Abstract
A major question in human-automation interaction is whether tasks should be traded or shared between human and automation. This work presents reflections—which have evolved through classroom debates between the authors over the past 10 years—on these two forms of human-automation interaction, with a focus on the automated driving domain. As in the lectures, we start with a historically informed survey of six pitfalls of automation: (1) Loss of situation and mode awareness, (2) Deskilling, (3) Unbalanced mental workload, (4) Behavioural adaptation, (5) Misuse, and (6) Disuse. Next, one of the authors explains why he believes that haptic shared control may remedy the pitfalls. Next, another author rebuts these arguments, arguing that traded control is the most promising way to improve road safety. This article ends with a common ground, explaining that shared and traded control outperform each other at medium and low environmental complexity, respectively.
Practitioner summary: Designers of automation systems will have to consider whether humans and automation should perform tasks alternately or simultaneously. The present article provides an in-depth reflection on this dilemma, which may prove insightful and help guide design.
Abbreviations: ACC: Adaptive Cruise Control: A system that can automatically maintain a safe distance from the vehicle in front; AEB: Advanced Emergency Braking (also known as Autonomous Emergency Braking): A system that automatically brakes to a full stop in an emergency situation; AES: Automated Evasive Steering: A system that automatically steers the car back into safety in an emergency situation; ISA: Intelligent Speed Adaptation: A system that can limit engine power automatically so that the driving speed does not exceed a safe or allowed speed.
1. Introduction
Automation, defined as ‘the execution by a machine agent (usually a computer) of a function that was previously carried out by a human’ (Parasuraman and Riley Citation1997, 231), was initially used for relatively simple routines. Examples of successful implementation of such automation can be found in households (e.g. dishwashers) and factories (e.g. robotic arms, assembly line), where the task is relatively constrained. Automation is now becoming increasingly viable in unstructured ‘open’ environments, such as the driving domain. Here, full automation is possible under some conditions, but not all. For example, in the current state of technology, computer vision algorithms are unable to detect and understand the social cues of vulnerable road users (Camara et al. Citation2021; Rudenko et al. Citation2020; Saleh et al. Citation2017). Although there have been continual advancements in sensors and artificial intelligence, automation in unstructured environments currently still requires some form of human supervision, interaction, or correction.
Given that human involvement is necessary, the question is how to design human-automation interaction, and in particular whether driving functions should be traded between human and automation, or shared. The topic of sharing versus trading was first addressed by Sheridan and Verplank (Citation1978), who explained: ‘In the case where both computer and human are working on the same task at the same time, we call this SHARING control. When they work on the same task at different times this is TRADING control’ (4–6) (). Thus, in traded control, the human operator delegates tasks to the automation, supervises the automation, and occasionally resumes manual control. On the other hand, in shared control, human and automation perform the same task congruently (Abbink et al. Citation2018; Sheridan and Verplank Citation1978).
Figure 1. Traded control and sharing control (from Sheridan Citation1991) as considered in the context of aviation in the 1990s. More recently, the topic has become important in the field of driving. In that case, the human is the driver, the computer is the automation, and the aircraft is the car. In traded control, either the driver or the automation controls the vehicle, whereas in shared control, they exert control inputs (e.g. forces) that jointly control the vehicle.
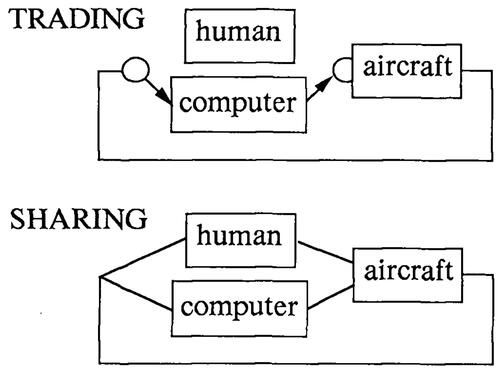
The term shared control tends to be used inappropriately in the literature, even by human factors experts (Tabone et al. Citation2021), to describe any human-automation interaction in which the automation is imperfect and the human has a vital role. However, as noted by Norman (quoted in Tabone et al. Citation2021), many of such interactions are, in fact, traded control, in which the human has to monitor while the automation executes a control task. In an attempt to define shared control more sharply, Abbink et al. (Citation2018) explained that shared control refers to a congruent human-automation interaction in a perception-action cycle to perform a task that the human or the automation can, under the right conditions, also perform alone. They clarified that the use of warning and decision support systems does not qualify as shared control. These systems provide unilateral suggestions on what action the human should (not) take, while the human subsequently has to make the decision and execute the action. Thus, although sensory and perceptual task elements are shared between human and machine, there is no congruent interaction. Stability and control augmentation systems do not belong to the category of shared control either, as such systems are meant to perform tasks that humans cannot do by themselves. For example, the control of various military aircraft relies on rapid computer-based ‘inner loop’ control, as such aircraft have marginal or even negative aerodynamic stability (Avanzini and Minisci Citation2011). In these cases, although humans and automation perform a task at the same time, it is not the same task that is shared; in fact, if the computer fails, the aircraft will be unable to fly.
Shared control can come in a variety of forms. Two main types of shared control can be distinguished (Abbink et al. Citation2012): shared control involving a physically coupled interaction between input device and vehicle or robot (called haptic shared control; Benloucif et al. Citation2019; Ghasemi et al. Citation2019; Griffiths and Gillespie Citation2005; Losey et al. Citation2018; Mars et al. Citation2014) and shared control involving a physically decoupled interaction (also called: blending, indirect, or input-mixing shared control; Crandall and Goodrich Citation2002; Dragan and Srinivasa Citation2013; Storms and Tilbury Citation2014). We refer to review papers for additional consideration and detail (Ghasemi et al. Citation2019; Marcano et al. Citation2020; Wang et al. Citation2020). Shared control methods involving multiple humans and/or multiple robots (Crandall et al. Citation2017; Gao et al. Citation2005; Musić and Hirche Citation2017; Shang et al. Citation2017; Tso et al. Citation1999), or methods where human and automation jointly arrive at a plan, decision, or strategy have been conceived as well (e.g. Abbink et al. Citation2018; Kaber and Endsley Citation2004; McCourt et al. Citation2016; Pacaux-Lemoine and Itoh Citation2015). It is further noted that shared control, like traded control, does not need to refer to the entire task but can also be applied to separate control inputs. For example, a driver may use haptic shared control on the steering wheel, but without car-following assistance. In this example, lateral control is shared, while longitudinal control is performed manually. If the same driver uses adaptive cruise control (ACC), then lateral control is shared, and longitudinal control is traded. If the same driver uses a haptic gas pedal (e.g. Mulder et al. Citation2008), then lateral and longitudinal control are both shared.
Traded control may also come in variants that differ in their activation and deactivation triggers. For example, a human may enable the automation system via a button press, but in time-critical conditions, the automation may activate automatically and override the human (Lu et al. Citation2016). The latter solution is seen in advanced emergency braking (AEB), for example. Parasuraman et al. (Citation1992) report several other activation triggers for traded control, such as task phase (e.g. an automated vehicle may change mode depending on geospecific information) and physiological signals (e.g. an automated vehicle may switch back to manual if it determines that the driver is distracted). Each of these examples is a manifestation of traded control.
Since the seminal work of Sheridan and Verplank (Citation1978), a large number of human factors researchers have examined specific variants of traded control and shared control. However, only a few articles have empirically evaluated sharing and trading side-by-side (Metcalfe et al. Citation2010; Van Dintel et al. Citation2021) or have provided a theoretical outline on sharing versus trading (Inagaki Citation2003). De Winter and Dodou (Citation2011) provided a critical reflection on shared control in driving, noting that it yields only meagre performance benefits compared to manual control. Abbink et al. (Citation2012, Citation2018) explained that the aim of haptic shared control is not to improve performance, but to keep humans in the loop and to let them catch automation errors when the automation (suddenly) reaches the limits of its capabilities. Although a large number of empirical papers have been published on shared control and traded control, a rigorous reflection on the pros and cons of these methods of human-automation interaction seems lacking.
1.1. Aim
The present article offers critical reflections on the arguments for and against shared or traded control, which originated from debate sessions the authors organised during human factors lectures over the past 10 yearsFootnote1. This write-up of the debate is meant to stimulate the exchange of ideas, and to formulate new hypotheses for research, an approach sometimes used in academic outlets (e.g. Wilde et al. Citation2002, for a debate on a road safety theory with a ‘for’ vs. ‘against’ structure).
In a debate, it is important to clearly define the positions of both parties. That is why it was decided not to focus the debate on shared and traded control in general, but on the specific domain of car driving. So far, the introduction of automation in driving has been evaluated mostly through the lens of traded control. To illustrate, Zhang et al. (Citation2019) documented as many as 129 experiments that measured how quickly drivers take over control from an SAE Level 3 ‘traded control’ automated driving system, while Jansen et al. (Citation2022) surveyed 189 studies on the design of take-over warnings. To encourage sharp debate, it was decided to focus on one specific type of shared control, namely haptic shared control. In this type of shared control, the driver receives guiding torques at the control interfaces, but there remains a directly coupled relationship between input (e.g. steering wheel angle) and output (e.g. wheel angle). Basic haptic shared control solutions in the form of torque feedback for hands-on-wheel lane-keeping assistance are currently available on ithe automotive market (Reagan et al. Citation2018).
This article first surveys known and lesser-known pitfalls of human-automation interaction in general. These pitfalls have mostly been studied in the context of traded control, but as this debate paper will elucidate, some of the pitfalls may also apply to shared control. Although the pitfalls of automation, sometimes called ironies of automation, have already been recognised for many decades (Bainbridge Citation1983; and see Parasuraman and Riley Citation1997, for automation misuse and disuse specifically), a concise yet comprehensive overview of the origins, definitions, and operationalisations of the pitfalls was still lacking in the literature.
Next, we focus on driving and present the position of one of the authors that haptic shared control is the remedy for these pitfalls and the recommended way for introducing automation on the roads. This is followed by a rebuttal that argues that haptic shared control is not the way forward and explains why traded control is the better alternative. We end with a reflection towards a consensus by outlining the main factors that determine whether to share or trade control.
2. De winter: general pitfalls of automation
Although automation offers many benefits, it comes with several pitfalls, as a result of which the benefits of automation are not realised to their full extent. When examining the literature, six pitfalls can be identified. These pitfalls all relate to the traded-control solution for human-automation interaction. In traded control, the human is not in direct control of the process, but most of the time supervises the automation. That is, the human intermittently monitors and adjusts the automation, which itself controls the process (Sheridan Citation1992). This brings the human out of the direct control loop, resulting in problems when the human needs to be in control again after the boundaries of the operational design domain are exceeded.
2.1. Pitfall 1: loss of situation awareness and mode awareness
When supervising automation, the human may sample limited information from the task environment and automation-status displays, as a result of which the human may lose situation awareness, mode awareness, or both.
First, loss of situation awareness occurs when the human fails to detect and perceive relevant elements of the environment, does not comprehend the meaning of these elements, and does not anticipate how the environment will evolve (Endsley Citation1995; see for an illustration of the meaning of situation awareness).
Figure 2. Illustration of the meaning of situation awareness. Situation awareness is high if the driver is able to perceive relevant elements in the task environment (turned wheel of the pink sports car), comprehend its meaning (it might pull out), and predict what will happen in the figure (I may have to brake) (from Vlakveld Citation2011; see also De Winter et al. Citation2019). The term situation awareness has been regarded as equivalent to hazard anticipation (Horswill and McKenna Citation2004).
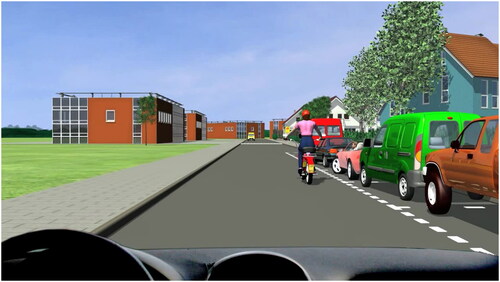
Loss of situation awareness can be detected in virtual environments through so-called freeze-probe methods, such as the Situation Awareness Global Assessment Technique (SAGAT; Endsley Citation1988), whereas in real environments, real-time probes such as the Situation-Present Assessment Method (SPAM; Durso et al. Citation1998) and eye-tracking (De Winter et al. Citation2019) can be used. In certain applications, basic human-monitoring systems are already available. For example, driver monitoring systems that use cameras to detect whether the driver is keeping attention to the road can be found in several car models, which can then warn the driver to redirect attention to the main task (e.g. BMW Citation2019; and see Stein et al. Citation2019, for a system developed for train drivers).
Although automation is known for being able to cause a loss of situation awareness, the literature contains several examples where automated driving improves situation awareness, something that can occur if the driver is motivated or incentivized to remain attentive to the task environment (for a review of experimental data in automated driving, see De Winter et al. Citation2014; for an interview study of Tesla Autopilot users, see Nordhoff et al. Citation2022). A likely explanation is that because the automation is in control, the human is offloaded from necessary manual tasks and therefore may have more spare visual and mental capacity.
Second, mode awareness may occur when humans lose awareness of the mode the automation is in, where the term mode refers to the automation’s functionality (control laws, settings) that is currently active. Humans may then commit mode errors, where the ‘system behaves differently depending on the state it is in, and the user’s action is inappropriate for the current state’ (Norman Citation1980, 32). In the same vein, Potter et al. (Citation1990) defined a mode error as ‘acting in a way appropriate to one mode when the device is actually in another mode’ (393). Mode errors are more likely when the automation features a large number of modes and when mode changes can be triggered automatically based on environmental triggers (Sarter and Woods Citation1995).
Mode errors have been implicated in aviation accidents (Silva and Hansman Citation2015), oil spill accidents (Seastreak Wall Street; National Transportation Safety Board, Citation2014)Footnote2, and industrial accidents (Three Mile Island; Rogovin and Frampton Citation1980), and also start to be of concern in daily tasks in which automation finds its entrance (Andre and Degani Citation1997), such as driving (Wilson et al. Citation2020). Current automated driving systems consist of various subsystems, such as lane-keeping assistance, AEB, and traffic light detection, and drivers may have difficulty understanding which of these subsystems are active (mode on vs. off, or their current functionality) (Banks et al. Citation2018; Dönmez Özkan et al. Citation2021; Feldhütter et al. Citation2018).
2.2. Pitfall 2: deskilling
Known under various terms, including skill erosion (Wall et al. Citation1980), skill loss (Wiener and Curry Citation1980), skill degradation (Birkemeier Citation1968), and skill atrophy (Sheridan et al. Citation1983), deskilling refers to the hypothesis that the human operator may, over time, lose manual control skills because of automation useFootnote3. That is, because of not rehearsing manual skills, operators become less proficient relative to those who continue to perform the task manually. Deskilling is a frequently mentioned topic in the human factors literature (Billings Citation1997; Endsley and Kiris Citation1995; Ferris et al. Citation2010; Lee and Seppelt Citation2009).
Experiments on the retention of skills in tracking and procedural tasks show that performance declines with the duration of the no-practice interval (Ammons et al. Citation1958). Other research suggests that retention of performance in tracking tasks is generally high (Fleishman and Parker Citation1962) and that performance on discrete procedural tasks is forgotten more readily than performance in tracking tasks (see Adams Citation1987, for a review). shows participants’ performance on a tracking task during a practice phase and a retention phase 1–2 years later. In this lab experiment by Ammons et al. (Citation1958), participants had to keep a model plane, which was affected by various disturbances, ‘on target’, i.e. level and pointed straight ahead. Deskilling can be recognised from the beginning of the retention interval, that is, performance in the first 15 min after the long break was lower compared to the end of the practice phase.
Figure 3. Results from classical research which showed that deskilling can be identified after a long no-practice interval (Ammons et al. Citation1958; data extracted from combined). Participants practiced a pursuit tracking task (Airplane Control Test) for two degrees of practice: 1 h (n = 150) or 8 h (n = 150). They then performed a retention test after a no-practice interval of either 24 h, 1 month, 6 months, 1 year, or 2 years (n = 30 per group). The present figure shows the tracking performance as a function of elapsed time-on-task in the practice and retention phases, for the participants with a 1- or 2-year no-practice interval combined (n = 60 for the 1-h group, n = 60 for the 8-h group). Deskilling can be identified for the approximate first 15-min of the retention phase, i.e. participants’ performance was lower compared to the performance in the end of the practice phase. The figure also shows that the participants recovered quickly (in about 15 min).
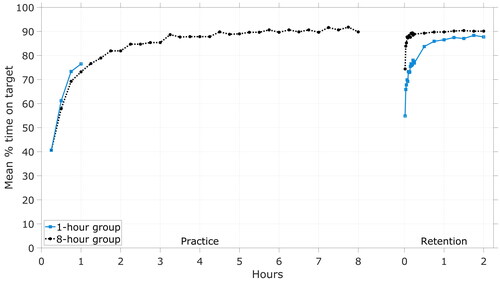
In the area of human-automation interaction, experimental evidence for deskilling is scarce, or as noted by Wood (Citation2004): ‘Little research exists to provide a structured basis for determination of whether crews of highly automated aircraft might lose their manual flying skills’ (v). The reason may be that proving whether deskilling has occurred requires a longitudinal study with an experimental group that agrees not to use the automation for an extended period and a control group that continues to use the automation, something that is difficult to accomplish.
So far, the evidence of deskilling is correlational (Casner et al. Citation2014; Ebbatson et al. Citation2010). For example, a flight simulator study by Haslbeck and Hoermann (Citation2016) found that short-haul pilots had better manual control skills than long-haul flight pilots, presumably because the latter group completed fewer landings per year. However, it is difficult to draw definitive conclusions because the two groups flew different aircraft (i.e. different weight classes). It is also difficult to determine whether a skill deficit is caused by the small amount of initial practice in manual flying or whether it is caused by forgetting how to fly manually due to the no-practice interval (cf. ).
At the same time, anecdotal evidence of deskilling is abundant. We can refer here to matters of daily life where it is said that people have become less proficient in mental arithmetic due to the introduction of computers and calculators (e.g. Penny Citation2022). An interview study by Nordhoff et al. (Citation2022) revealed that some users of Tesla’s Autopilot felt uncomfortable or somewhat scared when having to drive a manual car after being used to automated driving. In aviation, it has been reported that airline pilots have little stick time and that simulator training is essential to maintain manual skills (Doyle Citation2009; Hanusch Citation2017). The Federal Aviation Administration (Citation2017) issued a Safety Alert for Operators, asking air carriers to ‘permit and encourage manual flight operations….when conditions permit, including at least periodically, the entire departure and arrival phases, and potentially the entire flight, if/when practicable and permissible’.
2.3. Pitfall 3: unbalanced mental workload
When supervising automation, human operators may (have the impression that they) have little to do because the computer controls the task environment. The human operator should set up and monitor the automation, but most of the time does not have to provide any inputs. This situation may lead to low workload, where workload can be defined as ‘the cost of accomplishing task requirements for the human element of man-machine systems’ (Hart and Wickens Citation1990, 258).
Although low mental workload is not a problem when the automation works as intended, it may lead to problems when the automation acts on the wrong information, makes wrong decisions, or fails altogether. As noted by Fitts (Citation1951, 6): ‘if the primary task of the human becomes that of monitoring, maintaining, and calibrating automatic machines, some men will need to be capable of making intelligent decisions and taking quick action in cases of machine breakdown or in unforeseen emergencies.’ The phrase ‘99% boredom and 1% sudden terror’ (Bibby et al. Citation1975, 665)Footnote4 captures the notion that workload can increase strongly when the human has to reclaim control. The human operator may have to comprehend warning signals on the human-machine interface or diagnose why the automation malfunctions. Krebsbach and Musliner (Citation1999) noted that ‘minor incidents may cause dozens of alarms to trigger, requiring the operator to perform anywhere from a single action to dozens, or even hundreds, of compensatory actions over an extended period of time’. When the human operator is stressed or mentally overburdened, accident probability is elevated, especially when the human needs to regain situation awareness simultaneously.
In non-automated tasks, humans can usually cope well with high task demands, something that Norman and Bobrow (Citation1975) called the ‘principle of graceful degradation … when human processes become overloaded, there often appears to be a smooth degradation on task performance rather than a calamitous failure’ (44–45). Similarly, Fitts (Citation1951) used the example of a telephone switchboard, with switchboard operators managing to connect most calls even in busy times while ‘automatic dial telephone systems are known to have broken down completely under overload conditions’ (6). This problem of automation has also been called automation brittleness, i.e. automation operates well for the range of conditions for which it was designed but requires human intervention for situations not covered by its programming (Cummings et al. Citation2010). When automation fails abruptly, the human is confronted with a situation that needs immediate resolution. The term clumsy automation is used for automation that has been designed in such a way that it reinforces issues of unbalanced mental workload rather than resolves it (Cook and Woods Citation1996; Wiener Citation1989).
Hart and Wickens (Citation1990) explained that workload ‘may be reflected in the depletion of attentional, cognitive, or response resources, inability to accomplish additional activities, emotional stress, fatigue, or performance decrements’ (258). Accordingly, mental workload can be measured using questionnaires, such as the widely used NASA Task Load Index (Hart and Staveland Citation1988). The use of secondary tasks to measure spare mental capacity (Brown and Poulton Citation1961; Williges and Wierwille Citation1979) and eye-tracking and other physiological measurements are common as well (Cain Citation2007; Kramer Citation1991; Marquart et al. Citation2015). Under conditions of workload and stress, common physiological responses are visual tunnelling (Rantanen and Goldberg Citation1999), increased heart rate (Roscoe Citation1992), and dilated pupils (Kahneman and Beatty Citation1966). Psychophysiological signals can act as a dependent variable in human-subject experiments, or in combination with performance measures to assess workload/performance (dis)associations (Hancock Citation1996). Alternatively, psychophysiological signals can serve as a trigger in traded-control automation systems (Cabrall et al. Citation2018).
2.4. Pitfall 4: behavioural adaptation
Behavioural adaptation refers to the fact that human operators may be inclined to use new technology, including robotic or automation systems, in such a manner that the benefits of this technology are not realised to their expected extent. The Organisation for Economic Co-operation and Development (Citation1990) defined behavioural adaptations in the context of driving as ‘those behaviours which may occur following the introduction of changes to the road-vehicle user system and which were not intended by the initiators of the change’ (115).
Risk compensation/homeostasis (cf. Wilde Citation1982), but also workload/difficulty homeostasis (Fuller Citation2005), control-activity homeostasis (Melman et al. Citation2018), a satisfaction of personal needs (Organisation for Economic Co-operation and Development Citation1990), or a production/protection trade-off (Reason Citation1997) are among the proposed mechanisms behind behavioural adaptation. The essence of behavioural adaptation is that automation, which helps perform tasks, results in a compensatory mechanism by the human operator.
Behavioural adaptation has been observed in manual control and human-supervised automation. When ships were fitted with radar in the mid-twentieth century, this protective feature resulted in the vessels travelling faster through the seaways (Schmidt Citation1958; see also Reason Citation1997). Similarly, when a simulated vehicle was equipped with ACC, drivers drove this vehicle at greater speeds compared to manual driving (Hoedemaeker and Brookhuis Citation1998). Thus, the automation feature was used in such a way that the safety benefits were not realised to their expected extent, or, in the words of Elvik (Citation2004), the engineering effect (e.g. a radar providing better visibility compared to no radar) was offset by a behavioural effect (sailing the ship faster with radar than without), see .
Figure 4. Illustration of behavioural adaptation, where an automation feature is less effective than it could be, because of an adverse behavioural effect that mitigates the engineering effect (based on Elvik Citation2004).
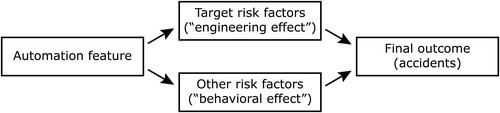
Behavioural adaptation has been described as a universal phenomenon of systems (Wilde Citation2013). Also at a macroscopic level, protective features may lead organisations to take more risks (Reason Citation1997). Behavioural adaptation can also be seen in other forms of human-machine interaction. For example, in military robotics, there are concerns that remoteness from actual hazards may cause operators to engage in war activity more easily (Lin et al. Citation2008).
2.5. Pitfall 5: misuse
Misuse refers to the unjustified use of automation by its user. The term is often used in conjunction with the terms overtrust (i.e. trust that is too high given the automation’s actual reliability), overreliance, and complacency (i.e. insufficient scepticism and insufficient counterchecking of automation functioning). Misuse also relates to the term automation bias, that is, the user’s reliance on automation to preserve cognitive effort (Parasuraman and Manzey Citation2010).
Automation misuse may be exacerbated by a poor human-machine interface, for example, when the automation modes depicted on the interface are insufficiently salient or when the automation’s behaviour is insufficiently transparent. Billings (Citation1991) explained: ‘automation … should never be permitted to fail silently’ (85). Furthermore, highly reliable automation may give the impression that the automation is perfectly reliable, as a result of which the operator may enter a state of overtrust (). For example, Radlmayr et al. (Citation2018) showed that time to collision (TTC) in a take-over scenario was reduced when the automation reliability was 100% compared to 50%-reliable automation and manual driving, i.e. participants braked later because they may have assumed that automation would handle the situation for them. According to Parasuraman and Riley (Citation1997), low operator confidence and low skill are other purported causes of misuse, as unskilled operators may more easily delegate responsibility to automation.
Figure 5. Illustration that says that misuse and disuse arise from miscalibrated trust in automation (from Gempler Citation1999; Merlo et al. Citation1999).
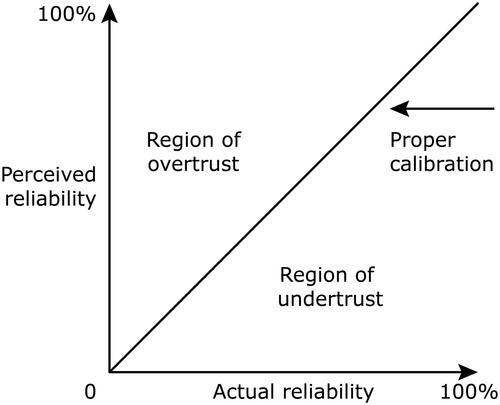
Misuse of automation has often been reported as a cause of accidents. One of the first fatal accidents involving a partially automated vehicle, for example, has been attributed to overreliance on automation (National Transportation Safety Board Citation2017). In this case, the car crashed into the side of a truck, while the driver was reported to have ignored various hands-on-wheel alerts.
Misuse can also manifest itself when interacting with decision aids. An error of commission is said to occur when the human follows the advice of a decision aid even though other available indicators suggest that this advice is invalid (Skitka et al. Citation1999). Bahner et al. (Citation2008) found that operators who made more errors of commission while supervising a simulated life support system of a spacecraft showed signs of complacency, i.e. they were less likely to countercheck the raw sensor data. Oppositely, errors of omission occur when the human operator did not take appropriate action because the decision aid failed to provide advice (Skitka et al. Citation1999). Lin et al. (Citation2017) reviewed 158 errors of omission and commission associated with the use of GPS devices while driving, 28% of which involved a fatality.
2.6. Pitfall 6: disuse
Disuse is the opposite of misuse and refers to the failure to use automation when automation use would, in fact, be appropriate. There are various causes of disuse. One factor is the human’s general negative predisposition to automation or dislike of ‘bells and whistles’ in the cockpit. The literature reports substantial individual differences in automation (dis)use, with some accidents caused by operators (creatively) disabling the automation system (Parasuraman and Riley Citation1997).
A second factor is annoyance or distrust that arises due to the need to switch off the automation and false alarms (Bliss Citation2003; Muir and Moray Citation1996; Sorkin Citation1988). Parasuraman et al. (Citation1997) explained that false alarms are essentially unavoidable given omnipresent sensor noise and the imperfect predictability of the task environment: If the warning threshold is liberal, the operator has sufficient time to respond, but at the cost of false alarms. On the contrary, if the warning threshold is strict, the likelihood of false alarms is low, but the operator may have too little time to intervene. Hence, imperfect automation implies that the human operator should decide when not to use or ignore the automation. As a result, some operators may develop distrust in automation () and never reap its benefits.
Also with today’s assisted/automated driving, such as ACC and lane-keeping assist, drivers show disuse for reasons related to personal predispositions (e.g. ‘I don’t need it’, ‘I am able to stay in the lane myself’) and low perceived reliability (‘The system does not work well’, ‘The system annoys me’, ‘The systems distract me and I want to concentrate only on driving’) (Stiegemeier et al. Citation2022).
2.7. Automation pitfalls summary
In summary, automation induces a number of pitfalls. Pitfalls 1–3 concern a change in human knowledge or mental/physiological state, while Pitfalls 4, 5, and 6 concern a change in the way the human operator uses automation.
Apart from these six pitfalls, which describe the effects on human operators, researchers have been concerned with the broader implications of automation. The term abuse of automation (Parasuraman and Riley Citation1997) describes the implementation of automation by managers without due regard for its implications for human well-being and safety. Others have lamented that automation may cause a loss of jobs or job dissatisfaction (Bhargava et al. Citation2021; McClure Citation2018), issues going back to the Luddite movement (Darvall Citation1934; Hobsbawm Citation1952). These broader factors are beyond the scope of the present work.
3. Abbink: a solution to the pitfalls: haptic shared control
I fully agree with the above pitfalls of automation, and argue that they are very much present in the automotive domain. It should be noted that these pitfalls are not the result of automation itself, but rather the consequence of the design choice to use traded control for driver-automation interaction. I argue that traded control should be avoided and that shared control is the solution to the discussed pitfalls.
3.1. In an open world, driver input will remain needed
For many decades, society has been promised fully self-driving vehicles. As early as Citation1956, America’s Independent Electric Light and Power Companies published an ad depicting self-driving vehicles in which families were comfortably transported on the highway. In the meantime, many vehicles have been demonstrated to operate ‘driverlessly’ under specific conditions, with the latest examples, such as Waymo, operating in the less congested parts of some major cities. However, we are still a long way from having driverless vehicles commonly available, operating without human input always and everywhere.
So far, commercially available vehicles are not self-driving, but rather automated, which means that the driver must remain attentive at all times. Higher levels of automation that permit the driver to engage in non-driving tasks are mostly still in a research phase. These higher levels of automation would have to be able to provide enough time (typically discussed as being 10–30 s) to allow the driver to get back in the loop. Note that even if it becomes technologically feasible to ensure lead times that are sufficiently large for drivers to engage in multitasking while being able to take over control (beyond slow-moving traffic), such solutions are likely to suffer from deskilling (Pitfall 2) and behavioural adaptation (Pitfall 4). In other words, in the present state of automation technology, there is still an important role for the driver, and safe traded-control solutions hardly exist.
Real-world evaluations of commercially available traded-control automation systems confirm that these systems are not immune to unsafe incidents. The first broad examination of crashes involving automated driving technologies on US roads showed that almost 400 crashes were reported by automakers between July 2021 and May 2022 (National Highway Traffic Safety Administration Citation2022a). Kim et al. (Citation2022) showed that participants experienced safety-critical events with traded-control automation such as ACC. The same study also showed that drivers felt comfortable engaging in secondary tasks (Pitfall 5), something they clearly should not, given the safety-critical events mentioned above. Participants also reported discomforting situations (automation disengagements, insufficient braking or acceleration), as well as more dangerous events such as unnecessary braking without any lead vehicle, or even silent automation failures (Kim et al. Citation2022). These phenomena may result in annoyance and disuse (Pitfall 6).
Three phenomena outside of the academic literature further illustrate the need for human inputs in automated driving. First, no car manufacturer assumes responsibility for accidents when their automation mode is being used, and only a few car manufacturers seem to commit themselves to Level 3 or higher automation. To leave the driver legally responsible for situations in which automation fails to work can be seen as moral scapegoating (Elish Citation2019) and points to so-called responsibility gaps (Calvert et al. Citation2020) and a lack of meaningful human control (Siebert et al. Citation2021). A recent study (Beckers et al. Citation2022) illustrates such moral scapegoating in the general public. When asking participants to attribute blame for a crash resulting from a sudden automation failure, which the driver could in principle prevent when not suffering from lack of situational awareness (Pitfall 1), the respondents would blame the driver rather than the manufacturer or legislator. Second, ironically, during the outbreak of COVID-19, the major developers of ‘driverless cars’ had to shut down their operations due to the inaccessibility of test drivers (Vengattil Citation2020). In fact, it can be argued that the only reason why ‘driverless cars’ are able to drive around safely outside designated areas is that test drivers catch all automation failures. Third, a cursory glance on YouTube (e.g. ‘autopilot’ failures) reveals ample examples where supposedly driverless vehicles appear unable to perform basic operations, such as interacting with pedestrians, handling intersections, late merging, or even staying in the lane. Automated vehicles can also be misled by ambiguous perceptual information (e.g. false-positive traffic light detections), and misused by pedestrians (e.g. Millard-Ball Citation2018).
Of course, high-end self-driving cars are being tested on public roads, but there is a big difference between demonstrating that automation can work versus demonstrating it can work always and everywhere. Remember that already in 1947, a fully automated plane demonstrated the ability to perform take-off, flying, and landing (Boxer Citation1948; Howard Citation1973). Today, we still need two pilots in the cockpit to guarantee high levels of safety. Similarly, in the automotive domain, the challenge is not only to demonstrate autonomous capabilities on wide roads under uncongested and sunny conditions but to make the automation work always and everywhere in mixed traffic. As long as drivers are responsible for always taking over, we have to deal with driver-automation interaction and the associated pitfalls of automation.
3.2. Why traded control works well in aviation but not in driving
The traded control approach has been widely used in commercial aviation. However, this has only been possible because pilots are selected, extensively trained, and paired to operate the aircraft, because there are strict procedures, checklists, and assistance through air traffic control, and because time margins are generally much larger than in the driving domain. Yet even in aviation, the six pitfalls of automation are a concern.
The pitfalls of automation are more severe in the automotive domain than in the aviation domain. The requirements for automation algorithms (including underlying computer vision) are more daunting since the driving environment is less structured and more dynamic, and generally with much smaller temporal safety margins. Engineers are inclined to think that collecting more data will allow them to train smarter neural networks, resulting in more intelligent automated vehicles that will soon not require human input. This has been the narrative for the last two decades, often citing Moore’s law. Yet the actual exponential growth that takes place concerns the billions of dollars spent on creating supposedly driverless vehicles (Metz Citation2021). Today, there are no driverless vehicles that function always and everywhere; and how many times did drivers intervene to correct a dangerous situation? We still need human input (see also Noy et al. Citation2018), and traded control is not the right design approach for that.
Automation, such as AEB and automated evasive steering (AES), may indeed save lives and prevent safety-critical situations. However, such automation systems do not replace the driver always and everywhere, but constitute ‘envelope protection’ only for specific cases that are beyond human control bandwidth. AEB, for example, engages only at the last possible moment (when time-to-collision drops below, e.g. 0.8 s; Federal Ministry of Transport and Digital Infrastructure Citation2015; United Nations Citation2013), and the pitfalls of automation are therefore much less of a concern: No driver presses the gas pedal down and trusts the AEB system to maintain safety for them. Also, the AEB controllers are not useful for nominal car-following (see the definition of shared control in the Introduction, which stated that shared control concerns tasks either the human or the automation can perform). Therefore, such systems should not be confused with the current debate about trading or sharing control over lateral and longitudinal tasks, which either the automation or the driver could do.
Inappropriate human-automation interaction costs lives, as was dramatically made clear by fatal accidents involving Tesla and Uber, where ‘the automatic system misperceived a situation that a human driver would be very unlikely to misperceive’ (Noy et al. Citation2018, 70). Investigation reports on these crashes refer to the driver’s distraction and overreliance on automation as key causes (Pitfalls 1 & 5; National Transportation Safety Board Citation2019a, Citation2019b, Citation2020).
How many more crashes will occur for higher penetration rates of vehicles that apply traded-control automation? And what should guide our design solutions? Should we attribute these crashes to human error, or should we see them as the result of inappropriate human-automation interaction design? Consistent with the systems view on human error (Read et al. Citation2021), I argue the latter: These tragic accidents occur because traded control is used where it should not be, exactly because it produces the well-known associated pitfalls of automation. In fact, researchers have been talking about these pitfalls for many decades, such as Bainbridge (Citation1983) in her work ‘ironies of automation’: Why expect this to be different in the automotive domain?
In short, we should not apply the traded control paradigm to the driving domain, even if it is successful in aviation. The six pitfalls of automation will certainly be more pernicious in driving compared to aviation: automation limitations will be encountered more often and with much less time to respond, and drivers will largely remain non-professionals. So, if the problem is human-automation interaction design, what is the solution?
3.3. Haptic shared control as a solution
I, in agreement with many colleagues, advocate that when a driver is still ultimately responsible while interacting with automated systems, the driver and automation should not trade control but should share control (Abbink et al. Citation2012). In the automotive domain, the shared control approach has generated much research in design and evaluation (Flemisch et al. Citation2003; for reviews, see Abbink et al. Citation2018; Marcano et al. Citation2020).
Note that shared control and traded control are interaction design choices for exactly the same automation system (see ). As Grill Pratt of Toyota put it: ‘The technology that will eventually make self-driving cars safe can be implemented in human-driven cars’ (Ross Citation2018). If the driver is coupled to the automation via haptic shared control, the driver is continuously informed and guided, leading to a more balanced workload (reducing Pitfall 3) but also allowing the driver to feel the automation limitations, thus resulting in better mode and situation awareness (Pitfall 1) and better trust calibration (Pitfalls 4, 5 & 6). This continuous engagement in the driving task can also be expected to mitigate the erosion of skills (reducing Pitfall 2). Accordingly, a shared control approach to human-automation interaction can be expected to be more resilient than a human-alone or automation-alone approach. Of course, this means that the driver cannot let go of the vehicle controls and engage in secondary tasks, i.e. the promise of fully autonomous vehicles. But to be clear, neither should drivers do this with traded control!
The driver must understand the automation, including its boundaries and system failures, and the automation must also understand the driver. In currently available traded-control automation, this understanding is achieved via warnings and other displays that aim to keep the driver’s eyes on the road or request the driver to intervene when needed. Furthermore, when a driver in the supervisory role prefers another action, the driver will have to adjust setpoints (e.g. setting different target headways in ACC), while the automation disengages as soon as the driver exerts a small control action. This non-continuous form of communication (i.e. warnings, changing of automation parameters, automation on/off) is characteristic of all traded-control automation (Sheridan Citation2011).
Haptic shared control, on the other hand, involves continuous force feedback on a control interface (e.g. the steering wheel) without decoupling the mechanical connection between the steering wheel and front wheels. It allows drivers to develop a feel for the driver-automation interaction, which may help in building up appropriate mental models of the automation capabilities and behaviours. An appropriate metaphor for this is the horse metaphor (Flemisch et al. Citation2003). In horse riding, the rider and horse have a dynamic relationship: They communicate via forces and tactile cues to understand each other’s limitations and to learn about each other’s behaviour over time.
In our lab, which started in 2002, we developed a haptic gas pedal as an alternative to the traded control approach of ACC. Using the functionality of the same automation system behind ACC, we used its inputs to translate them into forces on the gas pedal. This approach reduces control effort, increases safety margins to the lead vehicle, and allows drivers to respond to automation failures more quickly. Together with colleagues in the shared control community, this concept was extended to lateral control, exploring different design options, including adjustments of the steering wheel stiffness (e.g. Abbink and Mulder Citation2009; Saito et al. Citation2021). With haptic shared control for lane-keeping (commercially available as active lane-keeping or lane-keeping assist), drivers continuously feel the automation’s intentions to steer, which they can follow or correct, without prompting the automation to switch off. When drivers correct the force feedback, they provide context-specific cues to the automation that can be used to update the reference trajectory (Scholtens et al. Citation2018). In this way, one enables a bidirectional channel of communication (Abbink et al. Citation2012), as well as a channel for reciprocal learning, which we have coined symbiotic driving (Abbink et al. Citation2018).
Does the concept of haptic shared control work? Many driving simulator studies have provided evidence that it does. For example, Mulder et al. (Citation2012) found that haptic shared control in a steering task improves lane-keeping performance compared to manual steering (, left panel). Note that the forces are relatively small, and when releasing the steering wheel, a relatively poor lane-keeping performance will result, although the vehicle still stays on the road (see , left panel). In short, when using haptic shared control, the joint system (i.e. driver and automation together) yields better performance than manual driving (driver alone) and automated driving (hands-off driving), i.e. ‘the whole is greater than the sum of its parts’. But these safety and comfort benefits in basic driving environments are not the main benefit of shared control. It is because the driver stays within the loop that automation pitfalls are mitigated. An example of this is illustrated in the right panel of , where in the case of a silent automation failure right before a curve, drivers with shared control (green line) easily recover and stay on the road, while drivers with traded control (red line) get back in the loop too late.
Figure 6. Example of the benefits of shared control on a road with mild curves (left panel) and upon automation failure (right panel). The left panel (adapted from Mulder et al. Citation2012) shows that haptic shared control on the steering wheel results in better driving performance (i.e. smaller maximal lateral excursions, averaged across participants) than manual control and automatic (= haptic shared control with hands-off) control. Note that no comparison with traded control is shown. The right panel (from Flemisch et al. Citation2008) depicts behaviour right after a silent automation failure that reverts the driver back to manual control just before a curve.
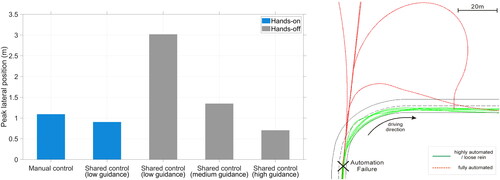
In conclusion, haptic shared control is a design philosophy that impels drivers to stay in the loop by providing small safety and comfort benefits while mitigating automation pitfalls that occur when the automation’s limits are exceeded. Continuous engagement of the driver with the automation allows drivers to build a mental model of the automation, inherently augmenting mode awareness and situation awareness (Pitfall 1), avoiding deskilling (Pitfall 2), maintaining appropriate workload (Pitfall 3), and discouraging misuse (Pitfall 5). Behavioural adaptation (Pitfall 4) does still occur with shared control but can be anticipated and resolved in the interaction design. Additionally, the continuous communication avoids the issues with threshold settings that plague binary trading or alerts (reducing disuse, Pitfall 6) and engages the fast neuromuscular reflexes that allow quick responses also used in walking or biking. Moreover, because the forces constitute bidirectional communication, the automation can learn from corrections and adapt trajectories to reduce conflicts, which may prevent misuse (Pitfall 5) and disuse (Pitfall 6).
Note that the interaction paradigm of haptic shared control, like that of traded control, can be linked to any automation system that generates target steering inputs. Once the automation is reliable enough for automotive companies to take responsibility when the automation is active, traded control is the best solution. But as long as drivers are held responsible when automation fails and are required to be able to intervene at any time, shared control should be used.
4. De Winter: use traded control, not shared control
Abbink makes a caricature of the capabilities of automated driving and suggests that this technology is so dangerous that only a few car manufacturers seem to commit themselves to it. He fails to mention that companies such as Waymo currently deploy vehicles that do not even have a steering wheel, something regulators are already adapting to (National Highway Traffic Safety Administration Citation2022b), while Tesla’s beta-testing program demonstrates increasingly compelling automated driving in city environments. In the same vein, in Europe, Mercedes-Benz recently claimed to be the first to deploy a hands-off SAE Level-3 automated driving system on public roads, where under certain conditions, ‘the driver can focus on other activities such as work or reading the news on the media display’ (Mercedes-Benz Citation2021).
Abbink further suggests that future driving should be like riding a horse, i.e. instead of removing the human from the loop, he wants to bring an animal into the loop. The horse metaphor, which was proposed by Flemisch et al. (Citation2003) and which gained broader popularity through Norman’s (Citation2007) book The Design of Future Things provides no suggestions about how the horse metaphor should be accomplished. It should also be noted that horse riding is difficult and dangerous, or as noted by Norman: ‘smooth, graceful interaction between horse and rider requires considerable skill’ (25). In Camargo et al. (Citation2018), various statistics are reported that indicate that horse riding has a high likelihood of injury and mortality, possibly the highest of all recreational activities. This is surely not what the authors of the horse metaphor had in mind!
Some of my arguments below resemble those from Sheridan’s (Citation1995) paper: Human Centred Automation: Oxymoron or Common Sense? In this work, Sheridan argued that keeping the human in the loop is not necessarily a good idea, as humans may provide slow and unreliable input. It is exactly for this reason that operators of high-speed trains and nuclear power plants are excluded from the control loop in critical situations, ‘hands-off’ (Sheridan Citation1995, 824; see also Carvalho et al. Citation2008). Sheridan also hinted that human-centred automation is an oxymoron: ‘to the degree that a system is human-centred is it precisely to that degree NOT automatic?’ (823). Similarly, I indicate below that haptic shared control does not lead to a driver-automation partnership that is ‘greater than the sum of its parts’. Rather, it provides negligible benefits compared to manual driving while inheriting some of the same automation pitfalls that were discussed for traded control, and also giving rise to confusion and conflicts.
4.1. Shared control versus traded control in routine driving
The research so far on haptic shared control has been performed in car-following, lane-keeping, and other tracking tasks (see Xing et al. Citation2020, for a review), where it has revealed modest performance benefits. For example, a study in a driving simulator found that the mean absolute lateral position on a narrow road was 0.087 m for manual steering and 0.074 m for haptic shared control on the steering wheel (Melman et al. Citation2017). That is, participants, on average, stayed closer to the lane centre, indicating better driving performance. On a wider road, the mean absolute lateral position was found to be 0.23 m for manual steering versus 0.14 and 0.16 m for different types of haptic shared control (Petermeijer et al. Citation2015). Similarly, in car-following, Mulder et al. (Citation2008) found a standard deviation of time headway of 0.23 m for shared control on the accelerator pedal versus 0.20 m for manual car-following. De Winter et al. (Citation2008), on the other hand, reported slightly poorer car-following performance for haptic shared control (standard deviations of time headway of 0.49–0.55 s) than for manual car-following (0.48 s).
Although modest performance improvements can be expected, haptic shared control comes at a price. First, the evidence so far indicates that haptic shared control suffers from behavioural adaptation (Pitfall 4). A simulator study found that drivers, on average, drove faster (113.3 km/h) with shared steering control than without (105.7 km/h) (Melman et al. Citation2017). That same study also found that behavioural adaptation could be prevented by gradually decreasing the feedback torques when the vehicle was driving at a speed greater than a threshold speed. However, if behavioural adaptation (Pitfall 4) is indeed deemed to be a concern, then traded control in the form of intelligent speed adaptation (ISA) (Carsten and Tate Citation2005) could be enforced to make speeding impossible in the first place.
Furthermore, although deskilling is a known pitfall of traded control (Pitfall 2), haptic shared control may also result in deskilling. In the field of motor learning, there is a rich empirical base on the guidance hypothesis, summarised by Marchal-Crespo et al. (Citation2010): ‘A number of studies have confirmed this hypothesis finding that physically guiding movements does not aid in motor learning and may in fact hamper it’ (205). A proponent of haptic shared control could argue that deskilling in haptic shared control is less serious than the deskilling that occurs with traded control. However, this statement is entirely speculative. In fact, the long-term effects of driving with haptic shared control may well be worse compared to traded control, because the driver makes transitions between manual driving and a car with varying force-feel characteristics.
Another concern is that of conflicts. The latest-technology vehicles on the market can perform automated lane changes (Lambert Citation2022), but how lane changes would work with haptic shared control is rather contentious. Suppose the algorithm plans to overtake another vehicle but the driver intends to stay in the lane, or conversely, the driver wants to change lanes while the algorithm thinks that staying within the lane is more appropriate. This scenario will lead to counter-torques on the wheel and possible annoyance (Pitfall 6). One may argue that conflicts can be prevented through clever driver modelling, such as an intent-inference module that predicts what action the human driver wants to take (Benloucif et al. Citation2019; Doshi and Trivedi Citation2011). However, the prediction of human intentions can never be perfect, which means that occasional conflicts are inevitable. An early study already recognised that ‘the counterforce on the gas pedal … was assessed by drivers as uncomfortable’ (Van der Hulst et al. Citation1996, 179). More modern research on drivers’ trust in warning and automation systems of commercially available cars found that lane-keeping assist (which applies torque to the steering wheel, equivalent to haptic shared control) produced relatively low trust ratings, with discomfort being one of the explanations (Kidd et al. Citation2017). It goes almost without saying that if drivers disengage the shared-control system (Pitfall 6), they cannot reap any benefit from automation intelligence, and the driving task becomes equivalent to manual driving. Traded-control automation, such as ACC, as well as warning systems generally, received higher trust ratings than lane-keeping assist (Kidd et al. Citation2017).
In summary, haptic shared control suffers from automation pitfalls and yields only modest performance improvements compared to manual driving. Ironically, these performance improvements have been found in tasks for which automation is known to surpass humans. ACC, for example, can keep a near-constant headway, whereas automated lane-keeping ensures that the car stays neatly in the centre of the lane (, left panel; also note that , left panel, demonstrates that high guidance, i.e. hardly overridable haptic shared control outperforms human drivers). If performance improvements in lane-keeping and car-following were truly the goal, there would be no need to close the control loop through a human.
Figure 7. Example of a benefit of traded control in highway driving (left panel) and in emergency situations (right panel). The left panel shows vehicle test data that illustrates that an automated controller drives more accurately than a human driver (Tan et al. Citation1998). Lane-keeping was achieved via magnetic markers buried along the road centre; modern vehicles may use cameras to detect the lane. The right panel shows results from Bhardwaj, Lu, et al. (Citation2020) on the use of traded control versus haptic shared control in emergency steering, illustrating “a trade-off in automation design for emergency situations: high impedance automation can significantly reduce unwarranted driver input on the steering wheel during emergency situations but may cause driver discomfort and may be too strong to override during automation faults” (p. 1744). Note that traded control decoupled the steering wheel. Adversarial automation steered the vehicle into oncoming traffic.
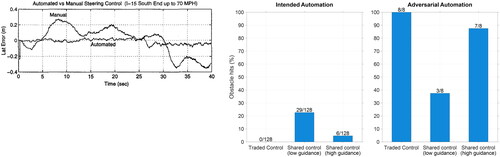
4.2. Shared control versus traded control in non-routine driving and accident scenarios
It was shown above that haptic shared control offers no meaningful benefits compared to automation when there is no need to take over control. What then are the advantages of haptic shared control? The proclaimed advantages are that haptic shared control helps prevent loss of situation awareness and overreliance (Pitfalls 1 & 5) and therefore prevents crashes.
First, it should be noted that haptic shared control may well cause a loss of mode awareness (Pitfall 1). The haptic forces may at times be below perceptual thresholds, for example, when the driver follows the target path closely, when having a low grip on the steering wheel, or when releasing the accelerator pedal, such as during coasting to a traffic light. Accordingly, the driver cannot just rely on the perceived forces to determine whether the system is engaged, risking mode confusion. Current haptic shared control systems, such as Volvo’s Pilot Assist, require status lights as well as warnings to ensure that the driver is keeping the hands on the steering wheel, which contributes to increased workload relative to manual driving (Solís-Marcos et al. Citation2018). Thus, haptic shared control currently on the market is not as continuous and reflexive as Abbink suggests it to be, but in fact prone to mode confusion (Pitfall 1) and workload issues (Pitfall 3).
It is true that loss of situation awareness (Pitfall 1) and overreliance (Pitfall 5) are critical pitfalls of traded-control automation: Fatal automation-related crashes with inattentive drivers have received extensive news coverage (e.g. Uber’s fatal crash with a pedestrian in Tempe, Arizona, in 2018), while within academia, there is a wealth of driving-simulator studies that have shown that some drivers are sometimes unable to reclaim control in time (De Winter et al. Citation2021; Zhang et al. Citation2019). Frequently cited is a study by Flemisch et al. (Citation2008), which showed that participants driving with haptic shared control kept their vehicle in the lane while all participants driving with automation drove their vehicle off the road after a simulated sensor failure just before a curve (as also shown in , right panel).
But how likely is it that drivers are misinformed about system limitations and unaware of their responsibilities, combined with a dangerous cocktail of a complete sensor outing at the most unfortunate moment that can be imaged (i.e. right before a curve, such as shown in , right panel)? These situations are rare, and if they occur, receive formal scrutiny (e.g. National Transportation Safety Board Citation2017, Citation2019a, Citation2019b, Citation2020). Not only automation-related crashes, but even automation disengagements are reported and analysed (Wang and Li Citation2019). This process resembles the Aviation Safety Reporting framework; that is, by collecting data on incidents, imperfections are engineered out of the system.
Furthermore, in the automation-related accidents that have occurred, these accidents were not just attributed to loss of situation awareness and overreliance (Pitfalls 1 & 5) but also to the behaviour of other road users (e.g. truck driver not giving right of way; National Transportation Safety Board Citation2017, Citation2019a) or poor road infrastructure (crash attenuator in a poor state; National Transportation Safety Board Citation2020). In summary, traded-control automation is not as dangerous as Abbink suggests it to be. Serious automation-driving-related accidents are rare, and in the fatal accidents that have occured, automation pitfalls were not the only cause.
Although it is undeniable that traded-control automation is one of the contributors to crashes, the exclusive focus on the dangers of automation is unproductive (De Winter Citation2019). The risks of traded-control automation should be compared with the number of lives saved relative to manual driving or haptic shared control. The power of traded-control automation was demonstrated by Bhardwaj, Lu, et al. (Citation2020) for AES. In their simulator study, the automated steering was programmed to always avoid a collision with a pedestrian. To test the impact of automation errors, it was also programmed to cause a collision with oncoming traffic, called adversarial automation, or to not respond at all, called idle automation. The experiment showed that haptic shared control caused a substantial number of collisions, even when the automation worked as intended, because it still required valid driver input. At the same time, haptic shared control also resulted in collisions when coupled to idle or adversarial automation, because it required drivers to steer (against the guidance) (see , right panel, for results for intended and adversarial automation). Granted, traded control resulted in more collisions during idle or adversarial automation, but in reality, how often would idle or adversarial automation happen compared to correct automation actions? Suppose that the automation algorithms function reliably in 99% of the emergency cases and adversarially in 1% of the emergency cases. Based on , the expected number of collisions for 1000 emergency steering cases would be 10, 55, and 228 for automation, high-guidance haptic shared control, and low-guidance haptic shared control, respectively. Even in an extreme case of automation that is only 75% reliable and crashes itself in 25% of the emergency cases, automation would still cause fewer crashes than haptic shared control. These numbers echo Sheridan’s (Citation1995) statements that human-centred automation is an oxymoron; keeping the human in the loop preserves, or even causes extra, human errorsFootnote5.
Abbink argued above that AES and AEB should be excluded from this debate because these systems surpass human ability and do not suffer from the pitfalls of automation. Abbink’s argument constitutes a debating fallacy known as No true Scotsman (Flew Citation1977, 47), that is, protecting one’s argument (shared control is better than traded control) from a counterexample (AEB is superior to shared control) by trying to remove the counterexample from the debate. Abbink’s attempt to exclude AEB from the debate is reminiscent of the AI effect (Kaplan and Haenlein Citation2020), which says that humans tend to discount automation progress post hoc. For example, chess was previously considered the epitome of intelligence, but after a computer first defeated the world champion, critics argued: ‘that’s just computation; it’s not true intelligence’. In the same way, traded-control automation continues to advance, and driving has become increasingly automated over the past half-century. Cruise control initially took over speed control, while full-range ACC is now able to bring the car to a full stop. More recent technology permits drivers to engage in non-driving tasks on designated highway sections (Mercedes-Benz Citation2021). Soon, more cars will be able to drive themselves on highways, including changing lanes, without needing driver input. Will Abbink continue to argue: ‘that’s just low-level control, not true traded-control automation’?
Worldwide, 1.35 million fatal accidents happen each year (World Health Organization Citation2019), primarily because of human errors in attention, perception, and control, as well as violations such as speeding and running red lights (Stanton and Salmon Citation2009)Footnote6. It is for good reason that regulatory bodies enforce traded-control automation, in particular AEB (European Commission Citation2020). Continuous non-emergency shared-control-type technologies, such as lane-keeping assist systems, are not among the evidence-based mandatory technologies, and why would they be? Haptic shared control hardly outperforms manual driving, as shown above.
Abbink also argues that while traded-control automation is successful in aviation, it should not be applied to driving. He points out that traded-control automation is suitable in aviation because the flight task is highly structured, with large time margins that allow trained pilots to resume control when needed. The actual reason the flight task is highly automated is necessity (it seems unsafe and highly impractical to let long-haul pilots fly manually along a precise flight path in a vast three-dimensional airspace) and economy (e.g. fuel efficiency gains can be achieved through automation). In driving, there are still major safety gains to be achieved through automation. In fact, driving is an excellent candidate for automation: the driving task is so dangerous, and causes of death are often so banal (e.g. distraction, running a red light) that it is surprising that manual driving is still allowed today.
In conclusion, shared control is an oxymoron. By keeping the human in the loop, one remains vulnerable to driver error and inherits some of the pitfalls of automation while also introducing nuisances in the form of conflicting torques on the steering wheel. Given the large number of human-induced traffic accidents, it seems a moral imperative to delegate control to automation where feasible. The pitfalls of automation should be studied with the help of vehicle data and remedied via technological advancements, just as these pitfalls are studied and remedied in aviation. Consequently, the operational design domain of traded-control automation keeps expanding, and road safety keeps improving. Although Bainbridge’s (Citation1983) Ironies of Automation suggest a doomsday scenario, the reality is that traded-control automation is already upon us.
5. Petermeijer: some nuance in the debate
Abbink and De Winter make strong cases for their respective solutions to the pitfalls of automation in the driving domain. Although both agree that a fully autonomous vehicle is a desirable end goal, they strongly disagree on the best approach for imperfect automation. Abbink advocates haptic shared control, arguing that this provides comfort benefits in routine driving situations while mitigating automation pitfalls when the automation capabilities are exceeded. De Winter counters that such an approach is an oxymoron and that shared control retains human error while still suffering from automation pitfalls. How do these two seemingly rational scientists come to such different conclusions, and who is right? In order to answer this question, we need to look at their arguments a little more closely.
5.1. Convenient blind spots in the argumentation
Abbink and De Winter overlook important aspects in the attempt to make their case. In particular, Abbink does not mention that imperfect automation (due to sensor and algorithm limitations) will result in nuisances not only for traded control but also for shared control. This was made clear during a recent study conducted on a test track in which shared and traded control were compared for the same underlying control algorithm (Petermeijer et al. Citation2022). In this study, the car was guided along a target path closely past a parked car. During traded control, the response of drivers of the automated vehicle was to take over, which turned off the automation and put them in manual control. In the haptic shared control condition, on the other hand, the automation could indeed be corrected without switching it off, but this did result in drivers experiencing counter-torques on the steering wheel. Both situations can be expected to be annoying and could result in disuse (Pitfall 6), although for the experimental conditions studied, the shared control solution was preferred by drivers over traded control.
Moreover, Abbink argued that drivers stay engaged in the driving task through the physical interaction of haptic shared control, mitigating the impact of Pitfalls 1 and 3. Although this is plausible, there is currently little evidence to support this claim. It is possible that prolonged use of a haptic shared control system results in a driver who is physically engaged (hands-on) but mentally distracted (mind-off).
De Winter presents the argument that nearly all accidents are due to human error. However, it is a fallacy to use this statistic to argue in favour of automation. By current law, drivers are responsible for the control of a vehicle, so as long as the vehicle operates as designed, it cannot be blamed for accidents. Moreover, reducing the argument of automated driving to mere statistics ignores any ethical or societal debate regarding automation-induced crashes. According to survey research, self-driving vehicles will only be tolerated if they are considerably safer than human-driven vehicles (Liu et al. Citation2019).
Abbink and De Winter both present valid points in favour of their arguments but can also be accused of cherry-picking certain results to support their case. I argue that it is not a question of which approach is better in general, but a question of when traded control and shared control can be used appropriately.
5.2. Towards a resolution
Abbink and De Winter make certain assumptions that provide indications on when trading or sharing should be used. For example, the assumptions of Abbink and De Winter about environmental complexity appear to be very different. De Winter appears to suggest that automation can function in most types of environments (or that it is only a matter of time until they do) and that automation drives in a way that is overall safer than manual driving. Abbink takes the stance that automation progress will be slow and, for the foreseeable future, will have a hard time dealing with situations that are moderately or highly complex. Abbink therefore believes that automation requires human correction in the form of haptic shared control.
If the environment is simple (or the automation is capable, i.e. accurate perception and prediction of the environment), then take-over requests can be provided early, i.e. with long time budgets. Early take-over studies (Damböck et al. Citation2012; Gold et al. Citation2013) already clarified that if time budgets are short, driver mistakes are common, which argues in favour of haptic shared control. Conversely, when time budgets are long (i.e. the driver has plenty of time to make a transition to manual), driver mistakes will be uncommon, which argues in favour of traded control. The difficulty, however, is that time budgets are not a priori known in scenarios such as urban driving. In urban driving, therefore, shared control, or traded control where the human keeps the hands near the steering wheel at all times, will be required.
It seems sensible to conclude that traded control is preferred in relatively structured environments and when proper safeguards are in place (e.g. the vehicle can bring itself into safety). In these conditions, it is unlikely that drivers will have to take control in safety-critical conditions, and the adverse consequences of the automation pitfalls will be small. Haptic shared control, on the other hand, is preferred when system limits are ill-defined and when the environment is more complex and dynamic. In these cases, the in-the-loop driver can act quickly upon automation failure.
These observations are in line with Parasuraman et al. (Citation2000), who suggested that ‘automation can be applied across a continuum of levels from low to high, i.e. from fully manual to fully automatic’ (286). These authors explained that higher levels of automation (cf. traded control instead of shared control) become justified (1) when ‘human performance consequences’ (i.e. the automation pitfalls) are expected to be small, (2) when automation is reliable, and (3) when the cost of decision outcomes is low. Parasuraman et al. further pointed to a relationship between automation reliability and human performance consequences, where, for example, complacency (Pitfall 5) is more likely when the automation is highly (but not perfectly) reliable. This echoes the ‘lumberjack hypothesis’ of Wickens et al. (Citation2020), which says that the better the automation performs in routine circumstances, the more severe the negative consequences when automation happens to fail or commit errors (‘the higher the trees are, the harder they fall’).
A representation of hypothesised efficacy of traded control, shared control, and manual control is shown in . It clarifies that if environmental complexity is moderate, shared control is more appropriate than traded control because drivers can correct automation errors as they occur. The range of conditions in which shared control can be safely used is wider than that of traded control, but in complex environments, such as in city centres with mixed traffic, even shared control will have to be disengaged.
Figure 8. Hypothesised performance level (i.e. accuracy in driving, safety) for traded, shared, and manual control, as a function of environmental complexity. Traded-control automation is known to outperform human drivers (i.e. manual control) but only in simple environments, such as highways (see also ). Humans are known to be able to drive in a wide range of environmental conditions but suffer from drowsiness in simple environments and high workload in complex environments such as dense city traffic, hence the inverted U-curve. Shared control helps generate higher performance than manual control in a wide range of environments; it provides benefits by supporting drivers in simple task environments () and allows drivers to benefit from automation in moderately complex environments. If the environment becomes complex and performance drops, drivers will have to make a transition from traded/shared control to manual control, which is costly.
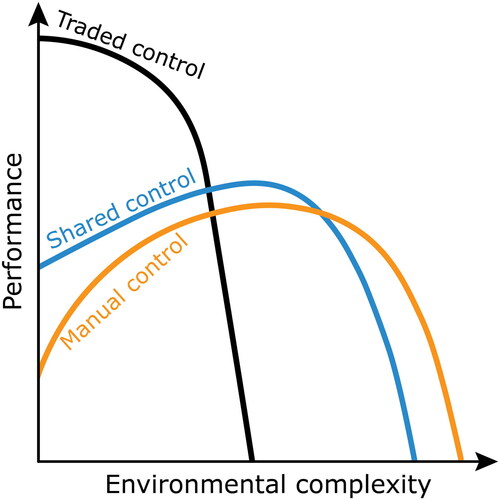
further illustrates that traded control is only feasible in relatively simple environments. If the environment becomes complex, the driver may have to suddenly reclaim control, which may be dangerous considering the above-mentioned pitfalls. The latest traded-control solutions implemented thus far, such as Mercedes-Benz’s Level-3 automation, for example, operates on specific motorway sections up to 60 km/h to ensure that the automation functions reliably and take-over requests can be provided 10 s in advance (Mercedes-Benz Citation2021). Similarly, the mandatory use of AEB can be explained because environmental complexity is low (i.e. it is relatively easy to establish that a crash is about to occur and that the best action is to brake strongly). Furthermore, the cost of acting is low as well (in fact, not braking autonomously guarantees an adverse outcome).
The illustration shown in is, of course, still hypothetical. The shapes of the curves depend on automation functionality (that may improve over time). It can be anticipated that, as technology progresses, automation will be able to perform an increasing number of subtasks, and the shape of the traded-control curve will advance to the right. Shared control will also benefit from technological progress, although when systems can safely hand over control (such as envisioned in SAE L4 automation), the need for shared control will become smaller (but note the above-mentioned lumberjack effect; Wickens et al. Citation2020).
Future research should empirically evaluate shared versus traded control using a wide range of measures, ranging from objective performance measures to more qualitative outcomes. Think-aloud methods, for example, may clarify why drivers disable the automation (Kolekar et al. Citation2021; Pitfall 6) and whether they develop a proper understanding of system functioning (Pitfall 1). Furthermore, it is recommended that any driver-automation interaction approach (sharing or trading) should not just be evaluated in environments of low complexity (where automation is expected to surpass humans) but also in complex environments where the performance envelope may be exceeded. A key aspect of honest evaluation is that the automation algorithms that underlie each interaction approach (i.e. trading and sharing) should be identical (see ). Furthermore, data from real-life driving is needed to move beyond current arguments that are mainly based on the results of simulator studies.
6. Conclusion
The question of sharing versus trading is a fundamental one, first brought up by Sheridan and Verplank (Citation1978). The current debate focussed on driving, a dynamic and safety-critical task in a relatively unstructured environment governed by formal and informal rules (Björklund and Åberg Citation2005). Consequently, driving can be seen as a task representative of a broader set of human-robot applications.
This article provided a series of arguments as to why shared control may be a powerful remedy to the pitfalls of automation. More specifically, shared control was argued to allow the driver to benefit from automation functionalities while remaining involved in the driving task. At the same time, this article provided a series of counterarguments, stating that drivers should not be kept in the loop because machines surpass humans in vital ways. Additionally, while tasks can be successfully shared between driver and automation, there is a risk of misalignment between driver and automation.
This work ended with the statement that it is a matter of environmental complexity. A thought experiment may prove the point: If the driving environment were very simple (or the automation perfectly reliable and capable), then the pitfalls of automation would not be an issue, and there would be no need for shared control. Conversely, if the complexity of the environment causes the automation to fail at unexpected moments, then the automation pitfalls are a concern and shared control is required to ensure that drivers complement the automation.
There are still several unresolved issues that need to be addressed in future research. In particular, there may be a qualitative or legal barrier when it comes to permitting the driver to perform non-driving tasks while driving, with some arguing that manufacturers should ‘skip Level 3’ (e.g. Ayre Citation2017; Weigl et al. Citation2021). Then again, Mercedes-Benz recently claimed to be the first company to have received certification for Level 3 automated driving (Mercedes-Benz Citation2021).
Some may argue that shared control and traded control are not mutually exclusive and should be combined. For example, haptic lane-keeping assistance (shared control) may be combined with automated evasive steering, thus benefiting from both philosophies. However, this type of unification may need further research regarding biomechanical safety and arbitration (i.e. the algorithmic negotiation of control actions of the driver and automation, see e.g. Marcano et al. Citation2020). Yet other types of philosophies to consider are maneuver-based control (Detjen et al. Citation2020; Hakuli et al. Citation2011) and envelope protection, as well as if/how the driver should be able to override such systems—topics currently of interest in aviation automation (e.g. Van Baelen et al. Citation2020). In an attempt to integrate the different control philosophies that may exist, Flemisch et al. (Citation2014) proposed a framework of ‘cooperative control’, which includes wireless communication, shared control, traded control (task delegation), and arbitration as core concepts (see Millot and Lemoine Citation1998, for a similar framework). Whether and how to integrate different control philosophies is a worthwhile topic of further research. We hope this article provides a way forward for researchers and practitioners to critically reflect on the arguments for and against sharing and trading control for the automotive domain and between human and automation systems in general.
Disclosure statement
No potential conflict of interest was reported by the author(s).
Notes
1 In a preceding lecture, De Winter had explained the six pitfalls of automation. On the day of the debate, Abbink gave a 20-min presentation in which he explained that traditional (traded-control) automation is a problem in driving, and why haptic shared control is a suitable solution to the pitfalls of automation. This was followed by a 20-min presentation in which De Winter explained that traded control, not shared control, is the best solution. After a 15-min break, Abbink and De Winter engaged in a debate (25 min), which left the students in the room (who occasionally brought in arguments as well) somewhat surprised about the fact that two academics could disagree so much. Next, Petermeijer added nuance by means of his presentation (15 min), followed by closing remarks (5 min). The current paper is written using the same chronological order as the lectures, i.e. (1) review of pitfalls, followed by (2) Abbink’s perspective, followed by (3) De Winter’s perspective, and (4) a nuance and conclusion.
2 A mode error was also one of the causes of the Torrey Canyon oil spill in 1967. However, the term mode error did not seem to exist at the time; the incident was simply described as the master noticing that the lever was in the wrong position (e.g. Oudet Citation1970).
3 As with the term mode error, deskilling was recognised before the introduction of the term in the human factors literature. As early as 1951, Fitts anticipated that ‘tasks can be set up so that human operators eventually become deficient in certain important skills which are infrequently used. As an illustration, a pilot who relies too much on the auto-pilot may lose some of his skill in manual control, or one who routinely uses automatic landing equipment may lose his skill in making manual landings’ (10).
4 This phrase is used often; as early as 1954, it was written that ‘flying is made up of hours of boredom broken by occasional moments of stark terror’ (Buck, Citation1954, 4). Note that captain Buck cites incidences of engine failure and poor visibility, but the greatest source of danger he referred to was complacency, such as ‘not bothering to know procedures well enough’ (5), see also Pitfall 5. Hancock (Citation2021) proposed the more ludicrous ‘months of monotony and milliseconds of mayhem’ (63).
5 The results shown in Bhardwaj, Lu, et al. (Citation2020) concern a decoupled steering wheel. Another option is to use hands-on automated emergency steering, an approach that risks conflicting torques on the steering wheel; Bhardwaj, Ghasemi, et al. (Citation2020) reported that ‘participants were oftentimes unwilling to let go of the steering wheel and give away the driving authority to automation’ (157).
6 Some authors reject this way of looking at driver error by arguing that a systems approach is more appropriate (e.g. Read et al. Citation2021). However, as pointed out by Reason (Citation1999), an overly systemic perspective does not ffer explanatory, predictive, or remedial value. Further, note that some have claimed that driving is ‘remarkably safe’ (e.g. Owens et al. Citation1993, 367) and that ‘drivers, in general, do a reasonable job of controlling their vehicles’ (Hancock and Stackhouse Citation1992, 494) because the number of fatal accidents per kilometer driven or per interaction is very low. However, this argument is not sustainable when considering that the lifetime probability of dying in a car accident in the USA is 1 in 101 (National Safety Council Citation2020), i.e. a substantial portion of the population dies by driver aberrations (e.g. distractions, misperceptions, violations) that could have been prevented by automation.
References
- Abbink, D. A., T. Carlson, M. Mulder, J. C. F. de Winter, F. Aminravan, T. L. Gibo, and E. R. Boer. 2018. “A Topology of Shared Control Systems—Finding Common Ground in Diversity.” IEEE Transactions on Human-Machine Systems 48 (5): 509–525. doi:10.1109/THMS.2018.2791570
- Abbink, D. A., and M. Mulder. 2009. “Exploring the Dimensions of Haptic Feedback Support in Manual Control.” Journal of Computing and Information Science in Engineering 9 (1): 011006. doi:10.1115/1.3072902
- Abbink, D. A., M. Mulder, and E. R. Boer. 2012. “Haptic Shared Control: Smoothly Shifting Control Authority?” Cognition, Technology & Work 14 (1): 19–28. doi:10.1007/s10111-011-0192-5
- Adams, J. A. 1987. “Historical Review and Appraisal of Research on the Learning, Retention, and Transfer of Human Motor Skills.” Psychological Bulletin 101 (1): 41–74. doi:10.1037/0033-2909.101.1.41
- America’s Independent Electric Light and Power Companies. 1956. “Power Companies Build for Your New Electric Living.” LIFE Magazine 40: 8.
- Ammons, R. B., R. G. Farr, E. Bloch, E. Neumann, M. Dey, R. Marion, and C. H. Ammons. 1958. “Long-Term Retention of Perceptual-Motor Skills.” Journal of Experimental Psychology 55 (4): 318–328. doi:10.1037/h0041893
- Andre, A., and A. Degani. 1997. “Do You Know What Mode You’re in? An Analysis of Mode Error in Everyday Things.” In Human-Automation Interaction: Research & Practice, edited by M. Mouloua and J. M. Koonce, 19–28. Mahwah, NJ: Lawrence Erlbaum.
- Avanzini, G., and E. A. Minisci. 2011. “Evolutionary Design of a Full-Envelope Full-Authority Flight Control System for an Unstable High-Performance Aircraft.” Proceedings of the Institution of Mechanical Engineers, Part G: Journal of Aerospace Engineering 225 (10): 1065–1080. doi:10.1177/2F0954410011414469
- Ayre, J. 2017. “Ford: Skip level 3 autonomous cars — Even engineers supervising self-driving vehicle testing lose ‘situational awareness’.” https://cleantechnica.com/2017/02/20/ford-skip-level-3-autonomous-cars-even-engineers-supervising-self-driving-vehicle-testing-lose-situational-awareness/
- Bahner, J. E., A.-D. Hüper, and D. Manzey. 2008. “Misuse of Automated Decision Aids: Complacency, Automation Bias and the Impact of Training Experience.” International Journal of Human-Computer Studies 66 (9): 688–699. doi:10.1016/j.ijhcs.2008.06.001
- Bainbridge, L. 1983. “Ironies of Automation.” Automatica 19 (6): 775–779. doi:10.1016/0005-1098(83)90046-8
- Banks, V. A., A. Eriksson, J. O’Donoghue, and N. A. Stanton. 2018. “Is Partially Automated Driving a Bad Idea? Observations from an on-Road Study.” Applied Ergonomics 68: 138–145. doi:10.1016/j.apergo.2017.11.010
- Beckers, N., L. C. Siebert, M. Bruijnes, C. Jonker, and D. A. Abbink. 2022. “Drivers of Partially Automated Vehicles are Blamed for Crashes that they Cannot Reasonably Avoid.” Scientific Reports 12: 16193. doi:10.1038/s41598-022-19876-0
- Benloucif, A., A.-T. Nguyen, C. Sentouh, and J.-C. Popieul. 2019. “Cooperative Trajectory Planning for Haptic Shared Control Between Driver and Automation in Highway Driving.” IEEE Transactions on Industrial Electronics 66 (12): 9846–9857. doi:10.1109/TIE.2019.2893864
- Bhardwaj, A., A. H. Ghasemi, Y. Zheng, H. Febbo, P. Jayakumar, T. Ersal, J. L. Stein, and R. B. Gillespie. 2020. “Who’s the Boss? Arbitrating Control Authority Between a Human Driver and Automation System.” Transportation Research Part F: Traffic Psychology and Behaviour 68: 144–160. doi:10.1016/j.trf.2019.12.005
- Bhardwaj, A., Y. Lu, S. Pan, N. Sarter, and B. Gillespie. 2020. “The Effects of Driver Coupling and Automation Impedance on Emergency Steering Interventions.” Proceedings of the IEEE International Conference on Systems, Man, and Cybernetics, 1738–1744. doi:10.1109/SMC42975.2020.9282961
- Bhargava, A., M. Bester, and L. Bolton. 2021. “Employees’ Perceptions of the Implementation of Robotics, Artificial Intelligence, and Automation (RAIA) on Job Satisfaction, Job Security, and Employability.” Journal of Technology in Behavioral Science 6 (1): 106–113. doi:10.1007/s41347-020-00153-8
- Bibby, K. S., F. Margulies, J. E. Rijnsdorp, R. M. J. Withers, I. M. Makarov, and J. E. Rijnsdorp. 1975. “Man’s Role in Control Systems.” IFAC Proceedings Volumes 8 (1): 664–683. doi:10.1016/S1474-6670(17)67612-2
- Billings, C. E. 1991. Human-Centered Aircraft Automation: A Concept and Guidelines (NASA Technical Memorandum 103885). Moffet Field, CA: NASA Ames Research Center.
- Billings, C. E. 1997. Aviation Automation—the Search for a Human-Centered Approach. Mahwah, NJ: Lawrence Erlbaum Associates.
- Birkemeier, J. R. 1968. Inflight Maintenance of Crew Skills on Long-Duration Manned Missions (Report No. NASA-CR-97081). National Aeronautics and Space Administration.
- Björklund, G. M., and L. Åberg. 2005. “Driver Behaviour in Intersections: Formal and Informal Traffic Rules.” Transportation Research Part F: Traffic Psychology and Behaviour 8 (3): 239–253. doi:10.1016/j.trf.2005.04.006
- Bliss, J. P. 2003. “Investigation of Alarm-Related Accidents and Incidents in Aviation.” The International Journal of Aviation Psychology 13 (3): 249–268. doi:10.1207/S15327108IJAP1303_04
- BMW 2019. “Learn How to Utilize Active Cruise Control With Driver Attention Camera.” https://www.bmwofminnetonka.com/bmw-active-cruise-control-with-driver-attention-camera
- Boxer, H. E. C. 1948. “Navigation in Automatic Flight.” Journal of Navigation 1 (1): 87–88. doi:10.1017/S0373463300034615
- Brown, I. D., and E. C. Poulton. 1961. “Measuring the Spare ‘Mental Capacity’ of Car Drivers by a Subsidiary Task.” Ergonomics 4 (1): 35–40. doi:10.1080/00140136108930505
- Buck, R. N. 1954. “An Airline Pilot Looks at Safety.” Flying Safety 11: 2–5. https://www.safety.af.mil/Portals/71/documents/Magazines/FSM/1950s/195401%20-%20FlyingSafetyMagazine.pdf.
- Cabrall, C. D. D., N. M. Janssen, and J. C. F. de Winter. 2018. “Adaptive Automation: Automatically (Dis)Engaging Automation During Visually Distracted Driving.” PeerJ. Computer Science 4: e166. doi:10.7717/peerj-cs.166
- Cain, B. 2007. A Review of the Mental Workload Literature (Technical Report). Canada: Defence Research and Development Toronto.
- Calvert, S. C., D. D. Heikoop, G. Mecacci, and B. van Arem. 2020. “A Human Centric Framework for the Analysis of Automated Driving Systems Based on Meaningful Human Control.” Theoretical Issues in Ergonomics Science 21 (4): 478–506. doi:10.1080/1463922X.2019.1697390
- Camara, F., N. Bellotto, S. Cosar, F. Weber, D. Nathanael, M. Althoff, J. Wu, J. Ruenz, A. Dietrich, G. Markkula, A. Schieben, F. Tango, N. Merat, and C. Fox. 2021. “Pedestrian Models for Autonomous Driving Part II: High-Level Models of Human Behavior.” IEEE Transactions on Intelligent Transportation Systems 22 (9): 5453–5472. doi:10.1109/TITS.2020.3006767
- Camargo, F., W. R. Gombeski, Jr., P. Barger, C. Jehlik, H. Wiemers, J. Mead, and A. Lawyer. 2018. “Horse-Related Injuries: Causes, Preventability, and Where Educational Efforts Should Be Focused.” Cogent Food & Agriculture 4 (1): 1432168. doi:10.1080/23311932.2018.1432168
- Carsten, O. M. J., and F. N. Tate. 2005. “Intelligent Speed Adaptation: Accident Savings and Cost–Benefit Analysis.” Accident; Analysis and Prevention 37 (3): 407–416. doi:10.1016/j.aap.2004.02.007
- Carvalho, P. V. R., I. L. dos Santos, J. O. Gomes, M. R. S. Borges, and S. Guerlain. 2008. “Human Factors Approach for Evaluation and Redesign of Human–System Interfaces of a Nuclear Power Plant Simulator.” Displays 29 (3): 273–284. doi:10.1016/j.displa.2007.08.010
- Casner, S. M., R. W. Geven, M. P. Recker, and J. W. Schooler. 2014. “The Retention of Manual Flying Skills in the Automated Cockpit.” Human Factors 56 (8): 1506–1516. doi:10.1177/0018720814535628
- Cook, R. I., and D. D. Woods. 1996. “Adapting to New Technology in the Operating Room.” Human Factors 38 (4): 593–613. doi:10.1518/001872096778827224
- Crandall, J. W., N. Anderson, C. Ashcraft, J. Grosh, J. Henderson, J. McClellan, A. Neupane, and M. A. Goodrich. 2017. “Human-Swarm Interaction as Shared Control: Achieving Flexible Fault-Tolerant Systems.” In Engineering Psychology and Cognitive Ergonomics: Performance, Emotion and Situation Awareness, edited by D. Harris, 266–284. Cham: Springer. doi:10.1007/978-3-319-58472-0_21
- Crandall, J. W., and M. A. Goodrich. 2002. “Characterizing Efficiency of Human Robot Interaction: A Case Study of Shared-Control Teleoperation.” IEEE/RSJ International Conference on Intelligent Robots and Systems 2 : 1290–1295. doi:10.1109/IRDS.2002.1043932
- Cummings, M. L., A. Clare, and C. Hart. 2010. “The Role of Human-Automation Consensus in Multiple Unmanned Vehicle Scheduling.” Human Factors 52 (1): 17–27. doi:10.1177/2F0018720810368674
- Damböck, D., M. Farid, L. Tönert, and K. Bengler. 2012. “Übernahmezeiten beim hochautomatisierten Fahren.” Tagungsband der VDI-Tagung Fahrerassistenz in München, 15, 16ff. https://mediatum.ub.tum.de/doc/1142102/file.pdf
- Darvall, F. O. 1934. Popular Disturbances and Public Order in Regency England: Being an Account of the Luddite and Other Disorders in England During the Years 1811–1817 and of the Attitude and Activity of the Authorities. New York: Oxford University Press.
- Detjen, H., S. Geisler, and S. Schneegass. 2020. “Maneuver-Based Control Interventions During Automated Driving: Comparing Touch, Voice, and Mid-Air Gestures as Input Modalities.” Proceedings of the 2020 IEEE International Conference on Systems, Man, and Cybernetics, 3268–3274. doi:10.1109/SMC42975.2020.9283431
- De Winter, J. C. F. 2019. “Pitfalls of Automation: A Faulty Narrative? Commentary on Hancock (2019) Some Pitfalls in the Promises of Automated and Autonomous Vehicles.” Ergonomics 62 (4): 505–508. doi:10.1080/00140139.2019.1563334
- De Winter, J. C. F., and D. Dodou. 2011. “Preparing Drivers for Dangerous Situations: A Critical Reflection on Continuous Shared Control.” Proceedings IEEE International Conference on Systems Man and Cybernetics, 1050–1056. doi:10.1109/ICSMC.2011.6083813
- De Winter, J. C. F., Y. B. Eisma, C. D. D. Cabrall, P. A. Hancock, and N. A. Stanton. 2019. “Situation Awareness Based on Eye Movements in Relation to the Task Environment.” Cognition, Technology & Work 21 (1): 99–111. doi:10.1007/s10111-018-0527-6
- De Winter, J. C. F., R. Happee, M. H. Martens, and N. A. Stanton. 2014. “Effects of Adaptive Cruise Control and Highly Automated Driving on Workload and Situation Awareness: A Review of the Empirical Evidence.” Transportation Research Part F: Traffic Psychology and Behaviour 27: 196–217. doi:10.1016/j.trf.2014.06.016
- De Winter, J. C. F., M. Mulder, M. M. van Paassen, D. A. Abbink, and P. A. Wieringa. 2008. “A Two-Dimensional Weighting Function for a Driver Assistance System.” IEEE Transactions on Systems, Man, and Cybernetics. Part B, Cybernetics : A Publication of the IEEE Systems, Man, and Cybernetics Society 38 (1): 189–195. doi:10.1109/TSMCB.2007.908860
- De Winter, J. C. F., N. A. Stanton, and Y. B. Eisma. 2021. “Is the Take-over Paradigm a Mere Convenience?” Transportation Research Interdisciplinary Perspectives 10: 100370. doi:10.1016/j.trip.2021.100370
- Dönmez Özkan, Y., A. G. Mirnig, A. Meschtscherjakov, C. Demir, and M. Tscheligi. 2021. “Mode Awareness Interfaces in Automated Vehicles, Robotics, and Aviation: A Literature Review.” Proceedings of the 13th International Conference on Automotive User Interfaces and Interactive Vehicular Applications, 147–158. doi:10.1145/3409118.3475125
- Doshi, A., and M. M. Trivedi. 2011. “Tactical Driver Behavior Prediction and Intent Inference: A Review.” Proceedings of the 2011 14th International IEEE Conference on Intelligent Transportation Systems, 1892–1897. doi:10.1109/ITSC.2011.6083128
- Doyle, A. 2009. “AA09: Pilot Handling Skills Under Threat, Says Airbus.” https://www.flightglobal.com/news/articles/aa09-pilot-handling-skills-under-threat-says-airbus-331991
- Dragan, A. D., and S. S. Srinivasa. 2013. “A Policy-Blending Formalism for Shared Control.” The International Journal of Robotics Research 32 (7): 790–805. doi:10.1177/0278364913490324
- Durso, F. T., Hackworth, C. A., Truitt, T. R., Crutchfield, J., Nikolic, D. and Manning C. A. 1998. “Situation Awareness as a Predictor of Performance for en Route Air Traffic Controllers.” Air Traffic Control Quarterly 6 (1): 1–20. doi:10.2514/atcq.6.1.1
- Ebbatson, M., D. Harris, J. Huddlestone, and R. Sears. 2010. “The Relationship Between Manual Handling Performance and Recent Flying Experience in Air Transport Pilots.” Ergonomics 53 (2): 268–277. doi:10.1080/00140130903342349
- Elish, M. C. 2019. “Moral Crumple Zones: Cautionary Tales in Human-Robot Interaction.” Engaging Science, Technology, and Society 5: 40–60. doi:10.17351/ests2019.260
- Elvik, R. 2004. “To What Extent Can Theory Account for the Findings of Road Safety Evaluation Studies?” Accident; Analysis and Prevention 36 (5): 841–849. doi:10.1016/j.aap.2003.08.003
- Endsley, M. R. 1988. “Situation Awareness Global Assessment Technique (SAGAT).” Proceedings of the IEEE 1988 National Aerospace and Electronics Conference, 789–795. doi:10.1109/NAECON.1988.195097
- Endsley, M. R. 1995. “A Taxonomy of Situation Awareness Errors.” In Human Factors in Aviation Operations, edited by R. Fuller, N. Johnston, and N. McDonald, 287–292. Aldershot, England: Avebury Aviation, Ashgate Publishing Ltd.
- Endsley, M. R., and E. O. Kiris. 1995. “The Out-of-the-Loop Performance Problem and Level of Control in Automation.” Human Factors: The Journal of the Human Factors and Ergonomics Society 37 (2): 381–394. doi:10.1518/001872095779064555
- European Commission 2020. “Regulation (EU) 2019/2144. Type-Approval Requirements to Ensure the General Safety of Vehicles and the Protection of Vulnerable Road Users (Summary Regulation (EU) 2019/2144).” https://eur-lex.europa.eu/legal-content/EN/LSU/?uri=uriserv:OJ.L_.2019.325.01.0001.01.ENG
- Federal Aviation Administration 2017. Manual Flight Operations Proficiency (Safety Alert for Operators SAFO 17007). Washington, DC: Flight Standards Service.
- Federal Ministry of Transport and Digital Infrastructure. 2015. “Protective Braking for ACSF.” https://wiki.unece.org/download/attachments/27459841/ACSF-04-04%20%20%28D%29%20-%20ACSF-Protective-Braking.pdf?api=v2
- Feldhütter, A., C. Segler, and K. Bengler. 2018. “Does Shifting Between Conditionally and Partially Automated Driving Lead to a Loss of Mode Awareness?” In Advances in Human Aspects of Transportation. AHFE 2017, edited by N. Stanton, 730–741. Cham: Springer. doi:10.1007/978-3-319-60441-1_70
- Ferris, T., N. Sarter, and C. D. Wickens. 2010. “Cockpit Automation: Still Struggling to Catch Up….” In Human Factors in Aviation, edited by E. Salas, T. Allard, and D. Maurino, 479–503. New York: Elsevier.
- Fitts, P. M. (Ed.). 1951. Human Engineering for an Effective Air Navigation and Traffic Control System. Washington, DC: National Research Council.
- Fleishman, E. A., and J. F. Parker, Jr. 1962. “Factors in the Retention and Relearning of Perceptual-Motor Skill.” Journal of Experimental Psychology 64: 215–226. doi:10.1037/h0041220
- Flemisch, F., C. A. Adams, S. R. Conway, K. H. Goodrich, M. T. Palmer, and P. C. Schutte. 2003. The H-Metaphor as a Guideline for Vehicle Automation and Interaction (Technical Memorandum NASA/TM-2003-212672). Hampton, VA: National Aeronautics and Space Administration.
- Flemisch, F., J. Kelsch, C. Löper, A. Schieben, J. Schindler, and M. Heesen. 2008. “Cooperative Control and Active Interfaces for Vehicle Assistance and Automation.” Proceedings of the FISITA World Automotive Congress.
- Flemisch, F. O., K. Bengler, H. Bubb, H. Winner, and R. Bruder. 2014. “Towards Cooperative Guidance and Control of Highly Automated Vehicles: H-Mode and Conduct-by-Wire.” Ergonomics 57 (3): 343–360. doi:10.1080/00140139.2013.869355
- Flew, A. 1977. Thinking Straight. Buffalo: Prometheus Books.
- Fuller, R. 2005. “Towards a General Theory of Driver Behaviour.” Accident; Analysis and Prevention 37 (3): 461–472. doi:10.1016/j.aap.2004.11.003
- Gao, Y., J. Zhao, J. Yan, Y. Zhu, and H. Cai. 2005. “Multi-Robot Tele-Operation System Based on Multi-Agent Structure.” Proceedings of the IEEE International Conference Mechatronics and Automation, 1726–1730. doi:10.1109/ICMA.2005.1626819
- Gempler, K. S. 1999. Display of Predictor Reliability on a Cockpit Display of Traffic Information (Report No. FY99-178). University of Illinois at Alabama.
- Ghasemi, A. H., P. Jayakumar, and R. B. Gillespie. 2019. “Shared Control Architectures for Vehicle Steering.” Cognition, Technology & Work 21 (4): 699–709. doi:10.1007/s10111-019-00560-9
- Gold, C., D. Damböck, L. Lorenz, and K. Bengler. 2013. “Take Over!” How Long Does It Take to Get the Driver Back into the Loop?” Proceedings of the Human Factors and Ergonomics Society Annual Meeting 57 (1): 1938–1942. doi:10.1177/2F1541931213571433
- Griffiths, P. G., and R. B. Gillespie. 2005. “Sharing Control Between Humans and Automation Using Haptic Interface: Primary and Secondary Task Performance Benefits.” Human Factors 47 (3): 574–590. doi:10.1518/2F001872005774859944
- Hakuli, S., S. Geyer, H. Winner, and J. Henning. 2011. “Integrated Concept for Maneuver-Based Driver Assistance.” ATZ Worldwide eMagazine 113 (3): 40–42. doi:10.1365/s38311-011-0032-5
- Hancock, P. A. 1996. “Effects of Control Order, Augmented Feedback, Input Device and Practice on Tracking Performance and Perceived Workload.” Ergonomics 39 (9): 1146–1162. doi:10.1080/00140139608964535
- Hancock, P. A. 2021. “Months of Monotony – Moments of Mayhem: Planning for the Human Role in a Transitioning World of Work.” Theoretical Issues in Ergonomics Science 22 (1): 63–82. doi:10.1080/1463922X.2020.1753260
- Hancock, P. A., and S. Stackhouse. 1992. “Human Factors and Safety Issues in IVHS.”. Proceedings of the 25th ISATA Conference on Road Transport Informatics/Intelligent Vehicle Highway Systems, 489–496.
- Hanusch, M. 2017. Manual Flying Skills – Airline Procedures and Their Effect on Pilot Proficiency (MSc Final Project). City University of London.
- Hart, S. G., and L. E. Staveland. 1988. “Development of NASA-TLX (Task Load Index): Results of Empirical and Theoretical Research.” In Human Mental Workload, edited by P. A. Hancock and N. Meshkati, 139–183. Amsterdam: North Holland Press.
- Hart, S. G., and C. D. Wickens. 1990. “Workload Assessment and Prediction.” In Manprint, edited by H. R. Booher, 257–296. Dordrecht: Springer. doi:10.1007/978-94-009-0437-8_9
- Haslbeck, A., and H.-J. Hoermann. 2016. “Flying the Needles: Flight Deck Automation Erodes Fine-Motor Flying Skills Among Airline Pilots.” Human Factors 58 (4): 533–545. doi:10.1177/0018720816640394
- Hobsbawm, E. J. 1952. “The Machine Breakers.” Past and Present 1 (1): 57–70. https://www.jstor.org/stable/649989. doi:10.1093/past/1.1.57
- Hoedemaeker, M., and K. A. Brookhuis. 1998. “Behavioural Adaptation to Driving With an Adaptive Cruise Control (ACC).” Transportation Research Part F: Traffic Psychology and Behaviour 1 (2): 95–106. doi:10.1016/S1369-8478(98)00008-4
- Horswill, M. S., and F. P. McKenna. 2004. “Drivers’ Hazard Perception Ability: Situation Awareness on the Road.” In A Cognitive Approach to Situation Awareness, edited by S. Banbury and S. Tremblay, 155–175. Aldershot, UK: Ashgate.
- Howard, R. W. 1973. “Automatic Flight Controls in Fixed Wing Aircraft. The First 100 Years.” The Aeronautical Journal 77: 533–562. doi:10.1017/S0001924000041889
- Inagaki, T. 2003. “Adaptive Automation: Sharing and Trading of Control.” In Handbook of Cognitive Task Design, edited by E. Hollnagel, 221–245. Mahwah, NJ: Erlbaum.
- Jansen, R. J., A. M. Tinga, R. de Zwart, and S. T. van der Kint. 2022. “Devil in the Details: Systematic Review of TOR Signals in Automated Driving with a Generic Classification Framework.” Transportation Research Part F: Traffic Psychology and Behaviour 91: 274–328. doi:10.1016/j.trf.2022.10.009
- Kaber, D. B., and M. R. Endsley. 2004. “The Effects of Level of Automation and Adaptive Automation on Human Performance, Situation Awareness and Workload in a Dynamic Control Task.” Theoretical Issues in Ergonomics Science 5 (2): 113–153. doi:10.1080/1463922021000054335
- Kahneman, D., and J. Beatty. 1966. “Pupil Diameter and Load on Memory.” Science 154 (3756): 1583–1585. doi:10.1126/science.154.3756.1583
- Kaplan, A., and M. Haenlein. 2020. “Rulers of the World, Unite! The Challenges and Opportunities of Artificial Intelligence.” Business Horizons 63 (1): 37–50. doi:10.1016/j.bushor.2019.09.003
- Kidd, D. G., J. B. Cicchino, I. J. Reagan, and L. B. Kerfoot. 2017. “Driver Trust in Five Driver Assistance Technologies Following Real-World Use in Four Production Vehicles.” Traffic Injury Prevention 18 (sup1): S44–S50. doi:10.1080/15389588.2017.1297532
- Kim, H., M. Song, and Z. Doerzaph. 2022. “Is Driving Automation Used as Intended? Real-World Use of Partially Automated Driving Systems and Their Safety Consequences.” Transportation Research Record: Journal of the Transportation Research Board 2676 (1): 30–37. doi:10.1177/03611981211027150
- Kolekar, S., S. M. Petermeijer, E. Boer, J. C. F. de Winter, and D. A. Abbink. 2021. “A Risk Field-Based Metric Correlates with Driver’s Perceived Risk in Manual and Automated Driving: A Test-Track Study.” Transportation Research Part C: Emerging Technologies 133: 103428. doi:10.1016/j.trc.2021.103428
- Kramer, A. F. 1991. “Physiological Metrics of Mental Workload: A Review of Recent Progress.” In Multiple-Task Performance, edited by D. L. Damos, 279–328. London: Taylor & Francis. doi:10.1201/9781003069447-14
- Krebsbach, K. D., and D. J. Musliner. 1999. Adjustable Autonomy in Procedural Control for Refineries (Technical Report AAAI Spring Symposium No. SS-99-06). https://www.aaai.org/Papers/Symposia/Spring/1999/SS-99-06/SS99-06-015.pdf
- Lambert, F. 2022. “Tesla Releases New Full Self-Driving Beta Update, and It’s a Big One.” https://electrek.co/2022/05/19/tesla-full-self-driving-beta-update-v10-12
- Lee, J. D., and B. D. Seppelt. 2009. “Human Factors in Automation Design.” In Springer Handbook of Automation, edited by S. Nof, 417–436. Berlin, Heidelberg: Springer. doi:10.1007/978-3-540-78831-7_25
- Lin, A. Y., K. Kuehl, J. Schöning, and B. Hecht. 2017. “Understanding ‘Death by GPS’: A Systematic Study of Catastrophic Incidents Associated with Personal Navigation Technologies.” Proceedings of the 2017 CHI Conference on Human Factors in Computing Systems, 1154–1166. doi:10.1145/3025453.3025737
- Lin, P., G. Bekey, and K. Abney. 2008. Autonomous Military Robotics: Risk, Ethics, and Design. California Polytechnic State University San Luis Obispo.
- Liu, P., R. Yang, and Z. Xu. 2019. “How Safe is Safe Enough for Self‐Driving Vehicles?” Risk Analysis : An Official Publication of the Society for Risk Analysis 39 (2): 315–325. doi:10.1111/risa.13116
- Losey, D. P., C. G. McDonald, E. Battaglia, and M. K. O’Malley. 2018. “A Review of Intent Detection, Arbitration, and Communication Aspects of Shared Control for Physical Human–Robot Interaction.” Applied Mechanics Reviews 70 (1): 010804. doi:10.1115/1.4039145
- Lu, Z., R. Happee, C. D. D. Cabrall, M. Kyriakidis, and J. C. F. de Winter. 2016. “Human Factors of Transitions in Automated Driving: A General Framework and Literature Survey.” Transportation Research Part F: Traffic Psychology and Behaviour 43: 183–198. doi:10.1016/j.trf.2016.10.007
- Marcano, M., S. Díaz, J. Pérez, and E. Irigoyen. 2020. “A Review of Shared Control for Automated Vehicles: Theory and Applications.” IEEE Transactions on Human-Machine Systems 50 (6): 475–491. doi:10.1109/THMS.2020.3017748
- Marchal-Crespo, L., S. McHughen, S. C. Cramer, and D. J. Reinkensmeyer. 2010. “The Effect of Haptic Guidance, Aging, and Initial Skill Level on Motor Learning of a Steering Task.” Experimental Brain Research 201 (2): 209–220. doi:10.1007/s00221-009-2026-8
- Marquart, G., C. Cabrall, and J. de Winter. 2015. “Review of Eye-Related Measures of Drivers’ Mental Workload.” Procedia Manufacturing 3: 2854–2861. doi:10.1016/j.promfg.2015.07.783
- Mars, F., M. Deroo, and J.-M. Hoc. 2014. “Analysis of Human-Machine Cooperation When Driving with Different Degrees of Haptic Shared Control.” IEEE Transactions on Haptics 7 (3): 324–333. doi:10.1109/TOH.2013.2295095
- McClure, P. K. 2018. “You’re Fired,” Says the Robot: The Rise of Automation in the Workplace, Technophobes, and Fears of Unemployment.” Social Science Computer Review 36 (2): 139–156. doi:10.1177/0894439317698637
- McCourt, M. J., S. S. Mehta, E. A. Doucette, and J. W. Curtis. 2016. “Human-Machine Teaming for Effective Estimation and Path Planning.” In Micro-and Nanotechnology Sensors, Systems, and Applications VIII, Vol. 9836, 98361 W. doi:10.1117/12.2224121
- Melman, T., D. A. Abbink, M. M. van Paassen, E. R. Boer, and J. C. F. de Winter. 2018. “What Determines Drivers’ Speed? A Replication of Three Behavioural Adaptation Experiments in a Single Driving Simulator Study.” Ergonomics 61 (7): 966–987. doi:10.1080/00140139.2018.1426790
- Melman, T., J. C. F. de Winter, and D. A. Abbink. 2017. “Does Haptic Steering Guidance Instigate Speeding? A Driving Simulator Study into Causes and Remedies.” Accident, Analysis and Prevention 98: 372–387. doi:10.1016/j.aap.2016.10.016
- Mercedes-Benz 2021. “Easy Tech: Conditionally Automated Driving With the DRIVE PILOT.” https://group.mercedes-benz.com/magazine/technology-innovation/easy-tech-drive-pilot.html
- Merlo, J. L., C. D. Wickens, and M. Yeh. 1999. Effect of Reliability on Cue Effectiveness and Display Signaling (Technical Report No. ARL-99-4/FED-LAB-99-3). Savoy: University of Illinois, Institute of Aviation, Aviation Research Lab.
- Metcalfe, J. S., J. Alban, K. Cosenzo, T. Johnson, and E. Capstick. 2010. “Field Testing of Tele-Operation versus Shared and Traded Control for Military Assets: An Evaluation Involving Real-Time Embedded Simulation and Soldier Assessment.” Unmanned Systems Technology XII 7692: 769206. doi:10.1117/12.850597
- Metz, C. 2021. “The Costly Pursuit of Self-Driving Cars Continues on. And on. And on.” The New York Times. https://www.nytimes.com/2021/05/24/technology/self-driving-cars-wait.html
- Millard-Ball, A. 2018. “Pedestrians, Autonomous Vehicles, and Cities.” Journal of Planning Education and Research 38 (1): 6–12. doi:10.1177/2F0739456X16675674
- Millot, P., and M. P. Lemoine. 1998. “An Attempt for Generic Concepts Toward Human-Machine Cooperation.” Proceedings of the IEEE International Conference on Systems, Man, and Cybernetics, 1044–1049. doi:10.1109/ICSMC.1998.725555
- Muir, B. M., and N. Moray. 1996. “Trust in Automation. Part II. Experimental Studies of Trust and Human Intervention in a Process Control Simulation.” Ergonomics 39 (3): 429–460. doi:10.1080/00140139608964474
- Mulder, M., D. A. Abbink, and E. R. Boer. 2012. “Sharing Control With Haptics: Seamless Driver Support from Manual to Automatic Control.” Human Factors 54 (5): 786–798. doi:10.1177/0018720812443984
- Mulder, M., M. Mulder, M. M. van Paassen, and D. A. Abbink. 2008. “Haptic Gas Pedal Feedback.” Ergonomics 51 (11): 1710–1720. doi:10.1080/00140130802331583
- Musić, S., and S. Hirche. 2017. “Control Sharing in Human-Robot Team Interaction.” Annual Reviews in Control 44: 342–354. doi:10.1016/j.arcontrol.2017.09.017
- National Highway Traffic Safety Administration. 2022a. Summary Report: Standing General Order on Crash Reporting for Level 2 Advanced Driver Assistance Systems (Report No. DOT HS 813 325). https://www.nhtsa.gov/sites/nhtsa.gov/files/2022-06/ADAS-L2-SGO-Report-June-2022.pdf
- National Highway Traffic Safety Administration 2022b. Occupant Protection for Vehicles With Automated Driving Systems (Docket No. NHTSA-2021-0003, RIN 2127-AM06). https://www.nhtsa.gov/sites/nhtsa.gov/files/2022-03/Final-Rule-Occupant-Protection-Amendment-Automated-Vehicles.pdf
- National Safety Council 2020. “Injury facts. Odds of dying.” https://injuryfacts.nsc.org/all-injuries/preventable-death-overview/odds-of-dying
- National Transportation Safety Board. 2014. Allision of the Passenger Vessel Seastreak Wall Street with Pier 11, Lower Manhattan, New York, New York, January 9, 2013 (Marine Accident Report No. NTSB/MAR-14/01). Washington, DC: National Transportation Safety Board. https://www.ntsb.gov/investigations/AccidentReports/Reports/MAR1401.pdf
- National Transportation Safety Board. 2017. Collision Between a Car Operating With Automated Vehicle Control Systems and a Tractor-Semitrailer Truck Near Williston, Florida, May 7, 2016 (Accident Report No. NTSB/HAR-17/02). Washington, DC: National Transportation Safety Board. https://www.ntsb.gov/investigations/accidentreports/reports/har1702.pdf
- National Transportation Safety Board. 2019a. Collision between car operating with partial driving automation and truck-tractor semitrailer. Delray Beach, Florida, March 1, 2019 (Highway Accident Brief). Washington, DC: National Transportation Safety Board. https://www.ntsb.gov/investigations/AccidentReports/Reports/HAB2001.pdf
- National Transportation Safety Board 2019b. Collision between vehicle controlled by developmental automated driving system and pedestrian. Tempe, Arizona, March 18, 2018 (Accident Report No. NTSB/HAR-19/03). Washington, DC: National Transportation Safety Board. https://www.ntsb.gov/investigations/accidentreports/reports/har1903.pdf
- National Transportation Safety Board 2020. Collision between a sport utility vehicle operating with partial driving automation and a crash attenuator. Mountain View, California, March 23, 2018 (Accident Report No. NTSB/HAR-20/01). Washington, DC: National Transportation Safety Board. https://www.ntsb.gov/investigations/AccidentReports/Reports/HAR2001.pdf
- Nordhoff, S., J. D. Lee, S. Calvert, S. Hegna Berge, M. Hagenzieker, and R. Happee. 2022. Testing Unfinished Automated Driving Technology on Public Roads: Results from Interviews With Participants of Tesla’s Full-Self Driving (FSD) Beta. Manuscript submitted for publication. Retrieved from https://www.researchgate.net/publication/364336031_Testing_unfinished_automated_driving_technology_on_public_roads_Results_from_interviews_with_participants_of_Tesla’s_Full-Self_Driving_FSD_Beta
- Norman, D. A. 1980. Errors in Human Performance (Report No. 8004). La Jolla, CA: Center for Human Information Processing, University of California.
- Norman, D. A. 2007. The Design of Future Things. New York, NY: Basic Books.
- Norman, D. A., and D. G. Bobrow. 1975. “On Data-Limited and Resource-Limited Processes.” Cognitive Psychology 7 (1): 44–64. doi:10.1016/0010-0285(75)90004-3
- Noy, I. Y., D. Shinar, and W. J. Horrey. 2018. “Automated Driving: Safety Blind Spots.” Safety Science 102: 68–78. doi:10.1016/j.ssci.2017.07.018
- Organisation for Economic Co-operation and Development. 1990. Behavioral Adaptation to Changes in the Road Transport System. Paris: Organisation for Economic Co-operation and Development Scientific Expert Group.
- Oudet, L. 1970. “The Torrey Canyon Commission of Inquiry.” The Journal of Navigation 23 (2): 238–250. doi:10.1017/S0373463300038443
- Owens, D. A., G. Helmers, and M. Sivak. 1993. “Intelligent Vehicle Highway Systems: A Call for User-Centred Design.” Ergonomics 36 (4): 363–369. doi:10.1080/00140139308967893
- Pacaux-Lemoine, M.-P, and M. Itoh. 2015. “Towards Vertical and Horizontal Extension of Shared Control Concept.” Proceedings of the 2015 IEEE International Conference on Systems, Man, and Cybernetics, 3086–3091. doi:10.1109/SMC.2015.536
- Parasuraman, R., T. Bahri, J. Deaton, J. Morrison, and M. Barnes. 1992. Theory and Design of Adaptive Automation in Aviation Systems (Progress Report No. NAWCADWAR-92033-60). Warminster, PA: Naval Air Warfare Center.
- Parasuraman, R., P. A. Hancock, and O. Olofinboba. 1997. “Alarm Effectiveness in Driver-Centred Collision-Warning Systems.” Ergonomics 40 (3): 390–399. doi:10.1080/001401397188224
- Parasuraman, R., and D. H. Manzey. 2010. “Complacency and Bias in Human Use of Automation: An Attentional Integration.” Human Factors 52 (3): 381–410. doi:10.1177/0018720810376055
- Parasuraman, R., and V. Riley. 1997. “Humans and Automation: Use, Misuse, Disuse, Abuse.” Human Factors: The Journal of the Human Factors and Ergonomics Society 39 (2): 230–253. doi:10.1518/001872097778543886
- Parasuraman, R., T. B. Sheridan, and C. D. Wickens. 2000. “A Model for Types and Levels of Human Interaction With Automation.” IEEE Transactions on Systems, Man, and Cybernetics. Part A, Systems and Humans : A Publication of the IEEE Systems, Man, and Cybernetics Society 30 (3): 286–297. doi:10.1109/3468.844354
- Penny, S. 2022. “Sensorimotor Debilities in Digital Cultures.” AI & SOCIETY 37 (1): 355–366. doi:10.1007/s00146-021-01186-0
- Petermeijer, S. M., D. A. Abbink, and J. C. F. de Winter. 2015. “Should Drivers Be Operating within an Automation-Free Bandwidth? Evaluating Haptic Steering Support Systems with Different Levels of Authority.” Human Factors 57 (1): 5–20. doi:10.1177/2F0018720814563602
- Petermeijer, S. M., T. Sakuma, S. B. Kolekar, J. C. F. de Winter, and D. A. Abbink. 2022. Resolving Driver-Assistance Disagreements: How LKAS Interaction Design and Trajectory-Matching Affect Driver Behaviour and Acceptance. Manuscript in preparation.
- Potter, S. S., R. I. Cook, D. D. Woods, and J. S. McDonald. 1990. “The Role of Human Factors Guidelines in Designing Usable Systems: A Case Study of Operating Room Equipment.” Proceedings of the Human Factors Society Annual Meeting 34 (4): 392–395. doi:10.1177/154193129003400434
- Radlmayr, J., V. Weinbeer, C. Löber, M. Farid, and K. Bengler. 2018. “How Automation Level and System Reliability Influence Driver Performance in a Cut-in Situation.” In Advances in Human Aspects of Transportation. AHFE 2017, edited by N. Stanton, 684–694. Cham: Springer. doi:10.1007/978-3-319-60441-1_66
- Rantanen, E. M., and J. H. Goldberg. 1999. “The Effect of Mental Workload on the Visual Field Size and Shape.” Ergonomics 42 (6): 816–834. doi:10.1080/001401399185315
- Read, G. J. M., S. Shorrock, G. H. Walker, and P. M. Salmon. 2021. “State of Science: Evolving Perspectives on ‘Human Error’.” Ergonomics 64 (9): 1091–1114. doi:10.1080/00140139.2021.1953615
- Reagan, I. J., J. B. Cicchino, L. B. Kerfoot, and R. A. Weast. 2018. “Crash Avoidance and Driver Assistance Technologies–Are They Used?” Transportation Research Part F: Traffic Psychology and Behaviour 52: 176–190. doi:10.1016/j.trf.2017.11.015
- Reason, J. 1997. “Hazards, Defences and Losses.” In Managing the Risks of Organizational Accidents, edited by J. Reason, 1–20. Aldershot, UK: Ashgate.
- Reason, J. 1999. “Are We Casting the Net Too Widely in Our Search for the Factors Contributing to Errors and Accidents?” In Nuclear Safety: A Human Factors Perspective, edited by J. Misumi, R. Mille, and B. Wilpert, 199–205. Boca Raton, FL: CRC Press.
- Rogovin, M., and G. T. Frampton, Jr. 1980. Three Mile Island. A Report to the Commissioners and to the Public. Volume I. Washington, DC: Nuclear Regulatory Commission, Special Inquiry Group. doi:10.2172/5395798
- Roscoe, A. H. 1992. “Assessing Pilot Workload. Why Measure Heart Rate, HRV and Respiration?” Biological Psychology 34 (2–3): 259–287. doi:10.1016/0301-0511(92)90018-P
- Ross, P. E. 2018. “Gill Pratt of Toyota: Safety Is no Argument for Robocars: The Technology That Will Eventually Make Self-Driving Cars Safe Can Be Implemented in Human-Driven Cars.” https://spectrum.ieee.org/gil-pratt-of-toyota-safety-is-no-argument-for-robocars
- Rudenko, A., L. Palmieri, M. Herman, K. M. Kitani, D. M. Gavrila, and K. O. Arras. 2020. “Human Motion Trajectory Prediction: A Survey.” The International Journal of Robotics Research 39 (8): 895–935. doi:10.1177/0278364920917446
- Saito, Y., H. Muslim, and M. Itoh. 2021. “Design of Haptic Protection With an Adaptive Level of Authority Based on Risk Indicators under Hands-on Partial Driving Automation.” IEEE International Conference on Systems, Man, and Cybernetics, 1613–1618. doi:10.1109/SMC52423.2021.9658933
- Saleh, K., M. Hossny, and S. Nahavandi. 2017. “Towards Trusted Autonomous Vehicles from Vulnerable Road Users Perspective.” Proceedings of the 2017 Annual IEEE International Systems Conference. doi:10.1109/SYSCON.2017.7934782
- Sarter, N. B., and D. D. Woods. 1995. “How in the World Did We Ever Get Into That Mode? Mode Error and Awareness in Supervisory Control.” Human Factors: The Journal of the Human Factors and Ergonomics Society 37 (1): 5–19. doi:10.1518/001872095779049516
- Schmidt, P. N. 1958. “Radar and Marine Collisions Today.” The Hastings Law Journal 10: 71–88. https://repository.uchastings.edu/hastings_law_journal/vol10/iss1/5.
- Scholtens, W., S. Barendswaard, D. Pool, R. van Paassen, and D. Abbink. 2018. “A New Haptic Shared Controller Reducing Steering Conflicts.” Proceedings of the 2018 IEEE International Conference on Systems, Man, and Cybernetics, 2705–2710. doi:10.1109/SMC.2018.00462
- Shang, C., H. Fang, J. Chen, and J. Zhang. 2017. “Interacting with Multi-Agent Systems through Intention Field Based Shared Control Methods.” Proceedings of the 2017 11th Asian Control Conference, 150–155. doi:10.1109/ASCC.2017.8287158
- Sheridan, T. B. 1991. “Automation, Authority and Angst—Revisited.” Proceedings of the Human Factors Society Annual Meeting 35 (1): 2–6. doi:10.1177/154193129103500103
- Sheridan, T. B. 1992. Telerobotics, Automation, and Human Supervisory Control. Cambridge, MA: MIT Press.
- Sheridan, T. B. 1995. “Human Centered Automation: oxymoron or Common Sense?.” Proceedings of the 1995 IEEE International Conference on Systems, Man and Cybernetics. Intelligent Systems for the 21st Century, 823–828. doi:10.1109/ICSMC.1995.537867
- Sheridan, T. B. 2011. “Adaptive Automation, Level of Automation, Allocation Authority, Supervisory Control, and Adaptive Control: Distinctions and Modes of Adaptation.” IEEE Transactions on Systems, Man, and Cybernetics - Part A: Systems and Humans 41 (4): 662–667. doi:10.1109/TSMCA.2010.2093888
- Sheridan, T. B., T. Vámos, and S. Aida. 1983. “Adapting Automation to Man, Culture and Society.” Automatica 19 (6): 605–612. doi:10.1016/0005-1098(83)90024-9
- Sheridan, T. B., and W. L. Verplank. 1978. Human and Computer Control of Undersea Teleoperators (Technical Report, Man-Machine Systems Laboratory, Department of Mechanical Engineering). Cambridge, MA: MIT.
- Siebert, L. C., M. L. Lupetti, E. Aizenberg, N. Beckers, A. Zgonnikov, H. Veluwenkamp, D. Abbink, E. Giaccardi, G.-J. Houben, C. M. Jonker, J. van den Hoven, D. Foster, and R. L. Lagendijk. 2021. “Meaningful Human Control Over AI Systems: Beyond Talking the Talk.” arXiv. https://arxiv.org/abs/2112.01298
- Silva, S. S., and R. J. Hansman. 2015. “Divergence Between Flight Crew Mental Model and Aircraft System State in Auto-Throttle Mode Confusion Accident and Incident Cases.” Journal of Cognitive Engineering and Decision Making 9 (4): 312–328. doi:10.1177/1555343415597344
- Skitka, L. J., K. L. Mosier, and M. Burdick. 1999. “Does Automation Bias Decision-Making?” International Journal of Human-Computer Studies 51 (5): 991–1006. doi:10.1006/ijhc.1999.0252
- Solís-Marcos, I., C. Ahlström, and K. Kircher. 2018. “Performance of an Additional Task During Level 2 Automated Driving: An on-Road Study Comparing Drivers with and without Experience With Partial Automation.” Human Factors 60 (6): 778–792. doi:10.1177/2F0018720818773636
- Sorkin, R. D. 1988. “Why Are People Turning off Our Alarms?” The Journal of the Acoustical Society of America 84 (3): 1107–1108. doi:10.1121/1.397232
- Stanton, N. A., and P. M. Salmon. 2009. “Human Error Taxonomies Applied to Driving: A Generic Driver Error Taxonomy and Its Implications for Intelligent Transport Systems.” Safety Science 47 (2): 227–237. doi:10.1016/j.ssci.2008.03.006
- Stein, C., A. Liu, D. Brown, and A. Porch. 2019. Monitoring Engineer Fatigue (MEFA) (Report No. DOT/FRA/ORD-19/48). Cambridge, MA: Aurora Flight Sciences.
- Stiegemeier, D., S. Bringeland, J. Kraus, and M. Baumann. 2022. “Do I Really Need It?”: An Explorative Study of Acceptance and Usage of in-Vehicle Technology.” Transportation Research Part F: Traffic Psychology and Behaviour 84: 65–82. doi:10.1016/j.trf.2021.11.011
- Storms, J. G., and D. M. Tilbury. 2014. “Blending of Human and Obstacle Avoidance Control for a High Speed Mobile Robot.” Proceedings of the 2014 American Control Conference, 3488–3493. doi:10.1109/ACC.2014.6859352
- Tabone, W., J. de Winter, C. Ackermann, J. Bärgman, M. Baumann, S. Deb, C. Emmenegger, A. Habibovic, M. Hagenzieker, P. A. Hancock, R. Happee, J. Krems, J. D. Lee, M. Martens, N. Merat, D. Norman, T. B. Sheridan, and N. A. Stanton. 2021. “Vulnerable Road Users and the Coming Wave of Automated Vehicles: Expert Perspectives.” Transportation Research Interdisciplinary Perspectives 9: 100293. doi:10.1016/j.trip.2020.100293
- Tan, H. S., R. Rajamani, and W. B. Zhang. 1998. “Demonstration of an Automated Highway Platoon System.” Proceedings of the 1998 American Control Conference, 1823–1827. doi:10.1109/ACC.1998.707332
- Tso, K. S., G. K. Tharp, W. Zhang, and A. T. Tai. 1999. “A Multi-Agent Operator Interface for Unmanned Aerial Vehicles.” Proceedings of the 18th Digital Avionics Systems Conference. doi:10.1109/DASC.1999.821969
- United Nations 2013. “Agreement Concerning the Adoption of Uniform Technical Prescriptions for Wheeled Vehicles, Equipment and Parts Which Can Be Fitted and/or Be Used on Wheeled Vehicles and the Conditions for Reciprocal Recognition of Approvals Granted on the Basis of These Prescriptions (Addendum: 130: Regulation: 131).” https://unece.org/fileadmin/DAM/trans/main/wp29/wp29regs/2013/R131e.pdf
- Van Baelen, D., J. Ellerbroek, M. M. van Paassen, and M. Mulder. 2020. “Design of a Haptic Feedback System for Flight Envelope Protection.” Journal of Guidance, Control, and Dynamics 43 (4): 700–714. doi:10.2514/1.G004596
- Van der Hulst, M., T. Rothengatter, and A. Heino. 1996. “Effectiveness of Distance-Keeping Support Systems.” Proceedings of the International Conference on Traffic and Transport Psychology, 179–184.
- Van Dintel, K. M., S. M. Petermeijer, E. J. H. de Vries, and D. A. Abbink. 2021. “SAE Level-3 automation-Comparison of Traded and Shared Control.” ATZ Worldwide 123 (5–6): 26–33. doi:10.1007/s38311-021-0667-9
- Vengattil, M. 2020. “Self-Driving Technology Companies Suspend Testing on Virus Fears.” https://www.reuters.com/article/us-health-coronavirus-waymo-idUSKBN2143MO
- Vlakveld, W. P. 2011. “Hazard Anticipation of Young Novice Drivers.” Doctoral Diss., University of Groningen.
- Wall, R. L., J. L. Tate, and M. J. Moss. 1980. Advanced Flight Deck/Crew Station Simulator Functional Requirements (NASA Contractor Report 159331). Hamton, VA: Langley Research Center, National Aeronautics and Space Administration.
- Wang, S., and Z. Li. 2019. “Exploring Causes and Effects of Automated Vehicle Disengagement Using Statistical Modeling and Classification Tree Based on Field Test Data.” Accident; Analysis and Prevention 129: 44–54. doi:10.1016/j.aap.2019.04.015
- Wang, W., X. Na, D. Cao, J. Gong, J. Xi, Y. Xing, and F.-Y. Wang. 2020. “Decision-Making in Driver-Automation Shared Control: A Review and Perspectives.” IEEE/CAA Journal of Automatica Sinica 7: 1–19. doi:10.1109/JAS.2020.1003294
- Weigl, K., C. Schartmüller, A. Riener, and M. Steinhauser. 2021. “Development of the Questionnaire on the Acceptance of Automated Driving (QAAD): Data-Driven Models for Level 3 and Level 5 Automated Driving.” Transportation Research Part F: Traffic Psychology and Behaviour 83: 42–59. doi:10.1016/j.trf.2021.09.011
- Wickens, C. D., L. Onnasch, A. Sebok, and D. Manzey. 2020. “Absence of DOA Effect but No Proper Test of the Lumberjack Effect: A Reply to Jamieson and Skraaning (2019).” Human Factors 62 (4): 530–534. doi:10.1177/0018720820901957
- Wiener, E. L. 1989. Human Factors of Advanced Technology (“Glass Cockpit”) Transport Aircraft (NASA Tech. Report 117528). Springfield, VA: National Technical Information Service.
- Wiener, E. L., and R. E. Curry. 1980. “Flight-Deck Automation: Promises and Problems.” Ergonomics 23 (10): 995–1011. doi:10.1080/00140138008924809
- Wilde, G. J. S. 1982. “The Theory of Risk Homeostasis: Implications for Safety and Health.” Risk Analysis 2 (4): 209–225. doi:10.1111/j.1539-6924.1982.tb01384.x
- Wilde, G. J. S. 2013. “Homeostasis Drives Behavioural Adaptation.” In Behavioural Adaptation and Road Safety: Theory, Evidence and Action, edited by C. M. Rudin-Brown and S. L. Jamson, 61–86. Boca Raton, FL: CRC Press.
- Wilde, G. J. S., L. S. Robertson, and I. B. Pless. 2002. “Does Risk Homoeostasis Theory Have Implications for Road Safety.” BMJ 324 (7346): 1149–1152. doi:10.1136/bmj.324.7346.1149
- Williges, R. C., and W. W. Wierwille. 1979. “Behavioral Measures of Aircrew Mental Workload.” Human Factors: The Journal of the Human Factors and Ergonomics Society 21 (5): 549–574. doi:10.1177/001872087902100503
- Wilson, K. M., S. Yang, T. Roady, J. Kuo, and M. G. Lenné. 2020. “Driver Trust & Mode Confusion in an On-Road Study of Level-2 Automated Vehicle Technology.” Safety Science 130: 104845. doi:10.1016/j.ssci.2020.104845
- Wood, S. 2004. Flight Crew Reliance on Automation (CAA Paper 2004/10). Gatwick Airport South, West Sussex, UK: Civil Aviation Authority, Safety Regulation Group.
- World Health Organization 2019. “Global Status Report on Road Safety 2018.” https://www.who.int/publications/i/item/9789241565684
- Xing, Y., C. Huang, and C. Lv. 2020. “Driver-Automation Collaboration for Automated Vehicles: A Review of Human-Centered Shared Control.” Proceedings of the 2020 IEEE Intelligent Vehicles Symposium, 1964–1971. doi:10.1109/IV47402.2020.9304755
- Zhang, B., J. C. F. de Winter, S. Varotto, R. Happee, and M. Martens. 2019. “Determinants of Take-Over Time from Automated Driving: A Meta-Analysis of 129 Studies.” Transportation Research Part F: Traffic Psychology and Behaviour 64: 285–307. doi:10.1016/j.trf.2019.04.020