information Sharing and Performance: An Empirical Study in China.” Production and Operations Management 23 (4): 552–569. doi:10.1111/poms.12044.
- Janet, S. 1987. “Trade Credit and Informational Asymmetry.” The Journal of Finance 42 (4): 863–872. doi:10.1111/j.1540-6261.1987.tb03916.x.
- Jhang, S. -S. -S., H. -C. Su, and T. -W.D. Kao. 2021. “Major Customer Network Structure and Supplier Trade Credit.” International Journal of Operations & Production Management 41 (8): 1318–1349. doi:10.1108/IJOPM-05-2020-0278.
- Jia, N., and K. J. Mayer. 2017. “Political Hazards and firms’ Geographic Concentration.” Strategic Management Journal 38 (2): 203–231. doi:10.1002/smj.2474.
- Jory, S. R., H. D. Khieu, T. N. Ngo, and H. V. Phan. 2020. “The Influence of Economic Policy Uncertainty on Corporate Trade Credit and Firm Value.” Journal of Corporate Finance 64: 101671. doi:10.1016/j.jcorpfin.2020.101671.
- Kalwani, M. U., and N. Narayandas. 1995. “Long-Term Manufacturer-Supplier Relationships: Do They Pay off for Supplier Firms?” Journal of Marketing 59 (1): 1–16. doi:10.1177/002224299505900101.
- Kang, J. K., and J. M. Kim. 2008. “The Geography of Block Acquisitions.” The Journal of Finance 63 (6): 2817–2858. doi:10.1111/j.1540-6261.2008.01414.x.
- Kekre, S., B. Murthi, and K. Srinivasan. 1995. “Operating Decisions, Supplier Availability and Quality: An Empirical Study.” Journal of Operations Management 12 (3–4): 387–396. doi:10.1016/0272-6963(95)00002-A.
- Klapper, L., L. Laeven, and R. Rajan. 2010. “Trade Credit Contracts.” The Review of Financial Studies 25 (3): 838–867. doi:10.1093/rfs/hhr122.
- Knyazeva, A., and D. Knyazeva. 2012. “Does Being Your Bank’s Neighbor Matter?” Journal of Banking & Finance 36 (4): 1194–1209. doi:10.1016/j.jbankfin.2011.11.011.
- Krishnan, G., P. Patatoukas, and A. Wang. 2019. “Customer-Base Concentration: Implications for Audit Pricing and Quality.“ Journal of Management Accounting Research 31 (1): 129–152. doi:10.2308/jmar-52040.
- Lawrenz, J., and J. Oberndorfer. 2018. “Firm Size Effects in Trade Credit Supply and Demand.” Journal of Banking & Finance 93: 1–20. doi:10.1016/j.jbankfin.2018.05.014.
- Long, M. S., I. B. Malitz, and S. A. J. F. M. Ravid. 1993. “Trade Credit, Quality Guarantees, and Product Marketability.” Financial Management 22 (4): 117–127. doi:10.2307/3665582.
- Love, I., L. A. Preve, and V. Sarria-Allende. 2007. “Trade Credit and Bank Credit: Evidence from Recent Financial Crises.” Journal of Financial Economics 83 (2): 453–469. doi:10.1016/j.jfineco.2005.11.002.
- Luo, S. Q., and N. J. Nagarajan. 2015. “Information Complementarities and Supply Chain Analysts.” The Accounting Review 90 (5): 1995–2029. doi:10.2308/accr-51011.
- Maksimovic, V., and S. Titman. 1991. “Financial Policy and Reputation for Product Quality.” The Review of Financial Studies 4 (1): 175–200. doi:10.1093/rfs/4.1.175.
- McKinsey. 2010. “Managing Global Supply Chains.” The McKinsey Quarterly July: 2–9.
- Meyners, J., C. Barrot, J. U. Becker, and J. Goldenberg. 2017. “The Role of Mere Closeness: How Geographic Proximity Affects Social Influence.” Journal of Marketing 81 (5): 49–66. doi:10.1509/jm.16.0057.
- Nodari, G. 2014. “Financial Regulation Policy Uncertainty and Credit Spreads in the US.” Journal of Macroeconomics 41: 122–132. doi:10.1016/j.jmacro.2014.05.006.
- North, D. C., and D. C. North. 1992. Transaction Costs, Institutions, and Economic Performance. San Francisco, CA: ICS Press.
- Oh, S., and W. S. Kim. 2016. “Growth Opportunities and Trade Credit: Evidence from Chinese Listed Firms.” Applied Economics 48 (56): 5437–5447. doi:10.1080/00036846.2016.1178846.
- Pastor, L., and P. Veronesi. 2012. “Uncertainty About Government Policy and Stock Prices.” The Journal of Finance 67 (4): 1219–1264. doi:10.1111/j.1540-6261.2012.01746.x.
- Patatoukas, P. N. 2012. “Customer-Base Concentration: Implications for Firm Performance and Capital Markets: 2011 American Accounting Association Competitive Manuscript Award Winner.” The Accounting Review 87 (2): 363–392. doi:10.2308/accr-10198.
- Paul, S., and R. Boden. 2008. “The Secret Life of UK Trade Credit Supply: Setting a New Research Agenda.” The British Accounting Review 40 (3): 272–281. doi:10.1016/j.bar.2008.05.007.
- Petersen, M. A., and R. G. Rajan. 1997. “Trade Credit: Theories and Evidence.” The Review of Financial Studies 10 (3): 661–691. doi:10.1093/rfs/10.3.661.
- Petersen, M. A., and R. G. Rajan. 2002. “Does Distance Still Matter? The Information Revolution in Small Business Lending.” The Journal of Finance 57 (6): 2533–2570. doi:10.1111/1540-6261.00505.
- Piercy, N., and N. Lane. 2006. “The Underlying Vulnerabilities in Key Account Management Strategies.” European Management Journal 24 (2): 151–162. doi:10.1016/j.emj.2006.03.005.
- Porter, M. E. 1974. “Consumer Behavior, Retailer Power and Market Performance in Consumer Goods Industries.” The Review of Economics and Statistics 56 (4): 419–436. doi:10.2307/1924458.
- Raman, K., and H. Shahrur. 2008. “Relationship-Specific Investments and Earnings Management: Evidence on Corporate Suppliers and Customers.” The Accounting Review 83 (4): 1041–1081. doi:10.2308/accr.2008.83.4.1041.
- Rui, H., and G. Lai. 2015. “Sourcing with Deferred Payment and Inspection Under Supplier Product Adulteration Risk.” Production and Operations Management 24 (6): 934–946. doi:10.1111/poms.12313.
- Shenoy, J., and R. Williams. 2017. “Trade Credit and the Joint Effects of Supplier and Customer Financial Characteristics.” Journal of Financial Intermediation 29: 68–80. doi:10.1016/j.jfi.2015.09.001.
- Tosun, M. S., and S. Yildiz. 2022. “The Relationship Between Tax Uncertainty and Trade Credit: Firm-Level Evidence from the United States.” Applied Economics 54 (15): 1742–1758. doi:10.1080/00036846.2021.1982129.
- Wen, W., Y. Ke, and X. Liu. 2021. “Customer Concentration and Corporate Social Responsibility Performance: Evidence from China.” Emerging Markets Review 46: 100755. doi:10.1016/j.ememar.2020.100755.
- Wen, X., J. Xia, and Y. Ye. 2020. “Customer Stability, Managerial Overconfidence, and Enterprise Investment in Innovation: A Perspective Based on “Made in China 2025”.” Asia-Pacific Journal of Accounting & Economics 29 (3): 1–19. doi:10.1080/16081625.2020.1754249.
- Wilson, N., and B. Summers. 2002. “Trade Credit Terms Offered by Small Firms: Survey Evidence and Empirical Analysis.” Journal of Business Finance & Accounting 29 (3–4): 317–351. doi:10.1111/1468-5957.00434.
- Yang, S. A., and J. R. Birge. 2018. “Trade Credit, Risk Sharing, and Inventory Financing Portfolios.” Management Science 64 (8): 3667–3689. doi:10.1287/mnsc.2017.2799.
- You, J., B. Zhang, and L. Zhang. 2017. “Who Captures the Power of the Pen?” The Review of Financial Studies 31 (1): 43–96. doi:10.1093/rfs/hhx055.