Abstract
The complexity of composite grinding movement, the variety of machining features and available grinding wheels, and the changing working conditions pose a challenge to the rapid programming of safe and efficient composite grinding procedure. The procedure optimisation plays an important role in solving this difficult problem. Therefore, a procedure optimisation method is proposed for composite grinding based on a Digital Twin (DT) system, which takes procedure time as optimisation objective to achieve high efficiency and ensures process rationality and safety by constructing corresponding constraint conditions. Moreover, the actual working conditions mapped into the DT system, such as workpiece parameters, machining requirements, grinding wheel parameters and status, machine tool motion position, and so on, are obtained to update the parameters involved in the optimisation model. And thus, the proposed method has the ability to timely find the optimal procedure under changing working conditions. In addition, a combination algorithm based on genetic algorithm (GA) and dynamic programming is proposed, which greatly reduces the search space of GA and realises the two-class co-optimisation of grinding wheel selection and procedure. Finally, the case study verifies the effectiveness of the proposed method to reduce procedure time and the dynamic response ability to changing working conditions.
Disclosure statement
No potential conflict of interest was reported by the author(s).
Data availability statement
The authors confirm that the data supporting the findings of this study are available within the article and its supplementary materials.
Additional information
Funding
Notes on contributors
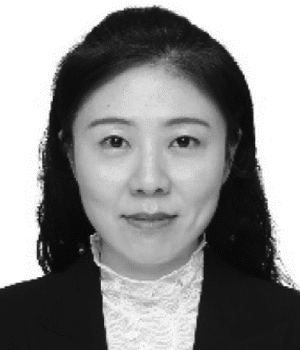
Nanyan Shen
Nanyan Shen is currently a professor/Ph.D at Shanghai Key Laboratory of Intelligent Manufacturing and Robotics, School of Mechatronic Engineering and Automation, Shanghai University, China.
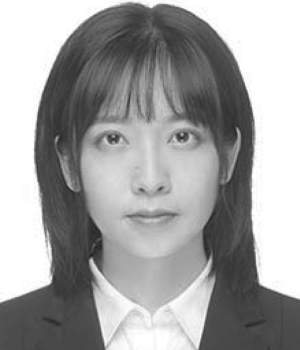
Yang Wu
Yang Wu is currently an assistant lab master at Shanghai Key Laboratory of Intelligent Manufacturing and Robotics, School of Mechatronic Engineering and Automation, Shanghai University, China.
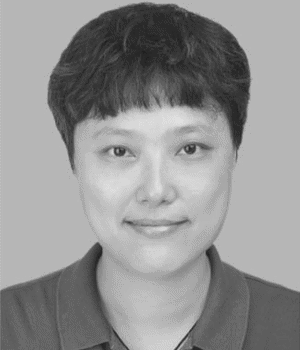
Jing Li
Jing Li is currently an associate professor/Ph.D at Shanghai Key Laboratory of Intelligent Manufacturing and Robotics, School of Mechatronic Engineering and Automation, Shanghai University, China.
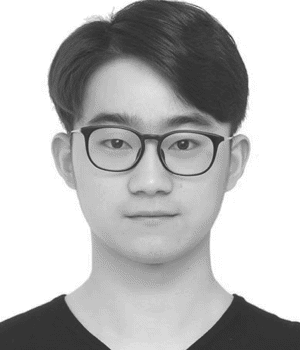
Tianqiang He
Tianqiang He is currently a master candidate at School of Mechatronic Engineering and Automation, Shanghai University, China.
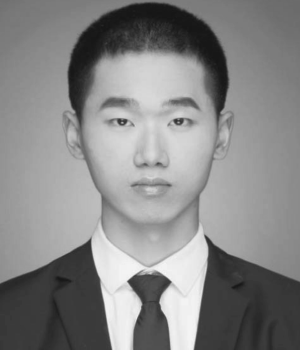
Yushun Lu
Yushun Lu is currently a master candidate at School of Mechatronic Engineering and Automation, Shanghai University, China.
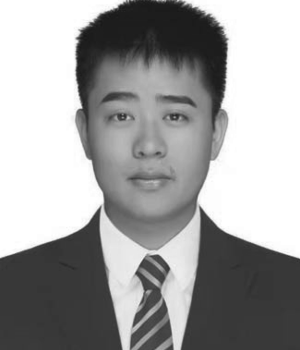
Yingjie Xu
Yingjie Xu is currently a master alumni from School of Mechatronic Engineering and Automation, Shanghai University, China.