Abstract
In various industrial problems, sensor data are often used to detect the abnormal state of manufacturing systems. Sensor data are sometimes influenced by contextual variables that are not related to the system health status and may exhibit different behaviours depending on their values, even if the system is in a normal condition. In this case, a conditional anomaly detection method should be used to consider the effects of contextual variables. In this study, we propose a conditional anomaly detection method, particularly for high-dimensional and complex data, using a deep embedding kernel mixture network. The proposed method comprises embedding and kernel mixture networks. The embedding network learns low-dimensional embeddings from high-dimensional data, and the kernel mixture network models the distribution of the learned embeddings conditional on contextual variables. The two networks enable a flexible estimation of conditional density using the high expressive power of deep neural networks. The two networks are trained simultaneously such that the high-dimensional data are embedded into a low-dimensional space, to assist conditional density estimation. The effectiveness of the proposed model is demonstrated using real data examples from the UCI repository and a case study from a tire company.
Acknowledgements
The authors would like to thank the referees, the associate editor, and the editor for reviewing this article and providing valuable comments.
Data availability statement
The datasets in Section 3 are available at http://archive.ics.uci.edu/ml, and the dataset in Section 4 is not publicly available due to confidentiality.
Disclosure statement
No potential conflict of interest was reported by the author(s).
Additional information
Funding
Notes on contributors
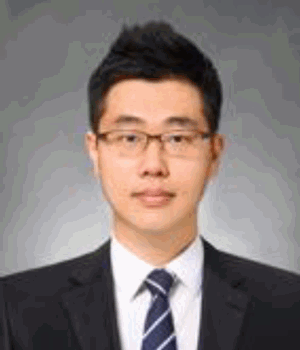
Hyojoong Kim
Hyojoong Kim received a B.S. degree in industrial engineering from Hanyang University in Korea and an M.S. degree in industrial and systems engineering from KAIST. He is currently a PhD candidate in industrial and system engineering at KAIST. His research interests include machine learning and applied statistics.

Heeyoung Kim
Heeyoung Kim received a B.S. degree in industrial engineering from KAIST, an M.S. degrees in statistics and industrial engineering from the Georgia Institute of Technology and KAIST, and a PhD degree in industrial engineering from the Georgia Institute of Technology. She is an associate professor with the Department of Industrial and Systems Engineering, KAIST. She was a Senior Member of Technical Staff with AT&T Laboratories. Her research interests include applied statistics and machine learning