Abstract
Job shop scheduling problem (JSP) is a widely studied NP-complete combinatorial optimisation problem. Neighbourhood structures play a critical role in solving JSP. At present, there are three state-of-the-art neighbourhood structures, i.e. N5, N6, and N7. Improving the upper bounds of some famous benchmarks is inseparable from the role of these neighbourhood structures. However, these existing neighbourhood structures only consider the movement of critical operations within a critical block. According to our experiments, it is also possible to improve the makespan of a scheduling scheme by moving a critical operation outside its critical block. According to the above finding, this paper proposes a new N8 neighbourhood structure considering the movement of critical operations within a critical block and the movement of critical operations outside the critical block. Besides, a neighbourhood clipping method is designed to avoid invalid movement, discarding non-improving moves. Tabu search (TS) is a commonly used algorithm framework combined with neighbourhood structures. This paper uses this framework to compare the N8 neighbourhood structure with N5, N6, and N7 neighbourhood structures on four famous benchmarks. The experimental results verify that the N8 neighbourhood structure is more effective and efficient in solving JSP than the other state-of-the-art neighbourhood structures.
Data availability statement
The data that support the findings of this study are openly available at http://optimizizer.com/jobshop.php.
Disclosure statement
No potential conflict of interest was reported by the author(s).
Additional information
Funding
Notes on contributors
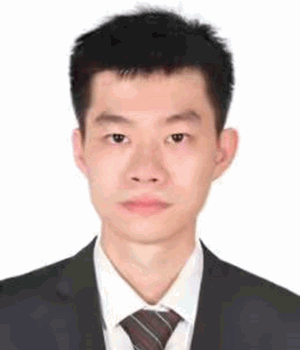
Jin Xie
Jin Xie received the B.S. degree in mechanical engineering and automation form China University of Geosciences, Wuhan, China, 2015. He is currently pursuing the Ph.D. degree in industrial engineering with the Huazhong University of Science and Technology, Wuhan, China. His current research interests include discrete optimisation and scheduling.
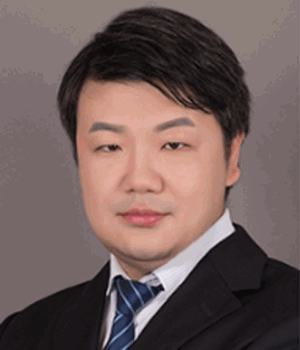
Xinyu Li
Xinyu Li received the Ph.D. degree in industrial engineering from Huazhong University of Science and Technology (HUST), China, 2009. He is a Professor of the Department of Industrial & Manufacturing Systems Engineering, State Key Laboratory of Digital Manufacturing Equipment & Technology, School of Mechanical Science & Engineering, HUST. He had published more than 70 refereed papers. His research interests include intelligent scheduling, machine learning etc.
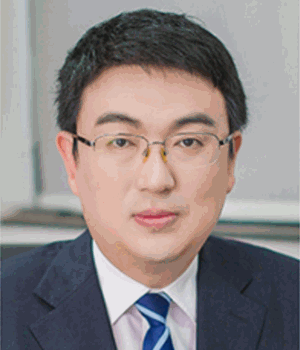
Liang Gao
Liang Gao received the Ph.D. degree in mechatronic engineering from Huazhong University of Science and Technology (HUST), China, 2002. He is a Professor of the Department of Industrial & Manufacturing System Engineering, State Key Laboratory of Digital Manufacturing Equipment & Technology, School of Mechanical Science & Engineering, HUST. He had published more than 470 refereed papers. His research interests include operations research and optimisation, scheduling, big data and machine learning etc. Prof. GAO is an Associate Editor of Swarm and Evolutionary Computation, Journal of Industrial and Production Engineering and. He is co-Editor-in-chief of IET Collaborative and Intelligent Manufacturing.
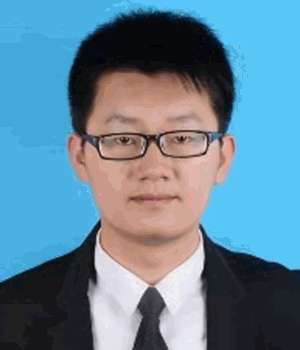
Lin Gui
Lin Gui received the B.S. degree in mechanical engineering from Shandong University, Jinan, China, 2018. He is currently pursuing the Ph.D. degree in industrial engineering with the Huazhong University of Science and Technology, Wuhan, China. His research interests are shop scheduling problem and algorithm optimisation.