Abstract
Diversified customer needs make the production system more susceptible to high-frequency fluctuations of uncertain factors (UFs), which puts forward higher requirements for the real-time and systematic decision-making of the system. The opti-state control strategy enables the system to maintain the adaptive optimal state after being disturbed. The core intelligent synchronisation of the opti-state control strategy needs to perceive the state of the affected system and its degree of change. Aiming at the challenge of difficulty in evaluating the uncertain factors impact degree (UFID) of the complex production logistics system, this work proposes a big data-enabled intelligent synchronisation under the opti-state control strategy. Based on the simulation data of system operation, big data is used to mine the relationship between the UFID and the system states, then use wrapper GA-DNN (Deep Neural Network) feature selection and classification method evaluates the UFID, which will be applied to the synchronisation decision. The results show that the method can accurately evaluate the UFID and avoid the waste of resources and the increase in operating costs caused by excessive evaluation of the UFID, thereby also improves the effectiveness and efficiency of the opti-state control strategy.
Acknowledgements
This paper is financially supported by the 2019 Guangdong Special Support Talent Program-Innovation and Entrepreneurship Leading Team (China) (2019BT02S593), National Natural Science Foundation of China (51875251), 2018 Guangzhou Leading Innovation Team Program (China) (201909010006), Blue Fire Project (Huizhou) Industry-University-Research Joint Innovation Fund of the Ministry of Education (China) (CXZJHZ201722), and the Fundamental Research Funds for the Central Universities (11618401).
Disclosure statement
No potential conflict of interest was reported by the author(s).
Data availability statement
The authors confirm that the data supporting the findings of this study are available within the article.
Additional information
Funding
Notes on contributors
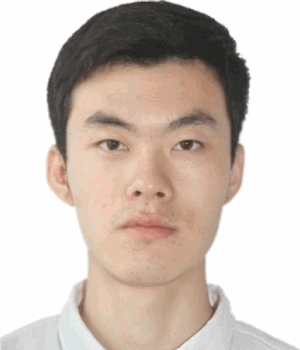
Kai Zhang
Kai Zhang is a PhD candidate in the school of management, Jinan University (China). He received his BEng degrees from School of Electromechanical Engineering of Guangdong University of Technology (China). His research interests include IoT-based smart manufacturing systems and production-logistics synchronisation decision-making.
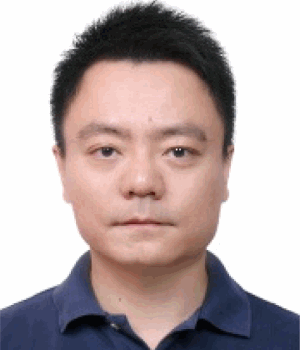
Ting Qu
Ting Qu is a full professor at School of Intelligent Systems Science and Engineering, Jinan University (China). He received his BEng and MPhil degrees from School of Mechanical Engineering of Xi'an Jiaotong University (China) and obtained PhD degree from the department of Industrial and Manufacturing Systems Engineering of The University of Hong Kong. His research interests include IoT-based smart manufacturing systems, logistics and supply chain management, and industrial product/production service systems. He has undertaken over twenty research projects funded by government and industry and has published nearly 200 technical papers in these areas, half of which have appeared in reputable journals. He serves as the director or board members of several academic associations in industrial engineering and smart manufacturing areas.
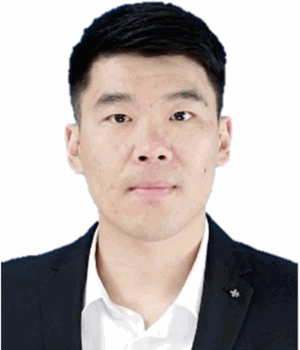
Yongheng Zhang
Yongheng Zhang is a PhD candidate in the school of management, Jinan University (China). He received his BEng degrees from College of Electronic Information and Automation of Tianjin University of Science and Technology (China). His research interests include big data enabled manufacturing planning and scheduling, and smart manufacturing systems.
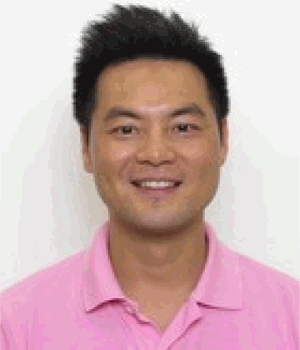
Ray Y. Zhong
Ray Y. Zhong is an Assistant Professor in The Department of Industrial and Manufacturing Systems Engineering, The University of Hong Kong. He was a lecturer in The Department of Mechanical Engineering, University of Auckland, New Zealand from 2016-2019. Ray gained his M.Phil. and Ph.D. in Signal & Information Processing and Industrial & Manufacturing Systems Engineering from the Guangdong University of Technology (China) and The University of Hong Kong (Hong Kong) respectively. His research interests include Internet of Things (IoT)-enabled manufacturing, Big Data in manufacturing & SCM and data-driven APS. Dr. Zhong is member of IEEE, CIRP RA, IET, and ASME.
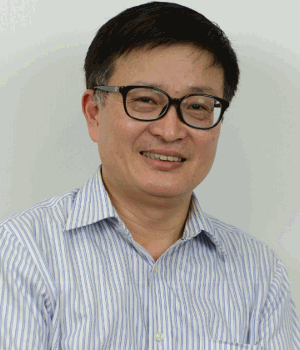
George Huang
George Q. Huang is Chair Professor and Head of Department in Department of Industrial and Manufacturing Systems Engineering, The University of Hong Kong. He gained his BEng and PhD in Mechanical Engineering from Southeast University (China) and Cardiff University (UK) in 1983 and 1991 respectively. He has conducted research projects in the field of Physical Internet (Internet of Things) for Manufacturing and Logistics Automation and Management with substantial government and industrial grants. He has published extensively in these fields. His research works have been widely cited in relevant fields. He serves as associate editors and editorial members for several international journals. He is a Chartered Engineer (CEng), a fellow of ASME, HKIE, IET and CILT, and member of IIE.