Abstract
In trauma teams, coordination can be established through a centralised leader. The team can also use a decentralised strategy. In this descriptive study of video-recorded trauma resuscitations, using quantification of qualitative data, Social Network analysis of all real-time communications of eight in-real-life (IRL) and simulated trauma teams explained team social structure. The communication network structures in the simulated scenarios were more centralised using individually directed speech and had a high proportion of communication to update all team members. Such a structure might be the result of work performed in a complexity-stripped simulation environment where simplified task-executions required less interactions, or from work revolving around a deteriorating patient, imposing high demands on rapid decision-making and taskwork. Communication IRL was mostly decentralised, with more variability between cases, possibly due to unpredictability of the IRL case. The flexibility to act in a decentralised manner potentiates adaptability and seems beneficial in rapidly changing situations.
Practitioner summary: Efficient collaboration in trauma teams is essential. Communication in in-real-life and simulated trauma teams was analysed using social network analysis. The simulation teams were overall more centralised compared to the IRL teams. The flexibility to act decentralised seems beneficial for emergency teams as it enables adaptability in unpredictable situations.
1. Introduction
Trauma resuscitation of an injured patient is a challenging and potentially escalating emergency, with high demands on team coordination for the quality and effectiveness of care (Georgiou and Lockey Citation2010). The interprofessional and interdisciplinary trauma team that assembles ad hoc is standardised according to trauma manuals (Tiel Groenestege-Kreb, van Maarseveen, and Leenen Citation2014), and thus contains role-specific competencies. The taskwork is structured according to the Advanced Trauma Life Support (ATLS Subcommittee Citation2013) principles. There has been much work undertaken to optimise the trauma team in terms of roles, task, and function delegation (Georgiou and Lockey Citation2010), which are some elements of the team structure (Lafond et al. Citation2011).
A functional team structure means that individuals are both specialised and interdependent, there is one appointed leader to coordinate the team’s actions, and there is some decisional authority differentiation in the team (Lafond et al. Citation2011; Hollenbeck et al. Citation2002). The advantages of functionally structured teams are that individuals are trained to perform their respective tasks, which allows for the honing of task execution skills and creates a predictable work environment. This makes it possible to streamline the work process on behalf of distribution of the operative tasks beforehand. But since the functionally structured team is dependent on coordination, information must be channelled through a central leader. The drawback hence of a functional team structure is that it has a potential to create a ‘bottle neck’, where information is not distributed in accordance with the needs of the team (Samarth and Gloor Citation2009).
A divisional team structure is characterised by overarching competencies but a priori divided responsibilities in the team. Theoretically, a divisionally structured team is less reliant on communication channelled through a central leader, and more communication is individually directed between members of the team than in a functionally structured team. A divisional structure is advantageous when complexity increases, and the situations are unpredictable and dynamic. This is because interaction including communication tends to be difficult under such contingencies, and the divisionally structured teams are less dependent on effective coordination than are the functionally structured teams (Lafond et al. Citation2011). The drawbacks of divisionally structured teams are that individuals need to be multi-skilled and somewhat autonomous, and the lack of central control might degrade situation awareness of the whole mission for some team members, however, it might increase situation awareness around a particular area of operation (Gao, Cummings, and Solovey Citation2016; Diedrich et al. Citation2002).
The differences in main team structure characteristics of functional and divisional team structures are displayed in .
Table 1. Characteristics of functional and divisional team structures.
Emergency care teams often accommodate multi-skilled professionals, allowing for more flexibility regarding division of labour (Wise et al. Citation2020). However, Trauma teams are organised to represent a functional team structure. The trauma team has an appointed leader with decisional authority and the workflow is controlled through a standardised examination protocol, which necessitates some level of central control (Courtenay, Nancarrow, and Dawson Citation2013). In a highly specialised team, specific dyads or triads of individuals might however share certain information regarding, for instance, anaesthesia, whereas over-arching goals, priorities, and predicted threats, etc. are updated and shared in the whole team and represent a common team mental model (Fernandez et al. Citation2017).
The expected structure and the nature of the team’s operations imply that coordination and communication are paramount for the maintenance of shared goals and task accomplishment (Johnsen et al. Citation2017; Manser Citation2009; Westli et al. Citation2010).
The use of implicit behaviours, and communication techniques addressing the whole team, pushes information and status updates out to the whole team. In the literature they are distinguished as information sharing [talking to the room (‘TTR’)], commenting real-time observations (‘online commentaries’), and raising concerns about potential threats or conflicting information (‘speaking up’). However, all these types of communication directed to the whole team are correlated with good performance in emergency teams and are believed to update and rectify a common mental model (Burtscher, Ritz, and Kolbe Citation2018; Kolbe et al. Citation2014), induce implicit desirable behaviour (Gundrosen et al. Citation2016) and mitigate erroneous decisions (Beament and Mercer Citation2016), respectively.
Studies comparing team structure and communication flow in emergency teams in healthcare are scarce. Team process theories and models from experimental studies in other disciplines could inform healthcare team science (Dinh et al. Citation2020), but the extrapolation of results from other domains ignores the relevance of context. Such studies often use teams with only a few interacting members (Lafond et al. Citation2011; Schraagen, Veld, and De Koning Citation2010) whereas resuscitation effectiveness in trauma teams seems to benefit from increasing the number of participants even though demands on communication and coordination will be higher (Maluso et al. Citation2016). Further, the temporal demands might differ and observational studies on trauma teams from the in-real-life (IRL) setting have found that a communication collapse may occur when challenged by task overload and escalation (Xiao, Mackkenzie, and Patchy Citation1998).
Although the trauma team is highly structured as a functional team and central coordination is deemed essential for its performance, such coordination might be demanding and difficult to uphold under certain conditions. Errors coupled with situation awareness and decision making have been found the most frequent during primary survey of trauma resuscitation, and although algorithms and protocols have improved trauma care, divergence from them might be appropriate in some circumstances (Fornander et al. Citation2023; Madani et al. Citation2018). It is possible that trauma teams can adopt a divisional structure, that is a less centralised network enforced by the situation.
Social Network Analysis (SNA) provides a method by which to quantify patterned interactions between actors and compare aspects of team social relations and team dynamics (Wellman and Berkowitz Citation1988). It can be used to explore the communication flow within a team.
In SNA terms, centralisation regards the concentration of influence and power in a network (Tröster, Mehra, and van Knippenberg Citation2014). A central node of information is more effective when dealing with easier tasks, but high performance in complex tasks is linked to lower degrees of centralisation or a decentralised network (Brown and Miller Citation2000; Shaw Citation1964; Park et al. Citation2020). The latter thus give rise to high degrees of information sharing (Bavelas Citation1948; Houghton et al. Citation2006; Leavitt Citation1951; Shaw Citation1964) ().
A functional team structure is expected to use a centralised communication network, whereas a divisional structure would use a decentralised communication network.
In earlier SNA studies on communication in small working teams, data are mostly collected through surveys (Benton et al. Citation2015; Han et al. Citation2022; Henttonen Citation2010), which creates a potential for recall bias. However, the use of real-time communications makes it possible to assess not only the preferred or self-assessed links that exist between team members but also the magnitude of interaction links created. This is especially interesting in trauma teams as they are particularly large and challenged by a high interaction frequency, task overload, escalation, and temporal demands (Georgiou and Lockey Citation2010). We found it of interest to examine and quantify relational links and social influence of trauma team members, which may inform about specific roles that have a risk for congestion or overload.
The notion that complex tasks sometimes are better solved by a team acting with a divisional structure, which perhaps communicates in a more distributed manner at the expense of an updated mental model for the team, makes it of value to examine patterns of communication. TTR communication is believed to be important for the trauma teams, since they are trained to and strive to operate according to a functional structure, where operations are controlled by a sole team leader and the teams aim for a shared mental model. In this study, the communication patterns with a specific focus on TTR communication were examined.
The environment in which trauma team simulation and IRL trauma teams operate differs. It is important to study strengths and weaknesses in the ‘work as done’ and about differences in these two representations of trauma team environments, as simulated trauma environments are used for training and implementing new work models, assessment, and research of teams.
The overarching aim of this observational study, in the true habitat of trauma teams and trauma simulation teams in the same environment, is to describe, analyse and compare team relations and communicative structures in terms of team social network metrics.
The research questions were:
What can communication distribution reveal about the social network of trauma resuscitation teams and the social influence of different team roles?
How is individually directed communication and TTR communication used in trauma resuscitation teams and does this affect the social networks?
How do IRL and simulated trauma resuscitations teams compare in terms of communicative distribution and social networks produced by communication patterns?
2. Materials and methods
2.1. Design and setting
This descriptive study of video-recorded trauma resuscitations, using quantification of qualitative data and Social Network Theory metrics, was set up at a mid-sized 300-bed regional hospital, Vrinnevisjukhuset, with around 2500 employees in Sweden. The emergency department annually receives 130–170 trauma patients, nationally categorised as level one according to physiological and anatomical criteria (Appendix A). The trauma team assembles in the emergency room in response to pager activation. Trauma teams are also paged to the same emergency room for simulated trauma resuscitation scenarios once every two weeks. The simulations are held by 1–3 educated simulation instructors working at the emergency department. They have no role in this research project. The emergency room is equipped with two cameras and a microphone controlled from a nearby room, for educational purposes.
2.2. Ethics
The Regional Ethical Review Board in Linköping, Sweden (Chairperson Åsa Nilsson Dahlström) approved the study on 9 March 2017 (2017/32-33).
2.3. Data collection and sample
All staff regularly participating in trauma resuscitations were informed about the study through meetings and emails during the spring of 2017. After written consent from all active visible participants, the videos were edited (LF) to include the primary survey after the transition from the ambulance tray to the trauma bed and until transfer out of the trauma bay. The patients were blurred unrecognisable in close connection to the recordings (manually using Adobe Premiere by LF) and could hence not be identified by the researchers during transcription and coding. Informed consent from the patients was waived in accordance with the ethical approval.
During the study period, from August 2017 to February 2018, eight videos from real and simulated resuscitations were included in the study. The included videos were the ones collected that could be analysed first on account of participant consent. The recordings were activated by a staff member not active in the trauma resuscitation teams. A short clinical description of the investigated cases is given in .
Table 2. Overview of the in-real-life (IRL) cases A–D and the simulated (SIM) cases 1–4.
2.4. The trauma teams
The team composition of each case is outlined in . The teams were all formed ad hoc. There were 10–15 team members in the IRL cases, and 12–13 in the simulated cases. All cases contained the following seven members: examining physician, team leader, anaesthesiologist, orthopaedic surgeon, two emergency room nurses, and airway nurse. Detailed information on team members is provided in Appendix B.
All physicians, except interns, were skilled with Advanced Trauma Life Support®, and all registered nurses with Trauma Nurse Core Course (Emergency Nurses Association Citation2022, Trauma Nurse Core Course Manual).
Table 3. Participating team roles in the in-real-life (IRL) cases A–D and in the simulated (SIM) cases 1–4.
2.5. Transcription and coding
The video-recorded material was transcribed verbatim by LF, KL, and IM. LF is an anaesthesiologist, KL is a medical student, and IM is an emergency medicine resident. Communication was transcribed as it occurred temporally. Patient utterances were disregarded, however, communication towards the patient was transcribed. The utterances were transcribed to the length that they could be pronounced without interruption. Hence the next occurring utterance was transcribed, and so forth. Simultaneously uttered sentences were transcribed in random order.
The utterances were coded for the direction of speech. How the speaker approached the others determined if one or many individuals were perceived as targeted by the speech. Delivery of general information, without seemingly approaching a specific individual, was coded as TTR. The three first videos (IRL A, SIM 1, and IRL B) were coded by all three coders for correlation of coding. Discussion and correction were henceforth made at regular intervals to achieve the most coherent coding.
An utterance directed between two individuals was regarded as an outgoing communication event for the sender and one incoming communication event for the receiver. The number of delivered and received verbal communication events for each individual (node) was quantified and put into valued adjacency matrices for each case (Butts Citation2008). Subsequent calculations were made on ‘all communication’, including both TTR and ‘individually directed communication’, and ‘individually directed communication’, respectively. Each case thus gave two different matrices. A fictive example with five utterances A–E, below, is coded and transferred to matrices.
(A) Examining physician: ‘The airway is free’, coded examining physician to the room.
(B) Anaesthesiologist: ‘I will not need the bougie’, coded anaesthesiologist to airway nurse.
(C) Airway nurse: ‘Ok’ coded airway nurse to anaesthesiologist.
(D) Examining physician: ‘Do you think we can go on to B?’, coded examining physician to anaesthesiologist.
(E) Anaesthesiologist: ‘Yes, I believe we can’, coded anaesthesiologist to examining physician.
An utterance with the direction TTR was thus regarded as one outgoing and several ingoing communication events, as there were several receivers of that message. In the matrices for individually directed communication, the TTR communication was not included. The patient was not regarded as a receiver of communication when the team was approached but was regarded as a receiver of direct approach ().
Table 4. Example of coding into a matrix for ‘all communications’.
Table 5. Example of coding into a matrix for ‘individually directed communications’.
2.6. Analysis
Matrices were exported to software tool Gephi 0.9.2 (Bastian, Heymann, and Jacomy Citation2009), and a social network analysis was made. The networks were examined as directed (a direction applied to the connections) and the following social network metrics were used.
2.6.1. Density metrics
Density (0–1) measures how tightly interconnected a network is through the calculation of the number of existing connections (edges) between the individual team members (nodes) compared to the possible number of edges in the network (). A higher number indicates higher density (Scott Citation2017, 82).
Average Clustering coefficient (0–1): Clustering is a global assessment of the tendency for the formation of small groups that may be separated from other parts of the network. Clustering statistics is based on the concept of triplets: three nodes connected to one another through two or three edges. Average global clustering is calculated by the number of closed triplets (connections between all three nodes) to the total number of open (connections from one node to the two other nodes) and closed triplets. A low coefficient indicates that the relation of tightly bound parts to all relational connections is low and illustrates a clustering tendency. A network in which the relation of tightly bound parts to all relational connections is high illustrates a group that is more cohesive on the whole and where specific tight parts are less prominent (lower clustering) (Wasserman and Faust Citation1994).
The calculation of density and clustering aid in interpreting the overall structure of a network. The weights of edges, i.e. number of communication events, are of no concern for these calculations.
2.6.2. Centrality metrics
The centrality metrics below, focus on the individual nodes and their theoretical positions in the network, based on number of delivered and received communication events. All calculations are weighted, i.e. related to the total number of communications between nodes for each case.
Weighted degree centrality is calculated by the weight of the edges for each node. In this study, the degree centrality scores depict busyness in overall communication, whether inward or outward. The magnitude of the degree centrality metrics can be compared between nodes of the same network but not between different networks, as they vary in size (nodes, duration of interaction, and thereby communication events in this study). To compare size differences between degree centralities of different nodes (team roles) between cases, the centrality scores were divided by the score of the most prominent node of the same case. A higher value represents a higher relative influence or sociometric status.
Degree centrality is the sum of In-degree centrality and Out-degree centrality, which represent the occupancy of each node in receiving and delivering communication, respectively.
Overall weighted centralisation (0–1) is an expression of how tightly a network is organised around its most central node in terms of degree centrality. To compare overall weighted centralities between the different networks (teams), overall weighted centralisation was calculated as the sum of the differences between the weighted point centralities of every node and the highest scoring node, divided by the maximum possible sum of differences (Scott Citation2017, 102).
SNA metrics from IRL and simulated cases were compared descriptively.
3. Results
The Intraclass Correlation Coefficient (Shieh Citation2016) on the third analysed video (IRL B) was 0.992 for the three coders.
Transcription details about the cases are outlined in . The densities of the examined cases varied as well as the relative contributions of individually directed communication and TTR (). The density increased for all cases when TTR was included, but the proportional difference varied between cases.
Table 6. Transcription details from the four real (IRL) and four simulated (SIM) cases.Table Footnotea
Average clustering using all and individually directed communication is displayed in . The clustering coefficients were higher when TTR was included in the calculations. A higher coefficient accounts for a lower degree of clustering in groups.
The overall weighted centralisations are displayed in .
presents the relative weighted degree centralities of team roles using individually directed communication. The examining physician had the highest weighted degree centrality in most of the IRL and simulated cases. In IRL A the examining physician, anaesthesiologist and team leader all had high weighted degree centrality, representing shared communicative authority. The same is seen in SIM 1 and 2 between the examining physician and the anaesthesiologist and the team leader, respectively. In IRL B and SIM 4, all team roles had proportionally low weighted degree centrality compared to the examining physician.
In the most prominent outward communicators (weighted out-degree centrality) and receivers (weighted in-degree centrality) of communication are displayed. Overall, the examining physician has a dominating role as outward communicator, but the pattern is more variable regarding the receiving of information.
Table 7. Nodes with the highest weighted point centrality scores of real (IRL A–D) and simulated cases (SIM 1–4) for individually directed and all communicative events.
4. Discussion
This study analysed all verbal communication events from the primary survey of trauma resuscitations in four IRL and four fictive simulated cases from a single emergency department. Communication within the trauma teams was used to calculate social network metrics and thereby explain different aspects of network structure and answer our research questions.
4.1. What can communication distribution reveal about the social network of trauma resuscitation teams and the social influence of different team roles?
The teams consisted of 10–15 members. Density and clustering statistics suggest that the networks were tightly connected and that there were communicative relations between most of the team members.
Overall weighted centralisation was generally high, indicating a concentrated communicative authority around specific roles in the trauma teams. It was the lowest in IRL C, which suggests a decentralised network with many equally influential team members. This case had the least number of interacting individuals, which could facilitate interaction between all regardless of communicative authority. The patient was the primary receiver of communication, indicating a less injured case, and perhaps a less challenging situation for the team.
In all but one case, the examining physician was the most central communicator, with the highest weighted degree centralities also when TTR was disregarded. This reflects the examining physician’s responsibility of delivering status updates, instructions about steps forward, and task prioritisation to the team. In cases with a responsive patient, the examining physician also directed specific communication to the patient during examination. The role of the examining physician as a central coordinator and a communication hub is further underlined as he/she was an important receiver of communication, with the highest individually directed in-degree communication in five of the eight cases. Communicative authority is not necessarily coupled with decisional authority. It rather explains the position of the team member in the communication network, hints about a coordinating role, and might indicate the possibility of communication overload and congestion.
In three of the cases, IRL A, SIM 1, and SIM 2, the communicative authority was shared between the examining physician, the team leader, and the anaesthesiologist in slightly different formations. These three roles correspond to the inner circle of a trauma team (Härgestam et al. Citation2016). These three communication networks suggest a divisional structure with peer-to-peer arrangements between a few important roles that cover different areas of work. Although the formal leadership was attributed to the team leader in these teams, such communication distribution might be the result of negotiated leadership, decision-making, or guidance, especially between the examining physician and the team leader, which can be expected from their appointed roles. It is not expected by the team leader to be the most frequent communicator, as the A-E-examination and directions according to manuals and algorithms are expected to be handled by the examining physician. However, the team leader often has a greater experience than the examining physician, which makes discussions, guidance, and decision-making between them probable, giving rise to the possible explanation of structural peer-to peer-arrangements and a divisional structure tendency.
Case IRL D presented with the most severely injured patient IRL and the team was expanded to 15 individuals. In such a large team there is a potential for loss of cohesion if parts of the team are peripheral and form clusters. The weighted overall centralisation was high, especially when TTR was included, and the average clustering was relatively high. This is an example of how the team acted according to a divisional structure with small parts engaging in different tasks and decisions. The frequency of interactions stood out from all the other cases with 87 verbal communication events per minute, which suggests a resuscitation intensity that perhaps forced a more divisional structure and decentralised network. Team adaptability is often addressed as an important feature of successful teams, and they are characterised by ‘extensive ties, exchangeable membership and expandable tiers’ (Wenger and Snyder Citation2000). This means that there are ties between all individuals, there is a core group that carries out activities, and some individuals assist the team on demand.
4.2. How is individually directed communication and TTR communication used in trauma resuscitation teams and how does this affect the social networks?
The results were calculated using both all and individually directed communication, enabling a study of the effects on network structure from these different communication activities. The TTR-concept was used to summon all TTR-utterances, without specification about what type of communication. Communication directed to the team could be both, e.g. commands and status updates.
TTR increased density and decreased clustering tendency in all the studied IRL and simulated cases, which corresponds to increasing structural cohesion in a team. Cohesion has been found to be coupled with team potency (Blau Citation1977; Tröster, Mehra, and van Knippenberg Citation2014). However, the effect on overall centralisation in the teams differed between the cases, regardless of the amount of TTR used, which was a surprising finding. Overall centralisation would be expected to decrease as a result of increasing connections between team members, but if a team’s composition of team members changes during the course of the resuscitation, a different pattern might be seen.
The relative significance of TTR for increasing density was comparatively low in IRL C. This suggests that connections were already maximised between individuals, also shown by the lowest weighted centralisation values. The opposite was seen in SIM 2 and IRL B, where TTR had a larger influence on density and clustering, indicating that the teams were relying on TTR for information sharing and communicative cohesion in the team. Both IRL cases B and C represent situations with less injured patients who were highly addressed during resuscitation. Still, the network structures were completely different: highly centralised (IRL B) and decentralised (IRL C)., with differences in the proportion of TTR to all communication, which was markedly higher in IRL B (18.8%) than in IRL C (10.1%). This could account for a low communicative authority differentiation in IRL C, and thereby less need for a central communicator to distribute information or coordinate tasks. It could also be that information was more easily shared in IRL C, due to fewer nodes.
A positive correlation between a high density, situation awareness and a shared mental model, which were operationalised as corresponding knowledge about the situation, was found in a study of teams responding to emergencies (Kvamme Solheim and Renke Bracht Citation2017). TTR is a communication strategy that is believed to increase situational awareness and update the team’s mental model, which is considered important for trauma team performance (Johnsen et al. Citation2017). Social networks statistics from this study support that this communication strategy contributes to structural cohesion in a network.
4.3. How do IRL and simulated trauma resuscitation teams compare in terms of communicative distribution and social networks produced by communication patterns?
The proportion of TTR communication was on average higher in the simulated compared to the IRL cases. The simulated cases were composed of life-threatening conditions requiring immediate actions to stabilise the patients. The IRL cases, excluding IRL D, did not force as much definite rapid measures. The intensity of communication was more variable IRL, and there was a high variability regarding overall centralisation. The variability was lower when TTR communication was used, opposite from the trend seen through the simulated scenarios. Among the IRL cases, IRL D showed the highest communication rate, the largest team, and apparently, the most seriously injured patient. The communication rate in IRL D was markedly higher than in the simulated scenarios. In this case, TTR communication increased centralisation. Even though the simulated cases were challenging in terms of resuscitative needs, the IRL environment contains a higher degree of unpredictability in terms of resuscitative needs and environmental challenges. This is probably reflected in the intensified communication rate.
Fernandez et al. (Citation2017) concluded that the balance between shared and distributed knowledge not only depends on the case and the team but also on the clinical environment. Distributed knowledge refers to that certain knowledge or information can reside in parts of the team, whereas shared knowledge represents the common information or knowledge for the team. They call for an understanding about how these factors interact with the team and how simulation training can be adequately designed to enhance team cognition. Our results suggest that the IRL environment challenged the team cognitively in IRL D through immense interaction frequency. If the team would strive for a functional structure, where one central node is used to channel information, that team member could become congested, and abundant information sharing would hamper performance. The team in IRL D seemed accordingly to use a distributed communication structure in its individually directed communication, resulting in a decentralised network. This corresponds to the findings from a similar medical context, where a flattened structure and a high communication intensity appeared in critical phases of non-routine situations, where the teams perceived increased complexity (Barth, Schraagen, and Schmettow Citation2015). The tendency in the simulated cases for high degrees of ‘TTR and a higher centralisation of communication when TTR was excluded, could result from strain from the simulation situation being put on the team, with high demands on task performance, resulting in a centralised communication network structure. It could also result from work being performed in an environment stripped of complexities that occur in IRL cases, creating situations that were more easily solved through beforehand negotiated tasks and roles as well as well-known algorithms for decision-making, despite the patient being severely injured. Price et al. (Citation2001) suggest that a functional structure can be used to increase efficiency in a predictable task-environment. Moreover, in a simulated environment, equipment, material, and patient factors are often created to be optimal for performance, whereas the IRL environment can pose challenges in every step of task performance, and require assistance, advice, and alternate plan formulations, which could induce tendencies to use a more divisional structure approach. Minor decisions and cognitive processes perhaps did not arise to the same extent in the simulated environment, which affected how the teams could manage to maintain a centralised communication network structure. It is also possible that communication and a strive towards working according to expectation was more in focus for the simulating teams, as communication and teamwork is often a learning objective of simulations. This would account for a Hawthorne effect, which might obscure the impact of case acuteness and/or lack of complexity.
The findings in this study underline the context-sensitive aspects of team functioning and structure suggested by Calder et al. (Citation2017), who found more complex team network structures IRL than from simulations. In a discourse analysis on simulated trauma teams, Gundrosen et al. (Citation2016) explain how multitasking and parallel dialogues bring complexity to the situation. ‘On-line commentaries’ (commenting to the team about own activities) that arise in team expansion phases, and in which new team members are engaged and comment on the case, give rise to specific action by original team members. The online commentaries thereby stimulate important implicit behaviour that helps to restore deviations in patient physiology. As shown in the present study, the complexity of an IRL case is exaggerated through an even higher communication intensity, larger teams, and parallel dialogues with a decentralised communication network structure. Trauma team adaptation might be reliant on this ability to decentralise communication and work in a more divisional structure whilst TTR communication helps to maintain team situation awareness.
4.4. Methodological considerations
This study represents one of the few studies examining IRL trauma teams and aspects of teamwork structure. The complexity of the situation and the many participants involved make their momentary interactions largely unmapped. When human individuals interact within a system, the emerging patterns are seldom a reflection of the pre-conceived ideas of the individuals and their presumed relationship (Scott et al. Citation2005). We applied a method to objectify the interaction patterns through social network analysis, which is optimal for large quantities of interactional data. The networks were analysed and weighted by the magnitude of observed verbal communications. To our knowledge, no other more efficient method exists to investigate team communication distribution. The rich amount of data can be used to visualise a scheme of connections that is ‘hidden’, and not possible to grasp even if one is present in the room. The length of resuscitations and the different number of members made some comparisons invalid, such as density comparisons and a direct comparison of degree centrality scores across cases. We therefore calculated the relative degree centrality of nodes. Some studies base their SNA-calculations only on if there are existing links between nodes or not, disregarding the amount. Such comparisons are less labour intense and could potentiate a larger sample, but for these teams and our research questions, it would not be serviceable.
We used quantified qualitative data in our analysis. Thus, transcription and coding have implications for the calculated social network metrics. During transcription, it was obvious that some context familiarity was required to grasp all conversations in the room, which often occurred simultaneously. The audio uptake from the room was made with two microphones, and two cameras visualised the scenario from two angles, making different speakers more apparent from either side. We can never be certain about the intent of speech, and therefore the coherence of the coding was controlled through discussions and calculation of interrater reliability. Yet, we cannot be sure that our coding agrees with the aim of the individual team member. This is an inherent difficulty of a behaviour study.
A behaviour study using video material needs selection (Derry et al. Citation2010). Behaviour and team performance can be assessed in different ways. It is seldom possible to capture all aspects of interaction. This study has not included other ways that the team members interacted, such as gestures and gazes, and it did not regard the content or the form of interaction. It neither included an assessment of the performance quality nor the perspective of the participants.
We have used some of this video material in a study of the verbal forms used to convey messages in IRL trauma, and we could see that for the three most prominent communicators—examining physician, anaesthesiologist, and trauma leader—their most frequent form for communication were edification, question and acknowledgement, respectively. Their most frequent intents were edification, disclosure, and advisement, respectively (Molin, Fornander, and Berggren Citation2021). We acknowledge that the quality, form, and type of communications might impact how leadership is perceived, how other people communicate, team member situation awareness, and the efficiency of operation. However, the SNA methodology gives the possibility to focus on the result of connections—a communication network—that possibly reveals some information about the team structure, which was the objective of this study. The dynamics of the resuscitations are not captured by this study, as the social networks represent a sum of the communication events that took place. It is probable, but beyond the scope of this study, that communication patterns change during phases of resuscitation, which is of interest to analyse further in future studies.
The number of studied cases is limited but balanced to the labour intensity of the research methodology. We were not able to control for the types of cases, as the cases were consecutively included and analysed after informed consent from all participants in the team. If recording had been possible for every trauma case, a greater number could have been collected, giving a possibility for the strategic sampling of the most severely wounded cases. The simulated cases involved more seriously wounded cases. However, the differences in case details and team compositions reflect reality. Compared with the hospital trauma statistics of 2017–2019, the IRL case sample included in the study is representative regarding severity and mechanism of injury. In the manuscript, the cases were sorted based on type (IRL/SIM). However, this was neither the temporal order nor the order of transcription and analysis. Although participants in all cases had been informed about video recording, we cannot tell how this awareness affected the behaviours and whether it differed between the IRL and simulated scenarios. The patients were not, for ethical reasons, considered participants in the team, and their communications were hence disregarded. We believe that their communication or lack of communication affected responses from the team, and all communications towards patients were included in the analysis. Although some information might have been lost due to this methodological choice, we believe that the ethical gains outweigh the losses.
5. Conclusion
The verbal communication in trauma resuscitation teams acting IRL or in a simulated scenario was analysed using social network metrics. Although the trauma team is designed to work according to a functional structure, which is supposed to centralise communication through a coordinating leader, this study shows that both centralised and decentralised communication networks appear when different teams are exposed to variable trauma types.
The IRL cases mostly resulted in more decentralised networks, whereas in the simulated cases, with more challenging injury panoramas, the centralisation using directed communication was higher and more localised to one or a few individuals. TTR activity was also higher in the simulated cases. TTR increased density and reduced clustering, which could imply an increased cohesion.
The results suggest that the context of IRL cases can enforce a more decentralised network structure. This could be due to the unpredictability of an IRL case, which necessitates planning, discussion, coordination, and evaluation also of tasks and decisions that in the simulation environment can be solved according to standards and algorithms. As many trauma team members have over-arching competencies, it is probable that many obstacles can be solved without engaging the team leader or examining physician. The use of TTR balances a distributed communication structure, increases centralisation of coordinating roles, contributes to structural team cohesion, and presumably situation awareness. In the greater research context, the flexibility to communicate as a decentralised network and work in a divisional structure seems beneficial for teams engaging in unpredictable and rapidly changing situations, as it potentiates adaptability. However, a functionally structured team is effective and perhaps superior as long as tasks can be achieved according to routine practice, which minimises interaction costs but enables leader situation awareness. This study complements the scarce earlier studies by including the weights of interaction and comparing the IRL and simulation contexts. To adequately practice communication in the training of resuscitation teams, simulation practices should be designed to put pressure on the teams, which might push teams into a more adaptive mode and mimic the IRL communication during non-routine and escalating situations.
Disclosure statement
No potential conflict of interest was reported by the author(s).
Data availability statement
Data sharing of original videos is not possible as they are sensitive in nature. The datasets supporting the conclusions of this article are available in this article and its Supplementary Files.
Additional information
Funding
References
- ATLS Subcommittee. 2013. “American College of Surgeons’ Committee on Trauma, International ATLS Working Group. Advanced Trauma Life Support (ATLS®): The Ninth Edition.” The Journal of Trauma and Acute Care Surgery 74: 1363–1366.
- Barth, S., J.M. Schraagen, and M. Schmettow. 2015. “Network Measures for Characterising Team Adaptation Processes.” Ergonomics 58 (8): 1287–1302. doi:10.1080/00140139.2015.1009951.
- Bastian, M., S. Heymann, and M. Jacomy. 2009. “Gephi: An Open Source Software for Exploring and Manipulating Networks.” Proceedings of the Third International AAAI Conference on Web and Social Media 3 (1): 361–362. doi:10.13140/2.1.1341.1520.
- Bavelas, A. 1948. “A Mathematical Model for Group Structures.” Human Organization 7 (3): 16–30. doi:10.17730/humo.7.3.f4033344851gl053.
- Beament, T., and S.J. Mercer. 2016. “Speak up! Barriers to Challenging Erroneous Decisions of Seniors in Anaesthesia.” Anaesthesia 71 (11): 1332–1340. doi:10.1111/anae.13546.
- Benton, D.C., F. Pérez-Raya, M.P. Fernández-Fernández, and M.A. González-Jurado. 2015. “A Systematic Review of Nurse-Related Social Network Analysis Studies.” International Nursing Review 62 (3): 321–339. doi:10.1111/inr.12161.
- Blau, P.M. 1977. Inequality and Heterogeneity: A Primitive Theory of Social Structure. Vol. 7. New York, NY: Free Press.
- Brown, T.M., and C.E. Miller. 2000. “Communication Networks in Task-Performing Groups; Effects of Task Complexity, Time Pressure, and Interpersonal Dominance.” Small Group Research 31 (2): 131–157. doi:10.1177/104649640003100201.
- Burtscher, M.J., E.M.J. Ritz, and M. Kolbe. 2018. “Differences in Talking-to-the-Room Behaviour between Novice and Expert Teams during Simulated Paediatric Resuscitation: A Quasi-Experimental Study.” BMJ Simulation & Technology Enhanced Learning 4 (4): 165–170. doi:10.1136/bmjstel-2017-000268.
- Butts, C.T. 2008. “Social Network Analysis: A Methodological Introduction.” Asian Journal of Social Psychology 11 (1): 13–41. doi:10.1111/j.1467-839X.2007.00241.x.
- Calder, L.A., G. Mastoras, M. Rahimpou, B. Sohmer, B. Weitzman, A.A. Cwinn, T. Hobin, and A. Parush. 2017. “Team Communication Patterns in Emergency Resuscitation: A Mixed Methods Qualitative Analysis.” International Journal of Emergency Medicine 10 (1): 24. doi:10.1186/s12245-017-0149-4.
- Courtenay, M., S. Nancarrow, and D. Dawson. 2013. “Interprofessional Teamwork in the Trauma Setting: A Scoping Review.” Human Resources for Health 11 (1): 57. doi:10.1186/1478-4491-11-57.
- Dinh, J.V., A.M. Traylor, M.P. Kilcullen, J.A. Perez, E.J. Schweissing, A. Venkatesh, and E. Salas. 2020. “Cross-Disciplinary Care: A Systematic Review on Teamwork Processes in Health Care.” Small Group Research 51 (1): 125–166. doi:10.1177/1046496419872002.
- Diedrich, F.J., S.P. Hocevar, E.E. Entin, S.G. Hutchins, W.G. Kemple, and D.L. Kleinman. 2002. “Adaptive Architectures for Command and Control: Toward An Empirical Evaluation of Organizational Congruence and Adaptation.” Proceedings of the Command and Control Research & Technology Symposium, Naval Postgraduate School, Monterey, CA, June 2002.
- Derry, S., R. Pea, B. Barron, R. Engle, F. Erickson, R. Goldman, R. Hall, T. Koschmann, J. Lemke, M. Sherin, and B. Sherin. 2010. “Conducting Video Research in the Learning Sciences: Guidance on Selection, Analysis, Technology, and Ethics.” Journal of the Learning Sciences 19 (1): 3–53. doi:10.1080/10508400903452884.
- Emergency Nurses Association. 2022. TNCC Manual. Accessed December 6, 2022. https://www.trauma.se/kurs/tncc/
- Fernandez, R., S. Shah, E.D. Rosenman, S.W.J. Kozlowski, S.H. Parker, and J.A. Grand. 2017. “Developing Team Cognition.” Simulation in Healthcare 12 (2): 96–103. doi:10.1097/SIH.0000000000000200.
- Fornander, L., C. Berterö, I. Molin, K. Laukkanen, L. Nilsson, and K. Björnström. 2023. “Development of Trauma Team Cognition Can Be Explained by “Split Vision”: A Grounded Theory Study.” Journal of Interprofessional Care 5: 1–9. doi:10.1080/13561820.2023.2171970.
- Gao, F., M.L. Cummings, and E. Solovey. 2016. “Designing for Robust and Effective Teamwork in Human-Agent Teams.” In Robust Intelligence and Trust in Autonomous Systems, 167–190. Boston, MA: Springer.
- Georgiou, A., and D. Lockey. 2010. “The Performance and Assessment of Hospital Trauma Teams.” Scandinavian Journal of Trauma, Resuscitation and Emergency Medicine 18 (1): 66. doi:10.1186/1757-7241-18-66.
- Gundrosen, S., E. Andenæs, P. Aadahl, and G. Thomassen. 2016. “Team Talk and Team Activity in Simulated Medical Emergencies: A Discourse Analytical Approach.” Scandinavian Journal of Trauma, Resuscitation and Emergency Medicine 24 (1): 135. doi:10.1186/s13049-016-0325-1.
- Han, Seungheon, Sugy Choi, Jayoung Park, Seoah Kweon, Se Jin Oh, Holly B. Shakya, Jongho Heo, and Woong-Han Kim. 2022. “The Teamwork Structure, Process, and Context of a Paediatric Cardiac Surgery Team in Mongolia: A Mixed‐Methods Approach.” The International Journal of Health Planning and Management 37 (4): 2224–2239. doi:10.1002/hpm.3463.
- Henttonen, K. 2010. “Exploring Social Networks on the Team Level – A Review of the Empirical Literature.” Journal of Engineering and Technology Management 27 (1–2): 74–109. doi:10.1016/j.jengtecman.2010.03.005.
- Hollenbeck, John R., Henry Moon, Aleksander P.J. Ellis, Bradley J. West, Daniel R. Ilgen, Lori Sheppard, Christopher O.L.H. Porter, and John A. Wagner. 2002. “Structural Contingency Theory and Individual Differences: Examination of External and Internal Person-Team Fit.” The Journal of Applied Psychology 87 (3): 599–606. doi:10.1037/0021-9010.87.3.599.
- Houghton, Robert J., Chris Baber, Richard McMaster, Neville A. Stanton, Paul Salmon, Rebecca Stewart, and Guy Walker. 2006. “Command and Control in Emergency Services Operations: A Social Network Analysis.” Ergonomics 49 (12–13): 1204–1225. doi:10.1080/00140130600619528.
- Härgestam, M., M. Hultin, C. Brulin, and M. Jacobsson. 2016. “Trauma Team Leaders’ Non-verbal Communication: Video Registration during Trauma Team Training.” Scandinavian Journal of Trauma, Resuscitation and Emergency Medicine 24 (1): 7. doi:10.1186/s13049-016-0230-7.
- Johnsen, B.H., H.K. Westli, R. Espevik, T. Wisborg, and G. Brattebø. 2017. “High-Performing Trauma Teams: Frequency of Behavioral Markers of a Shared Mental Model Displayed by Team Leaders and Quality of Medical Performance.” Scandinavian Journal of Trauma, Resuscitation and Emergency Medicine 25 (1): 3. doi:10.1186/s13049-017-0452-3.
- Kolbe, M., G. Grote, M.J. Waller, J. Wacker, B. Grande, M.J. Burtscher, and D.R. Spahn. 2014. “Monitoring and Talking to the Room: Autochthonous Coordination Patterns in Team Interaction and Performance.” The Journal of Applied Psychology 99 (6): 1254–1267. doi:10.1037/a0037877.
- Kvamme Solheim, E.L., and A. Renke Bracht. 2017. “Shared Beliefs in Operative Teams: A Social Network Analysis.” University of Bergen. Accessed December 6, 2022. https://bora.uib.no/bora-xmlui/bitstream/handle/1956/17495/Shared-Beliefs-in-Operative-Teams_-A-Social-Network-Analysis.pdf?sequence=1&isAllowed=y
- Lafond, D., M.E. Jobidon, C. Aubé, and S. Tremblay. 2011. “Evidence of Structure-Specific Teamwork Requirements and Implications for Team Design.” Small Group Research 42 (5): 507–535. doi:10.1177/1046496410397617.
- Leavitt, H.J. 1951. “Some Effects of Certain Communication Patterns on Group Performance.” Journal of Abnormal Psychology 46 (1): 38–50. doi:10.1037/h0057189.
- Madani, A., A. Gips, T. Razek, D.L. Deckelbaum, D.S. Mulder, and J.R. Grushka. 2018. “Defining and Measuring Decision-Making for the Management of Trauma Patients.” Journal of Surgical Education 75 (2): 358–369. doi:10.1016/j.jsurg.2017.07.012.
- Maluso, P., M. Hernandez, R.L. Amdur, L. Collins, M.E. Schroeder, and B. Sarani. 2016. “Trauma Team Size and Task Performance in Adult Trauma Resuscitations.” The Journal of Surgical Research 204 (1): 176–182. doi:10.1016/j.jss.2016.05.007.
- Manser, T. 2009. “Teamwork and Patient Safety in Dynamic Domains of Healthcare: A Review of the Literature.” Acta Anaesthesiologica Scandinavica 53 (2): 143–151. doi:10.1111/j.1399-6576.2008.01717.x.
- Molin, I., L. Fornander, and P. Berggren. 2021. “An Analysis of Trauma Team Communication Using the Verbal Response Mode Taxonomy.” Surgery 170 (6): 1849–1854. doi:10.1016/j.surg.2021.05.043.
- Park, S., T.J. Grosser, A.A. Roebuck, and J.E. Mathieu. 2020. “Understanding Work Teams From a Network Perspective: A Review and Future Research Directions.” Journal of Management 46 (6): 1002–1028. doi:10.1177/0149206320901573.
- Price, J., D. Miller, E. Entin, and B. Rubineau. 2001. “Collaborative Planning and Coordinated Team Performance.” Proceedings of the 6th International Command and Control Research and Technology Symposium, Annapolis, MD. http://www.dodccrp.org/events/6th_ICCRTS/Tracks/Papers/Track4/123_tr4.pdf
- Samarth, C.N., and P.A. Gloor. 2009. “Process Efficiency. Redesigning Social Networks to Improve Surgery Patient Flow.” Journal of Healthcare Information Management 23 (1): 20–26.
- Schraagen, Jan Maarten, Mirjam Huis in ‘t Veld, and Lisette De Koning. 2010. “Information Sharing During Crisis Management in Hierarchical vs. Network Teams.” Journal of Contingencies and Crisis Management 18 (2): 117–127. doi:10.1111/j.1468-5973.2010.00604.x.
- Scott, J., A. Tallia, J.C. Crosson, A.J. Orzano, C. Stroebel, B. DiCicco-Bloom, D. O'Malley, E. Shaw, and B. Crabtree. 2005. “Social Network Analysis as an Analytic Tool for Interaction Patterns in Primary Care Practices.” Annals of Family Medicine 3 (5): 443–448. doi:10.1370/afm.344.
- Scott, J. 2017. Social Network Analysis. 2nd ed., edited by M. Steel. London: SAGE Publications Ltd.
- Shaw, M.E. 1964. In Advances in Experimental Social Psychology, Vol. 1, edited by L. Berkowitz, 111. New York, NY: Academic Press.
- Shieh, G. 2016. “Choosing the Best Index for the Average Score Intraclass Correlation Coefficient.” Behavior Research Methods 48 (3): 994–1003. doi:10.3758/s13428-015-0623-y.
- Tiel Groenestege-Kreb, D., O. van Maarseveen, and L. Leenen. 2014. “Trauma Team.” British Journal of Anaesthesia 113 (2): 258–265. doi:10.1093/bja/aeu236.
- Tröster, C., A. Mehra, and D. van Knippenberg. 2014. “Structuring for Team Success: The Interactive Effects of Network Structure and Cultural Diversity on Team Potency and Performance.” Organizational Behavior and Human Decision Processes 124 (2): 245–255. doi:10.1016/j.obhdp.2014.04.003.
- Wasserman, S., and K. Faust. 1994. Social Network Analysis: Methods and Applications, 243. Cambridge: Cambridge University Press.
- Wellman, B., and S.D. Berkowitz, eds. 1988. Social Structures: A Network Approach. Cambridge: Cambridge University Press.
- Wenger, E.C., and W.M. Snyder. 2000. “Communities of Practice: The Organizational Frontier.” Harvard Business Review 78 (1): 139–145.
- Westli, H.K., B.H. Johnsen, J. Eid, I. Rasten, and G. Brattebo. 2010. “Teamwork Skills, Shared Mental Models, and Performance in Simulated Trauma Teams: An Independent Group Design.” Scandinavian Journal of Trauma, Resuscitation and Emergency Medicine 18 (1): 47. doi:10.1186/1757-7241-18-47.
- Wise, S., C. Duffield, M. Fry, and M. Roche. 2020. “Clarifying Workforce Flexibility from a Division of Labor Perspective: A Mixed Methods Study of an Emergency Department Team.” Human Resources for Health 18 (1): 17. doi:10.1186/s12960-020-0460-7.
- Xiao, Y., C.F. Mackkenzie, and R. Patchy. 1998. “Team Coordination and Breakdowns in a Real-Life Stressful Environment.” Proceedings of the Human Factors and Ergonomics Society 42nd Annual Meetings.
Appendix A.
Trauma statistics from the studied hospital
Table A1. Description of the hospitals level one trauma statistics in regards of trauma mechanisms, age groups and severity of incoming cases.
Appendix B.
Further details about participating roles in in-real-life cases IRL A–D and simulated cases SIM 1–4
Examining physician
An emergency room (ER) physician (IRL A, IRL B, IRL D, SIM 2, SIM 3), surgery resident (SIM 1, SIM 4), or intern (IRL C).
Team leader
An ER physician (SIM 1, SIM 4), surgery resident, or consultant (IRL A, IRL B, IRL C, IRL D, SIM 2, SIM 3).
Anaesthesiologist
A resident or consultant anaesthesiologist.
Orthopaedic surgeon
Orthopaedic surgery resident.
ER nurse 1 and 2
Registered nurse (3 years of nursing school), working in the ER.
Airway nurse
A nurse with 3-year nursing school and skilled with one year of anaesthesia or intensive care.
In addition, all but one case (SIM 4) contained one or two ER assistant nurses. In one case (IRL D) the documenting nurse, a skilled assistant nurse, assisted closure of wounds and immobilisation of extremity fracture.
The coordinating nurse (CN) is an experienced registered nurse, working with coordinating staff and patients at the emergency department. In the cases (IRL A, IRL D, SIM 2, SIM 4) where the CN was active during primary survey, he/she was not present/active in the room during the entire resuscitation.
Figure 1. Examples of social networks as examined by Leavitt (Citation1951). Black node: team leader; Grey node: team member; Black line: communication.
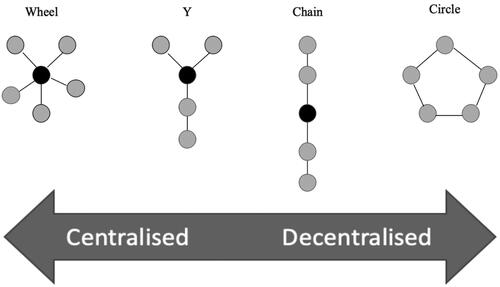
Figure 2. Density is calculated as 2M/(N(N − 1)), where N = number of nodes (circles A–E in the figure) and M = number of connections between nodes.
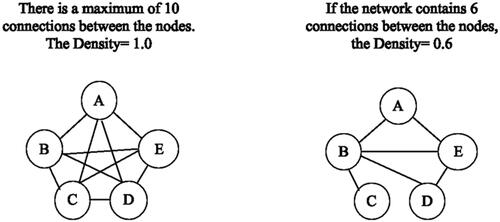
Figure 3. Density of real (IRL A–D) and simulated (SIM 1–4) cases, using all and individually directed communication. ‘All communication’ is the sum of individually directed communication and ‘talking to-the-room’.
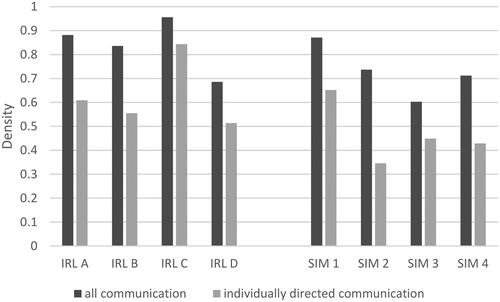
Figure 4. Average clustering of real (IRL A–D) and simulated (SIM 1–4) cases using all and individually directed communication. ‘All communication’ is the sum of individually directed communication and ‘talking to-the-room’.
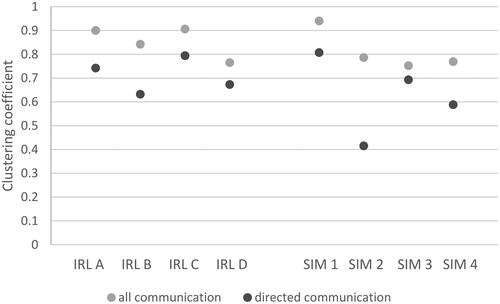
Figure 5. Overall weighted centralisation comparing all and individually directed communication for real (IRL A–D) and simulated (SIM 1–4) cases. ‘All communication’ is the sum of individually directed communication and ‘talking to-the-room’.
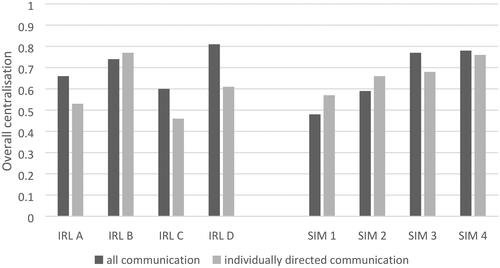
Figure 6. The relative weighted degree centralities of different team roles of the real (IRL A–D) and simulated (SIM 1–4) cases using individually directed communication. EP: examining physician; A: anaesthesiologist; TL: team leader; EN: emergency room nurse; EN2: emergency room nurse 2; AN: airway nurse; EAN: assistant nurse; P: patient; OS: orthopaedic surgeon; DN: documenting nurse; EAN2: assistant nurse 2; XN: radiology nurse; M: instructor; A2: anaesthesiologist 2; M2: instructor 2.
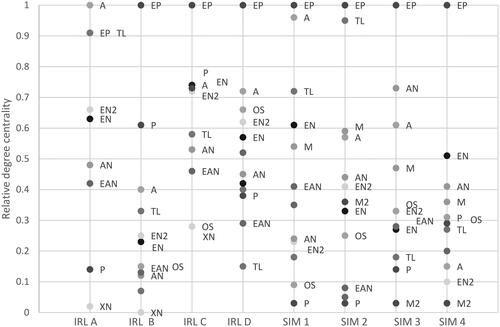