Abstract
Since the beginning of aeropalynology, aerobiologists have tried to develop models able to predict the pollen load of the atmosphere to help physicians and patients suffering pollen allergies to improve the efficiency of pollen allergies prophylaxis. Some models have been developed by aerobiological services and are used for real time predictions, now provided online. They are statistical models and require the pollen load of the previous days as input, as well as statistics on the pollen load of the previous years, daily meteorological data and geographic information on the pollen sources. Here we propose to use process-based phenological models to predict the time course of the pollen load of the atmosphere using solely daily mean temperatures as input. The model proposed has been fitted and validated for 13 major allergenic taxa (Alnus, Artemisia, Betula, Castanea, Corylus, Cupressaceae, Olea, Plantago, Platanus, Populus, Poaceae, Quercus and Tilia) in different areas of France and Spain. The model has been integrated into a freeware called PPF (Positive Pollen Forecast), which will be made available from the 5th Framework EU project POSITIVE web page at: http://www.forst.tu-muenchen.de/LST/METEOR/positive/.
The pollen sampler- and geographical data at observation sites.
Variance explained (R2) in the dates of flowering of each taxon in Bellaterra and Girona (Catalonia, Spain) by the Unified Model, and parameter estimates (20 years of observations).
Variance explained (R2) in the dates of flowering in Bellaterra and Girona (Catalonia, Spain) by the simplified models for each taxon.
Variance explained (R2) by the models in each region (R1-R9) and in all regions together (total).
Percentage of differentiation between the phenology of the different populations.
The socio-economic importance of allergies due to pollen has induced aerobiologists to look for predictive models of pollen load in the atmosphere (Citation Citation Citation Citation Citation Citation Citation Citation Citation Citation Citation Citation Citation Citation Citation Citation Citation CitationBoyer Citation1973, Bringfelt 1980, Bringfelt et al. 1982, Andersen 1991, Frenguelli et al. 1989, 1991; Larsson 1993, Pedersen & Moseholm 1993, Spieksma et al. 1995, Recio et al. 1997, Alba & Guardia 1998, Díaz de la Guardia et al. 1998, Iglesias et al. 1998, Alba et al. 2000, Bica et al. 2000, Galán et al. 1998, 2000, 2001; García-Mozo et al. 2000). Such models are intended to predict the time course and the level of pollen load in the atmosphere for coming weeks. These models can greatly increase the efficiency of the prophylaxis as physicians and patients can schedule more accurately their treatment against one particular pollen allergy. Models predicting the pollen load in the atmosphere of allergenic taxa currently in use for real time predictions for the public exist, but few of them have been published (Bica et al. Citation2000). They have been developed by aerobiological services, and they provide from one day or week to another the pollen load in the atmosphere of major allergenic plants of the country. These models use (1) biological parameters: the progress of the respective pollen season, i.e. the pollen load of the previous days, the long-term average peak day value, threshold values for low, moderate, and high pollen load, temperature threshold values for pollen release, and information about distance, direction and abundance of pollen sources; and (2) meteorological parameters: temperature, precipitation, relative humidity, wind turbulence, wind speed, wind direction, vertical air mass exchange (mixing depth), cloudiness, and overall conditions such as stability of weather patterns (Bica et al. Citation2000). The pollen load of the previous days is collected by local Pollen Networks. The collecting of pollen information has an enormous financial cost as the daily or weekly counting of pollen grains per pollen type must be done by palynologists.
Information provided to the public varies enormously from one Network to another, and is most developed in Europe. The European Pollen Information (EPI) network web site (http://www.cat.at/pollen/) provides maps of average pollen load of Alnus, Ambrosia, Artemisia, Betula, Corylus, Fraxinus, Cupressaceae, Oleaceae, Poaceae and Urticaceae every ten days during the pollen season of each taxon over Europe. These maps are created using pollen data gathered by the National Pollen Networks from Austria, Denmark, Italy, Finland, Germany, France, Netherlands, Poland, Spain, Sweden, Switzerland, and the United Kingdom. Not all of these national associations provide forecast of pollen load in their own country, but when they do, weekly coloured maps of the country with several (four to six) levels of pollen load (e.g. from “null” to “very important”) are provided for the most allergenic taxa.
So far, the recent advances in phenology modelling (Citation Citation Citation Citation CitationChuine Citation2000, Chuine et al. 1999, Häkkinen 1999, Chuine et al. 1998, Hänninen 1995, Kramer 1994) have not been applied to the modelling of pollen load in the atmosphere. These models could however provide a substantial improvement of the methodology. These models indeed predict flowering or growth initiation time using solely temperature data as an input variable. Their accuracy has been extensively tested (Citation Citation Citation Citation Citation Citation CitationChuine et al. Citation2000 a, b, Chuine et al. 1999, Chuine & Cour 1999, Häkkinen 1999, Chuine et al. 1998, Hänninen 1995, Kramer 1994), and they have been shown to be a useful tool to predict plant phenology under global warming scenarios (Osborne et al. Citation2000). They can thus be adapted to predict the time course of the pollen load over the year, which is equivalent to the time course of the number of individuals in bloom in a particular area. Such models cannot predict the absolute pollen concentration in the atmosphere of a particular taxon, because this requires information on the pollen load of the previous days and previous years as well as information on the geographic origin and intensity of the pollen sources. However, as the current pollen forecasting models do, they can provide a relative index of pollen load.
The aim of this study was thus to adapt process-based phenological models for the prediction of the relative pollen load of the most allergenic taxa in Europe except Urticaceae (Alnus, Artemisia, Betula, Castanea, Corylus, Cupressaceae, Olea, Plantago, Platanus, Populus, Poaceae, Quercus and Tilia), using solely daily temperatures. We were unable to provide a model for Urticaceae because in the countries studied Urticaceae pollen is present almost all the year round in the atmosphere so that peaks of pollen or beginning of the pollen season could not be identified with certainty. This study also allowed for the development of a freeware called Positive Pollen Forecast, made available as a freeware at the web site of the European Program POSITIVE (http://www.forst.tu-muenchen.de/LST/METEOR/positive/). This software has been developed to help physicians and the public to control pollen allergies by forecasting the right time to respectively prescribe and take adequate medicine.
MATERIAL AND METHODS
Datasets
We selected thirteen allergenic taxa on the basis of their degree of harmful effect on human health and proportion of people suffering from allergy due to these taxa (D'Amato et al. Citation1991). These taxa are Alnus (alder), Betula (birch), Castanea (chestnut), Corylus (hazelnut) Cupressaceae (cypress family), Olea (olive), Populus (poplar), Platanus (plane tree), Artemisia (mugwort), Plantago (plantain), Poaceae (grasses), Quercus (oak) and Tilia (linden).
We used pollen data for these taxa from Spain (17 stations), France (10 stations), Austria (1 station) and Poland (1 station). The time series varied between stations from 2 to 23 years, with an average of 7 years (, ). Pollen data were collected with either Cour (weekly data; Cour Citation1974) or Hirst (daily data; Hirst Citation1952) traps. A recent study by Belmonte et al. Citation2000 indeed shows that peaks (the item used in the present paper) are registered in the same week of the year in both methods. We also compiled daily mean temperature data for each station during the period of pollen record. In France and Spain the national meteorological services provided the temperature data. In France the aerobiological stations are always placed beside a meteorological station. In Spain, this is not always the case, but the meteorological data always comes from the nearest meteorological station. The temperature data used was the daily arithmetic mean between daily minimum and maximum temperatures.
Location of the 29 pollen- and associated meteorological stations used in the study (see for the correspondence between station codes and names, and geographical data).
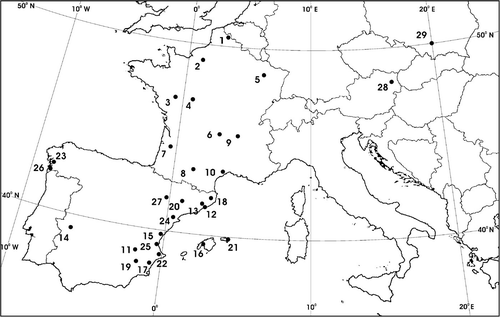
For each taxon, except Poaceae and Plantago, and in each station, we determined the date of peak of pollen concentration (day of maximum pollen concentration). This date represents the date when the majority of the plants are in full bloom around the pollen station. The phenological models will be used to predict this date as well as the time course of the pollen concentration along the year. For some taxa, it is impossible to determine this date because several peaks of pollen exist and do not occur in the same order every year. This is usually the case of herbaceous taxa because several species contribute to the pollen load. This was the case here for Poaceae and Plantago for which we determined the date of beginning of pollen shedding instead of peak of pollen shedding. The date of beginning of pollen shedding was determined by the date when the 7-day running mean value exceeded 1% of the annual total for the Hirst data (Frenguelli et al. Citation1989) and by the middle day of the week during which pollen concentration exceeded 1% of the annual total for the Cour data.
Development of models for tree taxa
Several process-based phenological models have been developed for trees since the seventies (Citation Citation Citation Citation Citation CitationLandsberg Citation1974, Sarvas 1974, Cannell & Smith 1983, Murray et al. 1989, Hänninen 1990, Kramer 1994, Hunter & Lechowicz 1992). Their assumptions are deeply rooted in experimental results on the response of phenology to various environmental variables and they can allow large spatial and temporal scale predictions even though fitted at a local scale (Chuine et al. 2000). They have been used to predict the dates of peak of pollen shedding of tree taxa (Citation Citation CitationBoyer Citation1973, Chuine et al. 1998, 1999; Chuine 2000). Among these models, the Unified Model (Chuine Citation2000) was used here to select the best model for each tree taxa considered in this study. This model has nine parameters and can be simplified for each particular taxon by relaxing some hypotheses of the model after examining the parameter estimates (see Chuine Citation2000). It was first fitted for each taxon to the Spanish data set of Bellaterra and Girona (Catalonia, Spain) using the least-square method and the simulated-annealing algorithm Metropolis (Chuine et al. Citation1998).
Variables used in the different models presented in this study
tf – date of flowering
t0 – 1st January
Sf (t) – state of development at day t
Rf, t – rate of development of at day t
Tt – mean daily temperature
Nt – daily night length
Unified Model (ac, bc, cc, df, ef, w, z, C, tc)
tf such as Sf (tf )=F with
t1 such as Sc (t1 ) =C with
The analysis of the parameter estimates allowed for a simplification of the model assumptions for each species. The simplified model was then fitted to the same Spanish dataset of Bellaterra and Girona (Catalonia both), and then tested by cross-validation against the Spanish dataset of Barcelona (Catalonia) to check the consistency of the model fit. Cross-validation is an efficient way of testing the predictive power of phenological models (Chuine et al. Citation1998).
Development of models for herbaceous taxa
To date, few phenology models for herbaceous species phenology exist (Citation Citation Citation Citation Citation Citation CitationBringfelt et al. Citation1982, Frenguelli et al. 1989, Iglesias et al. 1998, Larsson 1993, Pipper et al. 1996, Seddigh & Jolliff 1994, Sinclair et al. 1991, Subiza et al. 1992). Most of them are statistical models, predicting flowering dates or periods of pollen shedding using correlations with climatic variables, such as mean temperature of March and April (Citation CitationBringfelt et al. Citation1982, Frenguelli et al. 1989, Larsson 1993). Few of them are process-based models like phenological models developed for tree taxa, (Citation CitationPipper et al. Citation1996, Seddigh & Jolliff 1994, Sinclair et al. 1991). Two of these latter are based on an experimental background on soybean phenology (CitationPipper et al. Citation1996, Sinclair et al. 1991).
We first tried the statistical model of Frenguelli et al. (Citation1989) developed for Poaceae. This model is a correlation between dates of peak of pollen shedding or date of beginning of pollen shedding and the mean temperature of March–April. Predictions obtained with this model, when applied to our dataset, were very weak and inconsistent from one location to another. We thus tried two process-based models (Model 1 & Model 2) based on Pipper et al. (Citation1996) and Sinclair et al. (Citation1991).
Model 1 (F, a, b, c, d)
tf such as Sf (tf )=F with
Model 2 (F, e, f, g, h, k)
tf such as Sf (tf ) F with
Model 1 and Model 2 were fitted to the dates of peak of pollen shedding of Artemisia and the dates of beginning of pollen shedding of Plantago and Poaceae in Barcelona, Bellaterra and Girona. As Model 1 and Model 2 are process-based, the percentage of variance explained can be negative, meaning that the models are even worse than the Null Model, i.e. the mean date of peak or beginning of pollen shedding. This was the case for Artemisia whichever the model was used and for Plantago with Model 2. Otherwise, percentages of variance explained by the models were 0.349 (Model 1) for Plantago and 0.359 (Model 1) and 0.149 (Model 2) for Poaceae. None of them were significant. As the model goodness of fit was very low, other models were developed.
The Unified Model, initially developed for tree taxa (Chuine Citation2000) was fitted to the same data set as previously (Barcelona, Bellaterra, Girona) and then simplified according to the parameter estimates. The Unified Model developed for trees considers two phases of development of the buds: the phase of dormancy and the phase of quiescence. The phase of dormancy does not exist in annual plants and we should therefore obtain a simplification of the model for Poaceae with only one phase left, the phase of quiescence. However, most of the Spanish and French species of Artemisia and Plantago are perennials [25 out of 26 for Artemisia (Tutin et al. Citation1976) and 20 out of 26 for Plantago (Chater & Cartier Citation1976)]. The phase of dormancy may thus be critical in this case. Some Artemisia species are woody or shrub species and thus behave as trees regarding their phenology. Phenological models developed for trees could therefore apply to these taxa.
Estimation of genetic differentiation of flowering phenology among populations
Phenology is a highly selected trait and the response of phenology to climate of different populations of tree may differ significantly (Chuine et al. 2000). In such cases, parameter estimates derived from observations of a single population may not be accurate to predict the phenology of other populations. Genetic differentiation must be estimated and in case it is significant, different parameter estimates must be used in the different regions. For each taxon we thus estimated and tested the genetic differentiation of phenology as follows. We defined for each taxon different populations by grouping stations together on the base of their geographic origin ( ). Genetic differentiation among populations was estimated for each taxon by fitting the best model found for each of them to the dates of flowering of each population (i.e. each group of locations; see Appendix) and of all populations all together (Chuine et al. 2000).
RESULTS
Models for tree taxa
shows the variance explained in the dates of peak of pollen shedding in Catalonia (NE Spain) by the Unified Model as well as the parameter estimates for each taxon. The parameter estimates showed that the Unified Model could be simplified in three different models depending on the taxa considered: UniForc (UF), UniChill (UC) or Spring Warming (SW) Model (see Chuine et al. 2000).
UniForc Model (t1, df, ef, F)
tf such as Sf (tf )=F with
UniChill Model (ac, bc, cc, df, ef, C, F)
tf such as Sf (tf )=F with
t1 such as Sc (t1 )=C with
Spring Warming Model (tb, Tb, F)
tf such as Sf (tf )=F with
Rf,t =max(0,Tt−Tb )
shows the model selected for each taxon and the variance it explains in the dates of peak of pollen shedding in Bellaterra and Girona. Most of the variances explained are significant and some are highly significant (e.g. Olea, Platanus, Populus, Tilia).
Models for herbaceous taxa
The variance explained by the Unified Model in the dates of beginning or peak of pollen shedding of Artemisia, Plantago, and Poaceae were 0.426, 0.592 and 0.475 respectively, but they were not significant (). These explained variances were however on average higher than these obtained with Model 1 (Sinclair et al. Citation1991) or Model 2 (Pipper et al. Citation1996). The analysis of the parameter estimates () allowed for a model simplification for each taxon as follows: Photoperiod Model for Artemisia and UniForc Model for Poaceae and Plantago (see ).
Photoperiod Model (ac, bc, cc, C, F)
tf such as Sf (tf )=F with
Rf,t =1
t1 such as Sc (t1 )=C with
Genetic differentiation of phenology among populations
Tree taxa
shows the variance explained by the models in the dates of peak of pollen shedding in each region, and shows the results of the test of genetic differentiation among populations. Some taxa showed little differentiation although sometimes significant (Platanus), but usually not (Tilia). Other taxa showed higher and significant differentiation (Cupressaceae, Populus, Olea) and even highly significant differentiation (Quercus, Corylus, Betula, Alnus). Among these, some had complex patterns of differentiation with close populations being significantly differentiated (Quercus, Betula, Populus). These patterns of differentiation are consistent with the taxonomic complexity of each taxon (one vs several species contributing to the pollen load) or the complexity of the species distributions (the complex of species contributing to the pollen load differs from one location to another).
In conclusion, for tree taxa, genetic differentiation is not always significant among populations depending on the taxa considered. However, we recommend the use, whenever possible of different parameter estimates for each region to increase the accuracy of the predictions. Estimates of the genetic differentiation give an idea of how accurate predictions obtained for a region (which is far from that where parameter estimates come from) could be.
Herbaceous taxa
The overall genetic differentiation is similar for the three herbaceous taxa (between 24% and 27%) and is significant. This overall differentiation is mainly due to a differentiation between French and Spanish populations for the three taxa, and also to a differentiation among the Spanish populations for Plantago and Poaceae. The strongest differentiation is shown for Artemisia with an overall differentiation of 27% and a differentiation between French and Spanish populations of 25%. This is probably due to the fact that France and Spain share less Artemisia species than Plantago species. Among the 26 species of Artemisia either present in France or Spain, twelve are present in both countries, four are present only in Spain and ten are present only in France (Tutin et al. Citation1976). Among the 26 species of Plantago either present in France or Spain, 18 are present in both countries, seven are present only in Spain and one is present only in France (Chater & Cartier Citation1976). However, genetic differentiation is less structured on average than that of tree taxa because of the mixing of several species within each genus.
Cross-validation of the models
Phenological models were tested by cross-validation, except for Artemisia, to allow an estimation of the accuracy of predictions in each area ( ). The cross-validations for Artemisia are not shown because they were inaccurate presumably due to different complexes of species in each area. This is supported by the observed dates of peak of pollen shedding of Artemisia and start of pollen shedding of Plantago across a latitudinal gradient (). The dates of flowering of Plantago show a linear relationship with latitude, although different in France and Spain, suggesting that the two countries are characterized by different complexes of species. On the contrary, the dates of flowering of Artemisia do not show such a linear relationship with latitude. Moreover, the amplitude of variation within a location suggests that the pollen peak in some years is due to a particular species and some other years due to another species and not necessarily the same species from one location to another. This raises the principal drawback of pollen data, which is that pollen from different species within the same genus cannot morphologically be distinguished.
Results of cross-validation test: predictions in different Spanish locations not included in the model fit using parameter estimates of the closest population. A. Alnus; B. Betula; C. Castanea; D. Corylus; E. Olea; F. Platanus; G. Populus; H. Quercus; I. Tilia; J. Cupressaceae; K. Plantago; L. Poaceae. Dates are in day of the year. The dashed strait line is the bisector (100% matching between predictions and observations).
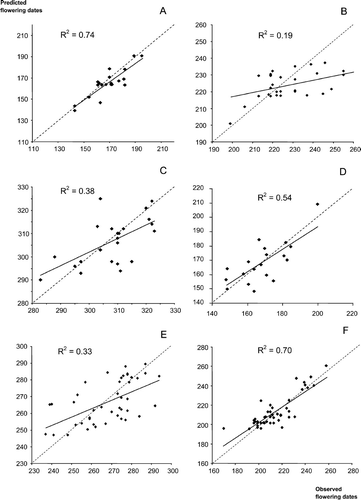
Variations in the dates of peak of pollen load of Artemisia (A) and in the beginning of pollen shedding of Plantago (B) with latitude. Dates are expressed in day of the year (DOY). Diamonds are the French locations and squares are the Spanish locations. Several years per location are plotted to show the among year variation.
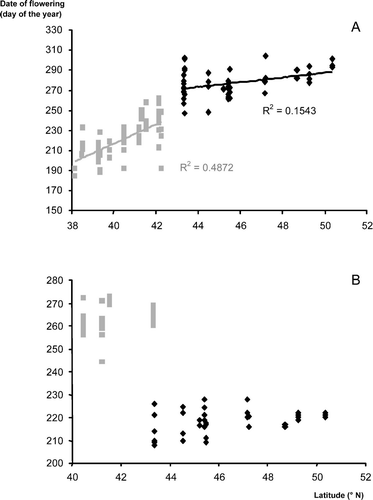
The results of the cross-validation show a great variance in the accuracy of prediction by cross-validation, i.e. ability to predict flowering dates for locations not taken into account in the model fit. For some taxa, the accuracy of predictions is low (Cupressaceae, Poaceae, Quercus and Betula), whereas it is very high for some other taxa (Alnus, Corylus, Platanus, and Plantago). The UniForc Model predictions were very accurate for Plantago (R2=0.85), but less so for Poaceae (R2=0.54). This is particularly striking considering that several species actually contribute to the pollen load of these taxa, and also encouraging as these two taxa represent a large part of the allergies among the public.
We also tested whether parameter estimates from French and Spanish regions could provide accurate predictions in other countries. For this task, some data was made available by the National Pollen Networks of Austria (1 station) and Poland (1 station). The predictions obtained for Vienna (Austria) included Alnus, Betula, Platanus and Populus, and for Krakow (Poland) included Alnus and Betula (). Predictions for other taxa are not shown either because the taxon does not exist in this area or because pollen concentrations were too low to estimate a date of peak of pollen shedding (in Krakow: Olea, Platanus, Tilia, Corylus and Cupressaceae; in Vienna: Olea) or because they were not significant (except for Corylus in Vienna, R2=0.50). Very accurate predictions were obtained for Alnus, Betula, Populus, Platanus and Corylus in Vienna using the parameter estimates from Lyon (France) and for Alnus and Betula in Krakow using the parameter estimates from northern France. These regions are indeed the closest ones to Vienna and Krakow respectively, for which we have parameter estimates. These predictions are consistent with these obtained in Spain by cross-validation except for Betula, Poaceae and Plantago, i.e. models which provide the most accurate predictions within a country are those which provide the most accurate predictions abroad. Predictions for Betula were not very accurate in Spain whereas they were in Austria and Poland, probably because birch populations are more abundant and dense in these countries so that pollen data may be more reliable. On the contrary, predictions for Poaceae and Plantago were accurate in Spain, but not in Austria and Poland probably because genetic differentiation between populations from France and Austria or Poland is more important compared to the genetic differentiation among populations within Spain.
Comparison of predictions of flowering dates (peak of pollen shedding, in day of the year) of Alnus (A), Betula (B), Populus (C) and Platanus (D) in Vienna (Austria) using for each of them parameter estimates from Lyon (France); and of Alnus (E) and Betula (F) in Krakow (Poland) using parameter estimates from northern France, with their respective observed flowering date.
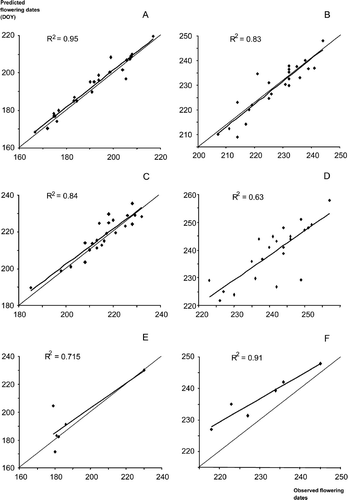
Prediction of the time course of pollen load
Phenological models provide dates of flowering of individuals or populations. In this study, they provide the dates of peak of pollen load. Absolute pollen load requires lots of parameters to be predicted, especially the pollen load of the previous days and information on the location and intensity of the pollen sources. For simplicity, we chose to predict a relative pollen load (PL). Up to the critical state of development F, parameter in every model, the relative pollen load can be calculated as the product of a specific parameter and the relative state of development:
The day when the pollen load reaches its maximum, i.e. 1, is the day when the majority of the plants of the taxon living around are in bloom. As the number of plants in bloom within a population usually follows a normal distribution centred on the day of peak of pollen load, we estimated the decreasing phase of the pollen load by symmetry with the ascending phase.The prediction of the timing of pollen load provides one peak of pollen. shows the prediction of the relative pollen load in Lyon (France) in 1982 for Castanea, Quercus, and Platanus, and in Bellaterra (Spain) in 1997 for Platanus, Olea and Populus. However, for some taxa (e.g. Cupressaceae) a second peak of pollen load occurs later in the season. Predicting subsequent pollen peaks is possible if they are distinct from each other, and appear in the same order every year, which is rarely the case. We have usually no way to know which species contribute to the first, second, third (sometimes more) peak in one particular year except if we observe the phenology of each species in natural populations for several years.
Prediction of the relative pollen load in Lyon (France) in 1982 for Castanea (A), Quercus (B), and Platanus (C), and in Bellaterra (Spain) in 1997 for Platanus (D), Olea (E) and Populus (F). Diamonds are the observed pollen load and grey curves are the predictions obtained with the Corresponding Model for each taxon.
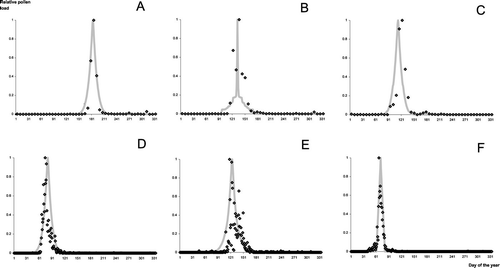
DISCUSSION
Prediction accuracy varied substantially from one taxon to another. Models developed for Betula and Quercus led to the least accurate predictions, presumably for two different reasons. Many species of these two taxa occur together in Spain and France, and different Quercus species may contribute to the pollen load in a particular region. Since phenology is taxon specific, it is difficult to derive consistent parameter estimates for different regions, as we do not know which species contributes most to the pollen peak each year. This is proven by the variation in the variance explained by the UniChill Model in the dates of flowering of Quercus with the number of Quercus species living in each area (r=−0.913). Predictions for Betula were accurate in France, Austria and Poland, but not in Spain. In Spain, only two wild Betula species occur (B. pendula and B. pubescens Walters Citation1964), and they occur in highlands, but birch trees are used as ornamentals in most of the country. The temperature data we used was recorded at the pollen stations, which are located in the lowlands, thus they are not the temperatures that birch trees grow under in the highlands.
Cross-validation tests and tests of genetic differentiation show that the use of parameter estimates of a particular region or location may not always be accurate to make predictions in another region or location. We have thus provided parameter estimates for as many regions as possible for each taxon, covering France and Spain. We have shown that accurate predictions could be obtained outside these countries, but the use of the European Pollen Information database could allow for accurate predictions of the time course of pollen loads anywhere in Europe.
The positive results we obtained, encouraged us to disseminate them to potential users who would like to predictions get the timing of pollen release of allergenic plants. We developed a Microsoft® Windows oriented freeware called PPF (Positive Pollen Forecast), of which version 1.0 is available in French, German, English and Spanish, and it can be downloaded from the web page of POSITIVE (www.forst.tu-muenchen.de/LST/METEOR/positive/). PPF is designed in such a way that anyone can obtain predictions of the relative pollen load of any of the thirteen taxa studied, providing only daily temperatures as input.
CONCLUSIONS
Our results show that simple, but process-based, models can predict accurately the timing of pollen release for many allergenic taxa using solely mean daily temperature data. Difficulties essentially come from the genetic differentiation of phenology among species and populations. The differences in the responses of phenology to temperature between populations within a species or between species within a genus or between genera within a family make the fit of the model parameters difficult. Despite these difficulties, prediction accuracy is shown to be consistent in most cases. The problem of genetic differentiation among populations remains manageable when parameter estimates can be obtained for several regions within the species distribution.
ACKNOWLEDGEMENTS
The authors are particularly grateful to Prof. S. Jäger, and Dr D. Myszkowska, who have kindly provided pollen date from Vienna and Krakow respectively, and to W. Lipa (ZAMG) for providing temperature data from Vienna and Krakow. Pollen data coming from Spain have been funded by a research project supported by Laboratorios CBF-LETI, S.A. This study was supported by the EU project POSITIVE, EVK2-1999-00109.
REFERENCES
- Alba , F. and Diaz de la Guardia , C. 1998 . The effect of air temperature on the starting dates of the Ulmus, Platanus and Olea pollen seasons in the SE Iberian Peninsula. . – Aerobiologia , 14 : 191 – 194 .
- Alba , F. , Diaz de la Guardia , C. and Comtois , P. 2000 . The effect of meteorological parameters on diurnal patterns of airborne olive pollen concentration. . – Grana , 39 : 200 – 208 .
- Andersen , T. B. 1991 . A model to predict the beginning of the pollen season. . – Grana , 30 : 269 – 275 .
- Belmonte , J. , Canela , M. and Guàrdia , R. A. 2000 . Comparison between categorical pollen data obtained by Hirst and Cour sampling methods. . – Aerobiologia , 16 : 177 – 185 .
- Bica H. Gmoser H. Jäger S. 2000 An automated pollen forecast model based on synoptical and biological observations. – Present. 2nd Eur. Symp. Aerobiol., Vienna 2000
- Boyer , W. D. 1973 . Air temperature, heat sums, and pollen shedding phenology of longleaf pine. . – Ecology , 54 : 421 – 425 .
- Bringfelt B. 1980 Studies of pollen concentration in Stockholm and weather data. – In: 1st Int. Conf. Aerobiol. München 1978. Proc. (ed. A. W. Frankland, E. Stix & H. Ziegler), pp. 14–21. – UBA Ber. 5. E. Schmidt, Berlin
- Bringfelt , B. , Engström , I. and Nilsson , S. 1982 . An evaluation of some models to predict airborne pollen concentration from meteorological conditions in Stockholm, Sweden. . – Grana , 21 : 59 – 64 .
- Cannell , M. G. R. and Smith , R. I. 1983 . Thermal time, chill days and prediction of budburst in Picea sitchensis. . – J. Appl. Ecol. , 20 : 951 – 963 .
- Chater A. O. Cartier D. 1976 Plantago L. – In: Flora Europaea. Vol. 4 (ed. T. G. Tutin et Coll.), pp. 38–44. – Cambridge Univ. Press, Cambridge
- Chuine , I. 2000 . A unified model for the budburst of trees. . – J. Theor. Biol. , 207 : 337 – 347 .
- Chuine , I. and Cour , P. 1999 . Climatic determinants of budburst seasonality in four temperate-zone tree species. . – N. Phytol. , 143 : 339 – 349 .
- Chuine , I. , Cour , P. and Rousseau , D. D. 1998 . Fitting models predicting dates of flowering of temperate-zone trees using simulated annealing. . – Plant, Cell & Environ. , 21 : 455 – 466 .
- Chuine , I. , Cour , P. and Rousseau , D. D. 1999 . Selecting models to predict the timing of flowering of temperate trees: implication for tree phenology modelling. . – Plant, Cell & Environ. , 22 : 1 – 13 .
- Chuine , I. , Cambon , G. and Comtois , P. 2000 a . Scaling phenology from the local to the regional level: advances from species-specific phenological models. . – Glob. Change Biol. , 6 : 943 – 952 .
- Chuine , I. , Mignot , A. and Belmonte , J. 2000 b . A modelling analysis of the genetic variation of phenology between tree populations. . – J. Ecol. , 88 : 1 – 12 .
- Cour , P. 1974 . Nouvelles techniques de détection des flux et des retombées polliniques. Etude de la sédimentation des pollens et des spores à la surface du sol. . – Pollen Spores , 16 : 103 – 141 .
- D'Amato G. Spieksma F. T. H. Bonini S. 1991 Allergenic pollen and pollinosis in Europe. – Blackwell Sci. Publ., Oxford
- Díaz de la Guardia , C. , Alba , F. and Sabariego , S. 1998 . An aerobiological study of Urticaceae pollen in the city of Granada (S. Spain): correlation with meteorological parameters. . – Grana , 37 : 298 – 304 .
- Frenguelli , G. , Bricchi , E. , Romano , B. , Mincigriucci , G. and Spieksma , F. T. M. 1989 . A predictive study on the beginning of pollen season for Gramineae and Olea europaea L. . – Aerobiologia , 5 : 64 – 70 .
- Frenguelli , G. , Spieksma , T. M. , Bricchi , E. , Romano , B. , Mincigriucci , G. , Nikkels , A. H. , Dankaart , W. and Ferranti , F. 1991 . The influence of air temperature on the starting dates of the pollen season of Alnus and Populus. . – Grana , 30 : 196 – 200 .
- Galán , C. , Fuillerat , M. J. , Comtois , P. and Dominguez , E. 1998 . A predictive study of Cupressaceae pollen season onset, severity, maximum value and maximum value date. . – Aerobiologia , 14 : 195 – 199 .
- Galán , C. , Alcazar , P. , Carinanos , P. , Garcia , H. and Dominguez-Vilches , E. 2000 . Meteorological factors affecting daily Urticaceae pollen counts in southwest Spain. . – Int. J. Biometerol. , 43 : 191 – 195 .
- Galán , C. , Garcia-Mozo , H. , Carinanos , P. , Alcazar , P. and Dominguez-Vilches , E. 2001 . The role of temperature in the onset of the Olea europaea L. pollen season in southwestern Spain. . – Int. J. Biometeorol. , 45 : 8 – 12 .
- García-Mozo , H. , Gálan , C. , Gomez-Casero , M. T. and Dominguez , E. 2000 . A comparative study of different temperature accumulation methods for predicting the start of the Quercus pollen season in Cordoba (South West Spain). . – Grana , 39 : 194 – 199 .
- Häkkinen , R. 1999 . Statistical evaluation of bud development theories: application to bud burst of Betula pendula leaves. . – Tree Physiol. , 19 : 613 – 618 .
- Hänninen , H. 1990 . Modelling bud dormancy release in trees from cool and temperate regions. . – Acta Forest. Fenn. , 213 : 1 – 47 .
- Hänninen , H. 1995 . Effects of climatic change on trees from cool and temperate regions: an ecophysiological approach to modelling of budburst phenology. . – Can. J. Bot. , 73 : 183 – 199 .
- Hirst , J. M. 1952 . An automatic volumetric spore trap. . – Ann. Appl. Biol. , 39 : 257 – 265 .
- Hunter , A. F. and Lechowicz , M. J. 1992 . Predicting the timing of budburst in temperate trees. . – J. Appl. Ecol. , 29 : 597 – 604 .
- Iglesias , I. , Aira , M. J. and Jato , V. 1998 . Poaceae pollen in the atmosphere of Santiago de Compostela: its relationship with meteorology. . – Aerobiologia , 14 : 141 – 145 .
- Kramer , K. 1994 . Selecting a model to predict the onset of growth of Fagus sylvatica. . – J. Appl. Ecol. , 31 : 172 – 181 .
- Landsberg , J. J. 1974 . Apple fruit bud development and growth; analysis and an empirical model. . – Ann. Bot. , 38 : 1013 – 1023 .
- Larsson , K.-A. 1993 . Prediction of the pollen season with a cumulated activity method. . – Grana , 32 : 111 – 114 .
- Murray , M. B. , Cannell , M. G. R. and Smith , R. I. 1989 . Date of budburst of fifteen tree species in Britain following climatic warming. . – J. Appl. Ecol. , 26 : 693 – 700 .
- Osborne , C. P. , Chuine , I. , Viner , D. and Woodward , F. I. 2000 . Olive phenology as a sensitive indicator of future climatic warming in the Mediterranean. . – Plant, Cell & Environ. , 23 : 701 – 710 .
- Pedersen , B. V. and Moseholm , L. 1993 . Precision of the daily pollen count. Identifying sources of variation using variance component models. . – Aerobiologia , 9 : 15 – 26 .
- Pipper , E. L. , Boote , K. L. , Jones , J. W. and Grimm , S. S. 1996 . Comparison of two phenology models for predicting flowering and maturity date of soybean. . – Crop Sci. , 36 : 1606 – 1614 .
- Recio , M. , Cabezudo , B. , Trigo , M. M. and Toro , F. J. 1997 . Accumulative air temperature as a predicting parameter for daily airborne olive pollen (Olea europaea L.) during the pre-peak period in Malaga (Western Mediterranean area). . – Grana , 36 : 44 – 48 .
- Sarvas , R. 1974 . Investigations on the annual cycle of development of forest trees. Autumn dormancy and winter dormancy. . – Communic. Inst. Forest. Fenn. , 84 : 1 – 101 .
- Seddigh , M. and Jolliff , G. D. 1994 . Pre-induction age and post-induction temperature effects on Meadow foam flowering. . – Crop Sci. , 24 : 503 – 508 .
- Sinclair , T. R. , Kitani , S. , Bruniard , J. and Horide , T. 1991 . Soybean flowering date: linear and logistic models based on temperature and photoperiod. . – Crop Sci. , 31 : 786 – 790 .
- Spieksma , F. T. H. , Emberlin , J. , Hjelmroos , M. , Jäger , S. and Leuschner , R. M. 1995 . Atmospheric birch (Betula) pollen in Europe: trends and fluctuations in annual quantities and the starting dates of the seasons. . – Grana , 34 : 51 – 57 .
- Subiza , J. , Masiello , M. , Subiza , J. L. , Jerez , M. , Hinojosa , M. and Subiza , E. 1992 . Prediction of annual variations in atmospheric concentrations of grass pollen. A method based on meteorological factors and grain crop estimates. . – Clin. Exp. Allergy , 22 : 540 – 546 .
- Tutin T. G. Peterson K. Gutermann W. 1976 Artemisia L. – In: Flora Europaea. Vol. 4 (ed. T. G. Tutin et Coll.), pp. 178–186. – Cambridge Univ. Press, Cambridge
- Walters S. M. 1964 Betula L. – In: Flora Europaea. Vol. 4 (ed. T. G. Tutin et Coll.), pp. 57–58. – Cambridge Univ. Press, Cambridge
APPENDIX
Pollen stations in the different geographical regions defined for each taxon
Alnus
R1- Lille, Rouen, Angers, Tours, Nancy
R2- Clermont-Ferrand, Bordeaux, Toulouse
R3- Montpellier
R4- Lyon
R5- Bellatera, Girona
R6- Vigo, Pontevedra
R7- Zaragoza, Lleida
R8- Caceres
R9- Vienna
R10- Krakow
Artemisia
R1- Clermont-Ferrand, Bordeaux, Toulouse
R2- Montpellier
R3- Lyon
R4- Barcelona, Bellaterra
R5- Roquetes
R6- Vienna
R7- Krakow
Betula
R1- Lille, Rouen, Angers, Tours, Nancy
R2- Clermont-Ferrand, Bordeaux, Toulouse
R3- Lyon
R4- Bellatera, Girona
R5- Zaragoza, Lleida
R6- Castello de la Plana, Valencia
R7- Caceres
R8- Vigo, Pontevedra
R9- Vienna
R10- Krakow
Castanea
R1- Lille, Rouen, Angers, Tours, Nancy
R2- Clermont-Ferrand, Bordeaux, Toulouse
R3- Lyon
R4- Bellatera, Girona
R5- Vigo, Pontevedra
R6- Vienna
Corylus
R1- Lille, Rouen, Angers, Tours, Nancy
R2- Clermont-Ferrand, Bordeaux, Toulouse
R3- Lyon
R4- Bellatera, Girona
R5- Zaragoza, Lleida
R6- Vigo, Pontevedra
R7- Caceres
R8- Vienna
Cupressaceae
R1- Lille, Rouen, Angers, Tours, Nancy
R2- Clermont-Ferrand, Bordeaux, Toulouse
R3- Lyon
R4- Montpellier
R5- Barcelona, Bellatera, Girona
R6- Caceres
R7- Pontevedra, Vigo
Olea
R1- Montpellier
R2- Bellatera, Girona
R3- Roquetes
R4- Castello de la Plana, Valencia
R5- Albacete, Pego, Elx
Plantago
R1- Lille, Rouen, Angers, Tours, Nancy
R2- Lyon
R3- Clermont-Ferrand, Bordeaux, Toulouse
R4- Montpellier
R5- Barcelona, Bellatera, Girona
R6- Roquetes
R7- Albacete, Hellin, Pego, Elx
R8- Ciutat de Palma, Mao
R9- Caceres
R10- Pontevedra, Vigo
R11- Vienna
R12- Krakow
Platanus
R1- Lille, Rouen, Angers, Tours, Nancy
R2- Clermont-Ferrand, Bordeaux, Toulouse
R3- Montpellier
R4- Lyon
R5- Bellatera, Girona
R6- Zaragoza, Lleida
R7- Castello de la Plana, Valencia
R8- Vigo, Pontevedra
R9- Caceres
Poacae
R1- Lille, Rouen, Angers, Tours, Nancy
R2- Lyon
R3- Clermont-Ferrand, Bordeaux, Toulouse
R4- Montpellier
R5- Barcelona, Bellaterra, Girona
R6- Roquetes
R7- Albacete, Hellin, Pego, Elx
R8- Ciutat de Palma, Mao
R9- Caceres
R10- Pontevedra, Vigo
R11- Vienna
R12- Krakow
Populus
R1- Lille, Rouen, Angers, Tours, Nancy
R2- Clermont-Ferrand, Bordeaux, Toulouse
R3- Montpellier
R4- Lyon
R5- Bellatera, Girona
R6- Caceres
R7- Roquetes
R8- Vienna
R9- Krakow
Quercus
R1- Lille, Rouen, Angers, Tours, Nancy
R2- Clermont-Ferrand, Bordeaux, Toulouse
R3- Montpellier
R4- Lyon
R5- Bellatera, Girona
R6- Zaragoza, Lleida
R7- Castello de la Plana, Valencia
R8- Vigo, Pontevedra
R9- Caceres
R10- Vienna
R11- Krakow
Tilia
R1- Lille, Rouen, Angers, Tours, Nancy
R2- Clermont-Ferrand, Bordeaux, Toulouse
R3- Lyon
R4- Bellatera, Girona
R5- Zaragoza, Lleida
R6- Vigo, Pontevedra