Abstract
We reflect briefly on the last forty years or so of ergonomics and human factors research in automation, observing that many of the issues being discussed today are the same as all those decades ago. In this paper, we explicate one of the key arguments regarding the application of automation in complex safety-critical domains, which proposes restraining the capabilities of automation technology until it is able to fully and completely take over the task at hand. We call this the ‘cliff-edge’ principle of automation design. Instead, we espouse a use for the technology in a more problem-driven, human-centred way. These are not entirely new ideas and such a philosophy is already gaining traction in ergonomics and human factors. The point is that in a given system, tasks should be controlled either by human or by automation; anything in between only causes problems for system performance.
Practitioner summary
Human factors problems with automation have been with us for over forty years, and have changed little in that time. This brief review shows a groundswell of opinion that points to what we call the cliff-edge automation principle – restraining the full capabilities of technology until it is ready to fully and completely take over the task. This approach improves human performance in the system by keeping the person in the loop and in control. Researchers and practitioners in ergonomics and human factors should continue to push this message to the designers and manufacturers of automated systems.
The (old) problems with automation
Readers of this journal will be aware that ergonomists and human factors (E/HF) specialists have been debating the relative merits of automation for at least forty years (e.g. Bainbridge Citation1983). Those same readers will also be aware that, for the most part, the core arguments from an E/HF perspective have changed little during that time.
It was in the 1970s that the information technology revolution led to predictions that automation would reduce complexity in our lives, enhance productivity, and improve quality of life through relieving us of tasks that are difficult, time-consuming, or subject to error (Boff Citation2006). In reality, we learned that such technology actually served to increase complexity in many tasks and, rather than reducing errors, it has in fact introduced new types of error (Billings Citation1991), a classic example being mode errors (Sarter and Woods Citation1995; Stanton and Salmon Citation2009; Stanton, Dunoyer, and Leatherland Citation2011). Anyone working in the arena of automation human factors would recognise these issues as equally applicable to the latest automated (complex) systems; because many such systems still rely on humans playing some role in the system, problems typically arise at the human-automation interface (Stanton and Marsden Citation1996).
One of the central pillars in the response of human factors to the challenges of automation has been to design the system around the user: their needs and wants, their capabilities and limitations. Originally, this took a Fitts-esque approach to allocation of function (see e.g. Hancock Citation2019), offering tasks to the human or machine depending on which they were better at performing. Subsequently, numerous taxonomies emerged describing levels of automation (e.g. Endsley and Kaber Citation1999; Parasuraman, Sheridan, and Wickens Citation2000; Sheridan and Verplank Citation1978): from fully human control, through various combinations of partial automation, to fully automated control. But these taxonomies were largely descriptive and did not really tell us how or why a given task should be allocated to human or machine. Accordingly, the current zeitgeist focuses on human-automation teaming, using the technology to support, rather than replace, the human operator (e.g. Dekker Citation2004; Schutte Citation1999; Young and Stanton Citation2002; Young, Stanton, and Harris Citation2007; see also de Winter, Petermeijer, and Abbink Citation2022). In doing so, the human is kept ‘in the loop’, improving their performance and thereby the performance of the overall human-automation system.
The situation is even more nuanced than that, because some tasks can be automated with no overt impact on the human operator, depending on the level of information processing involved (cf. Parasuraman, Sheridan, and Wickens Citation2000; Young, Stanton, and Harris Citation2007). Tasks that require little or no conscious processing, or those that require a response beyond the limits of human performance, may even benefit from automation. Examples of such include traction or stability control systems in cars, while some fast jet aircraft contain such complex avionics that it is impossible to fly them without the aid of a computer. What these represent, though, are part-task automation, which is fine if it is limited to the relevant sub-task. The problems arise when automation purports to assume a more consciously controlled task – and, worse still, only some of that task (i.e. partial automation).
Despite a weight of E/HF research literature demonstrating the consequences of this approach, in practice the technology-centred philosophy of ‘automate everything we can’ has prevailed. The problem with this philosophy lies in those two latter words, because ‘automate everything we can’ rarely equates to ‘automate everything’. Limitations in technology in many areas means that there often remains some role for the human in otherwise automated systems, being on hand to take over as and when the situation goes beyond the capabilities of the automation. Problems then arise with almost – but not perfectly – reliable automation, because human operators come to rely on it, and are then not prepared to take over when required (and then typically get the blame for doing so; see Chu and Liu, Citation2023).
As we have already noted, these problems are not new. Lisanne Bainbridge’s seminal 1983 paper on the ironies of automation was undoubtedly a watershed moment for the discipline; many of us working in this field have been inspired by this work and continue to cite it even to this day. One of Bainbridge’s core arguments is that the human operator is often only accommodated in automated systems to pick up the ‘tasks left over’ that the automation cannot do, leaving a patchwork quilt of tasks which does not offer meaningful work that the operator can make sense of. Crucially, one of these tasks is being ready to take over should the automation fail or should the situation go outside its design specification. Later, in 1987, James Reason expanded on this in describing the ‘catch-22′ of human supervisory control, in that operators can only take over if they are themselves practiced at the task, which is impossible if it is automated most of the time. Ironically, it is exactly in those scenarios when a human operator could use some automated support. The catch, then, is that automation is most effective when it is least required, and vice-versa. Picking up on this, Don Norman’s Citation1990 paper told us that the problem is not automation itself, just the fact that it is at an intermediate level of intelligence: it can cope with many things, but not everything. Bringing this brief review full circle, the problem then is that humans are only retained in the system to step in when the automation can no longer cope – a task for which people are ‘magnificently disqualified’ (Hancock Citation2014). Humans are not best suited to supervising an automated system for any length of time, as decades of research into vigilance have shown (e.g. Mackworth Citation1948). ‘[I]f you build systems where people are rarely required to respond, they will rarely respond when required’ (Hancock Citation2014, 453).
The cliff-edge automation principle
Norman (Citation1990) suggested that for automation to be useful, we should either improve it or remove it entirely. Rather than automating where we can, then, the human factors approach considers whether we should automate at all (cf. Parasuraman Citation1987). In our recent book on driving automation (Young and Stanton Citation2023), we developed this argument into a philosophy of automation design that we termed ‘cliff-edge automation’. Following the principle that the user should retain a meaningful role in the system, remaining in active control of the task to optimise their performance, we argued that automation should be restrained until such a time as it can fully take over the task with perfect reliability (which, in many cases, will not be any time soon). This is what we meant by the cliff-edge: rather than a gradual slide towards full automation, transitioning through the problematic intermediate levels as the technology evolves and becomes more capable, we should instead hold back until it is possible to jump straight to full automation (see for a conceptual illustration).
Figure 1. Conceptual illustration of the human-centred ‘cliff-edge’ principle through notional levels of automation (from 0 – no automation, to 5 – full automation). Rather than implementing each level of automation when it becomes technologically possible, thus reducing human involvement in a stepwise fashion (dotted line), we should maintain human control until we can step straight to full automation (solid line) (Source: Young and Stanton Citation2023).
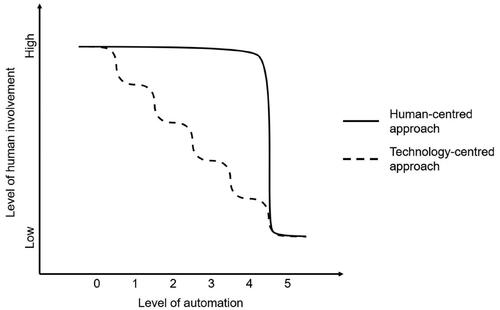
There is widespread support in human factors for this cliff-edge type of philosophy in limiting the full functionality of automation (e.g. de Winter, Petermeijer, and Abbink Citation2022; Hancock Citation2017; Kaber and Endsley Citation2004; Mueller, Reagan, and Cicchino Citation2021; Schutte Citation1999). In the automotive domain, Norman (Citation2015) made a convincing argument that almost-full automation is most problematic, as drivers come to rely on it and so struggle to take over control when needed (see also Noy, Shinar, and Horrey Citation2018). If the system appears to be very able, but is actually imperfect, drivers might overtrust it and think it can do more than it is actually capable of (Banks, Eriksson, et al. Citation2018; Banks, Plant, and Stanton Citation2018; Ljung Aust Citation2020). Lee and See (Citation2004) suggested that, in some circumstances, a simpler but less capable automation may be better than a more complex but less trustable version. Indeed, it was argued a very long time ago by Wiener and Curry (Citation1980) that aviation automation had already passed its optimal point. So full automation in itself is not the problem; the difficulties arise in transitioning through intermediate levels of automation to get there (Norman Citation2015).
We are starting to see shades of this approach in current practice, as some aviation models are indeed predicated on going straight to full autonomy, because this is seen as less complex than transitioning through a human-in-the-loop model (CIEHF Citation2020). Even some of the trailblazers of automated vehicles have considered skipping partial automation levels, in which the human might need to intervene, and instead pushing straight on to fully automated vehicles (Noy, Shinar, and Horrey Citation2018). The point is, we either hand over control fully and completely, or else keep a human in the driving seat – literally and metaphorically (cf. Banks and Stanton Citation2016; Stanton et al. Citation2020).
A way forward
But it is likely to be several decades before automation technology is good enough to fully and completely take over a task with no human fallback option – certainly as far as a complex system such as driving is concerned, anyway. So what happens in the meantime? Do we play Luddite and try to block the implementation of these systems? The answer, of course, is no. We said a long time ago (Young and Stanton Citation1997) that we are not technophobes and we would not be able to stop the tide of technology even if we wanted to. More to the point, there is a convincing argumentFootnote1 made for driving automation that we should embrace the technology as soon as possible anyway, even if it is not perfect, because although accidents of automation will happen, there will not be as many as those caused by (distracted) human drivers. There is some statistical merit to this argument, as some models suggest that even if automated vehicles are only slightly safer than human performance, hundreds of thousands of lives can be saved over a period of 15–30 years (Kalra and Groves Citation2017).
Such statistics may be debated as to whether they account for the shortfalls in benefits of automation as a consequence of the human factors problems (cf. Stanton and Marsden Citation1996). Nevertheless, as Seppelt and Victor (Citation2016) point out, this still puts us in a bind where if we automate then human performance gets worse, but if we do not automate then we negate any potential benefits of automation for road safety (cf. Norman Citation2015). We have been focusing in this paper on the effect of automation on the human, because in many automated systems the human is the crucial last link in the chain to maintain overall system performance. But, in fact, we should be considering the big picture of that overall performance, and the trade-offs between automated and human control. So how do we reconcile our cliff-edge principle with this argument?
The answer is to exploit the same technology that makes automation possible (that is, the sensors, processors and algorithms), but rather than using it to remove tasks from the human operator, which effectively neglects their needs, instead provide them with additional information or support where they need it most. This problem-driven approach maintains that we should not use technology for its own sake – even reverting to a low-technology solution, or possibly not using the full potential of the automation, in favour of optimising human performance (Hancock et al. Citation1996; Owens, Helmers, and Sivak Citation1993). Whatever solution is offered, it should address a need on the part of the human.
Take adaptive cruise control (ACC) as an example. As we know, the argument for automation is often based on evidence from errors or accidents (e.g. Broughton and Markey Citation1996); the case for ACC was in part based on the fact that over a quarter of all road traffic collisions are due to rear-end collisions (Gilling Citation1997). If we break this down, it follows that drivers have some difficulty perceiving relative speed in a car-following situation. But do we really need a technological solution for that problem, or would a low-tech approach suffice? Perhaps we should instead build on the success of centre high-mounted brake lights (Farmer Citation1996) and improve the perception of vehicle rear-ends?
We said earlier that we are not technophobic, so we could alternatively use the same technology to different ends, providing the driver with information to support the task that they normally do, rather than taking over that task for them (Billings Citation1991; Wiener and Curry Citation1980). This approach can reduce workload while maintaining situation awareness (Selcon and Taylor Citation1991; Selcon, Taylor, and Shadrake Citation1992) as well as negating any concerns about resuming control in the event of failure (Wickens et al. Citation2015), as the driver maintains control of the task and the system simply provides them with extra information. As such, this would avoid many of the problems of automation associated with being out of the loop.
Applying this to the ACC example, the system’s sensors could be used to provide drivers with advice and/or warnings about the speed (relative or actual) of, or headway from, the lead vehicle. Instead of assuming longitudinal control for them, this would support their judgement of time-to-collision (Stanton and Young Citation2005), which is a complex perceptual judgement especially difficult for inexperienced drivers (e.g. Cavallo and Laurent Citation1988). Using technology for information acquisition and analysis exploits the computing power to take care of calculating and integrating information (Seong and Bisantz Citation2008), supporting drivers’ judgement and thereby adding value to the human-automation relationship. In a similar way, Navarro, Mars, and Young (Citation2011) suggested vision enhancement as an example of perception support, for the 75% of crashes on rural roads that are a result of poor markings of lanes or road edges (That said, Stanton and Pinto (Citation2000) cautioned that behavioural compensation by drivers could negate any safety benefits of vision enhancement).
The kinds of solutions offered above are generic and are in line with the consensus towards human-centred support rather than technology-centred automated replacement (Young and Stanton Citation2002), fostering human strengths while compensating for their weaknesses (Grote et al. Citation1995). Much of this can be achieved through the interface display, without necessarily ‘automating’ in the traditional sense (cf. Endsley Citation1987), as improved sensor and display technology have shifted trends in display design from providing data towards supporting problem-solving and decision-making (Borst, Flach, and Ellerbroek Citation2015). Similarly, Endsley (Citation2017) stated that automation at earlier stages of information processing (i.e. information acquisition) is more beneficial for situation awareness than at action selection or implementation (see also Stanton et al. Citation2017; Wickens et al. Citation2015), arguing that we should automate only where necessary and at the lowest possible level.
In other words, it is better to use technology to support users’ perception than to replace control or make their decisions for them (Stanton et al. Citation2001). Given that people will still be involved in the control loop for some time to come, they should actually be in control rather than passively supervising (cf. Billings Citation1991). If that means holding off on full automation until the technology is capable enough, then so be it.
Conclusion
In reflecting on the last 40 years or so of E/HF research in automation, we have highlighted that many of the same human factors problems with automation continue to afflict us. Despite decades of research effort in our discipline, the technology-centred, incremental advance towards full automation still prevails. Just as researchers and practitioners in ergonomics and human factors must defend the role of the human in automated systems (see Chu and Liu, Citation2023), we must also continue to push our messaging that the technology-centred approach is beset with all manner of human factors concerns. These concerns lie mostly in that challenging middle ground on the levels of automation taxonomies, where the automation can do much of the task, but still relies on a human as fallback. In this respect we agree with Norman (Citation1990), that automation should be improved or removed, and recommend that the cliff-edge automation principle should be adopted as a solution. Notwithstanding technology-centred claims to the contrary, the human factors problems only disappear when fully automated systems that require no human oversight can assume all of the task in all situations.
The aim of this paper was to collate what is becoming a consensus opinion in E/HF research about the application of automation in complex systems, which we have labelled the ‘cliff-edge’ principle, and to present it as a possible way forward. Whilst we have drawn heavily on driving automation examples to illustrate our point, we suggest that the principles are not necessarily limited to the automotive domain. Indeed, many of the issues are rooted in lessons learned from aviation automation (e.g. Billings Citation1991; Wiener and Curry Citation1980). A notable case in point is the tragic loss of Air France flight 447 on 1 June 2009, which fundamentally resulted from a mismatch in understanding of the situation between the flight crew and the aircraft’s automated systems (see e.g. Salmon, Walker, and Stanton Citation2016, for a discussion). There is, undoubtedly, debate to be had about the relative merits of being in manual control for extended periods (such as a long-haul flight). Furthermore, a key distinction between aviation and driving is the level of training for those controlling the machines, which is of course much more rigorous for pilots than for drivers. Whilst such training may serve to offset some of the performance degradations seen when using automated systems (e.g. Young and Clynick Citation2005), there is still a good argument for designing out such problems in the first place.
But cases such as Air France flight 447 show us that the age-old problems of automation have not gone away, even in aviation, hence the calls for a move towards full autonomy (CIEHF Citation2020) as per the cliff-edge principle. With more automation entering into more complex, safety-critical domains, it behoves us in the discipline of human factors to continue researching, developing and promoting solutions.
We have put forward the cliff-edge principle as one such solution. Many questions remain about the contexts and circumstances under which it may be applied; these are outside the scope of this brief review and we hope that, instead, they be the subject of further debate and research on this topic.
Until then, we believe a more problem-driven use of technology can help to exploit its benefits while allowing people to remain in the control loop. But we are not entirely anti-automation; in fact, as a closing thought we would return to the observation that for simpler, non-safety-critical tasks, it does have a place. In these tasks, though, there is little need for a human in the loop anyway. Take domestic appliances as an example: once the dishwasher or washing machine door is closed, we can forget about it until it has finished its cycle and it is time to unload. In a similar way, there is a role for automated safety systems that sit quietly in the background until they are needed, without interfering with the task being performed by the human (cf. Young, Stanton, and Harris Citation2007). Note, though, that these cases have effectively followed the cliff-edge principle anyway, bypassing any reliance on human involvement. Our concerns lie with the complex tasks that cannot be fully automated and still need human supervisory input. It could be said that such systems are not genuinely automated anyway – and so, we might ask, why bother at all?
Disclosure statement
No potential conflict of interest was reported by the author(s).
Additional information
Funding
Notes
1 Put forward by Professor Don Norman in a 2015 blog post at: https://jnd.org/automatic_cars_or_distracted_drivers_we_need_automation_sooner_not_later/ (accessed 11 May 2022).
References
- Bainbridge, L. 1983. “Ironies of Automation.” Automatica 19 (6): 775–779. doi:10.1016/0005-1098(83)90046-8.
- Banks, Victoria A., Alexander Eriksson, Jim O’Donoghue, and Neville A. Stanton. 2018. “Is Partially Automated Driving a Bad Idea? Observations from an on-Road Study.” Applied Ergonomics 68: 138–145. doi:10.1016/j.apergo.2017.11.010.
- Banks, V. A., K. L. Plant, and N. A. Stanton. 2018. “Driver Error or Designer Error: Using the Perceptual Cycle Model to Explore the Circumstances Surrounding the Fatal Tesla Crash on 7th May 2016.” Safety Science 108: 278–285. doi:10.1016/j.ssci.2017.12.023.
- Banks, V. A., and N. A. Stanton. 2016. “Keep the Driver in Control: Automating Automobiles of the Future.” Applied Ergonomics 53(Pt B): 389–395. doi:10.1016/j.apergo.2015.06.020.
- Billings, C. E. 1991. “Toward a Human-Centred Aircraft Automation Philosophy.” The International Journal of Aviation Psychology 1 (4): 261–270. doi:10.1207/s15327108ijap0104_1.
- Boff, K. R. 2006. “Revolutions and Shifting Paradigms in Human Factors & Ergonomics.” Applied Ergonomics 37 (4): 391–399. doi:10.1016/j.apergo.2006.04.003.
- Borst, C., J. M. Flach, and J. Ellerbroek. 2015. “Beyond Ecological Interface Design: Lessons from Concerns and Misconceptions.” IEEE Transactions on Human-Machine Systems 45 (2): 164–175. doi:10.1109/THMS.2014.2364984.
- Broughton, J., and K. A. Markey. 1996. In-Car Equipment to Help Drivers Avoid Accidents (TRL Report No. 198.). Crowthorne, Berkshire: Transport Research Laboratory.
- Cavallo, V., and M. Laurent. 1988. “Visual Information and Skill Level in Time-to-Collision Estimation.” Perception 17 (5): 623–632. doi:10.1068/p170623.
- Chu, Y., and P. Liu. 2023. “Automation Complacency on the Road.” Ergonomics 2023: 1–20. doi:10.1080/00140139.2023.2210793.
- CIEHF. 2020. The Human Dimension in Tomorrow’s Aviation System. Chartered Institute of Ergonomics and Human Factors. Accessed 26 March 2023. https://ergonomics.org.uk/resource/tomorrows-aviation-system.html.
- Dekker, S. 2004. “On the Other Side of Promise: What Should we Automate Today?” In Human Factors for Civil Flight Deck Design, edited by D. Harris, 183–198. Aldershot: Ashgate.
- De Winter, J. C. F., S. M. Petermeijer, and D. A. Abbink. 2022. “Shared Control versus Traded Control in Driving: A Debate around Automation Pitfalls.” Ergonomics 2022: 1–27. doi:10.1080/00140139.2022.2153175.
- Endsley, M. R. 1987. “The Application of Human Factors to the Development of Expert Systems for Advanced Cockpits.” Proceedings of the Human Factors Society Annual Meeting 31 (12): 1388–1392. doi:10.1177/154193128703101219.
- Endsley, M. R. 2017. “From Here to Autonomy: Lessons Learned from Human-Automation Research.” Human Factors 59 (1): 5–27. doi:10.1177/0018720816681350.
- Endsley, M. R., and D. B. Kaber. 1999. “Level of Automation Effects on Performance, Situation Awareness and Workload in a Dynamic Control Task.” Ergonomics 42 (3): 462–492. doi:10.1080/001401399185595.
- Farmer, C. M. 1996. “Effectiveness Estimates for Center High Mounted Stop Lamps: A Six-Year Study.” Accident; Analysis and Prevention 28 (2): 201–208. doi:10.1016/0001-4575(95)00054-2.
- Gilling, S. P. 1997. “Collision Avoidance, Driver Support and Safety Intervention Systems.” Journal of Navigation 50 (1): 27–32. doi:10.1017/S0373463300023559.
- Grote, G., S. Weik, T. Wafler, and M. Zolch. 1995. “Criteria for the Complementary Allocation of Functions in Automated Work Systems and Their Use in Simultaneous Engineering Projects.” International Journal of Industrial Ergonomics 16 (4–6): 367–382. doi:10.1016/0169-8141(95)00019-D.
- Hancock, P. A. 2014. “Automation: how Much is Too Much?” Ergonomics 57 (3): 449–454. doi:10.1080/00140139.2013.816375.
- Hancock, P. A. 2017. “Imposing Limits on Autonomous Systems.” Ergonomics 60 (2): 284–291. doi:10.1080/00140139.2016.1190035.
- Hancock, P. A. 2019. “Some Pitfalls in the Promises of Automated and Autonomous Vehicles.” Ergonomics 62 (4): 479–495. doi:10.1080/00140139.2018.1498136.
- Hancock, P.A., R. Parasuraman, and E. A Byrne. 1996. “Driver-Centred Issues in Advanced Automation for Motor Vehicles.” In Automation and Human Performance: Theory and Applications edited by R. Parasuraman and M. Mouloua, 337–364. Mahwah, NJ: Lawrence Erlbaum Associates.
- Kaber, D. B., and M. R. Endsley. 2004. “The Effects of Level of Automation and Adaptive Automation on Human Performance, Situation Awareness and Workload in a Dynamic Control Task.” Theoretical Issues in Ergonomics Science 5 (2): 113–153. doi:10.1080/1463922021000054335.
- Kalra, N., and D. G. Groves. 2017. The Enemy of Good: Estimating the Cost of Waiting for Nearly Perfect Automated Vehicles (Report RR2150). Santa Monica, CA: RAND Corporation. Accessed 26 March 2023. https://www.rand.org/content/dam/rand/pubs/research_reports/RR2100/RR2150/RAND_RR2150.pdf.
- Lee, J. D., and K. A. See. 2004. “Trust in Automation: Designing for Appropriate Reliance.” Human Factors 46 (1): 50–80. doi:10.1518/hfes.46.1.50_30392.
- Ljung Aust, M. 2020. How do we know the driver is in the loop? Second Interactive Symposium on Research & Innovation for Connected and Automated Driving in Europe (EUCAD2020). Accessed 26 March 2023. https://www.connectedautomateddriving.eu/_old_wp-content/uploads/2020/09/3.-EUCAD2020-Mikael-Ljung-Aust-How-do-we-know-the-driver-is-in-the-loop.pdf.
- Mackworth, N.H. 1948. “The Breakdown of Vigilance During Prolonged Visual Search.” Quarterly Journal of Experimental Psychology, 1: 6–21.
- Mueller, A. S., I. J. Reagan, and J. B. Cicchino. 2021. “Addressing Driver Disengagement and Proper System Use: Human Factors Recommendations for Level 2 Driving Automation Design.” Journal of Cognitive Engineering and Decision Making 15 (1): 3–27. doi:10.1177/1555343420983126.
- Navarro, J., F. Mars, and M. S. Young. 2011. “Lateral Control Assistance in Car Driving: classification, Review and Future Prospects.” IET Intelligent Transport Systems 5 (3): 207–220. doi:10.1049/iet-its.2010.0087.
- Norman, D. A. 1990. “The ‘Problem’ with Automation: inappropriate Feedback and Interaction, Not ‘over-Automation.” Philosophical Transactions of the Royal Society of London 327 (1241): 585–593. doi:10.1098/rstb.1990.0101.
- Norman, D. A. 2015. “The Human Side of Automation.” In Road Vehicle Automation, edited by G. Meyer and S. Beiker, vol. 2, 73–79. Switzerland: Springer International Publishing.
- Noy, I. Y., D. Shinar, and W. J. Horrey. 2018. “Automated Driving: Safety Blind Spots.” Safety Science 102: 68–78. doi:10.1016/j.ssci.2017.07.018.
- Owens, D. A., G. Helmers, and M. Sivak. 1993. “Intelligent Vehicle Highway Systems: A Call for User-Centred Design.” Ergonomics 36 (4): 363–369. doi:10.1080/00140139308967893.
- Parasuraman, R. 1987. “Human-Computer Monitoring.” Human Factors 29 (6): 695–706. doi:10.1177/001872088702900609.
- Parasuraman, R., T. B. Sheridan, and C. D. Wickens. 2000. “A Model for Types and Levels of Human Interaction with Automation.” IEEE Transactions on Systems, Man, and Cybernetics. Part A, Systems and Humans 30 (3): 286–297. doi:10.1109/3468.844354.
- Reason, J. 1987. “Cognitive Aids in Process Environments: prostheses or Tools?” International Journal of Man-Machine Studies 27 (5–6): 463–470. doi:10.1016/S0020-7373(87)80010-X.
- Salmon, P. M., G. H. Walker, and N. A. Stanton. 2016. “Pilot Error versus Sociotechnical Systems Failure: A Distributed Situation Awareness Analysis of Air France 447.” Theoretical Issues in Ergonomics Science 17 (1): 64–79. doi:10.1080/1463922X.2015.1106618.
- Sarter, N. B., and D. D. Woods. 1995. “How in the World Did we Ever Get into That Mode? Mode Error and Awareness in Supervisory Control.” Human Factors 37 (1): 5–19. doi:10.1518/001872095779049516.
- Schutte, P. 1999. “Complemation: An Alternative to Automation.” Journal of Information Technology Impact 1 (3): 113–118.
- Selcon, S. J., and R. M. Taylor. 1991. “Decision Support and Situational Awareness.” In Designing for Everyone: Proceedings of the 11th Congress of the International Ergonomics Association, edited by Y. Quéinnec and F. Daniellou, 792–794. London: Taylor & Francis.
- Selcon, S. J., R. M. Taylor, and R. A. Shadrake. 1992. “Multi-Modal Cockpit Warnings: Pictures, Words, or Both?” Proceedings of the Human Factors Society Annual Meeting 36 (1): 57–61. doi:10.1177/154193129203600115.
- Seong, Y., and A. M. Bisantz. 2008. “The Impact of Cognitive Feedback on Judgment Performance and Trust with Decision Aids.” International Journal of Industrial Ergonomics 38 (7–8): 608–625. doi:10.1016/j.ergon.2008.01.007.
- Seppelt, B. D., and T. W. Victor. 2016. “Potential Solutions to Human Factors Challenges in Road Vehicle Automation.” In Road Vehicle Automation, edited by G. Meyer and S. Beiker, vol. 3, 131–148. Cham, Switzerland: Springer International.
- Sheridan, T. B., and W. L. Verplank. 1978. Human and Computer Control of Undersea Teleoperators. Office of Naval Research Report. Cambridge, MA: Massachusetts Institute of Technology. Accessed 26 March 2023. https://apps.dtic.mil/sti/pdfs/ADA057655.pdf.
- Stanton, N. A., A. Dunoyer, and A. Leatherland. 2011. “Detection of New in-Path Targets by Drivers Using Stop & Go Adaptive Cruise Control.” Applied Ergonomics 42 (4): 592–601. doi:10.1016/j.apergo.2010.08.016.
- Stanton, N. A., A. Eriksson, V. A. Banks, and P. A. Hancock. 2020. “Turing in the Driver’s Seat: Can People Distinguish between Automated and Manually Driven Vehicles?” Human Factors and Ergonomics in Manufacturing & Service Industries 30 (6): 418–425. doi:10.1002/hfm.20864.
- Stanton, N. A., and P. Marsden. 1996. “From Fly-by-Wire to Drive-by-Wire: Safety Implications of Automation in Vehicles.” Safety Science 24 (1): 35–49. doi:10.1016/S0925-7535(96)00067-7.
- Stanton, N. A., and M. Pinto. 2000. “Behavioural Compensation by Drivers of a Simulator When Using a Vision Enhancement System.” Ergonomics 43 (9): 1359–1370. doi:10.1080/001401300421806.
- Stanton, N. A., and P. M. Salmon. 2009. “Human Error Taxonomies Applied to Driving: A Generic Driver Error Taxonomy and Its Implications for Intelligent Transport Systems.” Safety Science 47 (2): 227–237. doi:10.1016/j.ssci.2008.03.006.
- Stanton, N. A., P. M. Salmon, G. H. Walker, E. Salas, and P. A. Hancock. 2017. “State-of-Science: Situation Awareness in Individuals, Teams and Systems.” Ergonomics 60 (4): 449–466. doi:10.1080/00140139.2017.1278796.
- Stanton, N. A., and M. S. Young. 2005. “Driver Behaviour with Adaptive Cruise Control.” Ergonomics 48 (10): 1294–1313. doi:10.1080/00140130500252990.
- Stanton, N. A., M. S. Young, G. H. Walker, H. Turner, and S. Randle. 2001. “Automating the Driver’s Control Tasks.” International Journal of Cognitive Ergonomics 5 (3): 221–236. doi:10.1207/S15327566IJCE0503_5.
- Wickens, C. D., A. Sebok, H. Li, N. Sarter, and A. M. Gacy. 2015. “Using Modelling and Simulation to Predict Operator Performance and Automation-Induced Complacency with Robotic Automation: A Case Study and Empirical Validation.” Human Factors 57 (6): 959–975. doi:10.1177/0018720814566454.
- Wiener, E. L., and R. E. Curry. 1980. “Flight-Deck Automation: Promises and Problems.” Ergonomics 23 (10): 995–1011. doi:10.1080/00140138008924809.
- Young, M. S., and G. F. Clynick. 2005. “A Test Flight for Malleable Attentional Resources Theory.” In Contemporary Ergonomics, edited by P. Bust and P. McCabe, vol. 2005, 548–552. London: Taylor & Francis.
- Young, M. S., and N. A. Stanton. 1997. “Automotive Automation: Investigating the Impact on Drivers’ Mental Workload.” International Journal of Cognitive Ergonomics 1 (4): 325–336.
- Young, M. S., and N. A. Stanton. 2002. “Malleable Attentional Resources Theory: A New Explanation for the Effects of Mental Underload on Performance.” Human Factors 44 (3): 365–375. doi:10.1518/0018720024497709.
- Young, M. S., N. A. Stanton, and D. Harris. 2007. “Driving Automation: learning from Aviation about Design Philosophies.” International Journal of Vehicle Design 45 (3): 323–338. doi:10.1504/IJVD.2007.014908.
- Young, M. S., and N. A. Stanton. 2023. Driving Automation: A Human Factors Perspective. Boca Raton, FL: CRC Press.