Abstract
Supply networks (SNs) play a vital role in fuelling trade and economic growth. Due to their interconnectedness, firm-level disruptions can cause perturbations to ripple through SNs, magnifying initial impact. Contemporary research on ripple effects focussed on understanding various structural features of SNs to predict and control disruption propagation. Our work adds to this body of knowledge by analysing an intriguing topological property that emerges in SNs: ‘nestedness’, which is defined as a pattern of organisation where products that are supplied by specialist suppliers are a subset of products that are supplied by generalist suppliers. In other words, generalists are also specialists. While previous research examined the emergence of nestedness and its possible reasons, its relationship to SN resilience remained unknown. Here, we develop a cascade model by bringing together the product-supplier-buyer structure; which provides us with fine-grained information on SN dependencies. We simulate disruptions in nested and non-nested organisations of the global automotive SN, and find that nested organisations are significantly more robust to random disruptions but vulnerable to hub disruptions under cascade conditions. However, nested structures are not as resilient; as they do not benefit from a response strategy where buyers seek alternative suppliers; because alternative suppliers do not exist. On the other hand, randomly connected SNs are vulnerable to cascades but can allow network reconfiguration.
Disclosure statement
No potential conflict of interest was reported by the author(s).
Additional information
Notes on contributors
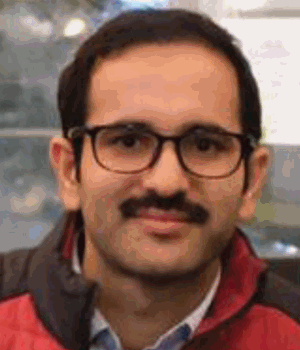
Vinod Kumar Chauhan
Vinod Kumar Chauhan is a Research Associate in Industrial Machine Learning in the Institute for Manufacturing at University of Cambridge UK. He has a PhD in Machine Learning from Panjab University Chandigarh India. His research interests are in Machine Learning, Optimisation and Network Science. He specialises in solving large-scale optimisation problems in Machine Learning, online handwriting recognition, flight delay propagation in airlines, robustness and nestedness in complex networks and supply chain design using mathematical programming, genetic algorithms and reinforcement learning.
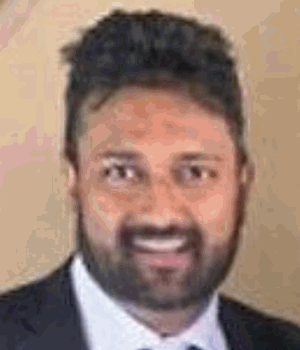
Supun Perera
Supun Perera is currently an Honorary Associate at the Institute of Transport and Logistics Studies in the University of Sydney Business School, Australia, where he carried out his PhD studies in network science. His research interests include development and application of network science and game theory-based models for understanding, quantifying and predicting the behaviour of complex systems such as transportation and inter-firm networks. Supun holds a bachelor’s degree in Civil Engineering with first-class honours, from the University of Auckland, New Zealand, and a master’s degree in Transportation Engineering from the University of California at Berkeley, USA.
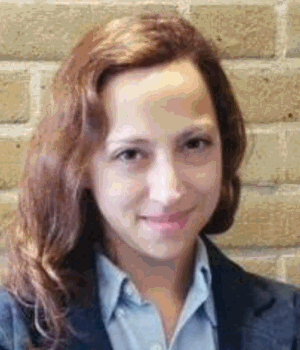
Alexandra Brintrup
Dr Alexandra Brintrup is Lecturer in Digital Manufacturing at Institute for Manufacturing, University of Cambridge. She has an MEng in Manufacturing Engineering and a PhD in Artificial Intelligence. Alexandra’s expertise lies in the design and development of agent based systems and probabilistic AI for manufacturing, with applications in Supply Chains and Logistics. She also specialises in graph mining and complex and nature inspired systems.