Abstract
Severe environmental problems have made green scheduling an emerging research hotspot. In this paper, a permutation flow shop energy-efficient scheduling problem that considers multiple criteria is investigated. The aim is to find the optimal job processing sequence and conveyor speed that minimise both the makespan and total energy consumption. In addition to two types of common criteria, namely, machine-based criterion (i.e. sequence-dependent setup time) and energy-based criteria (including both the transportation time control strategy and machine shutdown strategy), a human-based criterion (i.e. a position-based learning effect) is introduced. A bi-objective programming model is developed, and a multi-objective iterated greedy (MOIG) is designed to reach the Pareto front of the model. Considering that there are two types of decisions in the model (i.e. job sequence and conveyor speed), two algorithm alternatives are designed based on the job sequence and conveyor speed, respectively. Meanwhile, an acceptance criterion with advantages in terms of the convergence speed and solution diversity is proposed. Existing algorithms, including NSGA-II and MOEA/D, are introduced to evaluate the performance of the MOIG. The results emphasise the efficiency of the MOIG. Overall, the model and MOIG effectively improve the green efficiency of enterprises and can reasonably control operating costs.
Acknowledgements
The authors give the indebted thanks to the editor and referees for their valuable comments and suggestions, which help us improve the paper greatly. Finally, the first author wants to thank Dazhou Yu for the support.
Disclosure statement
No potential conflict of interest was reported by the author(s).
Data availability statement
The authors confirm that the data supporting the findings of this study are available within the article.
Additional information
Funding
Notes on contributors
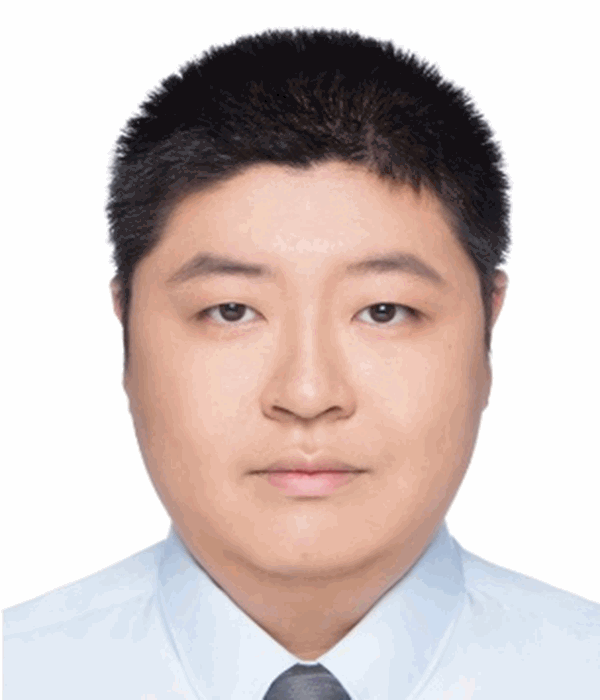
Xu Xin
Xu Xin is a PhD candidate at School of Economics and Management of Tongji University. His research interests include maritime logistics system design, transportation system management and industrial engineering and management. Since graduating from Dalian Maritime University in 2018, he has been engaged in supply chain management and production operations management and has published more than 10 papers in International Journal of Logistics Research and Applications, Applied Economics and Ocean & Coastal Management. He is also a reviewer for Ocean & Coastal Management, International Journal of Logistics Research and Applications and Maritime Policy & Management.
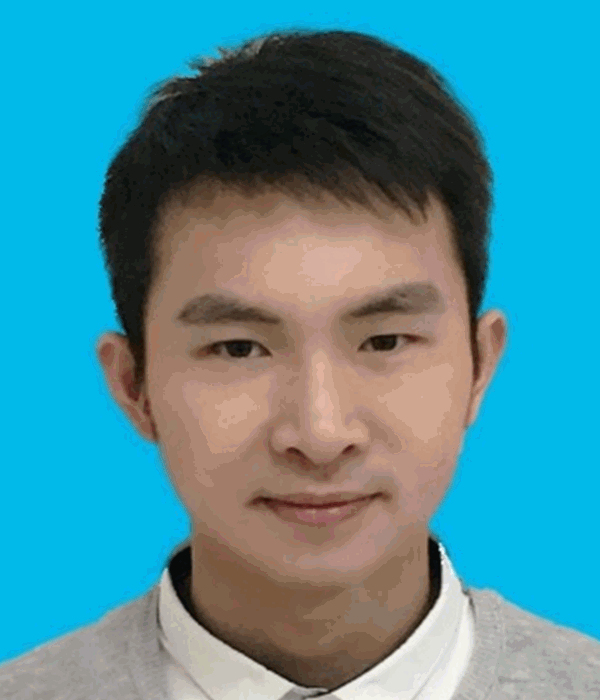
Qiangqiang Jiang
Qiangqiang Jiang is a PhD candidate at Institute of Space Science and Applied Technology, Harbin Institute of Technology, Shenzhen. He received M.Eng. degree in Software Engineering from Zhejiang University in 2020. His research interests include intelligent optimisation algorithm and high-performance computing.
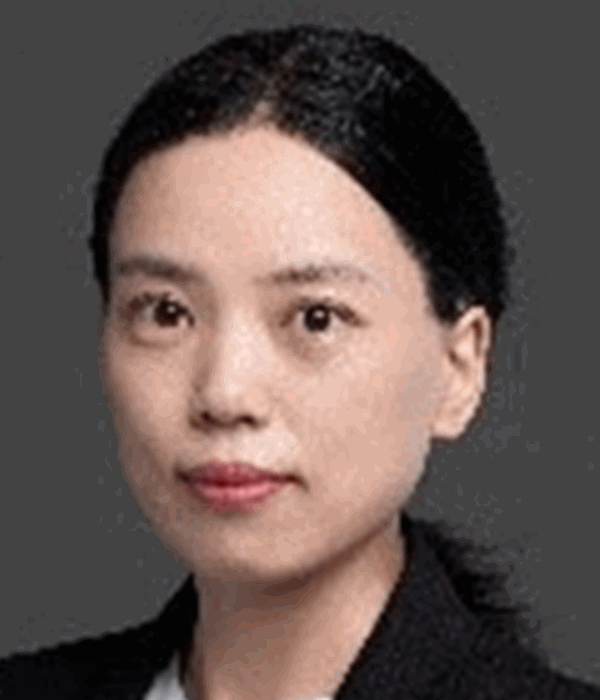
Cui Li
Cui Li received the B.Eng. degree from Jilin Jianzhu University, Jilin, China, in 2006; and the Ph. D. degree from the School of Mechanical Engineering Tongji University, Shanghai, China, 2014. From 2014-Present, she works as an Engineer at School of Mechanical Engineering Tongji University. Her current research interests include energy efficiency, occupants’ behaviour, and intelligent control in buildings.
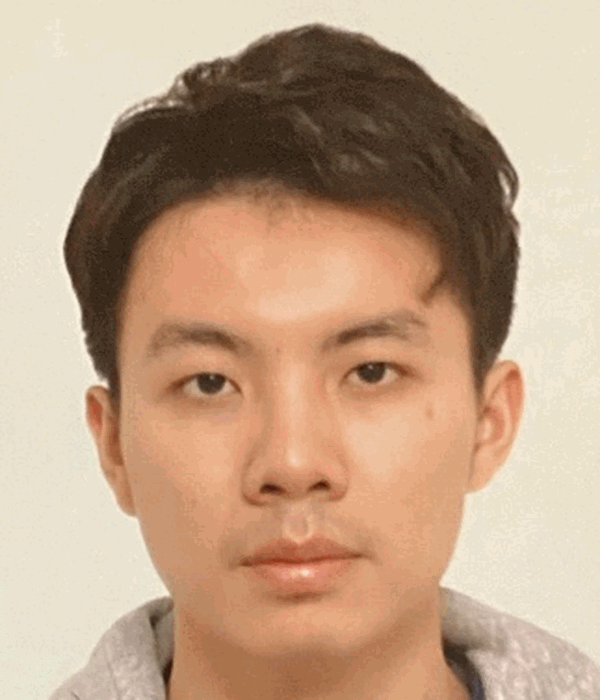
Sihang Li
Sihang Li is currently pursuing the B.Eng. degree in mechanical engineering with Tongji University, Shanghai, China. His research interests include stochastic optimisation and operations management.
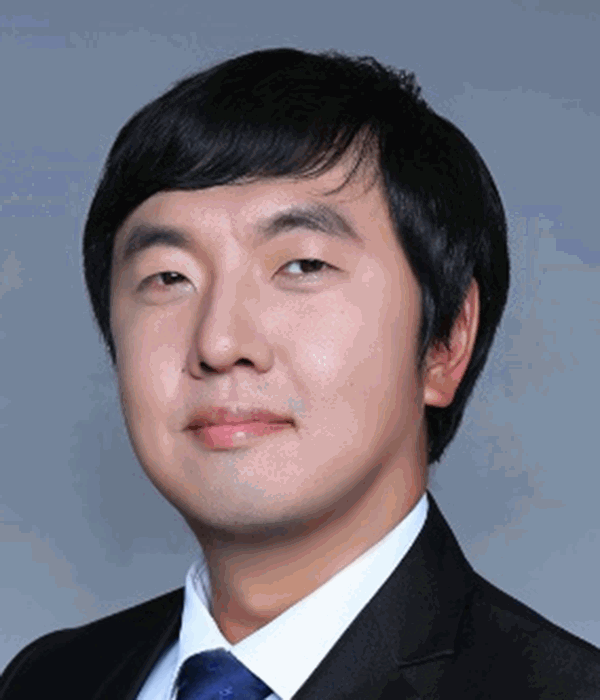
Kang Chen
Kang Chen received his Ph.D. degree in transportation engineering from Dalian Maritime University. He is currently a professor of School of Maritime Economics and Management, Dalian Maritime University, China. His research interests include transportation planning and automated terminal operation and scheduling. He has published more than 20 papers in journals in the field of transportation management such as Transportation Research Part E, Transport Policy and Maritime Economics & Logistics. He has served as a guest editor for Transport Policy in 2021.