Abstract
With most inventory theory investigating linear models, the dynamics of nonlinear inventory systems is not well understood. We explore the dynamics of the order-up-to policy under lost-sales for the case of i.i.d. normally distributed demand and unit lead times. We consider the ideal minimum mean-squared error forecast and two alternative scenarios: partial demand observation and dynamic demand forecasting, providing a broad understanding of the operational performance of lost-sales systems. In each scenario, we obtain analytical expressions for the order, inventory, and satisfied demand distributions. This allows us to quantify the Bullwhip and inventory variance amplification ratios as well as the fill rate and the inventory cover. We show the lost sales nonlinearity induces complex behaviours in inventory systems. Interestingly, lost sales smooth supply chain dynamics, significantly affecting the trade-off between service level and average inventory holding. We also reveal the inventory downsides of demand censoring and the production damages induced by dynamic forecasts. We identify a key parameter, the relative safety margin, that characterises the performance of lost-sales systems. We finish by offering some prescriptive results for the optimal safety stock and capacity level in both a retail and a manufacturing lost-sales setting.
Acknowledgements
An earlier version of this manuscript was presented on 30 August 2019 in Berlin (Germany) at the 9th IFAC/IFIP/IFORS/IISE/INFORMS Conference on Manufacturing Modelling, Management and Control MIM 2019, where it received a Commended Paper Award. Please refer to Disney, Ponte, and Wang (Citation2019).
Disclosure statement
No potential conflict of interest was reported by the author(s).
Notes
1 Indeed, meaningful interactions emerge between inventory and order variability, leading to a key concept in this area, the ‘variability trade-off’ (Disney, Towill, and de Velde Citation2004; Priore et al. Citation2019).
2 Due to the complexity of the analysis involved, simulation is the most common approach to explore nonlinear supply chains. Previous research efforts have also provided a fair understanding of other nonlinearities via simulation techniques, such as Ponte et al. (Citation2017) for capacity constraints (an upper limit on orders) and Chatfield and Pritchard (Citation2013) for forbidden returns (non-negative orders).
3 We highlight the Lean wastes of mura and muri are closely related to the Bullwhip effect.
4 In lost-sales systems the definition simplifies to the proportion of customer demand that is satisfied, given that demand not satisfied immediately is lost.
5 Note, in the linear backlog system it would be necessary to use in the numerator, so that τ becomes indicative of the mean inventory held by the retailer.
6 Note, while we call this operation ‘truncation of the pdf’, our defined pdf is different to the pdf of the regular ‘truncated normal distribution’ (Wikipedia Citation2020).
7 As safety stock,
can be interpreted as the safety stock divided by the standard deviation of demand.
8 When δ is sufficiently high, the relations and
hold, lost sales do not occur and the nonlinear system behaves as the linear system would.
9 For instance, consider a safety factor of , and
. With (Equation40
(40)
(40) ), we obtain
, a 60% reduction to the 20% target.
10 We use the tilde to highlight when the impulse response is taken.
11 This Excel Add-in is also available to download from http://www.bullwhip.co.uk/#shiny.
12 When all demand is lost, , which explains why BW = IVR = 0 when
(Figure (a)).
13 For interested readers the ‘optimised’ PDO parameters and associated costs can be easily obtained from the following MMSE analysis using the relation in (Equation40(40)
(40) ).
Additional information
Notes on contributors
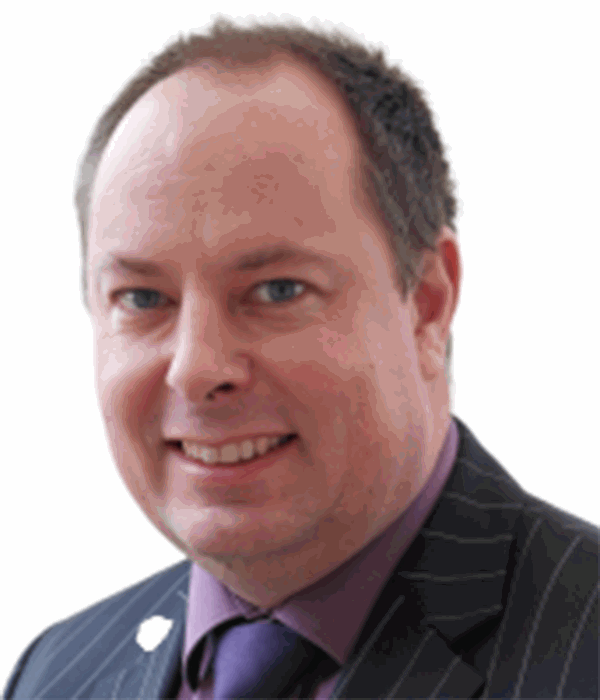
Stephen M. Disney
Stephen M. Disney is currently a Professor of Operations Management at the University of Exeter Business School where is Head of the Science, Innovation, Technology and Entrepreneurship Department. Stephen’s research interests involve the application of control theory and statistical techniques to operations management and supply chain scenarios to investigate their dynamic, stochastic, and economic performance. Stephen has a particular interest in the bullwhip effect, forecasting, and inventory management.
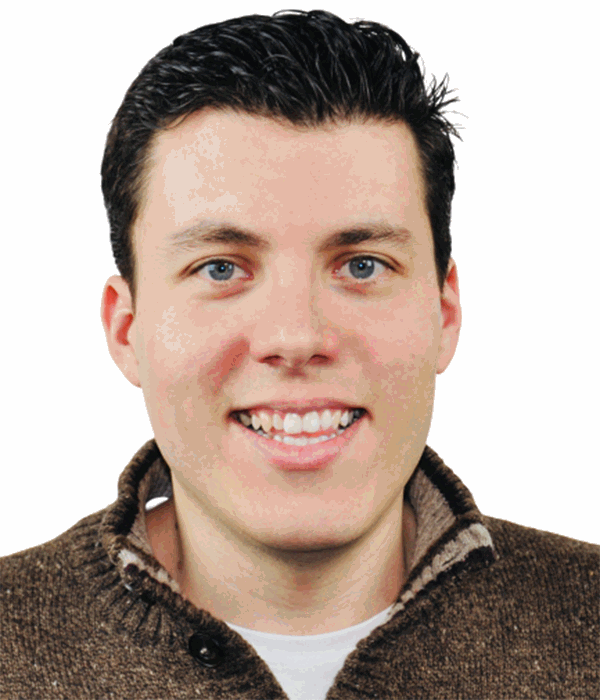
Borja Ponte
Borja Ponte is Lecturer in Business Administration at the University of Oviedo (Spain). His research interests involve the understanding of the dynamics and economics of supply chains, both in traditional and in circular economy settings, via different methodologies such as agent-based techniques and control theory.
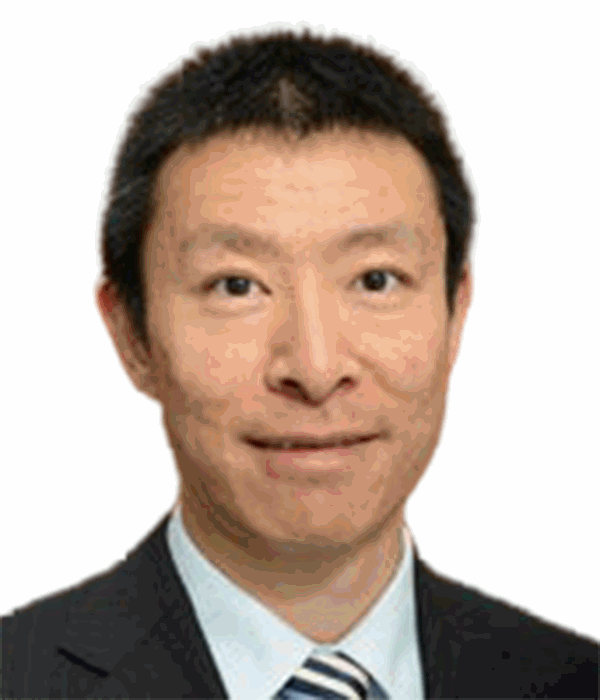
Xun Wang
Xun Wang currently holds the position of Senior Lecturer of Operations Management and Management Science at Cardiff Business School, UK. His current research interest is the causes and mitigation of inventory control problems from both systematic and behavioural perspectives, including the topics of newsvendor decisions, bullwhip effect, demand forecasting and perishable products.