Abstract
Past maintenance logs may encapsulate meaningful data for predicting the duration of machine breakdowns, the potential causes of a problem, or the necessity to stop production to perform repair activities. These insights may be accessed using machine learning (ML). However, maintenance logs tend to have imbalanced distributions and rely on noisy unstructured text data provided by operators. Additionally, the limited interpretability of ML models results in human reluctance when accepting model predictions. Hence, this study explored the use of two recent deep learning models (CamemBERT and FlauBERT) for natural language processing (NLP) to harness unstructured data from maintenance logs. The class imbalance effect was mitigated using data-level and algorithm-level approaches. To improve interpretability, a technique called LIME was employed to interpret single predictions and to propose a method for insight extraction from several maintenance reports. Results suggest three key points: CamemBERT and FlauBERT can achieve excellent results with minimum text pre-processing and hyperparameter tuning. Second, random oversampling (ROS) generally mitigates the effect of class imbalance. However, ROS was observed to be unnecessary when performing pertinent data pre-processing. Finally, at the maintenance level, the proposed insight extraction method can provide valuable information from a set of poorly structured maintenance reports.
Acknowledgements
This work was financially supported by a partnership between the company iFAKT France SAS and the Association Nationale de la Recherche et de la Technologie (ANRT) under Grant 2018/1266.
Disclosure statement
No potential conflict of interest was reported by the author(s).
Additional information
Funding
Notes on contributors

Juan Pablo Usuga-Cadavid
Juan Pablo Usuga Cadavid is a PhD student in artificial intelligence applied to Industry 4.0 at Arts et Métiers Institute of Technology within the LAMIH-CNRS laboratory. He received his BSc in Production Engineering from EAFIT University, an MSc. in Engineering from Arts et Métiers Institute of Technology and a Master in Management with a quantitative methods concentration from IAE de Paris – Sorbonne Business School. He is currently working in natural language processing applied to predictive maintenance for decision support, and he teaches Machine Learning at Arts et Métiers Institute of Technology. Besides his research activities, he also works at iFAKT France SAS as a consultant in the Data Analytics service on behalf of Airbus.
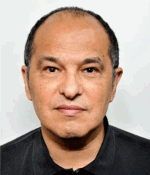
Samir Lamouri
Samir Lamouri received his Ph.D. (1989) from the Institut National Polytechnique of Lorraine (INPL), France. He is currently a full professor at Arts et Métiers Institute of Technology (France). He teaches Production Management, Linear Control, and Simulation. His research is conducted as part of the LAMIH-CNRS, the Automatic Control, Computer Science, and Mechanical research laboratory, also in Paris. His areas of interest include distributed and heterarchical control of discrete event systems (manufacturing, transport, logistics, and services). He is a member of the French CNRS research group, MACS, and author of several publications in the manufacturing domain.
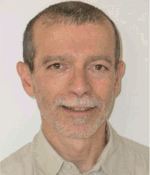
Bernard Grabot
Bernard Grabot is a Professor of Industrial engineering at the National Engineering School of Tarbes, France (ENIT/INP). His research activities are oriented toward knowledge engineering and artificial intelligence for supply chain management and information systems. Prof. Grabot is a member of the IFAC working groups 3.2 ‘Computational Intelligence in Control’ and 5.1 ‘Manufacturing Plant Control’ and of the IFIP group 5.7 ‘Advances in Production Management’. He is the Editor in Chief of ‘Computers in Industry’ (Elsevier) and a member of the Editorial boards of ‘International Journal of Production Research’, ‘International Journal of Computational Intelligence’ and ‘Production & Manufacturing Research’.
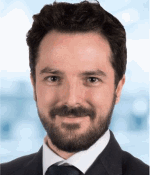
Arnaud Fortin
Arnaud Fortin is the Managing Director of iFAKT France SAS, and offers support based on management and strategy consulting as well as integrated solutions for production optimisation, particularly today in the aeronautics and automotive industries. After graduating from Arts et Métiers Institute of Technology, he started a career as a consultant within a major management consulting firm. This first experience allowed him to build up solid expertise in the governance of major transformation projects in the telecom and aeronautics sectors. Relying on these years, Arnaud gradually specialised in process optimisation for industry and services, strengthening his specialisation with a Lean Six Sigma Black Belt certification in 2013. Arnaud also participates in the development of Predictive ERP, a solution developed by iFAKT France SAS targeting production modelling, simulation, and optimisation.