Abstract
The feasibility of using deep learning techniques in industrial cold forging for fault detection was investigated. In this work, vibration data were collected from an industrial setting to detect machine conditions resulting in defective products (faults). After collecting data from several commonly encountered faults, a Convolutional Neural Network classifier detected fault conditions with 99.02% accuracy and further classified each fault with 92.66% accuracy. A decision tree (DT) model was also used in an attempt to detect and classify faults using time domain features. The model was able to detect faults with 92.5% accuracy but was unable to classify them. In addition, DT feature importance analysis was performed to understand how various faults impacted the machine signal for future refinement of the proposed system. The results suggest that the proposed deep learning method has the potential to detect faults in cold forging, but future work is required to validate the method.
Acknowledgments
This work was supported in part by the High-Potential Individuals Global Training Program of Institute for Information and Communication Technology Planning and Evaluation (IITP) under Grant 2019-0-01589. The authors also gratefully acknowledge the support of Semblex Co.
Nomenclature
CNN | = | Convolutional Neural Network |
DT | = | Decision Tree |
Disclosure statement
No potential conflict of interest was reported by the author(s).
Additional information
Notes on contributors
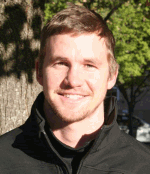
Andrew Glaeser
Andrew Glaeser received a B.S. in Mechanical Engineering from Northern Illinois University. He is currently pursuing a M.S. degree with the Manufacturing Innovation Network Laboratory at the University of Wisconsin-Madison. His research interests include industrial applications of smart manufacturing in mechanical systems.
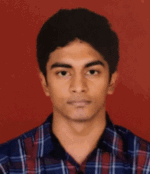
Vignesh Selvaraj
Vignesh Selvaraj received a B.S. in Mechanical Engineering from P.S.G College of Technology, Coimbatore, India. He received his M.S in Industrial and Systems Engineering from the University of Wisconsin Madison with the Quick Response Manufacturing Centre. He is currently pursuing his Ph.D. in Mechanical Engineering with the Manufacturing Innovation Network Laboratory at the University of Wisconsin Madison. His research interest includes Smart Manufacturing, IIoT Systems and Energy Monitoring.
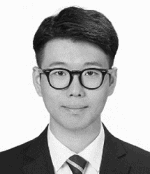
Sooyoung Lee
Soo Young Lee received the B.S. degree in Mechanical Engineering from Chung-Ang University, Seoul, South Korea. He is currently pursuing the Ph.D. degree with the industrial Artificial Intelligence Laboratory, POSTECH, South Korea. His research interests include industrial artificial intelligence with mechanical systems and applications of the AI-based smart manufacturing.
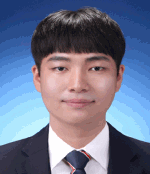
Yunseob Hwang
Yunseob Hwang received the B.S. degree from Chungnam National University, Daejeon, South Korea. He is currently pursuing the M.S. degree with the Industrial Artificial Intelligence Laboratory, POSTECH, South Korea. His research interests include industrial artificial intelligence with mechanical systems and AI-based health care.
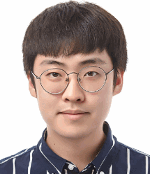
Kangsan Lee
Kangsan Lee received a B.S. degree of ME from Soongil University in 2019. He is a graduate student at industrial AI lab. of Pohang University of Science and Technology.
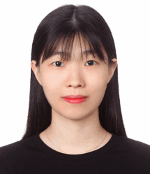
Namjeong Lee
Namjeong Lee received a B.S. degree from the Ulsan National Institute of Science and Technology in 2018. She is a graduate student at the industrial AI Lab. at Pohang University of Science and Technology. She is interested in machine health diagnosis.
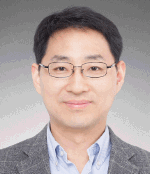
Seungchul Lee
Seungchul Lee is assistant professor at Department of Mechanical Engineering, POSTECH. His research interests include industrial artificial intelligence with mechanical systems, deep learning for machine healthcare, and IoT-based smart manufacturing.
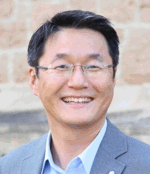
Sangkee Min
Sangkee Min is assistant professor at Department of Mechanical Engineering, University of Wisconsin-Madison. He is currently working on three major research topics; UPM (Ultra-Precision Machining), SSM (Smart Sustainable Manufacturing), and MFD (Manufacturing for Design).