Abstract
In a machining line with a Lean manufacturing environment, such as one used for mass production of automotive components, tool life is determined by a tool counter that is set on the safe side to avoid producing a failed product, so the cutting tools are typically underutilised. On the other hand, cutting parameters optimisation, which has a significant impact on tool life, is not feasible due to the rule that prohibits changing the cutting parameters during mass production to maintain the quality standard. To address both issues, this paper proposes a hybrid monitoring and optimisation process that can be carried out concurrently without interfering with production activities. The monitoring process involves two methods, namely threshold limit-based monitoring and machine learning-based monitoring, for ensuring product quality during the optimisation process. Meanwhile, Bayesian optimisation (BO) is used for the optimisation process due to its capability for autonomous search for the optimum condition of an unknown and expensive objective function. The case study demonstrated BO’s ability to find the optimal condition with a small number of samples, while the machine learning method used can monitor tool wear and surface roughness with average errors of 5.5% and 7.7%, respectively.
Disclosure statement
No potential conflict of interest was reported by the author(s).
Data availability statement
The data that support the findings of this study are available from the corresponding author, [JH], upon reasonable request.
Correction Statement
This article has been corrected with minor changes. These changes do not impact the academic content of the article.
Additional information
Funding
Notes on contributors
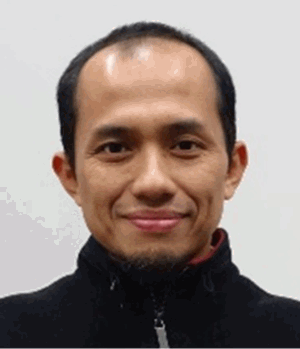
Jonny Herwan
Jonny Herwan is currently a researcher at Industrial CPS Research Center, National Institute of Advanced Industrial Science and Technology (AIST), Japan. He received his Ph.D. from Graduate School of System Design, Tokyo Metropolitan University in 2016. He is working in the field of machining process including high speed machining, machining of difficult to cut materials, smart machining, and optimisation. He has working experience in Japanese automotive companies for several years.
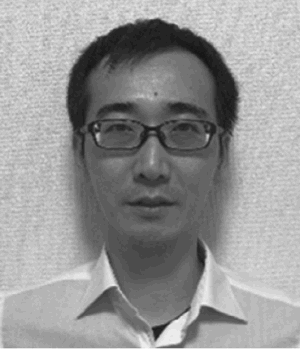
Takashi Misaka
Takashi Misaka received Ph.D. in Information Science from Tohoku University in 2008. After working at German Aerospace Center (DLR) and Tohoku University, he is currently affiliated with National Institute of Advanced Industrial Science and Technology (AIST). His research interests include CAE simulation, data assimilation and machine learning.
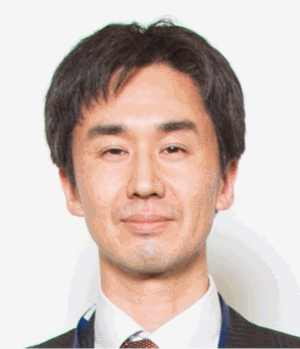
Yoshiyuki Furukawa
Yoshiyuki Furukawa is a research team leader of Industrial Cyber-Physical Systems (ICPS) Research Center at National Institute of Advanced Industrial Science and Technology (AIST) in Japan. He is leading Connected Factory Research Team to advance core technologies related to smart manufacturing and ICPS for manufacturing. He received his B.E., M.E. and Ph.D. from the University of Tokyo in 1998, 2000 and 2003 respectively, in the field of geometric modelling for computer-aided design. Since he joined AIST in 2003, he has been developing technologies to support informatisation and digitalisation in manufacturing industry, especially small and medium-sized enterprises in Japan.
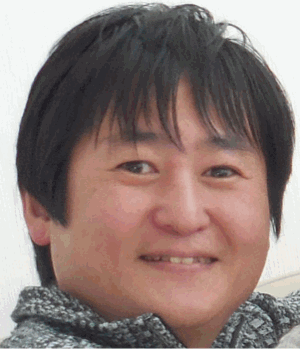
Ichiro Ogura
Ichiro Ogura is a senior researcher at Industrial Cyber-Physical Systems Research Center of National Institute of Advanced Industrial Science and Technology (AIST). He obtained his Ph.D. from School of Engineering of Tohoku University in 1999. He joined Mechanical Engineering Laboratory (MEL) of Agency for Industrial Science and Technology, which is previous organisation of AIST. After reorganisation to the current AIST, he was on New Energy and Industrial Technology Development Organization (NEDO) secondment from 2004 to 2006, and he returned to AIST. His main research focuses on monitoring and distinction of mechanical machined objects and machining processes by optical or image recognition. His research also includes mechanical machining, nano-order machining, and precision measurement.
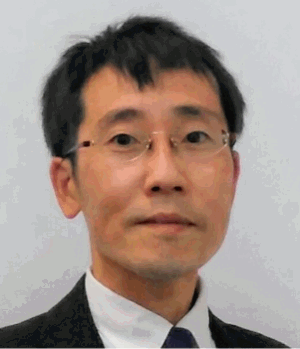
Hitoshi Komoto
Hitoshi Komoto joined National Institute of Advanced Industrial Science and Technology (AIST) in 2011 and he is currently a senior researcher at Industrial Cyber-Physical Systems Research Center, AIST. He obtained Dr. at Delft University of Technology in 2009, Dipl. -Ing and B.Sc at Karlsruhe Institute of Technology in 2004 and 2003, respectively. His research includes design theory and methodology, system architecting, smart manufacturing, and life cycle engineering. He wrote more than 60 international peer-reviewed publications in these research fields. He is an associate member of the International Academy of Production Engineering (CIRP), a member of the Japan Society of Mechanical Engineers (JSME), and a member of the Japan Society for Precision Engineering (JSME). He is an expert in ISO TC184/SC5/WG10 and IEC System Committee Smart Manufacturing.