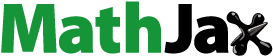
ABSTRACT
The aim of this paper is to decide whether or not term premiums appear and thus are predictable in Central European countries in the data sample of forward rate agreement interest rates and corresponding spot interest rates. Term premiums theoretically are explained thanks to the applications of the efficient market hypothesis theory, the expectations theory, and the liquidity preferences theory. Empirically, we used cointegration techniques for nonstationary time series, mainly using the cointegration method (simple and even for panel data) and the vector error correction model. We used data samples of Central European countries (the Czech Republic, Hungary, Poland) and also of the United Kingdom. We found that the best future estimate of term premiums is its current value. It does not refer in the same way to the market efficiency hypothesis (EMH) or theexpectations hypothesis (EH).
Introduction
The efficient market hypothesis, formulated by Eugene F. Fama, perfectly merges competition in economics with the theory of finance. In general, this hypothesis represents the impossibility of the existence of arbitrary risk-free profiting from trading in the long run. In the short term, this arbitrary profit from trading might appear, but thanks to market movements this opportunity immediately disappears. In general, this hypothesis is being most frequently tested in the FOREX (foreign exchange market) because it is the financial market with the highest volumes of trading, and there we expect the EMH (efficient market hypothesis) to be the most visible.
In the theoretical point of view of exchange rates, the forward rate is assumed to be the unbiased predictor of the future spot exchange rate according to the rate of the maturity of the forward rate (Fama and French Citation2016). But the EMH can be tested on any financial asset.
In this paper, we are interested in term premium features in Central European countries, particularly the interest-rate term premiums, FRA (forward rate agreement) rates. The term premium is being explained thanks to the so-called expectations hypothesis, which is deducted from the efficient market hypothesis for assets that differ from themselves in the time to maturity. The expectations hypothesis is deducted thanks to the no arbitration assumption for the financial market, the most important idea of quantitative finance theory. Lastly, needless to say, no arbitration assumptions are weakened thanks to the results of behavior financial theory and other modern approaches. The connection between the EMH and the interest rates term structure is clear. The term structure of interest rates is testing Fama’s hypothesis for the forward interest rates of different maturities – in the other words, whether different maturity forward rates (in our research, always three-month forward rates) are unbiased predictors of future spot interest rates. Moreover, the EMH is the basis of the quantitative finance approach (the non existence of arbitrage opportunities).
We see the research gap of this paper as having two levels. At first, theoretically, even after more than half a century of research, the financial literature has not reached a consensus on the presence or absence of validity of the EMH (Leković Citation2018). Secondly, empirically few studies present EMH anomalies in European countries from 2001 to 2020 (Rossi and Gunardi Citation2018). Our research covers this period for Central European countries, as well as the UK as a benchmark.
The UK, as a benchmark, is expected to be the economy with the most developed financial sector in Europe. Thus, we should see the most verified conclusions of quantitative finance theory (Term premium structure, Efficient market hypothesis, Expectations hypothesis) as evidence. We see many difficulties, even in the sample of the UK variables. If we assume the theoretical function of the financial markets, the pros and cons in Europe´s most developed financial economy (the UK) should be taken into account when analyzing the less developed financial markets in the CEE economies.
Theoretical Explanation of Term Premium Existence
The market efficient hypothesis is demonstrated, in the most simply way, in the form (1)
Michael Wickens (Citation2012) expands upon the idea about the time series of FOREX variables following the logarithmic – normal probabilistic distribution. The risk premium on the FOREX (contrary to equation No. 1) is then explained with the formula (2) on the C-CAPM assumption.
The differences from equation No. 2, which appeared, are as follows. These are reasoned first according to the transaction costs (moreover, these transaction costs vary in time), and second to the risk premium existence. In many empirical studies (e.g., Baillie and Bollerslev, Citation2000; Hodrick, Citation2014; Kočenda and Moravcová Citation2019; Božović Citation2020), they rejected the existence of the stable relationship No. 1 or even the stable independent and identical distribution of deviations from this relationship No 2. Moreover, these deviations are unexplainable, thanks to equation No. 2 (the covariance between the change in consumption and the spot exchange rate or the covariance between the expected inflation and the change in the consumption or both). We can explain the deviations only using a disproportionately high coefficient of the relative risk aversion. For our purposes, Abbot (Citation2017) concluded that using the higher coefficient of risk aversion leads to an unexplainable evolution of the risk-free rate. The C-CAPM puzzle must then be solved using the Hansen–Jagannathan bound (Hansen Citation2017), the solvation is unsatisfactory for the empirical results seen on the financial markets.
The falsification of the expected relationship appears empirically in financial market bubbles. In the modern literature, we solve bubbles, using at first the “technological bubbles” theory (Pástor and Veronesi Citation2009). Secondly, Gertler and Karadi (Citation2015) explained financial bubbles in terms of the capital limitations of the free entrance to the financial market. Thirdly, Brunnermeier (Citation2016) explained the persistence of the asset bubbles as the product of rational arbitrageurs. Lastly, Bernanke et al. (Citation2018) explained the existence of bubbles calculating the financial accelerator. This accelerator calculates asymmetric information, the difference in productivity of the real firms, and the difference in the debt options of firms. These factors, already in the real economy, influence the bubbles’ persistence in the financial sector. These three approaches together prove the so-called rational financial bubbles. These bubbles are explained due to the rational behavior of traders, not the irrational (chaotic) behavior visible on the markets. The New Keynesian approach can solve the problems of rational bubbles not only theoretically but also empirically (Yellen Citation2017).
This short explanation leads us to the main aim of this article, which is the term structure of the Central European money market – in other words, the way in which the implicit forward interest rates predict the structure of the spot interest rates, which evolve differently in terms of maturity. Moreover, macroeconomic problems of the so-called twisted interest rate are involved. In the following deduction, we loosely follow the explanation of term structure from Cuthbertson and Nitzsche (Citation2005).
At first, we make assumptions about two different zero-coupon bonds different to their terms of maturity. More, we assume that all of the future interest rates are known and fixed. At time t the R(1,t) is the one-year spot interest rate, the R(2,t) is the two-year spot interest rate p.a. Then we can calculate, using the Expectations Hypothesis (EH), the implicit forward rate following the analyzed period F(2,1:t) according to formula (3).
Using the continuously compounded rates, we transform this relationship into a linear relationship.
For the three time periods (there is an implicit forward rate between years t + 1 and t + 3):
for the different investment:
From this example, we see how to calculate any implicit forward rate for any horizon from the observed spot interest rates. This approach is called “bootstrapping.” This is the empirical method used to calculate any time to maturity interest rates (implicitly) from observed spot interest rates and forward rates.
The two time periods’ model with the term premium of the expectations hypothesis (not the pure form) provides the following relationship:
Equations No. 7 and No. 4. provide the final Equationequation (8)(8)
(8)
The final statement of the deduction is that the forward interest rate is an unbiased predictor of the future spot interest rate included in the term premium. Here we are consistent with the pure expectations hypothesis, where there are T(2,t) = 0. Moreover, by subtracting R(1,t) from both sides of the equation we derive the forward premium. The forward interest rate premium is an unbiased predictor of the expected change in the spot interest rates. Assuming rational expectations, we achieve:
The previous relationship is being tested using the regression
Here we expect α = -T and β = 1. In other words, under the pure expectations hypothesis, we expect the forward rate premium/discount to be an unbiased predictor of the change in the future spot interest rate.
The term premium is understood as being similar to the risk premium for stock assets (C-CAPM approach). That conclusion is usually referred to as the expectation hypothesis with liquidity preference. It means that interest rates of longer maturities are based on risk-free returns and, moreover, they are given with the average of short-term returns. Necessarily, at the same time, the interest rates of longer maturities are increased by the term premium. For example, although short-term interest rates are not increasing in the market, the appearing uncertainty results in demand for higher-term bonuses, which also increase spot returns for longer maturities. The expectations hypothesis is interlinked with the theory of liquidity preferences. We explain the interest rates of longer maturities as the average of shorter maturities interest rates and the average of term premiums.
According to the C-CAPM approach modified to the interest rates, we assume that for any capital asset (including zero-coupon bonds and corresponding interest rates), anyone can apply the optimal choice theorem. This theorem explains the motivation of the investor to compare between present costs and future expected benefits. This comparison is made in the way of the effects of a particular asset unit. In the stochastic environment, we realize that the price of any capital asset must follow:
In which Pt is the price of asset, Mt+1 is the stochastic discount factor (the division of the marginal utilities from intertemporal consumption multiplied by the individual discount factor), and Dt+1 is dividend paid (if any). Stochastic discount factor is the general mechanism of the transition of future value to present values. Moreover, it can be calculated in many ways.
If this relationship is modified for expected yields instead of for prices, and we use the expectation hypothesis with liquidity preference, we then gain:
where Ht+1(n) is an immediate rate of return of the bond. The original C-CAPM approach for capital assets assumes the logarithmic normal distribution of rate of returns (yields), and also logarithmic normal distribution of the stochastic discount factor. These assumptions together lead to:
Or, for specific function of utility (derived from the specific consumption and risk aversion formula):
The term premium is presented on the left side of the previous equation by the instantaneous difference between the discounted bond rate and the risk-free rate of return (spot interest rates). This term premium is explained by the tightness of the relationship between the development of consumption and the instantaneous discounted bond rates over time. Investors perceive bonds with longer maturities as risky (except for the one-period bonds, which are assumed to be benchmarks for spot rates). Because of the instantaneous longer maturities, returns of these bonds move in the same direction as the growth of their consumption. Then it is difficult (or even impossible) for these investors to smooth their consumption over time using bonds with longer maturities. Higher-term premiums appear for those bonds, which are positively correlated with the macroeconomic consumption (or simply said, positively correlated to the business cycle in an economy). These bonds cannot be used to smooth the consumption of a representative investor over time. This is why the general investor perceives this bond to be risky, thus requiring higher-term premiums. Empirical evidence in developed countries shows the lower variability of instantaneous returns on bonds compared to the return on shares. As a result, the term premium of a typical bond is lower than the risk premium of a typical stock. Of course, we find many more risky bonds than assets.
Term Premium Puzzles
As previously mentioned, the term premium for interest rates is only a generalization of the risk premium for capital assets (these ideas are most frequent described using the FOREX markets evolution). So, we do see the similarities of empirical evidence puzzle explanations like those in assets.
Rational Bubbles
Assuming a neutral relationship to risk, rational bubbles can explain excessive returns on capital assets precisely from the perspective of economic agents. It is also speculative or rational behavior that leads the price of a particular asset progressively from its equilibrium level, which is derived on the basis of economic fundamentals. Simply put, investors are still buying a capital asset, even though it is already clearly overvalued. It is because they simply believe that the bubble will be “blown” and thus they make additional profit. The reason for such behavior is the ambiguity of the equilibria of the price system of the capital asset.
In the standard, forward-looking differential Equationequation (16)(16)
(16) , the capital asset price is explained by the price in the future (where the λ is the discount factor) and the economic fundamental factors in the current period are in vt. If we solve (16), we get solution (17) (πt is the first derivative of the price upon time):
It is only one solution of theoretically infinitely many solutions, which are described by the general formula:
where
The Bt parameter causes the expansion of the rational bubble. Potential investors rationally decide whether the bubble will be blown or will burst. This probability distribution is asymmetric. Most often, this layout is described as follows:
Thus, it is apparent that the probability distribution is asymmetric, and therefore, assuming rational expectations, the expected values of residuals might be non-zero. Menkhoff et al. (Citation2017) find solutions using three possible methods. These are “variance bounds tests,” “run tests,” and “specifications tests.”
Learning by Doing
The ex-post error assessment process and efforts to prevent it in the future causes further observed deviations of actual values from the expected values of the term bond based on the expectations hypothesis with the liquidity preference. When there is a change in the monetary policy regime (e.g., restriction), this will affect expectations regarding the term bond.
The expectations about immediate bond value can be modeled as:
where It is the probability of the existence of the original monetary policy regime. Assuming that a change in the monetary policy regime is publicly announced in advance, we can write the rule of investor expectations (derived probability It) based on the Bayesian method:
Assuming all market agents can “learn” from their own mistakes, then when they decide (within a discontinuous risk factor) between two possible scenarios where one necessarily has to occur, the expected asset price must be non-zero.
The Peso Problem
A very similar situation to the problem in the previous chapter occurs when investors do not have the opportunity to be informed in advance of such a major change as in the monetary policy regime. Although these economic agents are fully rational and willing to absorb ex-post information, they are unable to predict the situation described (Rogoff Citation2017). The term “peso problem” was first used for the Mexican peso during the 1970s, when that currency, although traded for a long time with a forward discount against the US dollar, went through a long trend of depreciation.
where λt is the probability of changing the monetary policy regime. We can rewrite the expected exchange rate as (25) and the error of the forecast assuming that the change in the monetary policy regime is not realized as (26).
where Λpt + 1 represents the expected exchange rate difference depending on the monetary policy regime. Again, we observe that even with a low probability of changing the monetary policy regime, but with a significantly large difference in expected values, the system generates a very slanted probability distribution of forecasting errors. If the monetary policy regime changes, the forecast deviation is modified to (27).
In the second case, when there are no unexpected changes and there is an effective process of “learning” and, of course, assuming rational expectations, the “peso problem” does not exist. However, the effects of rational bubbles on the markets and the “peso problem” on foreign exchange asset markets are very similar. Notably, while the “peso problem” arises from expected changes in market fundamentals, bubbles appear as deviations from those fundamentals. Lewis (Citation2011) points out how the expected exchange rate is biased based on the peso problem. Above all, monetary policy in such a situation is ineffective. Needless to say, the peso problem is interconnected with the FOREX market as the most volatile financial market. The impact of the peso problem on the real bo nd market is much lower than was introduced. But many authors, mainly from Central European countries, solve similar impacts of Eurozone problems (ECB quantitative easing) and EU fiscal decisions impacts.
Rational Expectations – Imperfections in the Processing of Information
One significant problem of the current analysis (and many empirical analyses) is the assumption of rational expectations. The problem of an efficient market hypothesis explicitly assumes rationally generated expectations. Therefore, if we reject the hypothesis H0 about the efficiency of the particular asset price, does that mean that the market of this asset also does not meet rational expectations? The standard regression tested (for instance according to Froot, Citation1993) is as follows:
where, if the risk-neutral market efficiency hypothesis were to be applied, we would obtain the following coefficients based on the regression analysis: α = 0, β = 1 and νt + k is a zero vector based on the complete set of available information available at the time of expectation. Let us repeat how to express the beta coefficient:
If we consider simple algebraic transformation:
where βRE a βRP:
βRE is the ratio of the assumption of rational expectations based on the relationship (31). Once this coefficient is non-zero, then it is clear that the prediction error νt +k is correlated with the information available at time t. Furthermore, βRP is the risk spread dispersion coefficient over time. Therefore, the coefficient does not correspond to the acceptance of an efficient market hypothesis (deviation from the assumption of a neutral relationship to risk). For despite a zero coefficient of βRP, it is very likely that there is a risk premium. However, this risk premium is stable over time, assuming zero coefficient. So we can test two additional hypotheses: the rational expectations hypothesis and the “time-varying” risk premium hypothesis. The hypothesis of rational expectations on the foreign exchange market is as follows:
while the stable risk premium over time hypothesis is defined as:
Most practical analyses (e.g., Frankel Citation2017) have shown that the rejection of the efficient market hypothesis is due to the failure of the assumption of rational expectations (very statistically reliable non-zero coefficient βRE). Other studies, particularly by time series cointegration methods, show that at weekly time intervals the rejection of the efficient market hypothesis is mainly due to the existence of a risk premium, while on monthly data the rejection of the efficient market hypothesis is caused by both the risk premium and the assumption of rational expectations (Liu and Maddala Citation1992).
Literature survey/empirical Evidence in Countries Analyzed
To fully understand all of the consequences, we must describe the economic environment to which the theory is applied. This environment in the EU (and in the US) has seemed to be far from its equilibrium since the Great Financial Crisis of 2009 (GFC). Developed countries, with their monetary (and also fiscal) policy, use unconventional instruments to achieve its goals. The conditions complicate the evaluation of the EMH hypothesis and forward rate as unbiased predictor testing. But the hypotheses might be true. We do see many parallels, for instance, in the electricity and gas crisis of 2021. Here, the general theory assumption also said that the markets expect a future price decrease due to forward prices. But is this true when Central Authority could intervene?
In the Czech Republic, Bruna (Citation2006) showed a limited effect of changes in the central bank repo rate on the instantaneous adaption of swap and forward rates. This concluding remark was made during the disinflationary politics from 1998 to 2008. The central banks’ weakened credibility was caused by the related mistaken expectations of investors about the gradual tendency for the economy to reach a state of low inflation.
Kladıvko (Citation2010) has estimated the Czech Treasury yield curve from 1999 to 2010. He interested himself in the estimation errors of forward rates. He has done this because the estimated Czech Treasury yield curve is unavailable.
Kučera, Dvořák, and Komárková (Citation2016) perceive the term structure of yields as an important source of information on market expectations about future macroeconomic development and investor risk perceptions. Authors employed in the Czech National Bank were interested in obtaining such information through the decomposition of the Czech Government bond yield curve into its components. Decreasing bond yields consist of risk neutral yield and of term premium and from the 2009 great financial crisis also of the credit risk (ten-year zero-coupon bond yield). The one-year zero-coupon bond yield is under the previous scheme created only with the risk neutral yield. Notably, for both horizons in the Czech bond yield horizons, the portfolio effect has a negative value.
In Poland, Akhigbe, Madura, and Marciniak (Citation2012) described how the National Bank of Poland estimates the yield curve. They found differences between the base on zero-coupon bonds and the 3 M forward method. Moreover, they found that the Polish market of treasury bonds is characterized by moderate liquidity and periodically reduced efficiency due to the relatively low number of bond series and gaps in the time to maturity spectrum.
Jablecki, Raczko, and Wesolowski (Citation2016) published a study of the National Bank of Poland (NBP) regarding the negative term bond premium. The NBP’s strategy is to maintain price stability, presenting the views on price stability and economic growth developments that shape short-term rate policy. The main argument for how to achieve low inflation in the environment of the EU’s relaxed monetary policy is that the range of interest rates in a small open economy is not fully dependent on the domestic level of short-term rates. More specifically, a formal term structure model shows that Poland’s bond term premium has been driven to negative levels by yield-hungry foreign investors in the context of a restricted supply of short-term treasury stock and reduced uncertainty about the future path of short-term rates. As a result, long-term yields have fallen below the expected path of future short-term rates, adding to the monetary stimulus even as policy rates remain unchanged.
Wesoƚowski (Citation2018) analyzed the impact of long-term interest rates on macroeconomic variables in a small open economy. He showed that the time-varying term premium stabilized GDP and did not affect inflation volatility in Poland.
Brzeszczyński, Gajdka, and Kutan (Citation2019) provided many conclusions, one of which is that the central banks’ interest rate policy may not be effective in low-interest rates maturities, which suggests a larger role for fiscal policy. This weak reaction was observed in the segments analyzed: foreign exchange market, stock market, bonds market, and financial derivatives market in (Jablecki, Raczko, and Wesolowski Citation2016). A more intensive reaction was observed in case interest rates moving upward rather than downward. Furthermore, authors detected in the CEE economies, in the Central European economies the so-called “wealthy effect” in the movement in stock prices and foreign exchange rates as a response of the central bank.
In Hungary, Csajbók, Hudecz, and Tamási (Citation2010) tried to extract information about market expectations of future nominal interest rates and inflation from the prices of T-bills and T-bonds. By using the estimated zero-coupon rates, Csajbok et al. also tried to quantify the average difference between yields to maturities (YTMs) of coupon bonds and the corresponding zero-coupon rates in Hungary. Additionally, he wanted to infer nominal interest rates and inflation expectations from the zero-coupon yield curve. Finally, he concluded with an application of the estimated zero-coupon yields, which empirically demonstrates the bias in YTM-type yield curves when the underlying zero curve is non-horizontal. Moreover, there is a quantification of the inherent bias in the daily “benchmark yields” calculated by the State Debt Management Agency (SDMA).
Kicsák (Citation2017) is interested in the change in interest expenditures from 2000 to 2015. He solves the consequences of the decreasing yields on the government securities. He also highlights that interest rate cuts together with the Self-Financing Program, in which Hungary’s external vulnerability is mitigated. These, combined with international effects, have had a tangible impact on developments in Hungarian government securities’ yields.
Mariusz, Orlowski, and Radziwill (Citation2004) analyzed the transparency of monetary policy in the Czech Republic, Poland, and Hungary. They assumed that the expectations are determined upon the yield curve reflecting a predicted path of future official interest rates based on the expectations theory of the term structure that is included in the equation. Monetary policy should be credible by looking at the long-end of the yield curve. In 2004, the authors’ general finding was that the monetary authorities of Poland, Hungary, and the Czech Republic had advanced their monetary policy transparency to a level roughly comparable to that of the ECB. There were no doubts in 2004 that the adoption of DIT strategies by the three examined central banks contributed to a significant improvement in monetary policy transparency in all of the countries analyzed. Despite the statements of Mariusz, Orlowski, and Radziwill (Citation2004), Nagy (Citation2020) provided a sophisticated analysis about Hungarian term rates in an environment in which the economy is relatively developed, while the bond market has not matured.
On the other hand, Stoupos and Kiohos (Citation2017) assess whether the post-communist countries of the EU are mature enough to join the Economic and Monetary Union using nominal exchange rates as an empirical instrument. Their findings are based on three parameters: volatility shocks persistence, leverage effects, and short-term/long-term dynamic relationships. The error correction model highly suggests a positive dynamic linkage between the euro and the Czech koruna, the Hungarian forint, and the Croatian kuna, especially after EU accession. Therefore, from a financial perspective, there is strong evidence that these countries are not ready for eurozone participation. Nevertheless, Stoupos and Kiohos (Citation2017) admit that the dynamic linkages between the exchange rates is not the only strong indicator that a member state of the EU is eligible to become part of the eurozone. Notably, this statement is confirmed by Kočenda and Moravcová (Citation2019). Lastly, Stoupos and Kiohos (Citation2017) argue that the post-communist countries should continue to improve their economic and political relations with other members of the EU in order to adopt this exchange rate regime (euro adoption). Otherwise, they would face the fate of Greece because the euro is a really strong currency, unsuitable for the weak and vulnerable economies of Europe.
Moreover, according to Kladivko and Österholm (Citation2019), the data sample of Sweden’s most important four financial variables significantly outperforms the random walk for only one horizon. At the longest horizon for the repo rate, and at all horizons for the five-year government bond yield, the random walk significantly outperforms the market participants. For the exchange rates SEK/USD and SEK/EUR, no significant differences in forecasting precision can be established.
In the United Kingdom, Joyce, Lildholdt, and Sorensen (Citation2010) have researched the data sample of UK interest rates over time since October 1992. Their model imposes no-arbitrage restrictions across nominal and real yields, which means that the interest rates can be decomposed into expected real policy rates, expected inflation, real-term premium, and inflation-risk premium. Greenwood, Hanson, and Vayanos (Citation2015) discuss the situation in which most developed countries’ central banks, they have been conducting monetary policy through two primary instruments: quantitative easing, in which they buy long-term government bonds and other long-term securities; and so-called forward guidance, in which they guide market expectations about the path of future short rates. Because QE alters the maturity structure of the government debt that is available to the public. QE also changes the amount of duration risk that market participants must bear, thereby affecting bond risk premiums and long-term interest rates. Forward guidance may also affect long rates because it contains information about the central bank’s willingness to keep short rates low in the future. Long-Term interest rates are so low because of a variety of structural factors, such as notably slower productivity growth and a surplus of global saving, and long-term interest rates likely have lowered expectations of steady-state interest rates and pushed down long-term yields through the expectations component. In addition, accommodative monetary policy in the United States and abroad appears to have lowered the term premium on long-term bonds (Bauer and Glenn Citation2016).
Dell’Ariccia, Rabanal, and Sandri (Citation2018) compare the impact of the financial crisis of 2008 on the United Kingdom, the Eurozone, and Japan. In all of the countries analyzed, there was a great contraction in the real GDP. This was the main reason central banks cut interest rates so aggressively. Moreover, they deployed a variety of untested and unconventional monetary policies. They finally examined the experience with unconventional monetary policies in all three governances (QE, quantitative easing, forward guidance, and negative interest rate policies).
Analysis Methodology, Cointegration, Vector Error Correction Model, and Pedroni Panel Cointegration
From the econometric point of view, the principle of time series cointegration is the option of obtaining a stationary linear combination of nonstationary time series. Thus, for
m of one-dimensional time series, which we make stationary by differentiation, we get:
for any non-trivial linear combination of such series. In economic and financial practice, we can transform originally nonstationary time series and combine them linearly in the resulting stationary combination. Cointegration is therefore a state of long-term equilibrium of economic variables time series. Hendrych and Cipra (Citation2018) conclude that cointegration can be exactly defined as follows. Once {y1t}, …, {ymt} are nonstationary time series, where in each of them the nonstationary is caused by exactly one unit root of the respective autoregressive polynomial. Then, the series {y1t}, …, {ymt} are cointegrated if their non-trivial (non-zero) linear combination is stationary; or second, equivalently, the VAR model of a multidimensional series (y1t, …, ymt)´ has m – r unit roots. The symbol r represents the number of cointegration relations. Hendrych and Cipra (Citation2018) recommend differentiating members of this series when working with a one-dimensional nonstationary series. However, when working with more nonstationary quantities observed over time, the differentiation of these time series is statistically correct, but the model constructed for differentiated quantities usually does not reveal long-term equilibrium relationships between the original undifferentiated quantities.
If we consider two time series {xt} and {yt}, both nonstationary type I(1), the model of their influence on each other (despite the nonstationarity) will be decided by the model
However, we are interested in the relationship between the variables x and y only after reaching equilibrium. In this state, the differences are almost zero. But if we have some idea of the cointegration of these time series, then it is possible to use a modified model.
In such a model, a correction term formed from level variables t-1 is additionally attached. If this relationship proves to be a cointegration relationship, the elements in the model itself must also be stationary. In this way, an VEC model, the error correction model is derived, on the basis of which the VEC model VAR can be constructed. If we need to test cointegration, we basically want to find out the number of cointegration relations in the VAR model under investigation. Engle and Granger (Citation1987) designed the original time series cointegration testing. This test was based on the assumption of estimates of type I (0) least squares. These residuals are obtained from the model
Cipra (Citation2008) then presents the null hypothesis of a modified Dickey–Fuller test in the form
The difference from the classical Dickey–Fuller test is that the estimated residuals are being used, which modifies the critical values of the test (Engle and Granger Citation1987 or Bali, Engle, and Murray Citation2016). Engle (Citation2016) expands the approach on the dynamic conditional beta. This is an approach to estimating regressions with time varying parameters. The conditional covariance matrices of the exogenous and dependent variable for each time period are used to formulate the dynamic beta.
Engle (Citation2016) developed an estimation of the covariance matrices and other regression parameters. Tests of the hypothesis that betas are constant are non-nested tests, and Engle (Citation2016) developed several approaches, including a novel nested model. The methodology is applied to industry multifactor asset pricing and to global systemic risk estimation with non-synchronous prices.
The tests based on the Johansen test (named after Søren Johansen) seem to be very reliable (Dwyer Citation2015). According to Cipra (Citation2008), the method is based on the M-L estimation of canonical correlations, which in the VEC model measure the partial dependence between m – dimensional vectors ∆yt and yt+1 at fixed values of vectors ∆yt-1, …, ∆yt-p+1. These canonical correlations are the square roots of the eigenvalues λ1, …, λm of a matrix that is closely related to the Π matrix, but is positively semidefinite. So for the, respectively, M-L estimates of these eigenvalues based on y1, …, yn hold
Johansen tests test for zero values of eigenvalues Īi based on L – R tests, which are based on a plausibility ratio. Cipra (Citation2008) describes the Johansen test as follows:
This test is a composite test of the null hypothesis with the maximum number of r cointegration relationships. An alternative hypothesis is a greater number of cointegration relationships.
Pedroni Panel Cointegration
The traditional approach to panel data samples, developed by Peter Pedroni, is the Pedroni panel cointegration (Pedroni Citation1999, Citation2004), and new challenges and approaches to panel cointegration testing were also developed by Pedroni (Citation2019). Pedroni (Citation2019) does not weaken the assumption of the robustness of cointegration methods when temporal dependencies interact with both cross-sectional heterogeneities and dependencies. The main problem of the original method was solved.
We do see this challenge of generalizing to nonlinear and time varying cointegrating relationships. In the panel cointegration, we show that it is necessary to have all of the known estimators of the coefficients of econometric models inconsistent if their coefficients and error terms are not unique. Pedroni (Citation2019) presents models with unique coefficients and error terms, with specific applicability to the analyses of panel data sets. Swamy et al. (Citation2019) further asserts that the coefficient on an included nonconstant regressor of a model with unique coefficients and error term is the sum of bias-free and omitted-regressor bias components. This sum, when multiplied by the negative ratio of the measurement error to the observed regressor, provides a measurement-error bias component of the coefficient. The result is important because to measure the direct causal effect of an included nonconstant regressor of a model on its dependent variable, one needs the bias-free component of the coefficient on the regressor.
Returning to the basic traditional Pedroni approach, residual tests solve the problem of recognizing zero cointegration on panel data. These panel data assume the heterogeneity of the compared indicators in terms of both short-term dynamics and the size of indicators over a long period. Furthermore, we assume the possibility of combined intra-dimensional tests or group tests. Pedroni’s analysis further allows to derive a limiting probability distribution, but also to determine whether this stratification has normal characteristics. In addition, in the following description of the model, we will freely follow Pedroni (Citation2004). In its simplest form, we must begin with a regression of the type:
for the panel-watched time series of output variables yit. Index i = 1, ., N denotes the elements to be examined, and index t = 1, ., T, denotes the time periods. Xit is a m – dimensional column vector for each element i and βi is a m – dimensional row vector for each element i. The variables yit and Xit are both integrable in the first order I(1), for each element i of panel data. Moreover, for these variables, we assume non-integration with eit residuals, which are also of type I (1). Parameters ∝i and δi are included to record the existence of specific influences, respectively, deterministic trends. βi can be variable within a set of elements. Such assumptions sufficiently ensure the heterogeneity of the cross-sectioned elements in the panel. For regression (43) Pedroni (Citation2004) tests the hypothesis H0: “all elements from the panel are not cointegrated.” An alternative hypothesis is H1: “all panel elements are cointegrated.” Of course, with such formulations, we must assume that all panel elements are either uniformly cointegrated or uniformly non-integrated. Without such an assumption, Pedroni’s analysis would be significantly complicated. For such cases, the alternative hypothesis H1 would be tested: “a significant part of the panel elements is cointegrated.” This is different from previous ideas, when cointegration in panel data assumed that coefficients βi are homogeneous for all elements of the analysis. In such cases, assuming the strict exogenity of the regressors, the probability distribution of the residual-oriented zero cointegration tests is asymptotically equivalent to the probability distribution of the panel root unit tests. Such a claim is possible even in situations where residuals as such are estimated quantities. If the regressors are endogenous, the above does not apply. The probability distribution in the form of asymptotic equivalence assuming stable (homogeneous) values of coefficients βi is not identical to the probability distribution of panel root unit tests (Baltagi, Feng, and Kao Citation2016).
The problem of panel cointegration really arises with the assumption of homogeneity of regressor coefficients. In this case, the estimated residues for any element of the panel may be marked as non-stationary, even though they are cointegrated. For these reasons, it is necessary to perform several statistical tests based on residual testing for the absence of time series cointegration that will not depend on regressor coefficients. Such tests will convince us that the model from the beginning is not limited by the assumption of the same regressor coefficients. These tests are described in Appendix 1.
Data Analysis
Our dataset is a time series from the Patria database (Czech database of financial intermediator) of the interest rates. In the Patria database, we can gain the term rates as forward rate agreements (FRA) and interest rate swaps (IRS). In our research, we used only FRA contracts among different countries and different maturities. The time series are three-month interest rates from the Czech Republic (CZ_3 m), Poland (PO_3 m), Hungary (HU_3 m), and the United Kingdom (UK_3 m). We also used the FRA contracts from the same countries but with different future maturities. These are in the Czech Republic three-month FRA contracts payable for the next month (CZ_1_4), for the next three months (CZ_3_6), and for the next nine months (CZ_9_12). Moreover, we analyzed different maturities of FRA contracts from Poland, Hungary, and United Kingdom. For these countries, we also used the same notation. The data sample range is from June 20, 2003 to May 20, 2019. The sample range could seem like out of sample. There are two reasons. First, we excluded data from the COVID-19 pandemic period. The financial time series from this period nowadays behaves like statistic outliers, and we do see dramatic, unexpected changes quite frequently (lately, bitcoin prices have fallen dramatically). Secondly, the research itself, including the working paper presentation, was conducted one year ago. Moreover, the main goal of this paper is not to provide forecasts. The paper is primarily a comparative study of the evolution of the expectation hypothesis in Central European economies and the United Kingdom.
The time series consists of 4,208 daily observations (trading days). To achieve better statistical features, we transformed the daily time series into monthly averages. For further analysis (better statistical features, but with certain economic sense), we transformed data (month moving averages) from time series into logarithms. Totally, our data sample consists of 193 observations.
From the data features output, it is clear that the interest rates and forward interest rates are nonstationary. For all of the time series analyzed, we are unable to reject the null hypothesis regarding the 0.05 statistical significance of the existence of the unit root (ADF test). Moreover, for all of these time series, we confirm the hypothesis about the abnormal values of particular time series (Jarque-Bera test). Needless to say, even the logs of the time series do not reject the ADF hypothesis. All of the time series observed are not of the first order of integration. The interest rates and forward interest rates are even in their logarithms nonstationary. Notably, the use of unit root tests to distinguish between trend and difference stationary data has become an essential tool in applied research in the last few decades.
In order to analyze the stochastic properties of time series analyzed more precisely, we ran the unit root test with breaks. As Perron (Citation1990) points out, structural change and unit roots are closely related, and researchers should bear in mind that conventional unit root tests are biased toward a false unit root null when the data are trend stationary with a structural break. Finally, there are several types of modified augmented Dickey-Fuller tests that allow for levels and trends that differ across a single break date (or even two breaks).
For our analysis, we used Zivot-Andrews unit root test. Original Zivot and Andrews (Citation2002) was created according to the following features. It tested statistics of value −4,48, breakdate 2001Q3, H0 Unit root series with drift, HA Stacionary process with one-time break in the level, and the conclusion accordingly rejected the null at the 10% level
From the output in , we see that there is a structural break in the time series of the United Kingdom. In all the data samples from the UK (FRA rates with different maturities) and the 3 M spot interest rates, we observe a significant structural break in the 2008m09 period. When exploring this fact, we can assume the UK time series to be stationary. In these time series, we can use the general regression analysis and test the residuals. From these concluding remarks, there is assumed a sufficient output from linear regression analysis, but the residuals suffer from a great autocorrelation. This altogether led to the martingale-behavior concluding statement and the predictive power of the forward rates. This statement corresponds to the statement of the original ADF tests and the more complicated VEC analysis.
Table 1. Zivot-Andrews Unit Root Test with a break, whole data sample range, 213 observations.
However, there is another interesting concluding remark for the Polish samples of the time series. The original Zivot-Andrews calculates the 10% statistical significance. The significance reached all of the samples from the Polish economy (FRA rates, future one, three- and nine-month 3 M forward rates, and 3 M spot interest rate). The final statements are the same as for the UK stated before, but for the Polish situation the statistical significance is exactly 10%, whereas for the UK samples it is always less than 1%.
The linear regression approach is useless, although in many studies their authors used this linear regression approach unafraid about the potential misinterpretations caused by the spurious regression. The only way to solve the problem from the economic theory perspective is to find the cointegration equation between the nonstationary time series. When this time series exists, it is stationary and the cointegration equation makes economic sense. Under these assumptions, we can confirm a theoretical conclusion (in our analysis about the forward interest rate as an unbiased predictor of the future spot 3 M interest rates) even using the nonstationary time series.
It is very interesting to analyze constructed forward yield curves. Only for the UK do we observe a long-term increasing FRA yield curve according to the mean value (2.191, 2.199, 2.300). In the data sample of the Czech Republic, we observe (1.547, 1.510, 1.720). Here, there is an inverted FRA yield curve according to their means. The decreasing FRA yield curve shape we observe in the data sample of Hungary (4.57, 4.509, 4.423) and in Poland (3.795, 3.772, 3.655). These data output are averages in the observed countries for 16 years (observed monthly). Therefore, these are general trends in FRA yield curves in observed countries during the pre-crisis, crisis, and post-crisis periods. The presented conclusion is not too surprising because we observe many shapes in yield curves in general.
This great financial crisis started in 2008 in the US economy and affected all developed countries. The effects were different in different countries. For instance, the Polish economy recovered immediately. In the Czech Republic, two recessions appeared: one in 2009 and one from 2011 to 2013. Notably, the lowest FRA interest rates are in general in the Czech Republic and the highest are in Hungary. Very high rates also appear in Poland. The UK’s FRA rates are in general lower but not lower than those in the Czech Republic.
Analysis, Cointegration, and the Vector Error Correction Method (VEC)
From the previous , it is clear that the standard OLS linear regression methods are useless; even these methods would, at first sight, provide useful theoretical coefficients. For nonstationary time series, we expect so-called spurious regression. We do see an overly high level of autocorrelation and abnormally distributed residuals, but a suitably low level of heteroscedasticity in residuals. This all confirms a strong relationship between forward-rate interest rates (FRA) and future-spot interest rates.
Table 2. Time series features, whole data sample range, 213 observations.
Also from , it is clear that the time series analyzed are all nonstationary. Moreover, we see that the evolution of the spot interest rate and forward interest rate for a particular country is quite similar.
Nonstationary time series can have a common stationary equilibrium (cointegration equation). Their residuals must be tested to be i.i.d (difference between the spot interest rates and three-month lagged forward interest rates, or longer further lags for longer FRA maturities). The Johansen cointegration test indicates the total number of possible cointegration vectors.
Empirical analysis concluded that all trends are stochastic. Under these assumptions, we confirm the existence of a relationship between the two nonstationary time series analyzed in three of the four countries analyzed (in the Hungary time series, we rejected the cointegration hypothesis for all FRA contracts differed according to maturity). In the Czech Republic, the United Kingdom, and Poland we confirmed the existence of the cointegration equation (in an economic sense, the relationship) between the forward interest rate and the future spot rate for the one month, three months, and nine months of term interest rates (FRA contracts). The Johansen cointegration test, together with the Trace test, confirms one cointegration equation. These tests reject the null hypothesis about “no” cointegration vector and furthermore (these tests) are unable to reject “at most one” cointegration vector. This is why we need to run a more sophisticated cointegration test: the vector error correction (VEC) model. Moreover, we need to discuss avoiding the factor-augmented vector autoregressive (FAVAR) modeling approach. The FAVAR model is widely used in macroeconomics and finance. In the model, observable and unobservable factors jointly follow a vector autoregressive process, which further drives the co-movement of a large number of observable variables (Bai, Li, and Lu Citation2016).
While the factor-augmented error correction (FECM) model remains better than the FAVAR model in most of the cases. The bias in estimated impulse responses in a FAVAR model is positively related to the strength of the error correction mechanism and the cross-section dimension of the panel. On the other hand, simpler models such as a vector error-correction model (VEC) or even an autoregressive (AR) can become tough competitors when the explanatory power of the error correction terms and/or of the factors is reduced or the sample size is not large. This is also the reason why the relative rankings of the models are not always clear-cut, and why the forecasting performance of the FECM should also be evaluated in a large set of empirical applications. For forecasting the real variables for the United States, the FECM is systematically better than the FAVAR and the VECM over both the samples considered. This is not necessarily true for the nominal variables, where the results are more sample dependent (Banerjee, Marcellino, and Masten Citation2014). That is why, we finally decided to use the VEC approach.
In contemporary economic theory, we usually do not use sophisticated methods to explain dynamics in economic variables. We need to help ourselves with the theory of time series. Despite the economic theory, the variables are both frequently empirically endogenous. These variables influence themselves without any sense of causality. The vector autoregression (VAR) analysis assumes that all of the variables are endogenous. This approach models the influence of shock as a whole, not particularly. In the following analysis, we would like to rehabilitate the EMH hypothesis, in which the forward rate is included.
First, we tried several VAR models, each of which was different due to the lag structure. The output was always similar at first sight. Both coefficients in the spot interest rates and forward interest rates were achieved with no such efficient standard errors and t-statistics. In the further diagnosis, we have proven that the VAR approach is useful. From the table (graph) in EViews of the AR roots, we concluded that all of the roots lie inside the unit circle. This is the condition needed for further impulse: response diagnosis.
Vector error correction (VEC) models are used for nonstationary time series, which we know are cointegrated. For our purposes, in which we confirmed that the time series were nonstationary and that their common equilibrium is stationary, we can run the VEC model. VEC models restrict the long-run behavior of the endogenous variables in the way of limitation by the correction term. Observed time series behave according to a cointegration relationship, but this relationship is constantly changing. In other words, error correction models provide as a first step the cointegration Equationequation (53)(53)
(53) , which is corrected with the VAR of the correction term (54).
Needless to say, we assume no lag in the correction term. Finally, there is only cointegration correction, not lagged. The previous is explainable due to the fact that financial variables usually follow not adaptive but rather rational expectations. We have calculated the VEC output for all of the time series analyzed (four countries, in each three FRA maturities). These set of twelve results are viewed in .
Table 3. Time series features, whole data sample range, 213 observations.
The general VEC output using equation No. (53) and (54) is, according to for a particular country and maturity, modified. For instance, for the United Kingdom, for the FRA contract for 3 M, the interest rate after nine months (UK_9_12) is:
In this model, all of the coefficients are statistically significant. They achieve low standard errors and relatively high t-statistics (the p-values confirm the rejection of the null hypothesis about the coefficients to be zero). The potential constant has its own explanation with the existence of the transaction costs (or, for our purposes, the term premium) in the forward interest rates market.
From the EMH and the pure expectations hypothesis, we assumed the value of unity in the regression coefficient and zero value for the constant. In all of the statistical tests made, the corresponding spot interest rate achieved the approximate unit value. Despite this, the negative constant makes no economic sense in the way of transaction cost (it should be a term discount, but nowadays we must get used to seeing many economic miracles).
In the long period, the dynamics of the spot interest rates and forward rates provide more expectable features. These features are still very different from the EMH hypothesis in its weak form, in which the forward rate should serve as an unbiased predictor of the future spot interest rates with respect to corresponding maturities. According to Cuthbertson and Nitzsche (Citation2005), the possible constant refers to the minus value of term premiums. Needless to say, the EViews output and our discussed remarks correspond to this statement because it originated on the opposite regression equation side. Under a constant term premium assumption, Cuthbertson and Nitzsche (Citation2005) expected that no other variables dated t or earlier are statistically significant in the equation No. 9. But if the term premium is variable, and furthermore, if it is correlated with the forward premium, then OLS produces biased estimations. For short horizons (two- to six-month maturities), Mishkin (Citation1988) concluded that in the data sample of US treasury bills from 1959 to 1982, the forward premium had only small predictive power (a coefficient of only 0.4). As the time to maturity extended, there is no predictive power of the forward rate contracts to the corresponding spot interest rates.
These conclusions led to the rejection of the expectation hypothesis in the very short end of the maturity spectrum. Despite this, Fama and French (Citation2018) presented the results of robust research for longer horizons (spot rates dependent on US treasury bills) from one to five years’ maturity. Fama and French rejected the zero coefficient, but in most of the tests they also rejected the coefficient to be of unit value.
Moreover, Cuthbertson and Nitzsche (Citation2005) are unable to reject the standard unit predictive coefficient for four-year changes in the one-year spot interest rates. Namely, the literature review provided that in short-term horizons (less than four years) according to the expectations hypothesis, spot interest rates perform badly. But they behave the same way they do in longer maturities.
Coulibaly and Kempf (Citation2019) ran research based on quarterly data on 31 emerging countries (among which 16 are inflation targeting countries) from 1990Q1 to 2014Q3, obtaining strong support for the conjecture that the implementation of inflation targeting weakens the Fisherian relation between expected depreciation and the interest rate differential (uncovered interest parity condition) and thus is conducive to the appearance of the forward bias puzzle in emerging countries. They show that this reflects the performance of inflation targeting regimes in lowering the level and volatility of inflation. Our finding holds when controlling for country-specific effects, time-specific effects, global disinflation, exchange rate management, crises, and the use of different econometric techniques.
Pedroni Panel Cointegration Testing
To make our conclusions more plausible, we also transformed the original data in the panel data. Here, we can run a few verifying tests for the data sample as a whole for different countries. These tests differ only among the maturity of FRA contracts. All of the statistical tests rejected the null hypothesis that there is no cointegration among the panel data. This is viewed in . There was the same conclusion in any term of maturity FRA contracts. In summary, we ran a statistical test and rejected the null hypothesis on a statistical significance value of 5% (0.05). Moreover, these tests would have gone further (even 0.001). We must then be very careful about the type II error. This statistical error is the non-rejection of a false null hypothesis (also known as a “false negative” finding or conclusion, it is “the liberation of a criminal”). The probability of a type II error increases as the statistical significance value decreases. In this sense, it is necessary to use data samples with a very high number of statistical observations, which, despite the previous statement, decreases the probability of the type II error. For this reason, we constructed this hypothesis to reject the null hypothesis. In this sense, we made the right decision to “condemn the innocent” with the probability 0.05.
Table 4. Pedroni Residuals, Cointegration Test.
Discussion
Under the expectations hypothesis, no trader can achieve abnormal risk-free returns by combining FRA contracts with different maturities. The hypothesis is based on the no arbitrage principle and on rational expectations. The previous hypothesis is called the pure expectations hypothesis (PEH). The expectations hypothesis, assuming risk-neutral investors and the term premium (risk premium for the FRA interest rates market), is called the expectations hypothesis, assuming the liquidity preference (EH). The effect of this hypothesis is that the forward rate should be an unbiased predictor for the future spot interest rate due the specified time in the forward contract. The difference between these two economic variables is the term premium.
On the data sample of 4,028 observations from 2003 to 2019, we run statistical tests available in the EViews statistical software for the nonstationary time series to confirm/reject the EH. These tests were cointegration tests, VEC method tests, and the Pedroni panel cointegration tests. These tests falsified the expectations hypothesis with liquidity preference (market efficiency hypothesis) no matter how far the time to maturity of contract is. We do see in time highly variable, unexpected term premiums. But we found that there is a strong long-run relationship between the FRA contract and its corresponding future spot interest rate. The residuals are not i.i.d (because of fluctuating variance). Despite the EH falsification, we can confirm that the residuals follow a martingale stochastic process. This means that the best future estimation of an economic variable is its current value. It does not refer in the same way to the market efficiency hypothesis (EMH) or the expectations hypothesis (EH).
The differences from the analyzed relationship can be explained by the transaction cost (its amount is changing more). So we theoretically confirm a standard Keynesian (New Keynesian) approach to financial markets, first investors’ spirits (animal mood) and secondly, the financial capital limitations for new investors and other reasons for so-called rational bubbles. In further research, this problem is technically solvable thanks to nonlinear equilibrium adjustment methods.
Moreover, we theoretically described an explanation of the puzzle thanks to the representative agent model C-CAPM. One byproduct of this paper is our conclusion about the fundamental analysis being useless in the FRA trading in the short run (more likely even in long run), contrary to the technical analysis trying to find stochastic trends as a basis for trading. This altogether means that the technical analysis of trading in financial markets is preferred to the fundamental analysis.
Empirical evidence from the observed V4 economies provides very useful statements. In the Czech Republic, the limited effect of the central bank discount rates to forward and swap rates has been caused by disinflationary policies in many recent years. In Poland, the limited effects of the relationship between forward and spot interest rates are caused by the negative term premium, as foreign investors were unable to trade due to the restricted supply of short-term treasury bonds. In Hungary, there are problems with not-matured treasury bonds market and more the government provided the self-financing program. Although there appear to be many influences (puzzles) such as those mentioned, the economic research in any country calculates in general the forward rates as a result of the term structure of the spot interest rates. Moreover, the spot interest rate for further time in maturity is the combination of short-term interest spot rates plus the combination of the term premiums. We do see some potential problems from implicit interest spot rates as a byproduct of bootstrapped forward interest rates. Moreover, in the frequent research in the V4 economies, there are results about the future market expectation from the changes in the yield curves (of the obligations and treasury bonds). We do see many important unexpected influences, such as a financial crisis, floods, and recently the COVID-19 pandemic. In the Czech Republic, we observe the tendency of the spot interest rates of the money market being more dependent on the foreign rates than on the basis rates of the Czech National Bank. These combined influences the empirical conclusions from this work, falsifying standard economic relationships and confirming the martingale behavior for economic variables. Those innovative tools lower the risk for market participants. Combined with slower productivity growth and a surplus of savings, these tools push down long-term yields through the expectation component in an unnatural way.
Wang and Bilson (Citation2017) offered an empirical investigation of Eastern European bond markets. They examine the value of emerging Eastern European bond markets to global fixed income managers. In an environment where traditional developed markets bonds offer modest yields, emerging market bonds with attractive yields are becoming more popular with institutional managers. Furthermore, the returns on these bonds exhibit low correlations with traditional fixed income investments, and thus offer opportunities for portfolio diversification. Wang and Bilson (Citation2017) developed a multifactor forecasting model and estimated its parameters using a dynamic Kalman filter procedure. Forecasts are then used to construct optimal mean–variance portfolios with and without emerging market bonds. They also find that the portfolios that include emerging market bonds have significantly higher Sharpe ratios. Wang and Bilson (Citation2017) focus especially on the long-term government bond market to test the movement.
To highlight the effects of the great economic recession 2008 we used the paper Filipozzi and Staehr (Citation2013). They analyze the empirical validity of the covered interest rate condition (CIP) in four major CEE countries with floating exchange systems. The main focus is on the impact of the global financial crisis and the possible causal links between the crisis and deviations from the CIP (term premiums). In the period before the global financial crisis, the term premium condition is largely satisfied for the three more advanced countries in the sample but not for Romania, which has seen relatively sluggish financial development. After the outbreak of the crisis, the CIP does not hold for any of the four sample countries, but there are substantial differences between the countries. In the Czech Republic, the deviation from CIP is generally small and even negative at times. In Hungary and Poland, the deviation from CIP is greater, but while it gradually decreased in 2009–11 in Poland, it increased in Hungary (Filipozzi and Staehr Citation2013). Finally, in Romania, the deviation from CIP is very large, thus being in line with the period before the crisis.
Interestingly, the deviations from CIP closely correspond to the interventions in foreign exchange markets undertaken by central banks after the global financial crisis. The Czech central bank did not deem it necessary to intervene, as market-based arbitrage continued to function reasonably well. Hungarian and Polish central banks entered the foreign exchange swap markets to counteract the effects of limited private liquidity. The Romanian central bank did not take any direct measures, as forward exchange markets never played a major role, which is also reflected in the large deviations from the CIP before the crisis. The deviations from CIP can be linked to global and idiosyncratic, local risks, but the pattern differs between countries. For the Czech Republic, global risks that do not spread to the local risk measure appear to reduce the risk premium (and thus the term premiums, Bauer and Mertens Citation2018); for Hungary and Poland, both global and idiosyncratic, local risks lead to higher risk premiums; and in Romania, deviations from CIP do not depend on the risk factors in the model (Filipozzi and Staehr Citation2013).
In the precrisis period, there were clear differences between the three economically advanced countries in the sample, the Czech Republic, Hungary, and Poland – and the least developed country, Romania. The econometric analysis suggests that the differences are partly due to the low degree of financial integration and the perceived riskiness of investment in Romania. With the outbreak of the global financial crisis, the covered interest parity condition (CIP) generally ceases to hold, but at the same time differentiation across the three more advanced countries increases. The Czech Republic exhibits features of an advanced economy or even a safe haven, while Hungary and Poland are more characteristic of emerging market economies, where increased riskiness and reduced liquidity make investors flee the country (Filipozzi and Staehr Citation2013).
In the UK, the situation is different than it was in the V4 countries. In the UK, we can statistically significantly deduce forward rates, which are being empirically realistic and we do see them as the real trades. In the UK, after the GFC (the global financial crisis) of 2008, the next decade is solved the quantitative easing features, forward guidance, and negative real interest rate effects.
The empirical analysis we have made in the data sample of the V4 countries and United Kingdom has shown many useful conclusions. In all of the countries analyzed, the main question is whether the null hypothesis is rejected or not. The null hypothesis is a standard expectation hypothesis with liquidity preferences (included the term premium) for interest rates with different maturities (or for corresponding bonds). This hypothesis is a concretization for the interest rates/bond market of the general market efficiency hypothesis. In every country, we try to find the relationship between forward interest agreement (FRA) rates and corresponding future spot interest rates. All of the time series are nonstationary, so that we had to use sophisticated methods such as time series cointegration, the vector error correction method, and the Pedroni panel cointegration. Except for Hungary (for which the data was incomplete), we confirmed the existence of one cointegration relationship (the stable stationary common combination). The expected increasing term premium with the further date to maturities appeared only in the UK time series.
The main empirical conclusion of this paper is that we can create a model of the forward interest rate (FRA) to the spot interest rates corresponding by the day to maturities for every particular country analyzed (the Czech Republic, Poland, Hungary, and the UK). The only suitable method for those nonstationary time series is the VEC method. From the long-period point of view, only in the UK is the increasing yield curve confirmed. The predictable options for term premiums in the long run are also confirmed. In Central European countries, we are unable to confirm this feature. In the Czech Republic, there are decreasing and then increasing term premiums. In Poland, there are negative term premiums from the long-period point of view. In Hungary, the term premiums are positive, but the evolutions of term premiums are similar to those observed in the Czech Republic (but the inverse in the yield curve is strengthened, with a more twisted yield curve in the long run). The data altogether as panel data are rejected using the Pedroni panel cointegration method. The particular countries’ explanations are described in the empirical evidence mentioned above. Finally, the data sample concluded that term premium predictions were impossible (using the FRA rates) in Central European countries, contrary to the situation in the UK.
The explanation of this impossibility in Central Europe is caused by many monetary and fiscal factors. But these factors are very different among central European countries. Let us discuss the modern explanation of the negative term premiums in Poland but also elsewhere. The term spread, the difference between long-term and short-term interest rates, is a strikingly accurate predictor of future economic activity. Every US recession in the past 60 years was preceded by a negative term spread, that is, an inverted yield curve. Furthermore, a negative term spread was always followed by an economic slowdown and, except for one time, by a recession. Although the current environment is somewhat special, with low interest rates and risk premiums, the power of the term spread to predict economic slowdowns appears intact. A negative term spread, that is, an inverted yield curve, reliably predicts low future output growth and indicates a high probability of recession (Rudebusch and Williams Citation2009). This relationship holds not only in the United States but also for a number of other advanced economies (Estrella and Mishkin Citation1997). The term spread is one of the most reliable predictors of future economic activity among a wide range of economic and financial indicators and, as such, is closely watched by professional forecasters and policymakers alike (Bauer and Mertens Citation2018). A simple rule of thumb that predicts a recession within two years when the term spread is negative has correctly signaled all nine recessions since 1955 and had only one false positive, in the mid-1960s, when an inversion was followed by an economic slowdown, but not an official recession. The delay between the term spread turning negative and the beginning of a recession has ranged between 6 and 24 months (Bauer and Mertens Citation2018). But a number of observers have suggested that a low or even negative term spread may be less of a reason to worry than usual, arguing that historical experiences do not necessarily apply to the current situation. One factor that is different from before is that, despite some recent increases, the level of interest rates is low by historical comparison (Bauer and Glenn Citation2016). The argument goes that increases in the short-term policy rate may slow down the economy less than usual in such an environment. Similarly, given the currently low level of the natural policy rate (Williams Citation2017), a closely related argument suggests that low long-term rates do not necessarily reflect a pessimistic economic outlook, but rather a new normal for interest rates. The risk premium in long-term interest rates, called the term premium, has been close to zero or even negative, according to various different estimates (Bauer and Glenn Citation2016). This is relevant for interpreting the term spread because long-term rates reflect both this term premium and expectations of future short-term rates (Bauer and Mertens Citation2018). If long-term rates, and hence the term spread, are low because of a low risk premium, this may have different implications for the economic outlook than if they are low because of pessimistic expectations about future interest rates. Although the current environment appears unique compared to recent economic history, statistical evidence suggests that the spread of signal in the term-spread is not diminished. These findings indicate concerns about the scenario of an inverted yield curve. Any forecasts that include such a scenario as the most likely outcome carry the risk that an economic slowdown might follow soon thereafter.
Conclusion
The aim of this paper was to decide whether or not term premiums appear and thus are predictable in Central European countries in the data samples of forward rate agreement interest rates and corresponding spot interest rates. On the data sample of 4,028 observations from 2003 to 2019, we ran statistical tests available in the EViews statistical software for the nonstationary time series to confirm/reject the EH. These were the cointegration test, the VEC method test, and the Pedroni panel cointegration test. Statistical tests falsified the expectations hypothesis with liquidity preference (market efficiency hypothesis), but we found that there is a strong long-run relationship between an FRA contract and its corresponding future spot interest rate. The differences from the analyzed relationship can be explained due to the existence of transaction cost (the more its amount is changing). So we theoretically confirm the standard Keynesian (New Keynesian) approach to financial markets in the way in which the main financial market limitation are first in the form of the investors’ spirits (animal mood) expansion, and second, the financial capital limitations for new investors (barriers to entry to the financial market, which are enormous). In further research, this problem is technically solvable thanks to nonlinear equilibrium adjustments methods.
Empirical evidence from the observed V4 economies provides very useful statements. In the Czech Republic, the limited effect of the central bank discount basic rates to forward and swap rates has been caused by disinflationary policy in recent years. In Poland, the limited effects of the relationship between forward and spot interest rates are caused by the negative term premium, as foreign investors were unable to trade due to restricted supply of the short-term treasury bonds. In Hungary, there are problems with not matured treasury bonds market and moreover, the government provided the self-financing program.
The empirical analysis we have made in the data sample of the V4 countries and United Kingdom has shown many useful conclusions. The null hypothesis is a standard expectation hypothesis with liquidity preferences (included the term premium) for the interest rates with different maturities (or for corresponding bonds). This hypothesis is concretization for the interest rates/bond market of the general market efficiency hypothesis. In every country, we try to find the relationship between forward interest agreement (FRA) rates and corresponding future spot interest rates. Except for Hungary (where the data was incomplete), we confirmed the existence of one cointegration relationship (the stable stationary common combination). The expected increasing term premium with the further date to maturities appeared only in the UK time series.
In the Czech Republic, there are decreasing and then increasing term premiums. In Poland, there are negative term premiums from the long-period point of view. In Hungary, the term premiums are positive, but the evolutions of term premiums are similar to those observed in the Czech Republic (but the inverse in the yield curve is strengthened, with a more twisted yield curve in the long run). Finally, the data sample concluded that term premium predictions were impossible (using the FRA rates) in Central European countries in contrast with the situation in the United Kingdom. The explanation of this impossibility in Central Europe is caused by many monetary and fiscal factors. But these factors are very different among Central European countries.
Although it seems that we rejected FRA rates being unbiased predictors of future spot interest rates with various maturities, we do not contend that the quantitative approach to finance and economics is useless. Conversely, the martingale stochastic process for analyzed term premiums confirms the actual approach to financial assets as a full random variable. But these random effects are explained statistically significantly using standard tools of quantitative finance (random walk, risk neutral pricing [probabilities], Itô’s lemma, Brownian motion, etc.) compared with the behavior economics approach (which is then unnecessary), and more with empirical effects of technical analysis for trading being more useful than the fundamental analysis of the particular financial asset.
Acknowledgments
This article was made thanks to the financial support of the Masaryk Institute of Advanced Studies, Czech Technical University in Prague.
Data Availability Statement
The author confirms that the data supporting the findings of this study are available within the article and/or its supplementary materials.
Disclosure Statement
No potential conflict of interest was reported by the author.
Additional information
Funding
Notes on contributors
Petr Makovský
Petr Makovský received his Ph.D. in Economics from the University of Economics in Prague in 2013. At the Masaryk Institute of Advanced Studies, Czech Technical University in Prague, he is responsible for the study program Economics and Management. Petr Makovský is interested in economics of exchange rates, in financial economics and in the national accounting statistics.
References
- Abbot, Tyler 2017. Heterogeneous Risk Preferences in Financial Markets (November 10, 2017). Paris December 2016 Finance Meeting EUROFIDAI - AFFI. doi:10.2139/ssrn.2786257.
- Akhigbe, Aigbe, Jeff Madura, and Marek Marciniak. 2012. “Bank Capital and Exposure to the Financial Crisis.” Journal of Economics and Business 64 (5): 377–92. doi:10.1016/j.jeconbus.2012.05.002.
- Bai, J., K. Li, and L. Lu. 2016. “Estimation and Inference of FAVAR Models.” Journal of Business and Economic Statistics 34 (4): 620–41. doi:10.1080/07350015.2015.1111222.
- Baillie, Richard T., and Tim Bollerslev. 2000. “The Forward Premium Anomaly Is Not as Bad as You Think.” Journal of International Money and Finance 19 (4): 471–88. doi:10.1016/S0261-5606(00)00018-8.
- Bali, Turan G., Robert F. Engle, and Scott Murray. 2016. Empirical Asset Pricing: The Cross Section of Stock Returns. Oxford; John Wiley & Sons. doi: 10.1002/9781118445112.stat07954.
- Baltagi, Badi H., Qu Feng, and Chihwa Kao. 2016. “Estimation of Heterogeneous Panels with Structural Breaks.” Journal of Econometrics 191 (1): 176–95. doi:10.1016/j.jeconom.2015.03.048.
- Banerjee, A., M. Marcellino, and I. Masten. 2014. “Forecasting with factor-augmented Error Correction Models.” International Journal of Forecasting 30 (3): 589–612. doi:10.1016/j.ijforecast.2013.01.009.
- Bauer, M. D., and D. Rudebusch Glenn. 2016. “Why Are Long-Term Interest Rates So Low?” FRBSF Economic Letter 36 (5):1-5.
- Bauer, M. D., and T. M. Mertens. 2018. “Economic Forecasts with the Yield Curve.” FRBSF Economic Letter 7:8–07.
- Bernanke, Ben, S. Thomas Laubach, S. Mishkin Frederic, and S. Posen Adam. 2018. Inflation Targeting: Lessons from the International Experience. Princeton; Princeton University Press. doi: 10.2307/j.ctv301gdr.
- Božović, M. 2020. “Uncovered Interest-rate Parity and Risk Premium: Evidence from EUR/RSD Exchange Rate.” Eastern European Economics 1–24. doi: 10.1080/00128775.2020.1840275.
- Bruna, Karel. 2006. “Disinflationary Monetary Strategy and Instability of the Forward Yield Curve: The Case of the Czech Republic, 1999–2005.” Post-communist Economies 18 (4): 459–78. doi:10.1080/14631370601008613.
- Brunnermeier, Markus K. 2016. “Bubbles.” Banking Crises, 28–36. London: Palgrave Macmillan. doi:10.1057/9781137553799_5.
- Brzeszczyński, Janusz, Jerzy Gajdka, and Ali M. Kutan. 2019. “Evolution of the Impact of the Interest Rates Changes Announced by Narodowy Bank Polski (NBP) on the Financial Markets in the High, Medium and Low Level of Interest Rates Environments in Poland.” No. 303. Narodowy Bank Polski, Economic Research Department.
- Cipra, T. 2008. Finanční ekonometrie (Vol. 30). Praha, Czech Republic: Ekopress. doi: 10.1007/978-3-030-46347-2
- Coulibaly, Dramane, and Hubert Kempf. 2019. “Inflation Targeting and the Forward Bias Puzzle in Emerging Countries.” Journal of International Money and Finance 90:19–33. doi: 10.1016/j.jimonfin.2018.09.003
- Csajbók, Attila, András Hudecz, and Bálint Tamási.2010. Foreign Currency Borrowing of Households in New EU Member States. No. 87. MNB Occasional Papers.
- Cuthbertson, Keith, and Dirk Nitzsche. 2005. Quantitative Financial Economics: Stocks, Bonds and Foreign Exchange. Oxford: John Wiley & Sons.
- Dell’Ariccia, Giovanni, Pau Rabanal, and Damiano Sandri. 2018. “Unconventional Monetary Policies in the Euro Area, Japan, and the United Kingdom.” Journal of Economic Perspectives 32 (4): 147–72. doi:10.1257/jep.32.4.147.
- Dwyer, Gerald P. 2015. “The Johansen Tests for Cointegration.” White Paper.
- Engle, Robert F., and Clive WJ Granger. 1987. “Co-integration and Error Correction: Representation, Estimation, and Testing.” Econometrica: Journal of the Econometric Society 55 (2): 251–76. doi:10.2307/1913236.
- Engle, Robert F. 2016. “Dynamic Conditional Beta.” Journal of Financial Econometrics 14 (4): 643–67. doi:10.1093/jjfinec/nbw006.
- Estrella, A., and F. S. Mishkin. 1997. “The Predictive Power of the Term Structure of Interest Rates in Europe and the United States: Implications for the European Central Bank.” European Economic Review 41 (7): 1375–401. doi:10.1016/S0014-2921(96)00050-5
- Fama, Eugene F., and Kenneth R. French. 2016. “Commodity Futures Prices: Some Evidence on Forecast Power, Premiums, and the Theory of Storage.” The World Scientific Handbook of Futures Markets 79–102. doi: 10.1142/9789814566926_0004.
- Fama, Eugene F., and Kenneth R. French. 2018. “Choosing Factors.” Journal of Financial Economics 128 (2): 234–52. doi:10.1016/j.jfineco.2018.02.012.
- Filipozzi, F., and K. Staehr. 2013. “Covered Interest Parity and the Global Financial Crisis in Four Central and Eastern European Countries.” Eastern European Economics 51 (1): 21–35. doi:10.2753/EEE0012-8775510102.
- Frankel, Jeffrey A. 2017. “The currency-plus-commodity Basket: A Proposal for Exchange Rates in oil-exporting Countries to Accommodate Trade Shocks Automatically.” doi:10.2139/ssrn.3024910.
- Froot, Kenneth A. 1993. Currency Hedging over Long Horizons. No. w4355. Cambridge, Massachusetts: National Bureau of Economic Research.
- Gertler, Mark, and Peter Karadi. 2015. “Monetary Policy Surprises, Credit Costs, and Economic Activity.” American Economic Journal: Macroeconomics 7 (1): 44–76. doi:10.1257/mac.20130329.
- Greenwood, Robin, Samuel Hanson, and Dimitri Vayanos. 2015. Forward Guidance in the Yield Curve: Short Rates versus Bond Supply. No. w21750. Cambridge, Massachusetts: National Bureau of Economic Research.
- Hansen, Lars Peter. 2017. “Time-Series Econometrics in Macroeconomics and Finance.” Journal of Political Economy 125 (6): 1774–82. doi:10.1086/694625.
- Hendrych, Radek, and Tomáš Cipra. 2018. “Self-weighted Recursive Estimation of GARCH Models.” Communications in Statistics-Simulation and Computation 47 (2): 315–28. doi:10.1080/03610918.2015.1053924.
- Hodrick, Robert. 2014. The Empirical Evidence on the Efficiency of Forward and Futures Foreign Exchange Markets. vol. 24. Oxfordshire: Routledge. doi:10.4324/9781315014593.
- Jablecki, Juliusz, Andrzej Raczko, and Grzegorz Wesolowski. 2016. “Negative Bond Term premia-a New Challenge for Polish Conventional Monetary Policy.” BIS Paper 89t.
- Joyce, Michael AS, Peter Lildholdt, and Steffen Sorensen. 2010. “Extracting Inflation Expectations and Inflation Risk Premia from the Term Structure: A Joint Model of the UK Nominal and Real Yield Curves.” Journal of Banking & Finance 34 (2): 281–94. doi:10.1016/j.jbankfin.2009.07.018.
- Kicsák, Gergely. 2017. “Developments in Government Interest Expenditure for Hungary, 2000–2015.” Financial and Economic Review 16 (1): 46–73.
- Kladıvko, Kamil. 2010. “The Czech Treasury Yield Curve from 1999 to the Present.” Czech Journal of Economics and Finance 60 (4): 307–35.
- Kladivko, Kamil, and Pär Österholm. 2019. “Market Participants’ Forecasts of Financial Variables–Can Survey Data Outperform the Random Walk?” No. 2019: 10.
- Kočenda, Evžen, and Michala Moravcová. 2019. “Exchange Rate Comovements, Hedging and Volatility Spillovers on New EU Forex Markets.” Journal of International Financial Markets, Institutions and Money 58:42–64. doi: 10.1016/j.intfin.2018.09.009
- Kučera, Adam, Michal Dvořák, and Zlatuše Komárková.2016. “Decomposition of the Czech Government Bond Yield Curve.” CNB, Financial Stability Report 2017.
- Leković, M. 2018. “Evidence for and against the Validity of Efficient Market Hypothesis.” Economic Themes 56 (3): 369–87. doi:10.2478/ethemes-2018-0022
- Lewis, Karen K. 2011. “Global Asset Pricing.” Annual Review of Financial Economics 3 (1): 435–66. doi:10.1146/annurev-financial-102710-144841.
- Liu, Peter C., and Gangadharrao S. Maddala. 1992. “Rationality of Survey Data and Tests for Market Efficiency in the Foreign Exchange Markets.” Journal of International Money and Finance 11 (4): 366–81. doi:10.1016/0261-5606(92)90030-2.
- Mariusz, Jarmuzek, Lucjan T. Orlowski, and Artur Radziwill. 2004”Monetary Policy Transparency in the Inflation Targeting Countries: The Czech Republic, Hungary and Poland.” Case Studies and Analyses Working Paper 281. doi:10.2139/ssrn.1441163.
- Menkhoff, Lukas, Sarno Lucio, Maik Schmeling, and Andreas Schrimpf. 2017. “Currency Value.” The Review of Financial Studies 30 (2): 416–41. doi:10.1093/rfs/hhw067.
- Mishkin, Frederic S. 1988. “The Information in the Term Structure: Some Further Results.” No. w2575. National Bureau of Economic Research.
- Nagy, Krisztina. 2020. “Term Structure Estimation with Missing Data: Application for Emerging Markets.” The Quarterly Review of Economics and Finance 75:347–60. doi: 10.1016/j.qref.2019.04.002
- Pástor, Ľuboš, and Pietro Veronesi. 2009. “Technological Revolutions and Stock Prices.” American Economic Review 99 (4): 1451–83. doi:10.1257/aer.99.4.1451.
- Pedroni, Peter. 1999. “Critical Values for Cointegration Tests in Heterogeneous Panels with Multiple Regressors.” Oxford Bulletin of Economics and Statistics 61 (S1): 653–70. doi:10.1111/1468-0084.61.s1.14.
- Pedroni, Peter. 2004. ““Panel Cointegration: Asymptotic and Finite Sample Properties of Pooled Time Series Tests with an Application to the PPP Hypothesis.” Econometric Theory 20 (3): 597–625. doi:10.1017/S0266466604203073.
- Pedroni, P. 2019. “Panel Cointegration Techniques and Open Challenges.” In Panel Data Econometrics, 251–87, Cambridge, Massachusetts: Academic Press. Elsevier. doi:10.1016/B978-0-12-814367-4.00010-1.
- Perron, P. 1990. “Testing for a Unit Root in a Time Series with a Changing Mean.” Journal of Business and Economic Statistics 8 (2): 153–62. doi:10.2307/1391977.
- Phillips, Peter CB, and Hyungsik R. Moon. 1999. “Linear Regression Limit Theory for Nonstationary Panel Data.” Econometrica 67 (5): 1057–111. doi:10.1111/1468-0262.00070.
- Rogoff, Kenneth S. 2017. The Curse of Cash: How large-denomination Bills Aid Crime and Tax Evasion and Constrain Monetary Policy. Princeton: Princeton University Press. doi: 10.1515/9781400888726.
- Rossi, M., and A. Gunardi. 2018. “Efficient Market Hypothesis and Stock Market Anomalies: Empirical Evidence in Four European Countries.” Journal of Applied Business Research (JABR) 34 (1): 183–92. doi:10.19030/jabr.v34i1.10111.
- Rudebusch, G. D., and J. C. Williams. 2009. “Forecasting Recessions: The Puzzle of the Enduring Power of the Yield Curve.” Journal of Business and Economic Statistics 27 (4): 492–503. doi:10.1198/jbes.2009.07213.
- Stoupos, N., and A. Kiohos. 2017. “Post-communist Countries of the EU and the Euro: Dynamic Linkages between Exchange Rates.” Acta Oeconomica 67 (4): 511–38. doi:10.1556/032.2017.67.4.2.
- Swamy, P. A. V. B., P. von Zur Muehlen, J. S. Mehta, and I. L. Chang. 2019. “Alternative Approaches to the Econometrics of Panel Data.” In Panel Data Econometrics, 289–344. Cambridge, Massachusetts; Academic Press. Elsevier.
- Wang, J., and J. Bilson. 2017. “An Empirical Investigation of Eastern European Bond Markets.” Emerging Markets Finance and Trade 53 (1): 199–212. doi:10.1080/1540496X.2016.1172207.
- Wesoƚowski, Grzegorz. 2018. “Do long-term Interest Rates Drive GDP and Inflation in Small Open Economies? Evidence from Poland.” Applied Economics 50 (57): 6174–92. doi:10.1080/00036846.2018.1489507.
- Wickens, Michael. 2012. Macroeconomic Theory: A Dynamic General Equilibrium Approach. Princeton: Princeton University Press.
- Williams, J. C. 2017. “Interest Rates and the ‘New Normal.’” FRBSF Economic Letter 29. 1-6.
- Yellen, J. L. 2017. “Inflation, Uncertainty, and Monetary Policy.” Business Economics 52 (4): 194–207. doi:10.1016/0164-0704(89)90069-4.
- ZivotE., and D. W. K. Andrews. 2002. Further Evidence on the Great Crash, the Oil-price Shock, and the Unit-root Hypothesis. Journal of Business & Economic Statistics 20 (1): 25–44. https://doi.org/10.1198/073500102753410372
Appendix 1
The presented tests will convince us that the model from the beginning is not limited by the assumption of the same regressors coefficients. To analyze such tests, Pedroni (Citation2004) himself needs data generating the DGP process in the form of:
where:
and where the DGP process itself is based on a vector:
Moreover, we also assume that for each panel element i the following assumptions apply with respect to the dimension of the time series. These tests are also used in Swamy et al. (Citation2019) and extended. Now remember two basic principles of panel cointegration testing Baltagi, Feng, and Kao (Citation2016)
Assumption No. 1 – Invariance Principle
The stochastic process (44) can be replaced by formulation (47).
where for each element of panel i and T → ∞, and where the symbol → indicates weak convergence and Bi (Ωi) is the stochastic process of Brownian motion with asymptotic covariance Ωi. In addition, the diagonal block m x m fulfills the condition Ω22i> 0. Then, Bi (Ωi) is defined for all panel elements even in the same probability space. The invariance principle ensures the validity of the central limit theorem (for very long time intervals) individually for each element of the panel i. The stochastic deviation process must also follow the autoregressive moving average process (ARMA process). The asymptotic covariance matrix (m x 1) x (m x 1) is obtained as:
which can be broken down into:
where Ωi0 and Γi include simultaneous and dynamic covariance, respectively, for each element of panel i. The matrix is divided to match the dimension of the notation vector (134). This leads to a situation where Ω22i also contains a m x m dimensional matrix. Non-diagonal elements ΓiΓi´ capture feedback between regressors and explanations
Assumption No. 2 – Principle of cross-sectional independence
Individual processes should be independent and identically probable distributed (i. i. d). Therefore:
for all s, t, i ≠ j. More generally, the long-term asymptotic matrix of N x T scattering is diagonally positive definite with i diagonal blocks determined by asymptotic covariance for the ith panel member. The ζit process is based on a linear formula:
furthermore:
In (51) innovations follow the process of white noise ηit, while random coefficients Ci(L) are independent of each other, at the same time, both processes are i. i. d. in each dimension i and t. The principle of cross-sectional independence allows the application of a standard central limit theorem even in the presence of heterogeneous variations. Phillips and Moon (Citation1999) acquire Ci(L) on the basis of a distribution that is i. i. d. over i dimensions and at the same time independent of ηit innovations. The assumption Ωi> 0 ensures that there is no cointegration relationship between yit and Xit.