ABSTRACT
We examine a ripple effect in prehospital stroke care processes. Stroke patient care in prehospital stages is provided by emergency medical services (EMS). We divide EMS processes into three segments: dispatcher, field provider service without a patient (i.e., en route to the patient scene), and field provider service with a patient (i.e., transporting the patient to a hospital). We use both empirical and analytical models in this study. The results of the empirical analysis suggest that the dispatcher's stroke identification can influence the time performance of the dispatch center itself and of the subsequent downstream processes of the prehospital stroke care, indicating a potential ripple effect in the care system. Our analytical models demonstrate the impact of misidentification (i.e., a disruptive event at the dispatcher stage) and the unavailability of an Advanced Life Support (ALS) ambulance (i.e., a disruptive event at the field provider stage) on the severity of the stroke patient's prehospital condition. The models indicate that there is an optimal diagnostic time on the part of the dispatcher that minimizes the adverse consequences throughout the prehospital stages of care under disruptive events, and that a centralized system can mitigate a ripple effect in prehospital stroke care.
Acknowledgements
The authors appreciate the support of the NEMSIS Technical Assistance Centre for providing the dataset in this study.
Disclosure statement
No potential conflict of interest was reported by the author(s).
Notes
1 According to the NEMSIS Data Dictionary, the field values 1730 and 535 both refer to “Stroke/CVA.' CVA stands for Cerebrovascular Accident which is a medical term for stroke.
2 Note that is defined but not used in the model. Thus, the second subscript j looks unnecessary because j is always 1 in the model representation. However, we leave the subscript
in the model in order to intuitively express a correct diagnosis and misdiagnosis with subscripts 11 and 01, respectively. Moreover, subscript j will be used in defining the conditional probability in Section 4.1.
Additional information
Notes on contributors

Brandon W. Lee
Brandon Lee (Ph.D., Clemson University) is an Assistant Professor of Operations Management and Business Analytics at the University of Dayton. His research focuses on the areas of healthcare operations management, quality and process improvement, and supply chain management. He obtained his PhD degree in Supply Chain and Operations Management. Prior to his PhD study, he enjoyed a 12-year career in multinational corporations including Siemens and Aisin (a Toyota Group company), where he primarily worked in the field of quality management and Six Sigma process improvement.
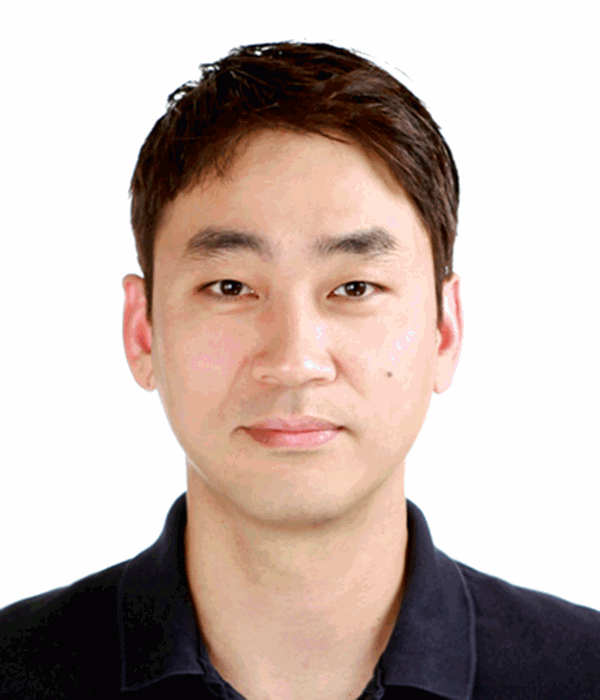
Jiho Yoon
Jiho Yoon (Ph.D., Michigan State University) is an Assistant Professor of Management Science at Chung-Ang University, Seoul, Korea. Prior to join Chung-Ang University, he worked at Kansas State University as an Assistant Professor. His research focuses on the areas of risk management in supply chains, sustainability, and Blockchain implementation in supply chains. His research has appeared in the Decision Sciences, Journal of Business Logistics, and International Journal of Production Research.
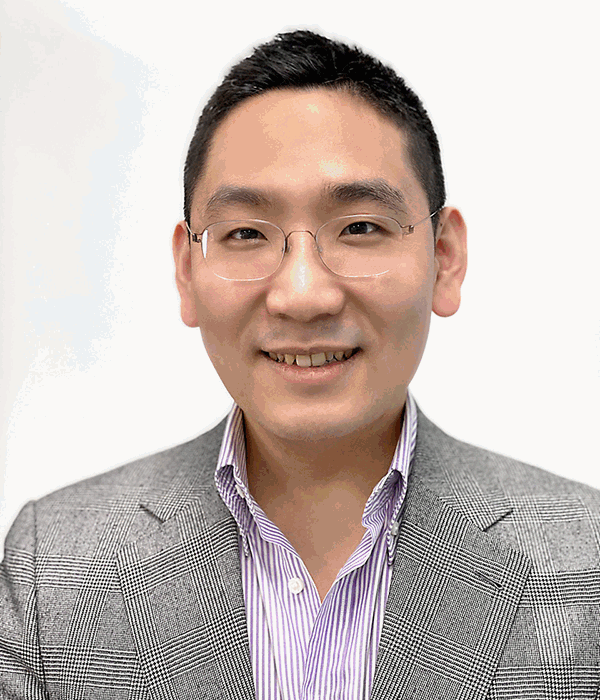
Seung Jun Lee
Seung Jun Lee (Ph.D., Texas A&M University) is an Assistant Professor of Management Science at Chung-Ang University, Seoul, Korea. Before joining at Chung-Ang University, Dr. Lee had worked at San Jose State University as an Assistant Professor. His research focuses on data-driven service operations or healthcare operations management. Specifically, his research has been published in different journals including Journal of Operations Management, Production and Operations Management, and International Journal of Production Research.