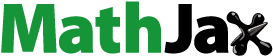
ABSTRACT
In this study, a three-dimensional computational fluid dynamics (CFD) model is employed to investigate the hydrodynamic and combustion characteristics of biomass particles in an industrial-scale circulating fluidized bed (CFB) furnace. The CFD model considered here is based on the Eulerian-Lagrangian framework, the multi-phase particle-in-cell (MP-PIC) collision model, the coarse grain method (CGM), and a recently developed distribution kernel method (DKM). The challenge of simulating industrial-scale CFB furnaces using CFD lies in the large number of particles in the system. MP-PIC and CGM showed that local particle overloading could occur, causing the numerical simulation to diverge. The combination of MP-PIC with CGM and DKM was shown to overcome this problem. The CFD predictions werecompared with onsite temperature experiments in the furnace, and the predicted furnace temperature agreed fairly well with the measured data. Using the CFD results, the study analyzed the transient solids mixing and fluidization characteristics, as well as the thermochemical process in biomass combustion. The simulated individual particle provided insight into the physical and chemical processes of the granular flow in the dilute/dense regions of the CFB furnace. The simulated results revealed the CO and NOx emission processes in the furnace.
Introduction
Biomass, which refers to all organic materials such as wood, agricultural residues, forestry residues, and energy crops, is a renewable energy source with the potential to substitute fossil fuels in the future (Chen et al. Citation2021; Di Blasi Citation2009). Direct burning of biomass is an important energy conversion technology for generating heat and power. Biomass combustion in circulating fluidized bed (CFB) furnaces is gaining attention due to its stable low-temperature combustion, high combustion efficiency, high fuel flexibility, and low environmental impact (Khan et al. Citation2009; Deng et al. Citation2021; Cai et al. Citation2018).
The physical and chemical processes that occur in industrial-scale CFB furnaces are complex and include granular motions, particle–particle and particle–wall collisions, heat and mass transfer, combustion and gasification of fuel particles, formation and emission of pollutants, etc. The CFB furnace is characterized by high fluidizing velocity, a large number of particles, complex flow structures, complex particle collisions, and a turbulent combustion process. Therefore, understanding the mechanisms of the transient hydrodynamic and combustion processes in CFB furnaces and developing efficient CFD simulation approaches are paramount to designing prototypes, scaling up furnaces, controlling the operating conditions, and optimizing the operating parameters.
Two main approaches, i.e., experimental measurement and numerical modeling, have been widely adopted to investigate granule-fluid flow and gasification/combustion processes in CFB furnaces. Numerous experimental studies have been conducted to investigate physical and chemical processes in industrial-scale CFB furnaces. Svensson, Johnsson, and Leckner (Citation1996), Leckner (Citation2017), and Johnsson et al. (Citation2000) investigated the characterization of fluidization regimes using time-series analysis of pressure fluctuations. The method was demonstrated to be powerful in revealing the structure of cold granule-fluid flow at the macro level. Larsson et al. (Citation2021) investigated the thermochemical conversion of solid fuels by steam gasification in different dual fluidized beds (DFBs), showing a strong correlation between the availability of active components in the reaction environment and the quality of the product gas. Kolbitsch et al. (Citation2010) investigated H, CO, and CH
conversion processes in two different oxygen carriers in a 120 kW dual circulating fluidized bed (DCFB) reactor. A natural oxygen carrier, i.e., ilmenite, was shown to improve the conversion efficiency compared to a fabricated Ni-based oxygen carrier. Vainio et al. conducted an experiment on the fate of fuel nitrogen in the furnace of a full-scale bubbling fluidized bed boiler (Vainio et al. Citation2012), measuring and analyzing the main components of nitrogen species at various height levels in detail. Based on the experimental research on the large-scale fluidized bed, the experimental research focuses more on the phenomena and mechanism of the granule-fluid flow at the furnace level. Compared to the experimental approach, the simulation approach is considered to be a more efficient, economical, and robust method to investigate hydrodynamic and combustion processes at multiple space-time scales (Alobaid et al. Citation2021).
summarizes the recent work on the numerical modeling of dense granule-fluid systems, predicting physical and chemical processes, e.g., gas–solid hydrodynamics, heat and transfer, and gasification/combustion. It can be seen that one-dimensional (1-D) and two-dimensional (2-D) simulations have been frequently conducted (Blaszczuk, Zylka, and Leszczynski Citation2016; Collado Citation2018; Deng et al. Citation2021; Dinh et al. Citation2021; Lu et al. Citation2018), taking into account relatively detailed physical and chemical processes of the gas and particle phases. The 1-D and 2-D models have the advantages of high computational efficiency, easy implementation, and flexible application. However, they only consider the variation of the physical parameters in the vertical direction of furnaces, while assuming a uniform distribution of the physical parameters along one horizontal direction (Deng et al. Citation2021). Three-dimensional (3-D) simulations, as shown in , have been used to study lab-scale fluidized bed furnaces, non-reactive two-phase flow, or reactive flows without taking into account fully granular collisions. However, 3-D CFD simulations of industrial-scale fluidized bed furnaces that take into account the full details of granular motions and thermochemical processes of the particle and gas phases are desirable but rarely performed. There are several challenges in 3-D modeling the solid fuel combustion process in industrial-scale CFB furnaces, such as a huge number of particles resulting in expensive computational cost, large particles in small-size grids resulting in numerical instability, and complex chemical kinetic mechanisms involved in the devolatilization, heterogeneous char reactions, and homogeneous gas-phase reactions.
Table 1. CFD simulations of FBs. D represent dimensional, H- Hydrodynamic, T- thermol, R-gasification/combustion.
Current 3-D CFD approaches for modeling particle flow under CFB furnace conditions can be classified into two categories: the Euler–Euler approach, e.g., the two-fluid model (TFM) (Anderson and Jackson Citation1967), and the Eulerian-Lagrangian approach, e.g., the discrete element method (DEM) (Tsuji, Kawaguchi, and Tanaka Citation1993) and multi-phase particle-in-cell (MP-PIC) approaches (Gidaspow Citation1994). The TFM heavily relies on the constitutive or closure relations for the source terms that describe the exchange between the gas and solid phases. The solid phase is treated as a different phase when different types of particles are present (Zhou et al. Citation2010), making it challenging to model complex solid-phase motion and collision processes with acceptable accuracy. The DEM accurately models granular collisions and tracks individual particles; however, modeling the collisions of quadrillions of particles on the individual particle level in an industrial-scale CFB furnace is impractical. The MP-PIC approach, which uses the kinetic theory of granular flow (KTGF) (Chapman, George, and Cowling Citation1990) to eliminate difficulties in calculating the inter-particle interaction, is considered an efficient and suitable method for simulating industrial-scale CFBs (Snider Citation2001). Additionally, the computational cost of simulating large-scale CFB furnaces can be further reduced with the coarse grain method (CGM), which clusters a number of particles with similar physical locations and properties into a virtual parcel tracked in the Lagrangian framework (Hilton and Cleary Citation2014). Previous work on biomass gasification in a lab-scale fluidized bed employed the CGM coupling with the Eulerian-Lagrangian method (Qi et al. Citation2019). However, due to the complex structure of industrial CFB furnaces and the use of unstructured grids, grid refinement is necessary. The CGM approach can cause a local overloading issue where the volume of the solid exceeds its physically possible limit in some Eulerian cells, i.e., the volume of the solid is larger than that the local cell could accommodate. A recently developed distribution kernel method (DKM) (Yang et al. Citation2022) remedies this issue by spreading the solid volume and source terms of solid particles in the parcel to the Eulerian domain, improving the accuracy and robustness of the model.
To the best of the authors’ knowledge, few 3-D CFD simulations that take into account granular collisions and gas-solid phase coupling have been reported for investigating the hydrodynamic and combustion processes of biomass in an industrial-scale full-loop CFB furnace, cf. . In this study, a 3-D CFD model consisting of the MP-PIC collision model, CGM, and DKM, which considers gas/solid interactions, granular collisions, heat and mass transfer, radiation, and homogeneous and heterogeneous chemical reactions, was applied to investigate a biomass-fired industrial-scale CFB furnace. The objective is to improve the understanding of gas/solid two-phase flow and the thermochemical process of biomass in industrial-scale CFB furnaces.
Mathematical formulation
In the present model, the governing equations of the continuous and discrete phases involved in fluidized bed furnaces are described in the Eulerian and Lagrangian frameworks, respectively. The governing equations of the continuous and discrete phases and main sub-models involved in the 3-D model are described in the present study.
Governing equations for the continuous phase
Reynolds-averaged Navier–Stokes (RANS) approach is used to describe the mean gas flow in the FB reactors. The gas-phase governing equations consist of the Reynolds-averaged continuity, momentum, energy, and species transport equations (Zhou et al. Citation2010). The Reynolds averaged continuity equation is
where overbar and tilde denote Reynolds averaged and Favre averaged, respectively. ,
, and
are the gas volume fraction, the gas density, and the velocity vector of the gas phase, respectively.
represents the gas formation rate due to the thermochemical conversion of the fuel particles.
The Reynolds averaged momentum equations are
where is the gas pressure,
is the sum of viscous stress and Reynolds stress, and
is the source term of momentum exchange from the solid phase.
The Reynolds averaged energy equation is
where denotes the specific enthalpy of the gas, and
denotes the kinetic energy of the gas flow.
denotes the source term due to radiative heat transfer,
denotes the source term due to volatile chemical reactions, and
denotes the source term due to thermochemical conversion of the solid fuel. Heat diffusion coefficient
is the sum of the molecular and turbulent heat diffusion coefficients given by
where is the molecular heat diffusion coefficient,
is the turbulent Prandtl number and
is the turbulent eddy viscosity.
The Reynolds averaged species transport equation is
in which is the mass fraction of species
in the gas mixture, and
denotes the chemical reaction rate of species
.
denotes the formation rate of species
due to thermochemical conversion of solid fuel particles. The mass diffusion coefficient
for species
taking both the molecular and turbulent contributions into account and is given by
where is the mass diffusion coefficient for species
due to molecular contribution and
is the turbulent Schmidt number.
A Partially Stirred Reactor (PaSR) model is used to account for turbulence–chemistry interaction when computing the mean source terms due to gas-phase chemical reactions (,
) (Chomiak and Karlsson Citation1996). In the PaSR model, the mean reaction rates are modeled as follows:
in which is the gas temperature, and
is the volume fraction of the reactive mixture and given by,
where and
denote the local chemical reaction time and the local mixing time, respectively. The chemical reaction time,
, is determined from the mean reaction rates of the fuel
and the oxidizer or the gasification agents
,
where subscripts and
denote the fuel and oxidizer or the gasification agents, respectively. The mixing time
is modeled as
where is a model constant (
in this study).
and
denote the kinematic viscosity and the dissipation rate of turbulent kinetic energy, respectively.
The stress tensor in Eq. (2) is the sum of the viscous and Reynolds stresses and can be written as
The stress tensor for a Newtonian fluid is expressed as
and the Reynolds stress is modeled according to
Standard model is employed to determine the eddy viscosity,
where is the turbulent kinetic energy.
and
are modeled using the following transport equations:
where is the production rate of turbulent kinetic energy. Standard values of model constants are used,
= 0.09,
= 1.44,
= 1.92,
= 1.0 and
= 1.3 (Ku, Li, and Løvås Citation2015; Yan et al. Citation2016). The mean source terms are due to the particle/gas interaction in EquationEquations 1
(1)
(1) , Equation2
(2)
(2) , Equation3
(3)
(3) and Equation5
(5)
(5) ), i.e.,
,
,
and
, require the modeling of particle phase as discussed below.
Solid phase governing equations
In the Eulerian-Lagrangian approach, biomass and sand particles are tracked using the Lagrangian approach. The interactions between the particles and the surrounding gas are through mass and momentum exchange and heat transfer. The mass, momentum, and energy conservation equations for the solid phase in the Lagrangian framework are presented in the following. For simplicity, the Reynolds/Favre averaged gas properties are indicated without using over-bars or tildes.
Mass conversion of solid phase
Biomass particles undergo thermochemical conversion reactions, i.e., drying, pyrolysis, and the heterogeneous reaction of char, while sand particles are assumed to be chemically inert. The mass conservation equation for the -th biomass particle is written as
where ,
,
and
denote the mass of
-th biomass particle, the evaporation rate, the devolatilization rate, and the char conversion rate, respectively.
Drying
The moisture evaporation rate (Ku et al. Citation2014; Yan et al. Citation2016) is modeled as
where ,
, and
represent the molar flux of vapor, the surface area of the particle, and the molar weight of the vapor, respectively.
is given by
where ,
and
denote the mass transfer coefficient, vapor concentration at the particle surface, and the vapor concentration in the bulk gas, respectively.
,
and
can be described as
and
where is the Sherwood number modeled using Ranz–Marshall correlation (Ranz and Marshall Citation1952)
where is the Schmidt number of the surrounding gas and
is the Reynolds number of
th particle.
,
,
, and
represent the vapor diffusion coefficient, the saturation pressure, the gas temperature, and the molar fraction of vapor in the surrounding gas, respectively.
is the universal gas constant,
is the particle temperature, and
is an equivalent spherical particle diameter computed based on the particle real-time mass
and a constant particle density
and is given by
where is the particle density.
Pyrolysis
There are four different types of pyrolysis models reviewed by Hameed et al. (Citation2019), Vikram, Rosha, and Kumar (Citation2021), and Fatehi et al. (Citation2021), i.e., (a) single-step model, (b) three-parallel-step model with secondary tar cracking reactions, (c) FG-DVC model, and (d) multicomponent pyrolysis model. In fluidized bed furnaces, especially large-scale industrial furnaces, the number of biomass particles is enormous. It would require tremendously long computational time to carry out numerical simulations of a 3-D fluidized bed furnace if multicomponent pyrolysis models were used. Three-parallel step model and FG-DVC model have some drawbacks, as they are developed based on specific experimental conditions. Therefore, using them to predict other experimental conditions may result in substantial experimental errors. Thus, single-step models have been employed in fluidized bed simulations (Gómez et al. Citation2014; Karim and Naser Citation2018; Ku, Li, and Løvås Citation2015; Luo et al. Citation2022, Citation2020; Qi et al. Citation2019; Wang et al. Citation2018; Yang et al. Citation2022; Yang et al. Citation2019; Zhou et al. Citation2006).
The rate of devolatilization is computed based on the pyrolysis reaction model,
where and
are rate constants (Ku, Li, and Løvås Citation2015),
is the mass of the volatile remaining in the particle.
A one-step pyrolysis model involving nitrogen conversion is written as
where C denotes char in the solid phase.
are the stoichiometric constants, i.e.,
= 0.5014,
= 0.0954,
= 0.0864,
= 0.0512,
= 0.1060,
= 0.0021,
= 0.0043,
= 0.0067,
= 0.0005, and
= 0.1458. In this model, volatile nitrogen-containing species released during the pyrolysis process include NH
, NO, HCN, and HNCO, whose release rates are proportional to biomass pyrolysis.
Biomass NO formation mechanism has been investigated for several decades. Winter, Wartha, and Hofbauer (Citation1999) investigated the NO
formation of different biomass fuels in a fluidized bed combustor and a grate furnace. NO, N
O, HCN, and NH
were measured in the flue gas shortly after biomass combustion, while N
O was rapidly converted to N
. HCN was formed in quantities similar to NH
during woody biomass combustion and the HCN/NH
ratios depend on the H/N ratio in biomass fuels. According to the measurements of Bassilakis, Carangelo, and Wojtowicz (Citation2001) and Hansson et al. (Citation2004), HNCO is a significant intermediate product for NO
formation during biomass combustion. In the study of Bassilakis, Carangelo, and Wojtowicz (Citation2001), the mass ratios (dry basis) of NH
/HCN/HNCO at a heating rate of 30 K/min are 37/43/20 for wheat straw and 35/26/39 for tobacco, respectively. Hansson et al. (Citation2004) reported that the mass ratios (dry basis) of NH
/HCN/HNCO are 57/28/15 at a pyrolysis temperature 973 K and 31/60/9 at 1273 K, respectively. According to studies by Leppalahti and Koljonen (Citation1995) and Weissinger, Fleckl, and Obernberger (Citation2004), NH
is the main nitrogen-containing intermediate product during biomass pyrolysis. Zhou et al. (Citation2006) showed that up to 1–4% of nitrogen is directly converted to NO during biomass pyrolysis. Despite numerous studies on NO
formation in biomass combustion, there is no general consensus on the ratio of NH
/HCN/HNCO/NO in the published literature. Based on the above studies, the components containing nitrogen in the pyrolysis products are NH
, HCN, HNCO, and NO in descending order. NH
is the main component of pyrolysis nitrogenous products, while the ratio of HCN/HNCO is approximately 2. The mass ratio of NH
/HCN/HNCO/NO during biomass pyrolysis is estimated to be 51/31/15/3 in the present work. This ratio is used to determine the model constants
in EquationEquation (26)
(26)
(26) .
Char conversion
Char conversion is a complex process in which chemical reactions occur at the surface of the porous medium structure with complex interior and microstructures. The heterogeneous rates of char conversion are affected by the fundamental components, e.g., surface area, surface accessibility, carbon active sites, added inorganic matter, and the gaseous reactant concentration (Di Blasi Citation2009). The rate of char conversion is computed based on all heterogeneous reactions,
where represent the char consumption rates by reactions with O
, H
O, and CO
, respectively.
where denotes the particle surface, and
represents the partial pressure of the gasifying agents or oxidizers in the gas surrounding the particle. A normalized Damköhler number
, which is the ratio of the kinetic reaction rate to the mass transport rate (Hazenberg and van Oijen Citation2021), is defined to take into account the contribution of the kinetic and the diffusion rates,
where and
represent, respectively, the diffusion rate coefficient and kinetic rate coefficient.
and
are defined as follows:
and
where and
represent the pre-exponential factor and activation energy for the char gasification reactions, respectively.
is the mass diffusion rate constant and
(s/K
) (Ku, Li, and Løvås Citation2015).
Simplified homogeneous reactions of volatile gas and heterogeneous reactions of char used in this study are listed in . In this model, thermal NO formation is neglected because the maximum temperature in the furnace is lower than 1600 K, i.e., fuel-NO from nitrogen in the biomass is the main source of NO
formation. This chemical kinetic model is selected mainly due to its high computational efficiency. The NO chemistry (R9–R16) has been used by H. Zhou et al. (Citation2006) to predict NO formation in straw combustion in a fixed bed furnace showing good accuracy.
Table 2. Homogeneous and heterogeneous reactions considered in biomass combustion and gasification. Note: C is solid phase char.
represents the molar concentration of gas species
. References for each reaction: R1 (Yan et al. Citation2016, Citation2018), R2 (Yan et al. Citation2016, Citation2018), R3 (Yan et al. Citation2016, Citation2018), R4 (Yan et al. Citation2016, Citation2018), R5 (Yan et al. Citation2016, Citation2018), R6 (Yan et al. Citation2016, Citation2018), R7 (Brink, Kilpinen, and Hupa Citation2001), R8 (Brink, Kilpinen, and Hupa Citation2001), R9 (Zhou et al. Citation2006), R10 (Zhou et al. Citation2006; Ma et al. Citation2021), R11 (Zhou et al. Citation2006; Ma et al. Citation2021), R12 (Ma et al. Citation2021; Zhou et al. Citation2006), R13 (Ma et al. Citation2021; Zhou et al. Citation2006), R14 (Ma et al. Citation2021; Zhou et al. Citation2006), R15 (Ma et al. Citation2021; Zhou et al. Citation2006), R16 (Ma et al. Citation2021; Zhou et al. Citation2006), R17 (Nikoo and Mahinpey Citation2008; Yang et al. Citation2019), R18 (Nikoo and Mahinpey Citation2008; Yang et al. Citation2019), R19 (Nikoo and Mahinpey Citation2008; Yang et al. Citation2019.).
Momentum equation of solid phase
The velocity of the -th particle
is governed by Newton’s second law,
The right-hand side terms represent the sum of all forces acting on the -th particle by the surrounding gas and particles. The forces considered include, from left to right, the drag
, pressure gradient
, gravity
, and interparticle stress
. With a given
, the position vector of the particle
is computed by integration of the equation
Drag model
The drag force model widely used for the -th individual particle
is given by (Gidaspow Citation1994; Ku, Li, and Løvås Citation2015; Yang et al. Citation2019)
where is the volume of the computational cell and
is the drag force parameter, which is modeled using the Wen & Yu drag correlation (Gidaspow Citation1994; Wen Citation1966) and is given as
where is the gas-phase volume fraction, and the drag coefficient
is modeled as (Gidaspow Citation1994)
where the particle Reynolds number is defined as
Interparticle stress
The particle stress is given by
where the contact normal stress can be given by the model of Lun et al. (Citation1984),
where ,
, and
represent, respectively, the radial distribution function, the mean density of particles in a local cell, and the coefficient of restitution.
is the granular temperature, and
is the radial distribution function.
The solid volume fraction of particles satisfies
and is modeled based on the particle distribution function
and can be given by
where and
denote the particle velocity and particle mass, respectively. The particle velocity
is the particle velocity in the Eulerian frame, which is different from
in EquationEquation (32)
(32)
(32) that represents the velocity of the
-th particle in the Lagrangian framework. This also applies to the distinctions between solid-phase parameters with subscripts of
and
.
In the MP-PIC model, is obtained from the Liouville equation, which is a mathematical expression of the conservation of particle numbers per volume moving along dynamic trajectories in the particle-phase space (Andrews and O’Rourke Citation1996),
The first term in the RHS of Eq. (41) denotes the collision return-to-isotropy effect and the second term denotes the collision damping effect. Physically, particle collision tends to dampen out the velocity fluctuations. The collision model assumed that within a damping relaxation time, the particle velocity approaches a mean value and the distribution function approaches
. The collision-damping particle distribution function
is given by (O’Rourke and Snider Citation2010, Citation2012)
where is the Dirac function. The mean value of particle velocity can be given by
The particle collision could result in a Gaussian distribution of particle velocity occurring within a relaxation time . The Gaussian distribution is described by the equilibrium-isotropic particle distribution function
,
where is a Gaussian velocity distribution with the mean
and variance
, which can be obtained by enforcing that the variance of
is equal to that of
.
Energy equation of solid phase
The particle temperature is obtained from the energy conservation equation for the -th particle,
where ,
and
denote, respectively, the particle heat capacity and convective and radiative heat transfer.
,
and
represent the heat transfer of latent, pyrolysis, and char reactions.
The convection heat and radiation
are given by
and
where ,
,
, and
represent interphase thermal transfer coefficient, emissivity, Stefan-Boltzmann constant, and incident radiation, respectively. The interphase thermal transfer coefficient
can be given
where is the thermal conductivity of the surrounding gas.
is the Nusselt number computed using the Ranz-Marshall correlation given by
where is the Prandtl number of the surrounding gas. The incident radiation
is obtained from the P-1 radiation model.
The heat fluxes due to evaporation, pyrolysis, and char reactions are, respectively, ,
and
, and can be given by
and
where ,
, and
represent the latent heat, the heat of pyrolysis, and the heat of char reactions, respectively.
Numerical methods and computation cases
Solution procedure for solid-phase governing equations
In order to achieve a converged statistical solution in the MP-PIC method, a sufficiently large number of particles need to be simulated. This is not an issue in fluidized bed reactors since there is a sufficiently large number of particles. In practical simulations, not all these particles could be simulated. A coarse grain method (CGM) is employed in this study to reduce the computational cost. In the CGM approach, a finite number of virtual particles (hereafter referred to as parcels) are simulated. Assume that the number of parcels is . The
-th parcel contains multiple real particles; however, all particles have the same properties, i.e., each real particle in the
-th parcel has the same mass
, velocity
, temperature
and diameter
.
The governing equations for the individual real particles in the -th parcel are presented in subsection 2.2. These equations are integrated to compute the particle quantities, i.e.,
,
,
, and
. Implicit backward Euler scheme is used in the temporal integration of these equations.
As an example, the velocity of the -th particles is obtained by integrating Eq. (32). The discrete form of the velocity equation for the
-th particles can be written as (O’Rourke and Snider Citation2012).
where superscript denotes the quantities at time
and
is the time step. The first term on the RHS is the drag term, and the second term
is the sum of source terms due to the pressure gradient force, gravity, and interparticle stress. The last term is explicitly added to model the effect of the collision damping term in the Liouville Equationequation (41)
(41)
(41) , where
is the mass-weighted average of
(O’Rourke and Snider Citation2012).
In this discrete form of particle velocity equation, is the gas velocity at (
), which is computed from the gas velocity in the Euler grid around the particle position
at time
. A trilinear interpolation scheme is used to interpolate the Eulerian field quantities defined in the Euler cells to the discrete Lagrangian particle location
. illustrates the interpolation procedure.
Once the mass, velocity, temperature, and position are computed for all particles, the solid volume fraction (which is an Euler field quantity) can be computed from the ensemble average of the particles. Assume that the number of real particles per unit volume that pertains to the -th parcel is
. The solid volume fraction at (
) is
where is the volume of the
-th particle.
is the location of the
-th particle, whereas
is the trilinear interpolation function that computes the Euler field properties at
from the Lagrangian quantities at
.
The source terms due to the gas/solid interaction for the continuity equation, momentum equations, enthalpy equation, and species transport equations are computed similarly,
where is the heat exchange rate from solid particle,
is the sum of the drag force and the pressure gradient force, and
is due to pyrolysis and char reactions.
shows the solution procedure of the MP-PIC model. In this figure, and
are the Euler cell centers. Black and yellow particles denote the biomass and sand particles, respectively. The solution procedure involves the following four steps (the order of steps is not the order of execution in the CFD code):
(1) As shown in , the Euler field quantities in cell
and
, e.g., the solid volume fraction and the source terms of the gas phase equations due to the particles, are computed from the Lagrangian particles in the cells.
(2) As shown in , the gas phase transport equations are numerically solved using the finite-volume method described in subsection 2.1.
(3) As shown in , the Euler field quantities in cell
and
, e.g.,
, is interpolated to the position of the
-th Lagrangian particles, i.e., to compute
in EquationEquation (53)
(53)
(53) .
(4) As shown in , the properties of the Lagrangian particles are computed by temporal integration of the particle phase equations, using the method described earlier.
In the CGM-PCM approach, a large number of parcels in a small cell contribute to large source terms and local overloading of solids, i.e., the solid volume fraction () is larger than the physically allowable value, e.g.,
(Sun and Xiao Citation2015; Yang et al. Citation2022). Since
, a large
leads to a small
. Too large source terms and too small
can result in numerical instability of the gas phase governing equations. Thus, a threshold in the CFD solver is often employed, e.g., when
,
is set to the value of 0.62. The use of such a threshold can result in mass loss of the solid phase in the gas-phase governing equations in PCM (due to the increased
). Hence, a distribution kernel method (DKM) is developed to address this issue, as well as the cell searching strategy and parallel computation method for the DKM.
Spatial redistribution of parcels and source terms
The distribution kernel method (DKM) model was proposed and validated in our previous work (Yang et al. Citation2022, Citation2023), providing a detailed description of the method. A filtering kernel function was introduced to redistribute the source terms to surrounding cells containing the parcel based on,
where represents redistributed source terms,
,
,
,
, and the solid phase volume fraction
. A distance of distribution (
) is prescribed, within which the solid volume fraction and source terms will be redistributed from the position of the centroid of the local cell (
). A simple filtering kernel function
is employed,
The filtering kernel function is then obtained from the normalization of
,
satisfying,
Numerical scheme
The governing equations are numerically solved using an open-source CFD code, Open-FOAM v6 (Weller et al. Citation1998). The MP-PIC collision model was implemented for the discrete phase, and the DKM model was implemented for the coupled source terms. To facilitate source term redistribution, an efficient cell search algorithm was implemented, and a message-passing interface (MPI) strategy was developed for the parallel computation using DKM. The finite volume method (FVM) was used to solve the governing equations of the continuous phase, with spatial derivatives calculated using second-order “Gauss-Limited linear” schemes and temporal integration using first-order Euler schemes. Velocity-pressure coupling in the continuity and momentum equations was performed using the PIMPLE algorithm, which combines the advantages of the PISO (Pressure Implicit with Splitting of Operator) and SIMPLE (Semi-Implicit Method for Pressure-Linked Equations) algorithms. A semi-implicit algorithm was employed to handle source terms between the solid phase and gas phase.
Computational cases
The CFD model was applied to simulate the granular flow and combustion process in an industrial-scale biomass-fired CFB furnace, which has a thermal power output of 110 MW. A schematic illustration of the furnace is shown in , with only half of the computational domain displayed due to the symmetry of the geometry.
Figure 2. Computational domain of the 110 MWth industrial-scale CFB furnace. Only half of the computational domain is shown due to the symmetry of the furnace geometry.

The furnace has a height of 30 m (direction), a width of 8.7 m (
direction), and a depth of 5.4 m (
direction). The primary air inlet, located at the bottom of the furnace, was rectangular with a cross-section of 8.7 m
2.4 m, while the eight secondary air inlets were circular with a diameter of 0.4 m for the lower row and 0.475 m for the upper row at a height of
m and
m, respectively, as shown in . The combustion chamber occupied the upper portion of the furnace, with a height of up to 28 m. The fluidized bed region containing sand particles was located in the lower part of the combustion chamber and had a height of 6.5 m. Above that was the region for volatile gas and char combustion, which had a height of 20 m and a cross-section of 5.4 m
8.7 m. The cylindrical cyclones, which had a height of 9.25 m and a diameter of 4.0 m, were connected to the furnace via two 0.5 m diameter pipes for circulating solid particles. Finally, flue gas was directed to the top-box above the furnace and flowed out through an outlet region as indicated in .
The proximate analysis data and physical properties of the initial biomass and sand particles are presented in . The biomass is a mixture of waste wood, wood chips, sawdust, and bark with a mass ratio of 6 to 3 to 2 to 1. At the start of the simulation, a total mass of 60,000 kg of sand particles was fed to the furnace, while the biomass was supplied from the secondary air inlets at the lower row nozzles at a mass injection rate of 12.7 kg/s. The properties of the sand and biomass particles, including size distribution described by the Rosin-Rammler distribution function, are also shown in . Although the sand particles are chemically inert, their temperature varies in space and time. The biomass particles are modeled as having constant density, but their size changes during the thermochemical conversion process, and they are removed from the computational domain once burned out.
Table 3. Initial biomass and sand particles used in the CFB furnace.
Regarding boundary conditions, the computational domain’s outlet boundary (located on the left surface of the top-box) is set as a fixed-pressure boundary condition, with a zero-gradient condition assumed for other variables. The air inflow boundary is prescribed with Dirichlet boundary conditions, with the inlet flow velocity computed from the mass-flow rate condition. The wall boundary is non-slip and maintained at a constant temperature of 1173 K. A CFL number of 0.2 is used in the gas phase flow calculations.
The CFD mesh was generated using the ANSYS Workbench V17.2 package. The fluidization gas from the primary and secondary air inlets had a temperature of 200 , with a mass flow rate of 15.21 m
/s and 22.8 m
/s, respectively. To evaluate mesh independence, three sets of unstructured grids were used: a fine mesh with 604,634 cells, 988,551 sand parcels, and 100,000 biomass parcels; a medium-mesh with 512,286 cells, 743,568 sand parcels, and 80,000 biomass parcels; and a coarse mesh with 413,541 cells, 497,781 sand parcels, and 50,000 biomass parcels. The simulation results obtained using these mesh resolutions did not show any systematic difference nor any significant difference in cost.
compares the mean gas temperature along the centerline of the furnace, i.e., along direction at
and
(). It is shown that the results from the three meshes are rather similar, with the results from the fine mesh and the medium mesh showing closer agreement. The results from the fine mesh are discussed in the following.
The gas temperature in the combustion furnace is measured using thermocouples at 20 monitoring locations. The coordinates of the monitoring locations are presented in . The origin of the coordinate is at the center of the primary air inlet as shown in .
Table 4. Locations of temperature measurement points in the furnace. and
represent the two horizontal coordinates and
represents the vertical coordinate, see .
Results and discussion
The MP-PIC and CGM methods were initially used to simulate the granular flow and combustion process. This method is also known as the particle centroid method (PCM) since source terms in a mesh cell are calculated from all parcels in the cell. However, the numerical simulation was found to be unstable, and no converged solution could be obtained. In contrast, the MP-PIC with DKM resulted in a stable numerical solution. Therefore, the following discussion will first address the local overloading problem in PCM and DKM.
Performance of PCM and DKM
Particle local overloading can occur when the solid volume fraction, , exceeds the physical packing limit in a mesh cell. The physical packing limit refers to the maximum solid volume fraction that a local cell can accommodate. For spherical particles, this limit is approximately 0.62 (Sun and Xiao Citation2015; Yang et al. Citation2022), meaning that the lowest gas volume fraction value in a cell is 0.38. presents the distribution of gas volume fraction
with and without the use of DKM, as well as the local particle load
without DKM.
Figure 4. Distribution of gas volume fraction and particle load
at the different heights of the furnace (
2, 3 and 4 m), predicted using PCM and DKM.

where and
denote respectively the cell volume and the solid phase volume in the local cell. For the furnace height between
= 2
4 m, where particles are densely concentrated, local overloading is clearly evident. Without DKM (termed as PCM), the local particle load (
) can reach as high as 0.94 at
m. In this case, the gas volume fraction is as low as 0.17, which is below the physical limit of 0.38. In order to maintain numerical stability and avoid nonphysical solutions in the OpenFOAM solver, a numerical limiter is applied. Specifically, when
, it is set to the value of 0.38. In the simulation with PCM, a large portion of the domain has this limiter applied. In the simulation with DKM, the region requiring the limiter is significantly smaller, especially at higher furnace height. The source terms used in the PCM method are rather “noisy,” meaning they are similar to the distribution of
and
. This is likely the reason why the numerical simulation could not converge. Additionally, the numerical limiter applied to
causes it to be numerically increased, which can lead to errors in the numerical solution (Yang et al. Citation2022).
compares the gas temperature at different locations obtained from numerical simulations using the MP-PIC and CGM/DKM models. lists the locations P1–P6 at the fuel-supplying region with m, where sand and biomass particles exchange heat, and the biomass particles initiate the thermochemical conversion. The gas temperature in this region is relatively low, ranging from 1100 K to 1150 K, and is uniform in the horizontal plane. P7 – P13 are at the furnace height
m, above the secondary air inlets. The higher gas temperature in this region indicates that the thermochemical conversion process has progressed, and exothermic volatile reactions are taking place. However, the gas temperature at this furnace height is non-uniform in the horizontal plane. P7, P10, and P13 are on the symmetric plane with
and near the side wall of the furnace
m, where the gas temperature is relatively low and similar. P8, P9, P11, and P12 are off the symmetric plane with
m and
m, where the gas temperature is higher than those on the symmetric plane. P14 is at furnace height
m, where the gas temperature is the highest. At higher furnace height (
m), the gas temperature is slightly lower than that at
m, but it is uniform in the horizontal plane, as shown at P15–P20. The numerical simulations using the MP-PIC and CGM/DKM models replicate the experimentally observed trend of gas temperature well.
Granular flow and characteristics of fluidization
To understand the temperature distribution discussed above, it is essential to consider the granular flow and fluidization process of sand and biomass particles. illustrates the instantaneous distribution of sand and biomass particles in the furnace and cyclones at an arbitrary time after the numerical simulation reached a statistically steady state. The figure also shows the distribution of biomass particles colored with their size and temperature, and the gas velocity streamlines colored with the gas temperature and gas flow velocity. Initially, the sand particles are deposited in the lower part of the furnace ( m), and the biomass particles are then injected. The fluidization air flow, supplied from the primary air inlet at a velocity of about 1 m/s and the secondary air inlet at a higher velocity (
10 m/s), fluidizes the sand and biomass particles until quasi-steady-state fluidization is reached after 15 s of physical time.
Figure 6. Spatial distribution of sand and biomass particles (left panel), biomass particles colored with particle size and temperature (second and third panels), gas flow streamlines colored with gas flow speed and gas temperature (fourth and fifth panels), at an instant of time during the stationary operation stage. The results are obtained using MP-PIC and DKM models.

The furnace can be divided into two distinct regions: the dense particle region located in the lower part of the furnace, within 8 m ( m) above the bottom plane, and the dilute particle region located further up in the furnace and in the cyclones, i.e.,
m. Most of the particles are concentrated in the dense particle region, where the size of the biomass particles is relatively larger. The temperature of the biomass particles is relatively low near the inlet and increases quickly in the dense particle region. The gas flow in the dense particle region is rather complex due to the gas/solid interaction resulting from the granular flow. In the dilute particle region, the gas flow is accelerated when it enters the cyclones, forming a swirling flow motion. The swirling gas flow exhibits a “vortex breakdown” structure upon entering the top-box, where an inner recirculating zone can be observed. The gas flow exits the furnace at the outlet located on the left surface of the top-box. The particles in the cyclones are observed to be separated from the gas flow and returned to the furnace via the two connecting pipes. The gas temperature exhibits a locally cold region and a hot region in the lower part of the furnace, indicating the non-uniform nature of the dense particle region. Further up in the furnace, the gas temperature becomes more spatially uniform, which explains the larger spatial variation of gas temperature observed in the experiments at the furnace height of
m (thermocouple locations P8–P13) and the more uniform temperatures observed at the furnace height of
m (thermocouple locations P15 – P20).
illustrates the instantaneous distributions of biomass particles in the dense particle region at four different times. The first instant, s, corresponds to an arbitrary time after the furnace has reached a statistically stationary operation state. The figure also displays the cross-sectional averaged Sauter mean diameters of biomass particles, biomass particle velocity, and temperature at different furnace heights. Gas bubbles can be identified, for instance, in the bottom row of the figure and the location and size of bubbles evolve over time. The gas bubbles in the upper part of the dense particle region are large in size, and they periodically break up (e.g., at
s) and form (e.g., at
s). It can be seen that larger particles are located near the bottom of the furnace, due to gravity. These particles undergo drying, pyrolysis (devolatilization), char oxidation, and gasification while moving around in the bottom of the furnace. The particle temperature is higher near the primary air inlet than that further up in the furnace, due to the exothermic reactions of the particles. As the particles become smaller and lighter, they are blown upward in the furnace; hence, the mean diameter of the particles tends to decrease along the furnace height.
Figure 7. Spatial distribution of biomass particles in the dense particle region, colored with the particle diameter (upper row) and temperature (bottom row), and velocity vector of the biomass particles at
0, 3 s, 6 s, and 9 s.
is an arbitrary time after the flow and combustion process reach statistically steady states. The right panel shows the cross-section averaged Sauter mean diameter (
) of the particles, the velocity of the particles, and the temperature of the particles along the furnace height at
0, 3 s, 6 s, and 9 s. The results are from numerical simulations using MP-PIC and DKM.

At the lower row of the secondary air inlet (close to the furnace height indicated by the tube connecting the cyclones), cold fresh biomass particles are injected, resulting in a relatively low mean temperature in this furnace height. Small particles tend to be found in the center of the furnace, where the particle velocity is low. The larger particles tend to move at a higher velocity and are found in the near-wall region around the gas bubbles, where the gas velocity is higher. The mean diameter of particles in the region from the particle inlet to the upper surface of the dense particle region may be increasing along the furnace height due to the larger particles flowing upward around the boundaries of the gas bubbles in the furnace. Further up in the dilute particle region, the biomass particles are smaller and hotter (due to loss of mass during thermochemical conversion). It is worth noting that the bubble formation and breakup are highly unsteady, leading to temporally evolving particle properties (diameter, velocity, and temperature). However, the overall trend of the particle characteristics discussed above is similar at different times, as shown in the right panel of .
Thermochemical conversion process of biomass particles
displays cross-sectional, averaged gas-phase properties from numerical simulations, including gas temperature , gas pressure drop
, gas velocity
, gas volume fraction
, and mass fractions of H
and CO
. The pressure drop increases along the furnace height, varying rapidly in the dense particle region and reaching a plateau in the dilute particle region (
m). The gas volume fraction also varies significantly in the dense particle region, becoming nearly 1 in the dilute particle region. Gas temperature increases slowly along the furnace height in the dense particle region, where fuel particles undergo drying and pyrolysis, releasing volatile gases such as CO, H
, and CH
, as well as CO
and H
O, along with char. Combustion of CO, H
, CH
, and char in the dilute particle region is responsible for the continued increase in gas temperature along the furnace height until
m and the rapid decrease in H
mass fraction. The rapid decrease in CO
at the surface of the dense particle region is likely due to rapid mixing with air that erupted from the gas bubbles. Further downstream, the gas temperature decreases slightly along the furnace height due to heat loss to the walls. This result is consistent with the temperature measurement shown in , where the highest gas temperature is around P14 (
m).
Figure 8. Cross section averaged gas properties at different heights of the furnace including gas temperature (), pressure drop (
), gas velocity (
), gas volume fraction (
), and mass fractions of H
and CO
.

In the dense particle region, the mean gas velocity () increases due to the supply of secondary air. The gas velocity decreases when it erupts from the dense particle region, reaching a relatively constant flow speed before accelerating upon entering the cyclones, as shown in . The gas velocity profile provides insight into the particle velocity as shown in . In most parts of the furnace, the gas velocity exceeds the particle velocity, indicating that the particles are dragged by the gas flow and accelerate/decelerate along with the gas flow. In the upper part of the furnace, the particle velocity is similar to the gas velocity, likely due to the smaller size of the particles, which tend to follow the gas flow.
NOx formation process
shows the spatial distribution of mass fractions of nitrogen-containing species, including NO, NH, HCN, HNCO, CNO, and NCO, while shows the cross-section averaged nitrogen-containing species. The NO concentration exhibits a local peak at
m, followed by a decrease to a low level at
= 4–14 m, resulting from reactions with NH
and CH
through reactions R8–R10. This local peak is caused by biomass pyrolysis, which releases NO along with other volatile species, as shown in EquationEquation (26)
(26)
(26) and . Further up the furnace, the NO concentration gradually increases, reaching a level of about 80 ppm at
= 24–30 m. The formation of NO primarily occurs in the dilute particle region (
m), due to the oxidation of NH
(R7), CNO (R12), and NCO (R15).
Figure 9. Spatial distribution of mass fractions of nitrogen-containing species including NO, NH, HCN, HNCO, CNO, and NCO at different heights of the furnace.

Figure 10. Cross-section averaged mass fractions of nitrogen-containing species at different heights of the furnace including NO, NH, HCN, HNCO, CNO, and NCO.

A similar tendency of NO was observed in the study by Vainio et al. (Citation2012), indicating reasonable predictions in the current simulation. The NH concentration also exhibits a local peak at
2 m due to the release of volatiles during pyrolysis. NH
is rapidly consumed along the furnace height by reactions with O
(forming NO via reaction R7) and with NO (consuming NO through reaction R8). Above
10 m, NH
concentration is almost negligible. HNCO and HCN initially increase (due to biomass pyrolysis) and then decrease (due to volatile combustion, e.g., R11, R14) along the furnace height. CNO and NCO gradually increase along the furnace height and peak near
= 24 m, largely due to volatile combustion (R11, R14).
As a summary, biomass pyrolysis mainly occurs in the dense region of the furnace (m), resulting in extreme values of NO, NH
, HCN, and HNCO, as seen in EquationEquation 26
(26)
(26) . After pyrolysis, NO is reduced via reactions R8, R9, R10, R13, and R16. Following the injection of a large amount of air into the secondary inlets, NH
is converted to NO by R7 in large quantities, leading to an increase in NO and a decrease in NH
. Above the secondary air inlets, HCN and HNCO are oxidized and converted to N
by reactions R11–R16. It should be noted that NO concentrations, as well as CNO and NCO, fluctuate significantly at
= 24–30 m due to interaction with the cyclones, which induces the formation of unsteady rotational flow (swirling flow) structures, as shown in .
Discussion
The information provided about the granular flow and thermochemical conversion in the CFB furnace can enhance our understanding of the flow and combustion process in the furnace. The chemical kinetic model can be used to explain the NO formation process, while the bubble formation, breakup process, and division of dense and dilute particle regions can explain the gas temperature field and the interaction of the particles with secondary air. By analyzing CFD simulations, the operation of the furnace can be improved by optimizing the primary air and secondary air supply for different biomass fuels to achieve better fluidization in the dense particle region and combustion in the furnace. However, it is important to note that the present CFD results need to be thoroughly validated under industrial CFB boiler operating conditions. Due to the lack of experimental data, the current CFD results are only validated against mean gas temperature experimental data at a few sampling locations. Therefore, more experimental data on the gaseous species and particles in the furnace are desirable for further validation of the model.
Conclusions
A recently developed 3-D computational fluid dynamics (CFD) model has been employed for numerical simulations of biomass combustion in an industrial-scale circulating fluidized bed (CFB) furnace. The CFD model is based on the multi-phase particle-in-cell (MP-PIC) collision model, coarse grain method (CGM), and a distribution kernel method (DKM) that aims to resolve the local particle overloading issue typically found in the conventional particle centroid method (PCM). The hydrodynamic and combustion properties of the solid and gas phases are analyzed to provide insight into the physical and chemical processes in the furnace. The main conclusions drawn are as follows:
The CFD model can well overcome the dense particle local overloading problem in the industrial CFB furnace. Without the use of DKM, the CFD simulation could not achieve any stable solution; with DKM, the model can simulate the dynamics of the granular flow and thermochemical conversion of the particles. The predicted temperature field agrees well with the thermocouple experiments in various locations in the furnace.
The CFD results show that the CFB furnace can be divided into different regions based on the characteristics of the granular flow. In the lower part of the furnace, there is a dense particle region where most particles are located. In this region, gas bubbles are formed and evolve in space and time. The bubbles break up near the upper boundary of the dense region. Above the dense particle region is the dilute particle region, where the particles are smaller and lighter and tend to follow the gas flow. Further downstream, the tiny particles are separated in the cyclones and returned to the furnace through connecting pipes.
When biomass particles are supplied to the furnace in the dense particle region, the larger particles tend to follow the high-speed gas flow in the boundary of the gas bubbles or fall to the bottom of the furnace due to gravity. The Sauter mean diameter of the particles is relatively low in the fuel injection region due to the fall of larger particles toward the bottom of the furnace.
Drying and pyrolysis of the biomass particles occur mainly in the dense particle region. Oxidation of volatile gas and char particles continues in the dilute particle region. This explains why the highest temperature in the furnace is in the mid-height region, where most of the volatile gas is combusted. Further downstream, the gas temperature becomes more uniform in space, and the gas temperature is slightly lower than that in the mid-height region.
Biomass pyrolysis in the dense particle region contributes to the release of NH
, HCH, and HNCO. The combustion of volatile gas further up in the dilute region contributes to converting the nitrogen-containing species to CHO, NCO, and NO.
Acknowledgements
The authors would like to thank Kraftringen AB for supporting the detailed information on the CFB furnace. Miao Yang is sponsored by the China Scholarship Council (201808410350). The computations were performed on resources provided by the Swedish National Infrastructure for Computing (SNIC) at PDC (Beskow).
Disclosure statement
The authors declare that they have no known competing financial interests or personal relationships that could have appeared to influence the work reported in this paper.
Additional information
Funding
Notes on contributors
Miao Yang
Miao Yang Methodology, Investigation, Visualization, Writing-Original draft preparation.
Shenghui Zhong
shenghui Zhong Methodology, Investigation, Visualization
Shijie Xu
Shijie Xu & Leilei Xu Methodology, Visualization, Writing-Original draft preparation.
Leilei Xu
Peter Ottosson Investigation, Writing-Original draft preparation.
Peter Ottosson
Hesammedin Fatehi Methodology, Investigation, Writing-Original draft preparation.
Hesameddin Fatehi
Xue-Song Bai Funding acquisition, Writing-Reviewing and Editing, Resource, Supervision.
References
- Alobaid, F., N. Almohammed, M. Massoudi Farid, J. May, P. Rößger, A. Richter, and B. Epple. 2021. Progress in CFD simulations of fluidized beds for chemical and energy process engineering. Prog. Energ. Combust. 91:100930. doi:10.1016/j.pecs.2021.100930.
- Anderson, T. B., and R. Jackson. 1967. Fluid mechanical description of fluidized beds. Equations of motion. Ind. Eng. Chem. Fund. 6 (4):527–39. doi:10.1021/i160024a007.
- Andrews, M. J., and P. J. O’Rourke. 1996. The multiphase particle-in-cell (MP-PIC) method for dense particulate flows. Int. J. Multiphas. Flow. 22 (2):379–402. issn: 0301-9322. doi:10.1016/0301-9322(95)00072-0.
- Bassilakis, R., R. M. Carangelo, and M. A. Wojtowicz. 2001. TG-FTIR analysis of biomass pyrolysis. Fuel 80 (12):1765–86. doi:10.1016/S0016-2361(01)00061-8.
- Blaszczuk, A., A. Zylka, and J. Leszczynski. 2016. Simulation of mass balance behavior in a large-scale circulating fluidized bed reactor. Particuology. 25:51–58. doi:10.1016/j.partic.2015.04.003.
- Brink, A., P. Kilpinen, and M. Hupa. 2001. A simplified kinetic rate ex- pression for describing the oxidation of volatile fuel-N in biomass combustion. Energy & Fuels 15 (5):1094–99. doi:10.1021/ef0002748.
- Cai, L., Z. Xu, X. Wang, H. Bai, L. Han, and Y. Zhou. 2022. Numerical simulation and optimization of semi-dry flue gas desulfurization in a CFB based on the two-film theory using response surface methodology. Pow. Technol. 401:117268. doi:10.1016/j.powtec.2022.117268.
- Cai, R., H. Zhang, M. Zhang, H. Yang, J. Lyu, and G. Yue. 2018. Development and application of the design principle of fluidization state specification in CFB coal combustion. Fuel Processing Tech- Nology 174:41–52. doi:10.1016/j.fuproc.2018.02.009.
- Chapman, S., and T. George Cowling. 1990. The mathematical theory of non-uniform gases: An account of the kinetic theory of viscosity, thermal conduction and diffusion in gases. Cambridge, United Kingdom: Cambridge university press.
- Chen, W.-H., B.-J. Lin, Y.-Y. Lin, Y.-S. Chu, A. T. Ubando, P. L. Show, H. C. Ong, J.-S. Chang, S.-H. Ho, A. B. Culaba, et al. 2021. Progress in biomass torrefaction: Principles, applica- tions and challenges. Prog. Energ. Combust. 82:100887. doi:10.1016/j.pecs.2020.100887.
- Chomiak, J., and A. Karlsson. 1996. Flame liftoff in diesel sprays. Symp. (Int.) Combust. 26 (2):2557–2564. doi:10.1016/S0082-0784(96)80088-9.
- Collado, F. J. 2018. Hydrodynamics model for the dilute zone of circulating fluidized beds. Pow. Technol. 328:108–13. doi:10.1016/j.powtec.2018.01.007.
- Deng, B., M. Zhang, L. Shan, G. Wei, J. Lyu, H. Yang, and M. Gao. 2021. Modeling study on the dynamic characteristics in the full- loop of a 350 MW supercritical CFB boiler under load regulation. J. Energy Inst. 97:117–30. doi:10.1016/j.joei.2021.04.014.
- Di Blasi, C. 2009. Combustion and gasification rates of lignocellulosic chars. Prog. Energy Combust. Sci. 35 (2):121–40. doi:10.1016/j.pecs.2008.08.001.
- Dinh, C.-B., S.-S. Hsiau, C.-Y. Su, M.-Y. Tsai, Y.-S. Chen, H.-B. Nguyen, and H.-P. Wan. 2021. Full-loop study of a dual fluidized bed cold flow system: Hydrodynamic simulation and validation. Adv. Powder Technol. 32 (3):670–82. doi:10.1016/j.apt.2021.01.012.
- Fatehi, H., W. Weng, Z. Li, X.-S. Bai, and M. Aldén. 2021. Recent development in numerical simulations and experimental studies of biomass thermochemical conversion. Energy & Fuels 35 (9):6940–63. doi:10.1021/acs.energyfuels.0c04139.
- Ghadirian, E., J. Abbasian, and H. Arastoopour. 2019. CFD simulation of gas and particle flow and a carbon capture process using a circulating fluidized bed (CFB) reacting loop. Pow. Technol. 344:27–35. doi:10.1016/j.powtec.2018.11.102.
- Gidaspow, D. 1994. Multiphase flow and fluidization: Continuum and kinetic theory descriptions. New York, USA: Academic press.
- Gómez, M. A., J. Porteiro, D. Patiño, and J. L. Míguez. 2014. CFD modelling of thermal conversion and packed bed compaction in biomass combustion. Fuel 117:716–32. doi:10.1016/j.fuel.2013.08.078.
- Gu, J., Q. Liu, W. Zhong, and A. Yu. 2020. Study on scale-up characteristics of oxy-fuel combustion in circulating fluidized bed boiler by 3D CFD simulation. Adv. Powder Technol. 31 (5):2136–51. doi:10.1016/j.apt.2020.03.007.
- Hameed, S., A. Sharma, V. Pareek, H. Wu, and Y. Yu. 2019. A review on biomass pyrolysis models: Kinetic, net- work and mechanistic models. Biomass Bioenergy 123:104–22. doi:10.1016/j.biombioe.2019.02.008.
- Hansson, K.-M., J. Samuelsson, C. Tullin, and L.-E. Åmand. 2004. Formation of HNCO, HCN, and NH3 from the pyrolysis of bark and nitrogen-containing model compounds. Combust. Flame 137 (3):265–77. doi:10.1016/j.combustflame.2004.01.005.
- Hazenberg, T., and J. A. van Oijen. 2021. Structures and burning velocities of flames in iron aerosols. Proc. Combust. Inst. 38 (3):4383–90. doi:10.1016/j.proci.2020.07.058.
- Hilton, J. E., and P. W. Cleary. 2014. Comparison of non-cohesive resolved and coarse grain DEM models for gas flow through particle beds. Appl. Math. Model. 38 (17–18):38.17-18, pp. 4197–4214. doi:10.1016/j.apm.2014.02.013.
- Johnsson, F., R. C. Zijerveld, J. C. Schouten, C. M. van den Bleek, and B. Leckner. 2000. Characterization of fluidization regimes by time-series analysis of pressure fluctuations. Int. J. Multiphas. Flow 26 (4):663–715. doi:10.1016/S0301-9322(99)00028-2.
- Kadyrov, T., L. Fei, and W. Wang. 2019. Impacts of solid stress model on MP- PIC simulation of a CFB riser with EMMS drag. Pow. Technol. 354:517–28. doi:10.1016/j.powtec.2019.06.018.
- Karim, M. R., and J. Naser. 2018. CFD modelling of combustion and associated emission of wet woody biomass in a 4 MW moving grate boiler. Fuel 222:656–74. doi:10.1016/j.fuel.2018.02.195.
- Khan, A. A., W. de Jong, P. J. Jansens, and H. Spliethoff. 2009. Biomass combustion in fluidized bed boilers: Potential prob- lems and remedies. Fuel Process. Technol. 90 (1):21–50. doi:10.1016/j.fuproc.2008.07.012.
- Kolbitsch, P., J. Bolhàr-Nordenkampf, T. Pröll, and H. Hofbauer. 2010. Operating experience with chemical looping com- bustion in a 120 kW dual circulating fluidized bed (DCFB) unit. Int. J. Greenhouse Gas Control 4 (2):180–85. doi:10.1016/j.ijggc.2009.09.014.
- Kong, D., S. Wang, M. Zhou, K. Luo, C. Hu, D. Li, and J. Fan. 2020. Three-dimensional full-loop numerical simulation of co-combustion of coal and refuse derived fuel in a pilot-scale circulating fluidized bed boiler. Chem. Eng. Sci. 220:115612. doi:10.1016/j.ces.2020.115612.
- Ku, X., T. Li, and T. Løvås. 2015. CFD–DEM simulation of biomass gasification with steam in a fluidized bed reactor. Chem. Eng. Sci. 122:270–83. doi:10.1016/j.ces.2014.08.045.
- Ku, X., L. Tian, T. Løvås, and Terese Løv°as. 2014. Eulerian–Lagrangian simulation of biomass gasification behavior in a high-temperature entrained-flow reactor. Energy & Fuels 28 (8):5184–96. doi:10.1021/ef5010557.
- Larsson, A., M. Kuba, T. Berdugo Vilches, M. Seemann, H. Hofbauer, and H. Thunman. 2021. Steam gasification of biomass–typical gas quality and operational strategies derived from industrial-scale plants. Fuel Process. Technol. 212:106609. doi:10.1016/j.fuproc.2020.106609.
- Leckner, B. 2017. Regimes of large-scale fluidized beds for solid fuel conversion. Pow. Technol. 308:362–67. doi:10.1016/j.powtec.2016.11.070.
- Lee, B.-H., K.-M. Kim, Y.-H. Bae, H.-S. Oh, G.-B. Kim, C.-H. Jeon, and Y.-H. Ahn. 2022. Effect of bed particle size on the gas-particle hydrodynamics and wall erosion characteristics in a 550 MWe USC CFB boiler using CPFD simulation. Energy 254:124263. doi:10.1016/j.energy.2022.124263.
- Leppalahti, J. and T. Koljonen. 1995. Nitrogen evolution from coal, peat and wood during gasification: Literature review. Fuel Process. Technol. 43 (1):1–45. doi:10.1016/0378-3820(94)00123-B.
- Lin, J., K. Luo, C. Hu, L. Sun, and J. Fan. 2022. Full-loop simulation of a 1 MWth pilot-scale chemical looping combustion system. Chem. Eng. Sci. 249:117301. doi:10.1016/j.ces.2021.117301.
- Li, S., and Y. Shen. 2021. CFD investigation of maldistribution in a full- loop circulating fluidized bed with double parallel cyclones. Pow. Technol. 381:665–84. doi:10.1016/j.powtec.2020.12.020.
- Liu, S., H. Wang, F. Jiang, and W. Q. Yang. 2002. A new image reconstruction method for tomographic investigation of fluidized beds. AIChE J. 48(8):1631–38. doi:10.1002/aic.690480806.
- Li, J.-S., L.-T. Zhu, W.-C. Yan, T. A. B. Rashid, Q.-J. Xu, and Z.-H. Luo. 2021. Coarse-grid simulations of full-loop gas-solid flows using a hybrid drag model: Investigations on turbulence models. Pow. Technol. 379:108–26. doi:10.1016/j.powtec.2020.10.052.
- Lun, C. K. K., S. B. Savage, D. J. Jeffrey, and N. Chepurniy. 1984. Kinetic theories for granular flow: Inelastic particles in Couette flow and slightly inelastic particles in a general flowfield. J Fluid Mech 140:223–56. doi:10.1017/S0022112084000586.
- Luo, H., X. Wang, X. Liu, X. Wu, X. Shi, and Q. Xiong. 2022. A review on CFD simulation of biomass py- rolysis in fluidized bed reactors with emphasis on particle-scale models. J Anal Appl Pyrolysis 162:105433. doi:10.1016/j.jaap.2022.105433.
- Luo, K., F. Wu, S. Yang, M. Fang, and J. Fan. 2015. High-fidelity simulation of the 3-D full-loop gas–solid flow characteristics in the circulating fluidized bed. Chem. Eng. Sci. 123:22–38. doi:10.1016/j.ces.2014.10.039.
- Luo, H., L. Zhimin, P. A. Jensen, P. Glarborg, W. Lin, K. Dam-Johansen, and H. Wu. 2020. Experimental and modelling study on the influence of wood type, density, water content, and temperature on wood devolatilization. Fuel 260:116410. doi:10.1016/j.fuel.2019.116410.
- Lu, Y., Y. Zhou, L. Yang, X. Hu, X. Luo, and H. Chen. 2018. Verification of optimal models for 2D-full loop simulation of circulating fluidized bed. Adv. Powder Technol. 29 (11):2765–74. doi:10.1016/j.apt.2018.07.024.
- Ma, Q., F. Lei, X. Xu, and Y. Xiao. 2017. Three-dimensional full-loop simulation of a high-density CFB with standpipe aeration experiments. Pow. Technol. 320:574–85. doi:10.1016/j.powtec.2017.07.094.
- Ma, W., C. Ma, X. Liu, T. Gu, S. K. Thengane, A. Bourtsalas, and G. Chen. 2021. Nox formation in fixed-bed biomass combustion: Chemistry and modeling. Fuel 290:119694. doi:10.1016/j.fuel.2020.119694.
- Muhammad, A., N. Zhang, and W. Wang. 2019. CFD simulations of a full-loop CFB reactor using coarse-grained Eulerian-Lagrangian dense discrete phase model: Effects of modeling parameters. Pow. Technol. 354:615–29. doi:10.1016/j.powtec.2019.06.016.
- Nikoo, M. B., and N. Mahinpey. 2008. Simulation of biomass gasification in fluidized bed reactor using ASPEN PLUS. Biomass Bioenergy 32 (12):1245–54. doi:10.1016/j.biombioe.2008.02.020.
- O’Rourke, P. J., and D. M. Snider. 2010. An improved collision damping time for MP-PIC calculations of dense particle flows with applications to polydisperse sedimenting beds and colliding particle jets. Chem. Eng. Sci. 65 (22):6014–28. issn: 0009-2509. doi:10.1016/j.ces.2010.08.032.
- O’Rourke, P. J., and D. M. Snider. 2012. Inclusion of collisional return-to- isotropy in the MP-PIC method. Chem. Eng. Sci. 80:39–54. issn: 0009-2509. doi:10.1016/j.ces.2012.05.047.
- Qi, T., T. Lei, B. Yan, G. Chen, Z. Li, H. Fatehi, Z. Wang, and X.-S. Bai. 2019. Biomass steam gasification in bubbling fluidized bed for higher- H2 syngas: CFD simulation with coarse grain model. Int. J. Hydrog Energy 44 (13):6448–60. doi:10.1016/j.ijhydene.2019.01.146.
- Ranz, W. E., and W. R. Marshall. 1952. Evaporation from drops, part i. chem- ical eng. progress 48:141–46.
- snider, d. m. 2001. An incompressible three-dimensional multiphase particle-in- cell model for dense particle flows. J. Comput. Phys. 170 (2):523–49. issn: 0021-9991. doi:10.1006/jcph.2001.6747.
- Sun, R., and H. Xiao. 2015. Diffusion-based coarse graining in hybrid continuum– discrete solvers: Theoretical formulation and a priori tests. Int. J. Multiphas. Flow 77:142–57. doi:10.1016/j.ijmultiphaseflow.2015.08.014.
- Svensson, A., F. Johnsson, and B. Leckner. 1996. Bottom bed regimes in a cir- culating fluidized bed boiler. Int. J. Multiphas. Flow 22 (6):1187–204. doi:10.1016/0301-9322(96)00025-0.
- Tsuji, Y., T. Kawaguchi, and T. Tanaka. 1993. Discrete particle simulation of two- dimensional fluidized bed. Pow. Technol. 77 (1):79–87. issn: 0032-5910. doi:10.1016/0032-5910(93)85010-7.
- Tu, Q., and H. Wang. 2018. CPFD study of a full-loop three-dimensional pilot-scale circulating fluidized bed based on EMMS drag model. Pow. Technol. 323:534–47. doi:10.1016/j.powtec.2017.09.045.
- Vainio, E., A. Brink, M. Hupa, H. Vesala, and T. Kajolinna. 2012. Fate of fuel nitrogen in the furnace of an industrial bubbling fluidized bed boiler during combustion of biomass fuel mixtures. Energy & Fuels 26 (1):94–101. doi:10.1021/ef201145j.
- Vikram, S., P. Rosha, and S. Kumar. 2021. Recent modeling approaches to biomass pyrolysis: A review. Energy & Fuels 35 (9):7406–33. doi:10.1021/acs.energyfuels.1c00251.
- Wang, S., K. Luo, H. Chenshu, and J. Fan. 2017. CFD-DEM study of the effect of cyclone arrangements on the gas-solid flow dynamics in the full-loop circulating fluidized bed. Chem. Eng. Sci. 172:199–215. doi:10.1016/j.ces.2017.05.052.
- Wang, S., K. Luo, H. Chenshu, L. Sun, and J. Fan. 2018. Impact of operating parameters on biomass gasification in a fluidized bed reactor: An Eulerian-Lagrangian approach. Pow. Technol. 333:304–16. doi:10.1016/j.powtec.2018.04.027.
- Weissinger, A., T. Fleckl, and I. Obernberger. 2004. In situ FT-IR spectroscopic investigations of species from biomass fuels in a laboratory-scale combustor: The release of nitrogenous species. Combust. Flame 137 (4):403–17. doi:10.1016/j.combustflame.2004.02.010.
- Weller, H. G., G. Tabor, H. Jasak, and C. Fureby. 1998. A tensorial approach to computational continuum me- chanics using object-oriented techniques. Comp. Phys. 12 (6):620–31. doi:10.1063/1.168744.
- Wen, C. Y. 1966. Mechanics of fluidization. Chem. Eng. Prog. Symp. Ser 62:100–11.
- Winter, F., C. Wartha, and H. Hofbauer. 1999. NO and N2O for- mation during the combustion of wood, straw, malt waste and peat. Bioresour. Technol. 70 (1):39–49. doi:10.1016/S0960-8524(99)00019-X.
- Yan, L., Y. Cao, H. Zhou, and B. He. 2018. Investigation on biomass steam gasification in a dual fluidized bed reactor with the granular kinetic theory. Bioresour. Technol. 269:384–92. doi:10.1016/j.biortech.2018.08.099.
- Yang, M., S. Morteza Mousavi, H. Fatehi, and X.-S. Bai. 2023. Numerical simulation of biomass gasification in fluidized bed gasifiers. Fuel 337:127104. doi:10.1016/j.fuel.2022.127104.
- Yang, S., and S. Wang. 2020. Eulerian-Lagrangian simulation of the full- loop gas-solid hydrodynamics in a pilot-scale circulating fluidized bed. Pow. Technol. 369:223–37. doi:10.1016/j.powtec.2020.05.043.
- Yang, S., S. Wang, and H. Wang. 2020. Numerical study of biomass gasification in a 0.3 MWth full-loop circulating fluidized bed gasifier. Energy Convers. Manage. 223:113439. doi:10.1016/j.enconman.2020.113439.
- Yang, S., H. Wang, Y. Wei, J. Hu, and J. W. Chew. 2019. Numerical investigation of bubble dynam- ics during biomass gasification in a bubbling fluidized bed. ACS Sust. Chem & Eng 7 (14):12288–303. doi:10.1021/acssuschemeng.9b01628.
- Yang, M., J. Zhang, S. Zhong, T. Li, T. Løvås, H. Fatehi, and X.-S. Bai. 2022. CFD modeling of biomass combustion and gasification in fluidized bed reactors using a distribution kernel method. Combust. Flame 236:111744. doi:10.1016/j.combustflame.2021.111744.
- Yan, L., C. Jim Lim, G. Yue, B. He, and J. R. Grace. 2016. Simulation of biomass-steam gasification in fluidized bed reactors: Model setup, comparisons and preliminary predictions. Bioresour. Technol. 221:625–35. issn: 0960-8524. doi:10.1016/j.biortech.2016.09.089.
- Zhou, H., A. D. Jensen, P. Glarborg, and A. Kavaliauskas. 2006. Formation and reduction of nitric oxide in fixed-bed combustion of straw. Fuel 85 (5–6):705–16. doi:10.1016/j.fuel.2005.08.038.
- Zhou, Z. Y., S. B. Kuang, K. W. Chu, and A. B. Yu. 2010. Discrete particle simulation of particle–fluid flow: Model formulations and their applicability. J. Fluid Mech. 661:482–510. doi:10.1017/S002211201000306X.