Abstract
With the advent of the socio-technical manufacturing paradigm, the way in which rescheduling decisions are taken at the shop floor has radically changed in order to guarantee highly efficient production under increasingly dynamic conditions. To cope with uncertain production environments, a drastic increase in the type and degree of automation used at the shop floor for handling unforeseen events and unplanned disturbances is required. In this work, the on-line rescheduling task is modelled as a closed-loop control problem in which an artificial autonomous agent implements a control policy generated off-line using a schedule simulator to learn schedule repair policies directly from high-dimensional sensory inputs. The rescheduling control policy is stored in a deep neural network, which is used to select repair actions in order to achieve a small set of repaired goal states. The rescheduling agent is trained using Proximal Policy Optimisation based on a wide variety of simulated transitions between schedule states using colour-rich Gantt chart images and negligible prior knowledge as inputs. An industrial example is discussed to highlight that the proposed approach enables end-to-end deep learning of successful rescheduling policies to encode task-specific control knowledge that can be understood by human experts.
Disclosure statement
No potential conflict of interest was reported by the author(s).
Data availability statement
The data that support the findings of this study are openly available in Mendeley Data at https://doi.org/10.17632/x9vdrdwyfh.1, reference (Jorge Palombarini and Martínez Citation2021).
Notes
1 The Average Reduced Tardiness percentage includes episodes in which the schedule was not repaired.
2 Negative values of Total Tardiness/Makespan reduction correspond to an increase of such values. FCFS, LTT/LM, EA and MP do not distinguish H/S configurations, therefore the obtained values are the same.
Additional information
Funding
Notes on contributors
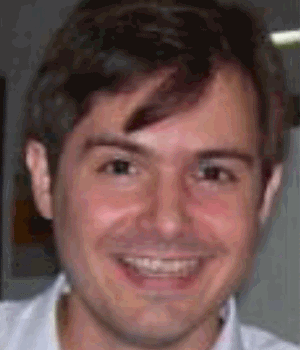
Jorge Andrés Palombarini
Jorge A. Palombarini received his PhD. in Information Systems Engineering from the Universidad Tecnológica Nacional of Argentina (UTN-Argentina) in 2014. Current academic position includes Associate Professor of Artificial Intelligence and Syntax and Semantic of Languages in the UTN, and Assistant Research Fellow of CONICET. He was a software developer in private sector institutions and auditor of information systems on Universidad Nacional de Villa María, Argentina (UNVM-Argentina). His current research interest includes Reinforcement learning, Deep Learning, Cognitive systems and Formal Frameworks for industrial process modelling and simulation.
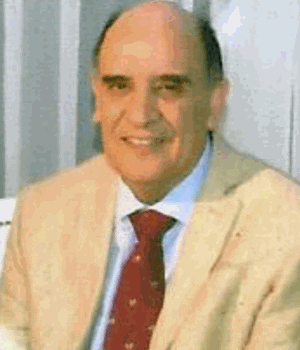
Ernesto Carlos Martínez
Ernesto C. Martínez received his PhD. in Chemical Engineering from the University of Litoral (UNL-Argentina) in 1989. Current academic position includes chair professor of Systems and Organisations in the Technical University of Argentina and Senior Research Fellow of CONICET. He was visiting professor in the University of Valladolid (Spain) and Research Fellow at Lehigh University (USA) and the University of Nottingham (UK). He had supervised the successful completion of 11 PhD Thesis and is the co-author of more than one hundred journal articles and book chapters. His current research interest includes Reinforcement Learning, Deep Learning, Cognitive systems, Bayesian Optimisation and optimal design of learning experiments.