Abstract
Industry 4.0, big data, predictive analytics, and robotics are leading to a paradigm shift on the shop floor of industrial production. However, complex, cognitive tasks are also subject of change, due to the development of artificial intelligence (AI). Smart assistants are finding their way into the world of knowledge work and require cooperation with humans. Here, trust is an essential factor that determines the success of human-AI cooperation. Within this article, an analysis within production management identifies possible antecedent variables on trust in AI and evaluates these due to interaction scenarios with AI. The results of this research are five antecedents for human trust in AI within production management. From these results, preliminary design guidelines are derived for a socially sustainable human-AI interaction in future production management systems.
Practitioner summary: In the future, artificial intelligence will assist cognitive tasks in production management. In order to make good decisions, humans trust in AI has to be well calibrated. For trustful human-AI interactions, it is beneficial that humans subjectively perceive AI as capable and comprehensible and that they themselves are digitally competent.
Introduction
Today's manufacturing companies need to master increasing complexity, to realise shorter delivery times and to react to dynamic changes (Kuhnle, Röhrig, and Lanza Citation2019). Current planning and control mechanisms (e. g. mathematical optimisation and heuristics) cannot efficiently deal with these complex, dynamic, and unpredictable environments (Stricker et al. Citation2018). Here, data-driven approaches from the field of artificial intelligence (AI) may provide valuable solutions (Stricker et al. Citation2018; Kuhnle, Röhrig, and Lanza Citation2019). Some argue that AI will increasingly take over complex cognitive tasks and support decision making (World Economic Forum Citation2015; McAfee and Brynjolfsson Citation2017). In this sense, AI is the counterpart to robotics in manufacturing companies: while robots facilitate physical doing of blue-collar workers, AI will support cognitive deciding of white-collar workers (McAfee and Brynjolfsson Citation2017). The long-term estimation is that AI will transform the system of production management to a cyber production management system where human and artificial intelligence cooperate successfully (Burggräf, Wagner, and Koke Citation2018).
De Visser, Pak, and Shaw (Citation2018) point out that AI is different from previous automated systems: autonomously (model-based) instead of automatically (rule-based). Thus, AI can behave proactively, unexpectedly, and incomprehensibly for humans. Consequently, to establish productive and humane cooperation within a human-AI system under these circumstances, a particular design is required (Norman, Ortony, and Russell Citation2003; Haslam Citation2006; Riedl Citation2019; Shneiderman Citation2020). For humans to delegate control to others (either machines or fellow humans) requires trust in the respective counterpart. Accordingly, trust in the AI is the crucial factor for its broader acceptance (de Visser, Pak, and Shaw Citation2018; Siau and Wang Citation2018). Since AI is a new technology requiring new forms of interaction, existing models and theories on trust need to be verified in light of this new technology and revised if necessary (Detweiler and Broekens Citation2009; Farooq and Grudin Citation2016). The question of human trust in intelligent systems has been and is being investigated for several disciplines and applications. For example, in autonomous driving, the aim is to predict the driver’s trust based on factors such as gaze behaviour, culture, and gender (Hergeth et al. Citation2016; Hu et al. Citation2019), in order to adapt the vehicle’s behaviour automatically (Xu and Dudek Citation2015). In healthcare and homecare applications the tendency is to create transparent and adaptable systems (Rossi et al. Citation2017; Fischer, Weigelin, and Bodenhagen Citation2018). In safety-critical applications, such as airport security (Merritt et al. Citation2015) and military operations, the focus lies increasingly on the effects of errors on trust and performance (Rovira, Pak, and McLaughlin Citation2017).
The present article aims to empirically explore and examine antecedent variables on trust in AI within production management. Specifically, we derive an explanatory model, which describes important antecedent variables on trust and their operating mechanisms for the field of production management. This is a necessary first step towards the design of trustful human-AI cooperation in future cyber production management systems.
Background
In the future, AI will have the potential to take over knowledge work (McAfee and Brynjolfsson Citation2017), so that human activities may shift focus to problem-solving and monitoring activities, and more broadly on creative and emotion work (Dellermann et al. Citation2019). AI will support managers in coping with the increasing complexity and uncertainty in management. This requires interactive, cooperative decision-making mechanisms shared between artificial and human intelligence (McAfee and Brynjolfsson Citation2017). In line with Parasuraman, Sheridan, and Wickens (Citation2000), Burggräf, Wagner, and Koke (Citation2018) describes the forms of cooperation between AI and humans in production management in five stages: from sole human management via various degrees of assistance to fully autonomous decision-making by AI. Employing AI in production management has been subject of research since 1980 (Russell and Norvig Citation2016). This includes methods of operations research, such as fuzzy logic, genetic algorithms, or knowledge-based systems (Russell and Norvig Citation2016). Currently, there are three promising applications of AI in the field of production management. Firstly, in quality-based process control AI is able to reveal and learn dependencies between parameter combinations in process control and the resulting quality of the product, which are unknown today (Kuhnle, et al. Citation2017; Burggräf, Wagner, and Weißer Citation2020). If there is a digital twin for the real process, it is possible to use it as a simulation for reinforcement learning (RL) so that the algorithm learns the process control by itself (Jaensch et al. Citation2018). Thus, AI can replace classic control systems. Secondly, in predictive maintenance AI is capable of predicting machine failure based on the current machine status and machine history. The earlier this forecast is performed, the more time is available for preparation and downtime can be minimised. In the long run, predictive maintenance and further developments like prescriptive maintenance will even change the business model of the machines (Burggräf, Wagner, Dannapfel, et al. Citation2020). Thirdly, AI algorithms are increasingly being considered for scheduling/order dispatching. In this area of application, RL algorithms are receiving a significant amount of attention. They compete against the following: traditional algorithms of operations research (Gabel and Riedmiller Citation2008), heuristics of engineering science (e. g. first in first out, earliest due date) (Stricker et al. Citation2018; Kuhnle, Röhrig, and Lanza Citation2019), modified versions of themselves (Qu et al. Citation2016) and humans (Burggräf, Wagner, Koke, et al. Citation2020). Special characteristics of these current AI algorithms are that they can react very quickly to deviations, similar to heuristics or human decision making, and achieve a better solution quality than heuristics (Kuhnle, Röhrig, and Lanza Citation2019), but not as good as the slower optimisation methods (Gabel and Riedmiller Citation2008).
Despite all progress, it is unlikely that humans will be completely displaced in production systems in the near future. Thus, the new technology must be adopted by humans in order to maintain or better increase the overall performance. Due to its opaque, hard to predict and proactive nature, AI-based systems may be rather understood as a social counterpart than a classical tool by its users. Accordingly, people may think of it more akin to a (human) assistant or a team-mate. For this type of relationship trust is crucial (de Visser, Pak, and Shaw Citation2018; Hancock, Stowers, and Kessler Citation2019).
Trust has been widely studied in various disciplines. It seems that there is a common core of trust in all disciplines comprising of positive expectations and vulnerability (Rousseau et al. Citation1998). Lee and See (Citation2004) summarised the core of trust as ‘the attitude that an agent will help achieve an individual’s goals in a situation characterized by uncertainty and vulnerability’. In information systems (IS) research on trust, the Integrative Trust Model of Mayer, Davis, and Schoorman (Citation1995) is widely accepted. It was originally developed to describe interpersonal trust and therefore uses three dimensions of the trustee: ability, benevolence, and integrity. While previous authors have argued that these dimensions do not hold true for automated technology or software applications (Lee and See Citation2004; Söllner, Pavlou, and Leimeister Citation2013), recent authors claim that they are quite appropriate for autonomous technology (de Visser, Pak, and Shaw Citation2018). The reason for this shift is that autonomous, proactive, opaque, or anthropomorphic systems, such as robots or smart algorithms, often create the impression of a counterpart to be in dialog with rather than of a tool-like extension of one’s own body or mind, which in turn implies social interactions (Hassenzahl, et al. Citation2020). In other words, while ‘control’ is an attribute of a successful relationship to a tool, ‘trust’ is an attribute of a successful relationship to all those technologies experienced as a counterpart. More specifically, this success lies in the appropriate calibration of trust (de Visser, Pak, and Shaw Citation2018), that is, to avoid overtrust, which leads to misuse, as well as undertrust, which leads to disuse (Parasuraman and Riley Citation1997; Lee and See Citation2004; Drnec et al. Citation2016). While some variables/antecedents support the development of trust, other variables/antecedents diminish trust. Previous research on trust in non-human trustees revealed sets of various antecedents for different disciplines and non-human trustees, e. g. robotics (Hancock et al. Citation2011; Hancock et al. Citation2020), automation except for robotics (Schaefer et al. Citation2016), and software applications (Söllner, Pavlou, and Leimeister Citation2013). This research shows that the antecedents can be assigned to three groups: the trustor, the trustee, and the context/environment. Hoff and Bashir (Citation2013, 2015) matched these three groups with the three layers of trust identified by Marsh and Dibben (Citation2005): antecedents of the category trustor affect the dispositional trust; the antecedents of the non-human counterpart (trustee) affect the learned trust; and those of the environment affect the situational trust. First theoretical considerations assume that these groups and some of the antecedents hold true for AI as trustee (Siau and Wang Citation2018). However, to verify existing models and theories on trust in light of new technologies or new disciplines new research is needed (Detweiler and Broekens Citation2009; Farooq and Grudin Citation2016). Thus, the following section describes an approach to empirically examine trust in the new technology, AI, and within the new field of production management.
Research question and design
The research field addressed in this article is at the intersection of production management, artificial intelligence and trust. Consequently, all three facets are represented in the research question: ‘What does human trust in decisions of artificial intelligence depend on in the context of production management?’ The goal is to answer the research question with an explanatory model. First, it is necessary to understand the specifics of production management since a set of antecedents of trust for this field does not yet exist as it does in the described fields of robotics, automation, and software applications. For this reason, the existing sets of antecedent variables will be qualitatively mirrored and refined for AI and production management in the first phase. The second phase will quantitatively evaluate the resulting variables. For this purpose, a mixed-methods research strategy is used (Barton and Lazarsfeld Citation1984). It employs a qualitative approach as an auxiliary method for hypothesis generation with a subsequent quantitative approach for hypothesis testing. This design is characterised as follows: the results of the qualitative method are directly used in the quantitative method, so the overall analysis is not completely predefined. Due to the expected contribution to answer the research question, the second (quantitative) method is dominant over the first (qualitative) method. In summary, the two phases of this research design can be described as literature guided exploration (first phase) and statistical confirmation (second phase).
When carrying out the two phases and the discussion, two major topics of trust research are explicitly not dealt with, in order to reduce the complexity of the analysis. First, the distinction between different types of trust is excluded, e. g. contractual and relational in Rousseau et al. (Citation1998). Second, the discussion of whether or not trust and distrust are independent constructs, e. g. Lewicki, McAllister, and Bies (Citation1998), is not dealt with here.
Qualitative study for hypothesis development
In this first phase, we conducted a qualitative study to reveal potential antecedent variables influencing trust in the field of production management. We then structured and mirrored these identified antecedents by the existing antecedent sets of other fields and finally derive hypotheses.
Data collection method
The data was gathered in expert interviews (Meuser and Nagel Citation2009) and analysed with qualitative content analysis (Mayring Citation2014). The expert interviews aimed to systematically gather potential antecedents influencing trust in AI in production management. The interviews were intended to determine specific domain knowledge of production management and were therefore conducted as individual verbal interviews. To provide orientation and flexibility, the semi-structured interview form was used (Flick Citation2018). The guiding questions shown in were transferred into an interview guideline, which led to interviews of 60 min on average. To ensure the objectivity of qualitative research, subjectivity must be limited by methodical control and reflection. For this purpose, a pre-test with two participants was carried out, which led to an improvement of the guideline.
Table 1. Guiding questions for the expert interviews.
The expert interviews were conducted with four experts. They were carefully selected from the author’s professional and personal network. For us, it was important to select people who, in our eyes, are mentally able to imagine a future workplace with AI colleagues in production management. The first two interviewees are heads of production in the mechanical and plant engineering companies. One interviewee had 14 years, while the other had 20 years of experience in production management of companies with approximately 350 employees and high manufacturing depth. In recent years, both have implemented systems to automate previously cognitive processes within their companies, such as advanced planning and scheduling systems, and both are engaged in further automation based on AI. The last two interviews were conducted with employees of research institutions with a large consulting share in the field of production management. Both are managing groups of above 20 production researchers and have up to 25 years consulting experience. Both are working on questions of future AI applications for planning tasks in manufacturing companies. The combination of business- and science-oriented perspectives serves to generate a broad picture of potential antecedent variables of trust in AI in production management. The following analysis will show that multiple aspects were mentioned by all interviewees and that the hypotheses were well developed. Nevertheless, the small number of interviews needs to be mentioned as a limitation of this work.
Analysis approach
The interviews were analysed with qualitative content analysis according to Mayring and Fenzl (Citation2014). In light of the objective of this first phase, the technique of summarising qualitative content analysis was employed (Mayring and Fenzl Citation2014). This technique consists of four sequential steps: paraphrasing of the most important text passages; generalisation by adjusting the abstraction levels of all paraphrases; first reduction of unimportant or double paraphrases; second reduction by bundling the remaining paraphrases (Mayring and Fenzl Citation2014).
During the inductive formation of the categories/codes, the transcripts were repeatedly read and the developed categories were iteratively adjusted. For this purpose, two researchers independently used the web application QCAmap from Mayring and Fenzl (Citation2014). After that, they compared, discussed, and aligned their codes. The codes were then aggregated, renamed, and structured to a system of categories employing the theoretical models mentioned above, e. g. the Integrative Trust Model (Mayer, Davis, and Schoorman Citation1995). While some of the categories serve for the later formulation of human-AI decision scenarios (phase 2), six of them are identified as potential antecedent variables of trust in AI (see ). These factors have the potential to influence the trust in and subsequently the reliance on the system. Finally, it is expected to increase the performance of the human-AI team (Dzindolet et al. Citation2003; Lee and See Citation2004; Hoff and Bashir Citation2015; French, Duenser, and Heathcote Citation2018).
Figure 1. First potential antecedents for trust in AI within production management.
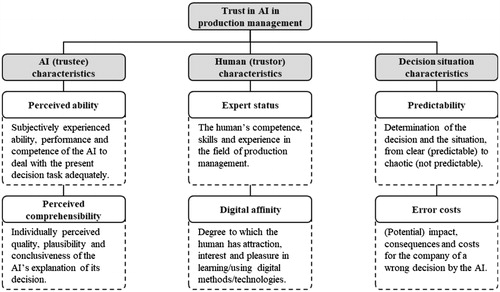
Hypotheses development
Similar to the trust model for interaction with an intelligent, autonomous robot of Matthews et al. (Citation2020), we assumed that not all of our six antecedents directly influence the trust, but get mediated through other antecedents. Thus, we employed the (probably) most generic expression of a mediation model (Baron and Kenny Citation1986), the SOR model of Woodworth (Citation1926), to structure our antecedents. summarises this structure and shows that trust is the response in the SOR model. The assignment of the independent variables and their presumed relationship to trust is described hereafter.
Figure 2. Model of hypotheses under investigation.
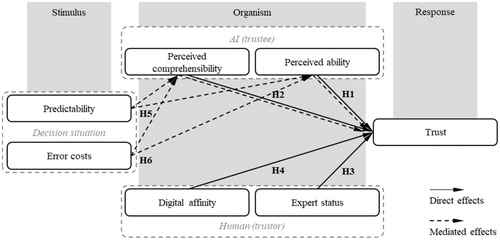
The two antecedents of the category AI (trustee) do not describe the objective but attribute characteristics of the AI. For example, not the measurable accuracy of a prediction was assumed to foster trust, but instead the subjective perception of AI’s ability. The same applied for perceived comprehensibility of the AI’s decision. For both antecedents, the individual and subjective perception of a given explanation was examined. Thus, we assigned these two antecedents to the organism in the SOR model and hypothesised the following direct relationships to trust.
H1. Perceived ability of AI in production management increases trust.
H2. Perceived comprehensibility of AI in production management increases trust.
The assignment of the category human (trustor) was trivial since it represents almost exactly the organism of the SOR model. The antecedent expert status describes the person’s experience in production management and skills to solve tasks in this field. The digital affinity characterises the degree to which a person has natural attraction, interest, and pleasure in learning and using (the latest) digital methods and technologies. Digital affinity should not be interpreted as familiarity. While affinity reflects a personal trait, familiarity describes acquired knowledge through experience. In line with the interviewed experts, we assumed that both – expert status and digital affinity – are directly related to trust, but that more experienced and skilled persons in production management trust less because they themselves are the experts and therefore are more sceptical.
H3. The expert status of humans decreases his/her trust in AI.
H4. The digital affinity of humans increases his/her trust in AI.
The antecedents of the category decision situation are the predictability (or inverted uncertainty) and the costs of an error (or risk) of the decision situation. We assigned both to the stimulus in the SOR model because they are determined by external characteristics. Based on the expert interviews, we assumed that these antecedents directly affect the perception of AI (trustee) characteristics and thus are mediated through them to indirectly affect the trust. We expected that trust is higher in riskier and more uncertain decision situations because managers themselves fear their inability and making poor decisions under these conditions (Riabacke Citation2006).
H5. The predictability of the situation in production management indirectly decreases trust in AI, mediated through perceived ability and/or perceived comprehensibility.
H6. The error costs of the situation in production management indirectly increase trust in AI, mediated through perceived ability and/or perceived comprehensibility.
Quantitative study for hypothesis testing
In this second research phase, a quantitative study was used to statistically test the significance of the hypotheses. Guided by a linear approach, the discovered antecedents were examined.
Data collection method
The quantitative study aimed to test the developed hypotheses. As a method for data collection, an online survey was conducted to acquire a large number of heterogeneous participants. Since the potential antecedents represent latent variables and are therefore not directly measurable, it was necessary to operationalise them with a measurement system and thus transfer them to a questionnaire (Kumar Citation2014). The measurement system is described in (and the formulation of the items is appended in Appendix A). In line with prior research (Mcknight et al. Citation2011; Lankton, McKnight, and Tripp Citation2015; Gulati, Sousa, and Lamas Citation2018) we modelled trust as a reflective construct. Moreover, the other antecedents were also modelled as reflective constructs, according to the decision rules of Jarvis, Mackenzie, and Podsakoff (Citation2003). To assure reliability and validity we used established items, modified established items, or followed the suggestions of Morgado et al. (Citation2017) for the development of new items.
Table 2. Measurements to operationalise the variables.
While all items were surveyed via a self-assessment of the participants, the variables predictability and error costs were operationalised by four scenarios (decision situations), that were derived from the expert interviews of phase one. Each scenario determined a specific value for the two variables, e. g. low, average, or high. The scenarios described an AI assistant recommending a specific decision in a defined situation of production management. Scenario 1 (layout optimisation) dealt with the repositioning of machines in the layout of a production. This was a decision of average predictability and average error costs. In scenario 2 (order acceptance), the AI assistant advised rejecting an order of 800,000 € because no capacities were available, which corresponded with high predictability and high error costs. Scenario 3 (job scheduling) described an AI assistant that scheduled the job sequence in the production shop. This task had high predictability and low error costs. In scenario 4 (production expansion) the AI assistant recommended expanding the production plant for 800,000 € to increase technological flexibility. Here, low predictability and high error costs were present. In the questionnaire, each of the four AI assistants was given a name to make it easier to distinguish between the scenarios (see Appendix B for the full descriptions of the scenarios).
In total, the questionnaire comprised 20 items, partially reverse-coded. Within the questionnaire, the items were divided into different blocks. Hereby, the items of one block were homogenous and the order of the items in one block supported an easy cognitive processing: the items build up logically on each other, and simple and heavy items alternate (Kumar Citation2014). The questionnaire designed in this way was subjected to a pre-test with three researchers, who have experience in production management. Besides, the pre-test also provided information on the duration it takes to answer it: approx. twelve minutes. The target group of the survey was current and former employees of manufacturing companies, previous experience with AI was not required. Potential participants were asked via personal messages and posts on different social networks to participate in this study. The questionnaire was published online from 08/01/2018 to 08/14/2018. At the end of the survey period, 182 respondents participated in the survey (gross sample). Of those 52 respondents did not complete the survey, so the retention rate was 71.4% and the net sample consisted of 130 respondents (43 females and 87 males), with a mean age of 32 years (SD = 10). Regarding the age distribution, accumulation was observed in the age range from 25 to 30 years (41.6%), while 21.5% of the participants were below and 36.9% above this age range. 67% of the participants had a university degree (bachelor, master, diploma, or PhD), while 33% were without an academic degree (skilled worker or technician). Also, 67% of the participants had professional experience in a manufacturing company, while 33% did not have this experience. The values of the net sample are publicly available on Mendeley Data, see Saßmannshausen (Citation2020).
Analysis approach
As the next step, the data had to be prepared. This included the coding of verbal response options to numerical values. The following analysis was performed with PLS using the software SmartPLS version 3.3.2 (Ringle, Wende, and Becker Citation2015). The rationale for choosing PLS as a method for structural equation modelling (SEM) was threefold. First, according to Hair et al. (Citation2016) as well as Jannoo et al. (Citation2014) PLS is superior to covariance-based SEM methods (CBSEM), when the goal lies in explorative theory development and predictive relevance of antecedent variables – as it is the case here. Second, the choice of the proper SEM method depended on the underlying nature of the data. Sarstedt et al. (Citation2016) developed a framework based on previous and own studies. For common factor data and reflective constructs, the CBSEM method is better suited, but PLS also provides valid results. In contrast, PLS is perfectly suited when the data follow a composite nature, while CBSEM should not be used here (Sarstedt et al. Citation2016). Thus, the use of PLS is preferred cases, when data nature is unclear or unknown because it can handle both. As an indicator of the data’s nature, the standardised root mean square residual (SRMR) is proposed by Sarstedt et al. (Citation2016). As a threshold, they reference to Hu and Bentler (Citation1998) and recommend that values of 0.08 or smaller indicate composite data. The data of the present study had an SRMR of 0.08, which indicated the use of PLS. The third reason for choosing PLS lied in its advantages to handle unusual data characteristics (e. g. non-normal distributions) and complex models (six or more antecedents) – as it is mostly the case in social science (Hair et al. Citation2014, Sarstedt, Ringle, and Hair Citation2017). Therefore, we investigated the data distribution using Shapiro-Wilk’s test (p < 2.2e−16, should be > 5% for normal distribution) and optical inspection of the density plot as well as QQ plot. The results showed that the data was not normally distributed. Consequently, PLS was preferred over CBSEM for the analysis.
The PLS assessment followed the two-stage approach of Sarstedt, Ringle, and Hair (Citation2017). The first stage was the evaluation of the measurement model (Sarstedt, Ringle, and Hair Citation2017). This was done in four steps by examining item loadings, internal consistency, convergent validity, and discriminant validity. In step one, the item loadings should at least be 0.7 (Hair et al. Citation2016; Sarstedt, Ringle, and Hair Citation2017). Consequently, we dropped three items, that did not fulfil this criterion (items Trust_3, Digi_3 and Digi_4). For the internal consistency (IC) in step two, we reported three coefficients. Two of them are the most common coefficients according to Cho (Citation2016): the congeneric reliability (also composite reliability and upper bound of IC) and the tau-equivalent reliability (also Cronbach's Alpha and lower bound of IC). As third coefficient for IC we employed the new reliability measure introduced by Dijkstra and Henseler (Citation2015): Roh A. As shown above our data was of composite nature, which caused the tau-equivalent reliability to underestimate the IC (Dijkstra and Henseler Citation2015), which was even reinforced by our scales with few items (Cortina Citation1993). Furthermore, we could assume that the congeneric reliability overestimated the IC because the item loadings in PLS tend to be upward-biased (Dijkstra and Henseler Citation2015). Consequently, we focussed on Roh A when evaluating the coefficients, but also considered the other two coefficients slightly, see . Values above 0.7 indicate good IC, while values between 0.6 and 0.7 are acceptable for exploratory research (Nunnally Citation1967; Hair et al. Citation2016). For Roh A all variables passed this threshold. Since the tau-equivalent reliability underestimated the IC it was not surprising that some variables would not have passed this threshold if regarded without differentiation. Given that Roh A exceeded the threshold there is no need for action such as dropping further items. In step three we evaluated the convergent validity by calculating the average variance extracted (AVE). The AVE should be 0.5 or higher (Hair et al. Citation2016), as it was for all variables under investigation (see ).
Table 3. Evaluation of measurement model.
The fourth step assessed the discriminant validity to ensure that the variables were unique and distinct from the other variables (Sarstedt, Ringle, and Hair Citation2017). Here the Fornell-Larcker criterion and the cross-loadings are the most frequently used approaches (Henseler, Ringle, and Sarstedt Citation2015). shows the assessment of the Fornell-Larcker criterion, where the bolt values on the diagonal should be greater than the values in the corresponding row and column (Hair et al. Citation2016) – which is fulfilled here. In addition, shows the cross-loading of the items. Here, the loading of each item to the assigned variable should be higher than the loading to any other variable (Gulati, Sousa, and Lamas Citation2018) – which is also fulfilled here. Thus, our instrument meets both criteria. According to Henseler, Ringle, and Sarstedt (Citation2015) we also assessed the heterotrait-monotrait ratio (HTMT) to prove the discriminant validity. The HTMT should be smaller than 0.9 for conceptual similar variables/constructs and smaller than 0.85 for distinct variables/constructs (Henseler, Ringle, and Sarstedt Citation2015). As shown in all HTMT ratios fulfil this requirement. Summing up the four steps for evaluation of the measurement model, our instrument met all criteria, after dropping three items, and had good internal consistency/reliability, convergent, and discriminant validity.
Table 4. Evaluation of Fornell-Larcker criterion.
Table 5. Evaluation of cross-loadings.
Table 6. Evaluation of heterotrait-monotrait ratio (HTMT).
The second stage of the PLS assessment was the evaluation of the structural model, which consists of five steps. For these steps, we used bootstrapping with 5,000 sub-samples as a non-parametric approach, especially for the evaluation of the mediation effects. In the first step, we assessed the multi-collinearity of the antecedents by use of the variance inflation factor (VIF). Depending on the literature the VIF should be smaller than ten (Hair et al. Citation2014) or even smaller than five (Sarstedt, Ringle, and Hair Citation2017). shows that the variables met this requirement. The second step evaluated the path coefficients (effects of the antecedents) and their significance. As shown in the direct effects of perceived ability, perceived comprehensibility and digital affinity were all significant. Here, the perceived ability had the strongest effect with 0.48, which means that the increase of one of perceived ability by one standard deviation is accompanied by the increase of trust by 0.48 standard deviations, whereas all other antecedents remain constant. In the case of the antecedents predictability and error costs, there were significant indirect effects. Since the direct effects were not significant, this is a full mediation, according to Nitzl, Roldan, and Cepeda (Citation2016). These mediations were further analysed, as the indirect effects were formed via two moderators (perceived ability and perceived comprehensibility). The indirect/moderating effect of predictability in is the combination of the two sub-effects: the indirect effect via perceived ability (−0.095, p < 0.001) and the indirect effect via perceived comprehensibility (−0.042, p < 0.01). In contrast, only the sub-effect of error costs via the mediator perceived ability was significant (0.073, p < 0.01), while the sub- effect via perceived comprehensibility was not significant (0.012, p > 0.05).
Table 7. Evaluation of structural model with trust as independent variable.
In the next, third, step we assessed the individual contribution of the antecedents. Thus, we reported the f2 (effect size), see . In line with the analysis above, the three significant direct paths showed effect sizes different from zero, which can be labelled as small to medium (Cohen Citation1988).
Following Hair et al. (Citation2016), in the next steps, we analysed the overall suitability of the model. Thus, we calculated the coefficient of determination in step four. With an R2 of 0.843 (adjusted R2 = 0.377), we nearly explained 40% of the variance of trust, which can be interpreted as weak to moderate (Henseler, Ringle, and Sinkovics Citation2009; Sarstedt, Ringle, and Hair Citation2017) or even as large effect size (Cohen Citation1988; Kotrlik and Williams Citation2003). To support this R2 we calculated the statistical power, which should be higher than 0.8 for α = 0.05 (Nitzl, Roldan, and Cepeda Citation2016). Using G*Power version 3.1.9.6 our statistical power was 1.000 (with α = 0.05, n = 130 and six predictors), which is substantial. As the last and fifth step of the evaluation of the structural model we reported the predictive accuracy of the model, Q2. The Q2 was calculated using a blindfolding approach with a recommended omission distance of seven (Hair et al. Citation2017). Our Q2 of the variable trust was 0.249. This value fulfilled the requirement to be above zero (Sarstedt, Ringle, and Hair Citation2017), so the model’s prediction of trust is acceptable and relevant. After this presentation of the analysis results, the following section outlines what these results mean regarding the developed hypotheses.
Hypotheses summary
We developed six hypotheses based on the literature, enriched by qualitative information from the conducted expert interviews. The significance of these hypotheses can be derived from the analysis results presented above. For a better overview, we have summarised the relevant findings for the hypotheses in .
Figure 3. Coefficients of the structural model.
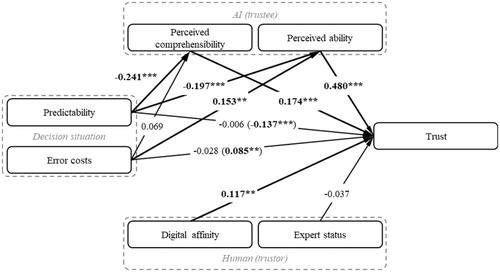
Both attributed characteristics of AI (trustee) – perceived ability and perceived comprehensibility – have a significant positive effect on trust. Thus, their respective hypotheses H1 and H2 hold true for this study. For both assumed relationships of the characteristic of the human (trustor) and trust, only one is statistically significant. While the expected negative effect of expert status on trust seems to be correct, this effect is not significant and therefore H3 is not confirmed. In contrast, H4 is significant, which means that a higher digital affinity leads to higher trust.
The two assumed indirect effects of the decision situation, namely H5 and H6, hold true in the present study. Since the corresponding direct effects on trust are not significant, full mediation is present. For the mediation the two mediators perceived ability and perceived comprehensibility were assumed. Looking at the partial mediations through only one of these mediators, we see that the antecedent predictability has a significant indirect effect on trust through both mediators. Thus, the sub-hypotheses H5a and H5b hold true. In contrast, for the antecedent error costs only the mediation through perceived ability is significant (H6a), but the one through perceived comprehensibility is not (H6b). The results of the hypothesis testing are summarised in .
Table 8. Results of hypothesis testing.
Discussion
The test of the hypotheses and the structural model show that the antecedents predictability and error costs have no significant direct effect on trust, which is in line with a meta-analysis of factors influencing trust in automation (Schaefer et al. Citation2016). However, our study supports the expectation of Lee and See (Citation2004) that there should be some effect. Instead, these antecedents contribute to the explanation of trust with their fully mediated indirect effects. This shows that the employment of the SOR model as a structural framework for trust antecedents is quite useful. In light of Hoff and Bashir (Citation2015)’s trust model, our findings indicate that the two identified antecedents of the group environment do not affect the situational trust as implied by the model, but indirectly affect the learned trust. Since the mediations through perceived ability are stronger than through perceived comprehensibility it seems that trust depends on the human’s understanding of how the situation affects the AI’s capabilities (Lee and See Citation2004).
Considering the category AI (trustee) characteristics, both antecedents contribute to the explanation of trust. In IS research, the most mentioned antecedents of this category are ability, benevolence, and integrity (Mayer, Davis, and Schoorman Citation1995) from human-human trust. Also, their further developments gain more attention, like performance, process, and purpose (Lee and See Citation2004) or performance, helpfulness, and predictability (Söllner, Pavlou, and Leimeister Citation2013). So, ability is known as a traditional and strong influencing factor (Hancock et al. Citation2011; Schaefer et al. Citation2016). In this study, the perceived ability has the strongest effect of all analysed antecedents (β = 0.48, p < 0.001). This effect indicates that an increase of one standard deviation in perceived ability goes along with a rise in trust of 0.48 standard deviations – assuming all other antecedents are held constant. Previous studies also underline that the use of perceived ability instead of objective/true ability was a suitable choice. Thus, our first recommendation for AI designers to foster trust is to make the AI appear capable. For instance, a certain ability is perceived depending on the features of the IT system’s interface, even if these features are not related to the true abilities (Tseng and Fogg Citation1999). At the beginning of an AI implementation, it is also conceivable to verify the decisions of the AI by a second (e. g. rule-based) system or a human being in order to avoid errors and error messages and thus to increase the perceived ability. This serves as a great adjustment mechanism for trust calibration.
The variable perceived comprehensibility is a novel trust antecedent that has become increasingly important since humans interact with intelligent and autonomous systems (Matthews et al. Citation2020) – as the efforts of governments and companies to explainable AI underline (Cutler, Pribić, and Humphrey Citation2019; Fjeld and Nagy Citation2020). The effect on trust is not as strong as the one of perceived ability: when perceived comprehensibility increases by one standard deviation, trust rises 0.174 standard deviations – assuming all other antecedents stay constant. This leads to the second design recommendation: subjectively better explanations or more expectable decision-making behaviour of the AI lead to higher trust. Consequently, there is a need for explainable AI (XAI). Waltl and Vogl (Citation2018) provide a taxonomy of different learning systems and their natural contribution to explainability. As neural networks are not transparent by nature there are some efforts to support their explainability (see e. g. Ribeiro, Singh, and Guestrin (Citation2016) or Schaaf, Huber, and Maucher (Citation2019)). Moreover, trust can be further increased using a visually represented agent communicate the explanation rather than just displaying it (Weitz et al. Citation2019). In the category of human (trustor) characteristics the expert status of the human in the field of production management seems not to contribute to the explanation of trust. In other IS research similar constructs like ability or competence of the human affect trust (Schaefer et al. Citation2016; Siau and Wang Citation2018). Although our study does not support it, more experienced production managers could tend to trust less. This relationship then could be explained by a study of Kantowitz, Hanowski, and Kantowitz (Citation1997) on vehicle navigation aid. The more familiar a person was with a city, the more negative navigation errors were judged, in terms of trust and reliability. Therefore, an experienced production manager may be more critical regarding the decisions of an AI assistant (risk of undertrust) than an inexperienced production manager, who might even tend to overtrust.
Digital affinity is a new trust antecedent that has not yet been considered in IS trust research on autonomous systems. An analogous antecedent, however, was found in research on flight automation: the general accustoming of pilots to the use of automated solutions (Riley Citation1996). It seems that familiarity with technology leads to higher trust, which is supported by the affinity to that technology. Here, digital affinity shows a strong and statistically significant effect. Our third recommendation is addressed to designers of human-AI systems. Since a trustful relationship leads also to a higher team performance, it is advisable to introduce the AI to digitally competent employees. In addition, this trait might become a criterion for staff recruiting.
The gained insights of trust in AI in production management can be used, for example, to introduce an AI assistant to employees and thus foster the trust in the AI. Here, the perceived ability seems to be a mandatory requirement. However, this model currently explains only about 40% of the trust variance. Thus, there is a need for further research to improve the model, partly because we built it on a small number of expert interviews. For example, further antecedents that were not considered in this study should be added, like the visual representation of the AI (Siau and Wang Citation2018) or the culture of an organisation (Lee and See Citation2004). Moreover, a new category of antecedents could be introduced next to the categories of the trustor (human), the trustee (AI), and the decision situation: the category of interaction. Employing the trust model of Hoff and Bashir (Citation2015) the antecedents of this new category could affect the dynamic learned trust – a result of dividing the learned trust into initial learned trust and dynamic learned trust. Here, adaptive or adaptable shifts between human and AI decision making/control could be introduced, depending on the human’s trust and capabilities in the situation (Lee and See Citation2004). All this will lead to a more accurate model and the verification of the presented results. As an extension to the refinement of the antecedent side of trust, we should also take a look at the consequence side. As seen in Hoff and Bashir (Citation2015)'s trust model, we could explore how trust affects reliability, performance, and satisfaction.
It should be noted that this study was an imagination study, so the participants did not face any consequences. This could have led to a distortion of the response behaviour, following the risk compensation theory (Hedlund Citation2000). Therefore, further research should address a corresponding experimental and experienceable design within production management. In such experimental settings, the linearity of the relationships assumed here could also be questioned, since there are indications that, for example, the relationship between ability and trust is not linear (Muir and Moray Citation1996; Kramer Citation1999). Additionally, the stability/robustness of trust in different settings can be examined to gain deeper insights, as indicated by McKnight, Cummings, and Chervany (Citation1998).
Conclusion
The objective of this study was to discover and evaluate antecedent variables of trust in AI for production management. Here, the antecedent of digital affinity stands out, as it has not yet been included in any set of antecedents for trust between humans and AI. Other examined antecedents are similar to those in the literature on robots and automation, with the difference that we have studied the perceived (not the objective) characteristics of AI. Since this perception happens inside humans, we employed the SOR model to structure the antecedents. Summing up, it was possible to contribute to answer the guiding question: ‘What does human trust in decisions of artificial intelligence depend on in the context of production management?’ The results serve as first design guidelines for a socially sustainable human-AI interaction in production management. Autonomous and independent decision-making AI assistants will offer a sensible and possibly necessary addition to human managers in the future. Because a broad explanation of trust was not achieved, the inclusion of further antecedents should be the topic of further research to ensure the human-centered design of future production management systems.
Disclosure statement
No potential conflict of interest was reported by the author(s).
References
- Baron, R. M., and D. A. Kenny. 1986. “The Moderator–Mediator Variable Distinction in Social Psychological Research: Conceptual, Strategic, and Statistical Considerations.” Journal of Personality and Social Psychology 51 (6): 1173–1182. doi:https://doi.org/10.1037/0022-3514.51.6.1173.
- Barton, A. H., and P. F. Lazarsfeld. 1984. “Einige Funktionen von qualitativer Analyse in der Sozialforschung.”. In Qualitative Sozialforschung, edited by C. Hopf and E. Weingarten, 41–89. Stuttgart: Klett-Cotta.
- Beierlein, C., C. J. Kemper, A. Kovaleva, and B. Rammstedt. 2014. Interpersonales Vertrauen (KUSIV3): Zusammenstellung sozialwissenschaftlicher Items und Skalen. Leibnitz, Germany: GESIS Leibniz Institute for the Social Sciences.
- Benner, P. E. 2017. Stufen zur Pflegekompetenz: From novice to expert. 3rd ed. Bern: Hogrefe.
- Burggräf, Peter, Johannes Wagner, Matthias Dannapfel, Tobias Adlon, Till Saßmannshausen, Carsten Fölling, Nils Föhlisch, Dennis Ohrndorf, and Richard Minderjahn. 2020. “Adaptive Remanufacturing for Lifecycle Optimization of Connected Production Resources: A Literature Review.” Procedia CIRP 90: 61–66. doi:https://doi.org/10.1016/j.procir.2020.01.054.
- Burggräf, Peter, Johannes Wagner, Benjamin Koke, and Milan Bamberg. 2020. “Performance Assessment Methodology for AI-Supported Decision-Making in Production Management.” Procedia CIRP 93: 891–896. (accepted). doi:https://doi.org/10.1016/j.procir.2020.03.047.
- Burggräf, P., J. Wagner, and B. Koke. 2018. “Artificial Intelligence in Production Management: A Review of the Current State of Affairs and Research Trends in Academia.”. International Conference on Information Management and Processing (ICIMP), 82–88. London, UK: IEEE.
- Burggräf, P., J. Wagner, and T. Weißer. 2020. “Knowledge-Based Problem Solving in Physical Product Development: A Methodological Review.” Expert Systems with Applications: X 5: 100025–100014. doi:https://doi.org/10.1016/j.eswax.2020.100025.
- Cho, E. 2016. “Making Reliability Reliable.” Organizational Research Methods 19 (4): 651–682. doi:https://doi.org/10.1177/1094428116656239.
- Cohen, J. 1988. Statistical Power Analysis for the Behavioral Sciences. 2nd ed. Hillsdale, NJ: L. Erlbaum Associates.
- Cortina, J.M. 1993. “What is Coefficient Alpha? An Examination of Theory and Applications.” Journal of Applied Psychology 78 (1): 98–104. doi:https://doi.org/10.1037/0021-9010.78.1.98.
- Cutler, A., M. Pribić, and L. Humphrey. 2019. “Everyday Ethics for Artificial Intelligence: A practical Guide for Designers & Developers.” Accessed 6 June 2020. https://www.ibm.com/watson/assets/duo/pdf/everydayethics.pdf.
- de Visser, E. J., R. Pak, and T. H. Shaw. 2018. “From 'Automation' to 'Autonomy': The Importance of Trust Repair in Human-Machine Interaction.” Ergonomics 61 (10): 1409–1427. doi:https://doi.org/10.1080/00140139.2018.1457725.
- Dellermann, Dominik, Philipp Ebel, Matthias Söllner, and Jan Marco Leimeister. 2019. “Hybrid Intelligence.” Business & Information Systems Engineering 61 (5): 637–643. doi:https://doi.org/10.1007/s12599-019-00595-2.
- Detweiler, C., and J. Broekens. 2009. “Trust in Online Technology: Towards Practical Guidelines Based on Experimentally Verified Theory.” In Human-Computer Interaction. Ambient, Ubiquitous and Intelligent Interaction, edited by J.A. Jacko, 605–614. Berlin, Heidelberg: Springer Berlin Heidelberg.
- Dijkstra, T. K., and J. Henseler. 2015. “Consistent Partial Least Squares Path Modeling.” MIS Quarterly 39 (2): 297–316. doi:https://doi.org/10.25300/MISQ/2015/39.2.02.
- Drnec, Kim, Amar R. Marathe, Jamie R. Lukos, and Jason S. Metcalfe. 2016. “From Trust in Automation to Decision Neuroscience: Applying Cognitive Neuroscience Methods to Understand and Improve Interaction Decisions Involved in Human Automation Interaction.” Frontiers in Human Neuroscience 10: 290. doi:https://doi.org/10.3389/fnhum.2016.00290.
- Dzindolet, Mary T., Scott A. Peterson, Regina A. Pomranky, Linda G. Pierce, and Hall P. Beck. 2003. “The Role of Trust in Automation Reliance.” International Journal of Human-Computer Studies 58 (6): 697–718. doi:https://doi.org/10.1016/S1071-5819(03)00038-7.
- Farooq, U., and J. Grudin. 2016. “Human-Computer Integration.” interactions 23 (6): 26–32. doi:https://doi.org/10.1145/3001896.
- Fischer, K., H. M. Weigelin, and L. Bodenhagen. 2018. “Increasing Trust in Human–Robot Medical Interactions: Effects of Transparency and Adaptability.” Paladyn, Journal of Behavioral Robotics 9 (1): 95–109. doi:https://doi.org/10.1515/pjbr-2018-0007.
- Fjeld, J., and A. Nagy. 2020. “Principled artificial intelligence: mapping consensus in ethical and rights-based approaches to principles for AI.” Berkman Klein Center at Harvard University. Accessed 6 June 2020. https://cyber.harvard.edu/publication/2020/principled-ai.
- Flick, U. 2018. An Introduction to Qualitative Research. 6th ed. Thousand Oaks, CA: SAGE Publications.
- French, B., A. Duenser, and A. Heathcote, 2018. “Trust in Automation – A Literature Review.” CSIRO Report, EP184082. http://www.tascl.org/uploads/4/9/3/3/49339445/trust_in_automation_-_a_literature_review_report.pdf.
- Gabel, T., and M. Riedmiller. 2008. “Adaptive Reactive Job-Shop Scheduling with Reinforcement Learning Agents.” International Journal of Information Technology and Intelligent Computing 24 (4): 14–18.
- Gulati, S., S. Sousa, and D. Lamas. 2018. “Modelling Trust in Human-Like Technologies.” Paper presented at Proceedings of the 9th International Conference on HCI IndiaHCI 2018 - IndiaHCI 18, New York, New York, USA: ACM Press, 1–10.
- Hair, J. F., W. C. Black, B. J. Babin, and R. E. Anderson. 2014. Multivariate Data Analysis. 7th ed. Harlow, Essex: Pearson.
- Hair, J. F., M. Sarstedt, L. Hopkins, and V.G. Kuppelwieser. 2014. “Partial Least Squares Structural Equation Modeling (PLS-SEM).” European Business Review 26 (2): 106–121.
- Hair, J. F., G. T. M. Hult, C. Ringle, and M. Sarstedt. 2016. A Primer on Partial Least Squares Structural Equation Modeling (PLS-SEM). 2nd ed. Los Angeles: SAGE.
- Hair, Joe, Carole L. Hollingsworth, Adriane B. Randolph, and Alain Yee Loong Chong. 2017. “An Updated and Expanded Assessment of PLS-SEM in Information Systems Research.” Industrial Management & Data Systems 117 (3): 442–458. doi:https://doi.org/10.1108/IMDS-04-2016-0130.
- Hancock, Peter A., Deborah R. Billings, Kristin E. Schaefer, Jessie Y C. Chen, Ewart J. de Visser, and Raja Parasuraman. 2011. “A Meta-Analysis of Factors Affecting Trust in Human-Robot Interaction.” Human Factors 53 (5): 517–527. doi:https://doi.org/10.1177/0018720811417254.
- Hancock, P. A., T. T. Kessler, A. D. Kaplan, J. C. Brill, and J. L. Szalma. 2020. “Evolving Trust in Robots: Specification Through Sequential and Comparative Meta-Analyses.” Human Factors. doi:https://doi.org/10.1177%2F0018720820922080.
- Hancock, P. A., K. L. Stowers, and T. T. Kessler. 2019. “Can We Trust Autonomous Systems?” In Neuroergonomics: The Brain at Work and in Everyday Life, edited by H. Ayaz and F. Dehais, 199. London: Academic Press.
- Haslam, N. 2006. “Dehumanization: An Integrative Review.” Personality and Social Psychology Review 10 (3): 252–264. doi:https://doi.org/10.1207/s15327957pspr1003_4.
- Hassenzahl, M., S. Boll, J. Borchers, A. Rosenthal-von der Pütten, and V. Wulf. 2020. “Otherware” Needs Alternative Interaction Paradigms beyond Naïve Anthropomorphism.” Paper presented at the Nordic Conference on Human-Computer Interaction (NordiCHI), Tallinn, Estonia.
- Hedlund, J. 2000. “Risky Business: safety Regulations, Risks Compensation, and Individual Behavior.” Injury Prevention 6 (2): 82–90. doi:https://doi.org/10.1136/ip.6.2.82.
- Henseler, J., C. M. Ringle, and M. Sarstedt. 2015. “A New Criterion for Assessing Discriminant Validity in Variance-Based Structural Equation Modeling.” Journal of the Academy of Marketing Science 43 (1): 115–135. doi:https://doi.org/10.1007/s11747-014-0403-8.
- Henseler, J., C. M. Ringle, and R. R. Sinkovics. 2009. “The Use of Partial Least Squares Path Modeling in International Marketing.” In New Challenges to International Marketing, edited by R. R. Sinkovics and P. N. Ghauri, 277–319. Bingley, UK: Emerald Group Publishing Limited.
- Hergeth, Sebastian, Lutz Lorenz, Roman Vilimek, and Josef F. Krems. 2016. “Keep Your Scanners Peeled: Gaze Behavior as a Measure of Automation Trust during Highly Automated Driving.” Human Factors 58 (3): 509–519. doi:https://doi.org/10.1177/0018720815625744.
- Hoff, K., and M. Bashir. 2013. “A Theoretical Model for Trust in Automated Systems.” In CHI 2013: Extended Abstracts of the 31st Annual CHI Conference on Human Factors in Computing Systems: 27 April - 2 May 2013, Paris, France, edited by W. Mackay, S. Brewster, and S. Bødker, 115. New York: ACM.
- Hoff, K. A., and M. Bashir. 2015. “Trust in Automation: integrating Empirical Evidence on Factors That Influence Trust.” Human Factors 57 (3): 407–434. doi:https://doi.org/10.1177/0018720814547570.
- Hu, L., and P. M. Bentler. 1998. “Fit Indices in Covariance Structure Modeling: Sensitivity to Underparameterized Model Misspecification.” Psychological Methods 3 (4): 424–453. doi:https://doi.org/10.1037/1082-989X.3.4.424.
- Hu, Wan-Lin, Kumar Akash, Tahira Reid, and Neera Jain. 2019. “Computational Modeling of the Dynamics of Human Trust during Human–Machine Interactions.” IEEE Transactions on Human-Machine Systems 49 (6): 485–497. doi:https://doi.org/10.1109/THMS.2018.2874188.
- Jaensch, F., A. Csiszar, A. Kienzlen, and A. Verl. 2018. Reinforcement Learning of Material Flow Control Logic Using Hardware-in-the-Loop Simulation. Paper presented at the 2018 First International Conference on Artificial Intelligence for Industries (AI4I) , 77–80. Laguna Hills: IEEE. doi:https://doi.org/10.1109/AI4I.2018.8665712.
- Jannoo, Z., B. W. Yap, N. Auchoybur, and M. A. Lazim. 2014. “The Effect of Nonnormality on CB-SEM and PLS-SEM Path Estimates.” International Scholarly and Scientific Research & Innovation 8 (2): 285–291.
- Jarvis, C. B., S. B. Mackenzie, and P.M. Podsakoff. 2003. “A Critical Review of Construct Indicators and Measurement Model Misspecification in Marketing and Consumer Research.” Journal of Consumer Research 30 (2): 199–218. doi:https://doi.org/10.1086/376806.
- Kantowitz, B. H., R. J. Hanowski, and S. C. Kantowitz. 1997. “Driver Acceptance of Unreliable Traffic Information in Familiar and Unfamiliar Settings.” Human Factors: The Journal of the Human Factors and Ergonomics Society 39 (2): 164–176. doi:https://doi.org/10.1518/001872097778543831.
- Karrer, K., C. Glaser, and C. Clemens. 2009. “Technikaffinität Erfassen - Der Fragebogen TA-EG.” In Der Mensch im Mittelpunkt Technischer Systeme: 8. Berliner Werkstatt Mensch-Maschine-Systeme, edited by A. Lichtenstein, C. Stößel, and C. Clemens, 196–201. Düsseldorf: VDI Verlag.
- Kotrlik, J. W., and H. A. Williams. 2003. “The Incorporation of Effect Size in Information Technology, Learning, and Performance Research.” Information Technology, Learning, and Performance Journal 21 (1): 1–7.
- Kramer, R. M. 1999. “Trust and Distrust in Organizations: Emerging Perspectives, Enduring Questions.” Annual Review of Psychology 50: 569–598. doi:https://doi.org/10.1146/annurev.psych.50.1.569.
- Kuhnle, A., M. Kuttler, M. Dümpelmann, and G. Lanza. 2017. “Intelligente Produktionsplanung Und -Steuerung: Erlernen Optimaler Entscheidungen.” wt Werkstattstechnik Online 107 (9): 625–629.
- Kuhnle, A., N. Röhrig, and G. Lanza. 2019. “Autonomous Order Dispatching in the Semiconductor Industry Using Reinforcement Learning.” Procedia CIRP 79: 391–396. doi:https://doi.org/10.1016/j.procir.2019.02.101.
- Kumar, R. 2014. Research Methodology: A Step-by-Step Guide for Beginners. 4th ed. Los Angeles, London, New Delhi, Singapore, Washington DC: SAGE Publications.
- Lankton, N., D. H. McKnight, and J. Tripp. 2015. “Technology, Humanness, and Trust: Rethinking Trust in Technology.” Journal of the Association for Information Systems 16 (10): 880–918. doi:https://doi.org/10.17705/1jais.00411.
- Lee, J. D., and K. A. See. 2004. “Trust in Automation: designing for Appropriate Reliance.” Human Factors 46 (1): 50–80. doi:https://doi.org/10.1518/hfes.46.1.50_30392.
- Lewicki, R. J., D. J. McAllister, and R. J. Bies. 1998. “Trust and Distrust: New Relationships and Realities.” The Academy of Management Review 23 (3): 438–458. doi:https://doi.org/10.2307/259288.
- Marsh, S., and M. R. Dibben. 2005. “The Role of Trust in Information Science and Technology.” Annual Review of Information Science and Technology 37 (1): 465–498. doi:https://doi.org/10.1002/aris.1440370111.
- Matthews, G., J. Lin, A. R. Panganiban, and M.D. Long. 2020. “Individual Differences in Trust in Autonomous Robots: Implications for Transparency.” IEEE Transactions on Human-Machine System 50 (3): 234–244. doi:https://doi.org/10.1109/THMS.2019.2947592.
- Mayer, R. C., J. H. Davis, and F. D. Schoorman. 1995. “An Integrative Model of Organizational Trust.” The Academy of Management Review 20 (3): 709–734. doi:https://doi.org/10.2307/258792.
- Mayring, P., and T. Fenzl. 2014. “Qualitative Inhaltsanalyse.”. In Handbuch Methoden Der Empirischen Sozialforschung, edited by N. Baur and J. Blasius, 543–556. Wiesbaden: Springer VS.
- Mayring, P. 2014. Qualitative Content Analysis: Theoretical Foundation, Basic Procedures and Software Solution. Klagenfurt: GESIS Leibniz Institute for the Social Sciences.
- McAfee, A., and E. Brynjolfsson. 2017. Machine, Platform, Crowd: Harnessing Our Digital Future. New York, London: W.W. Norton & Company.
- McKnight, D. H., L. L. Cummings, and N. L. Chervany. 1998. “Initial Trust Formation in New Organizational Relationships.” The Academy of Management Review 23 (3): 473–490. doi:https://doi.org/10.2307/259290.
- Mcknight, D. Harrison, Michelle Carter, Jason Bennett Thatcher, and Paul F. Clay. 2011. “Trust in a Specific Technology: An Investigation of Its Components and Measures.” ACM Transactions on Management Information Systems 2 (2): 1–25. doi:https://doi.org/10.1145/1985347.1985353.
- Merritt, Stephanie M., Deborah Lee, Jennifer L. Unnerstall, and Kelli Huber. 2015. “Are Well-Calibrated Users Effective Users? Associations between Calibration of Trust and Performance on an Automation-Aided Task.” Human Factors 57 (1): 34–47. doi:https://doi.org/10.1177/0018720814561675.
- Meuser, M., and U. Nagel. 2009. “The Expert Interview and Changes in Knowledge Production.” In Interviewing Experts, edited by A. Bogner, B. Littig, and W. Menz, 17–42. London: Palgrave Macmillan.
- Morgado, F. F. R., J. Meireles, C.M. Neves, and A.C.S. Amaral. 2017. “Scale Development: ten Main Limitations and Recommendations to Improve Future Research Practices.” Psicologia, Reflexao e Critica: revista Semestral Do Departamento de Psicologia da UFRGS 30 (1): 3.
- Muir, B. M., and N. Moray. 1996. “Trust in Automation. Part II. Experimental Studies of Trust and Human Intervention in a Process Control Simulation.” Ergonomics 39 (3): 429–460. doi:https://doi.org/10.1080/00140139608964474.
- Neyer, F. J., J. Felber, and C. Gebhardt. 2016. Kurzskala Zur Erfassung Von Technikbereitschaft (Technology Commitment). Zusammenstellung sozialwissenschaftlicher Items und Skalen. Leibniz, Germany: GESIS Leibniz Institute for the Social Sciences.
- Nitzl, C., J. L. Roldan, and G. Cepeda. 2016. “Mediation Analysis in Partial Least Squares Path Modeling.” Industrial Management & Data Systems 116 (9): 1849–1864. doi:https://doi.org/10.1108/IMDS-07-2015-0302.
- Norman, D. A., A. Ortony, and D. M. Russell. 2003. “Affect and Machine Design: Lessons for the Development of Autonomous Machines.” IBM Systems Journal 42 (1): 38–44. doi:https://doi.org/10.1147/sj.421.0038.
- Nunnally, J. C. 1967. Psychometric Theory. New York: McGraw-Hill.
- Parasuraman, R., and V. Riley. 1997. “Humans and Automation: Use, Misuse, Disuse, Abuse.” Human Factors: The Journal of the Human Factors and Ergonomics Society 39 (2): 230–253. doi:https://doi.org/10.1518/001872097778543886.
- Parasuraman, R., T. B. Sheridan, and C. D. Wickens. 2000. “A Model for Types and Levels of Human Interaction with Automation.” IEEE Transactions on Systems, Man, and Cybernetics. Part A, Systems and Humans : a Publication of the IEEE Systems, Man, and Cybernetics Society 30 (3): 286–297. doi:https://doi.org/10.1109/3468.844354.
- Qu, Shuhui, Jie Wang, Shivani Govil, and James O. Leckie. 2016. “Optimized Adaptive Scheduling of a Manufacturing Process System with Multi-Skill Workforce and Multiple Machine Types: An Ontology-Based, Multi-Agent Reinforcement Learning Approach.” Procedia CIRP 57: 55–60. doi:https://doi.org/10.1016/j.procir.2016.11.011.
- Riabacke, A. 2006. “Managerial Decision Making under Risk and Uncertainty.” IAENG International Journal of Computer Science, 32 (4): 1–7. Available from: http://www.iaeng.org/IJCS/issues_v32/issue_4/IJCS_32_4_12.pdf.
- Ribeiro, M. T., S. Singh, C. Guestrin. 2016. “Why Should I Trust You?” In Proceedings of the 22nd ACM SIGKDD International Conference on Knowledge Discovery and Data Mining, edited by B. Krishnapuram, 1135–1144. New York, NY: ACM.
- Riedl, M. O. 2019. “Human‐Centered Artificial Intelligence and Machine Learning.” Human Behavior and Emerging Technologies 1 (1): 33–36. doi:https://doi.org/10.1002/hbe2.117.
- Riley, V. 1996. “Operator Reliance on Automation: Theory and Data.” In Automation Theory and Applications, edited by R. Parasuraman and M. Mouloua, 19–35. Mahwah, NJ: Erlbaum.
- Ringle, C. M., S. Wende, and J.-M. Becker. 2015. SmartPLS 3. Boenningstedt: SmartPLS GmbH.
- Rossi, A., K. Dautenhahn, K. L. Koay, and J. Saunders. 2017. Investigating Human Perceptions of Trust in Robots for Safe HRI in Home Environments. In Proceedings of the Companion of the 2017 ACM/IEEE International Conference on Human-Robot Interaction - HRI '17, edited by B. Mutlu, M. Tscheligi, A. Weiss, and J.E. Young, 375–376. New York, NY: ACM Press. doi:https://doi.org/10.1145/3029798.3034822.
- Rousseau, Denise M., Sim B. Sitkin, Ronald S. Burt, and Colin Camerer. 1998. “Not so Different after All: A Cross-Discipline View of Trust.” Academy of Management Review 23 (3): 393–404. doi:https://doi.org/10.5465/amr.1998.926617.
- Rovira, E., R. Pak, and A. McLaughlin. 2017. “Effects of Individual Differences in Working Memory on Performance and Trust with Various Degrees of Automation.” Theoretical Issues in Ergonomics Science 18 (6): 573–591. doi:https://doi.org/10.1080/1463922X.2016.1252806.
- Russell, S. J., and P. Norvig. 2016. Artificial Intelligence: A Modern Approach. 3rd ed. London: Pearson Education.
- Sarstedt, Marko, Joseph F. Hair, Christian M. Ringle, Kai O. Thiele, and Siegfried P. Gudergan. 2016. “Estimation Issues with PLS and CBSEM: Where the Bias Lies!” Journal of Business Research 69 (10): 3998–4010. doi:https://doi.org/10.1016/j.jbusres.2016.06.007.
- Sarstedt, M., C. M. Ringle, and J. F. Hair. 2017. “Partial Least Squares Structural Equation Modeling.” In Handbook of Market Research, edited by C. Homburg, M. Klarmann, and A. Vomberg, 1–40. Cham: Springer.
- Saßmannshausen, T.M. 2020. Data from an online survey on initial trust in artificial intelligence for production management tasks [online]. Mendeley Data. Accessed 6 June 2020. doi:https://doi.org/10.17632/pg8hnttzkm.2.
- Schaaf, N., M. F. Huber, and J. Maucher. 2019. “Enhancing decision tree based interpretation of deep neural networks through L1-orthogonal regularization.” https://arxiv.org/pdf/1904.05394.
- Schaefer, Kristin E., Jessie Y C. Chen, James L. Szalma, and P A. Hancock. 2016. “A Meta-Analysis of Factors Influencing the Development of Trust in Automation: Implications for Understanding Autonomy in Future Systems.” Human Factors 58 (3): 377–400. doi:https://doi.org/10.1177/0018720816634228.
- Schyns, B., and G. V. Collani. 2014. Berufliche Selbstwirksamkeitserwartung: Zusammenstallung sozialwissenschaftlicher Items und Skalen. Leibniz, Germany: GESIS Leibniz Institute for the Social Sciences.
- Shneiderman, B. 2020. “Human-Centered Artificial Intelligence: Reliable, Safe & Trustworthy.” International Journal of Human–Computer Interaction 36 (6): 495–504. doi:https://doi.org/10.1080/10447318.2020.1741118.
- Siau, K., and W. Wang. 2018. “Building Trust in Artificial Intelligence, Machine Learning, and Robotics.” Cutter Business Technology Journal 31 (2): 47–53.
- Söllner, M., P. A. Pavlou, and J. M. Leimeister. 2013. “Understanding Trust in IT Artifacts: A New Conceptual Approach.” Accessed 6 June 2020. doi:https://doi.org/10.2139/ssrn.2475382.
- Stricker, Nicole, Andreas Kuhnle, Roland Sturm, and Simon Friess. 2018. “Reinforcement Learning for Adaptive Order Dispatching in the Semiconductor Industry.” CIRP Annals 67 (1): 511–514. doi:https://doi.org/10.1016/j.cirp.2018.04.041.
- Tseng, S., and B. J. Fogg. 1999. “Credibility and Computing Technology.” Communications of the ACM 42 (5): 39–44. doi:https://doi.org/10.1145/301353.301402.
- Waltl, B., and R. Vogl. 2018. “Explainable Artificial Intelligence: The New Frontier in Legal Informatics.” Jusletter IT 4: 1–10.
- Weitz, K., D. Schiller, R. Schlagowski, T. Huber, and E. André. 2019. “"Do you trust me?": Increasing User-Trust by Integrating Virtual Agents in Explainable AI Interaction Design.” In Proceedings of the 19th ACM International Conference on Intelligent Virtual Agents - IVA '19, edited by C. Pelachaud, J.-C. Martin, H. Buschmeier, G. Lucas, and S. Kopp, 7–9. New York, NY: ACM Press.
- Woodworth, R. S. 1926. “Dynamic Psychology.” In The Psychologies of 1925, edited by C. Murchison, 111–125. Worcester, MA: Clark University Press.
- World Economic Forum. 2015. Deep Shift: Technology Tipping Points and Societal Impact: Survey Report, September 2015. Geneva: World Economic Forum.
- Xu, A., and G. Dudek. 2015. “Towards Efficient Collaborations with Trust-Seeking Adaptive Robots.” In Proceedings of the Tenth Annual ACM/IEEE International Conference on Human-Robot Interaction Extended Abstracts - HRI'15 Extended Abstracts, edited by J.A. Adams, W. Smart, B. Mutlu, L. Takayama, 221–222. New York, NY: ACM Press.
Appendix A. Operationalisation of variables for online survey
Appendix B. Description of the decision scenarios for the survey