Abstract
Traditionally, manufacturers use past orders (received from some downstream supply chain level) to forecast future ones, before turning such forecasts into appropriate inventory and production optimisation decisions. With recent advances in information sharing technologies, upstream supply chain (SC) companies may have access to downstream point of sales (POS) data. Such data can be used as an alternative source of information for forecasting. There are a few studies that investigate the benefits of using orders versus POS data in upstream SC forecasting; the results are mixed and empirical evidence is lacking, particularly in the context of multi-echelon SCs and in the presence of promotions. We investigate an actual three-echelon SC with 684 series where the manufacturer aims to forecast orders received from distribution centres (DCs) using either aggregated POS data at DCs level or historical orders received from the DCs. Our results show that the order-based methods outperform the POS-based ones by 6–15%. We find that low values of mean, variance, non-linearity and entropy of POS data, and promotion presence negatively impact the performance of the POS-based forecasts. Such findings are useful for determining the appropriate source of data and the impact of series characteristics for order forecasting in SCs.
Acknowledgments
The first author is grateful to The University of Newcastle, Australia, and the Center for Food and Beverage Supply Chain Optimisation for providing the opportunity to visit Cardiff University, UK. The authors also thank the reviewers for their helpful comments that helped us to improve the presentation of the paper.
Disclosure statement
No potential conflict of interest was reported by the authors.
Data availability statement
The data that support the findings of this study are available on request from the corresponding author subject to permission from data owners. The data are not publicly available due to containing confidential information that could compromise the privacy of stakeholders.
Additional information
Notes on contributors
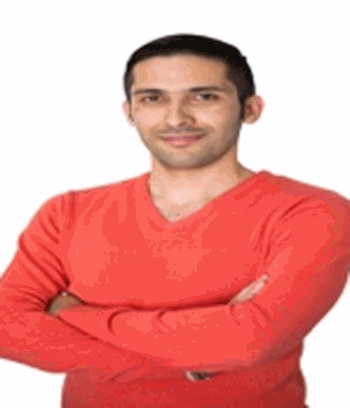
Mahdi Abolghasemi
Mahdi Abolghasemi is Lecturer in Data Science and Business Analytics at The University of Queensland, Australia. His research interests include forecasting, data science and machine learning with applications in supply chain and energy sectors. Mahdi has published dozens of articles in leading international journals and consulted several companies in energy, manufacturing, food, and automotive industries.
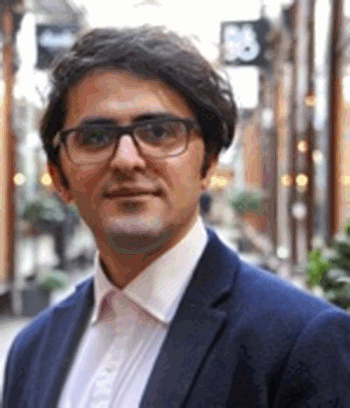
Bahman Rostami-Tabar
Bahman Rostami-Tabar, Ph.D., is Associate Professor of Management Science at Cardiff Business School. He received his Ph.D. in Industrial Engineering from the University of Bordeaux in 2014. Bahman's research goals are directed toward the use of Operations Research techniques towards improving decisions with societal impact. Dr Rostami-Tabar's current research interests involve forecasting for social good and its interface with decision-making. Bahman is leading democratising forecasting and forecasting for social good initiatives supported by the International Institute of Forecasters. He has worked with many organisations such as NHS Wales, Welsh Ambulance Service NHS Trust, the International Committee of the Red Cross and the U.S. Agency for International Development.
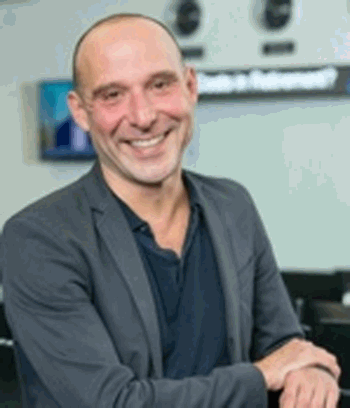
Aris Syntetos
Aris Syntetos is Professor of Operational Research and Operations Management at Cardiff Business School, Cardiff University, where he also holds the PARC Chair in Manufacturing and Logistics. His research looks at how organisations can make more accurate predictions of future sales and how such predictions can help them reduce their immense investments in inventories. Currently, he is researching inventory (and/or) forecasting issues in circular (closed loop) supply chains, additive manufacturing (3DP) enabled supply chains and retail supply chains.