Abstract
Intermittent demand forecasting is a ubiquitous and challenging problem in production systems and supply chain management. In recent years, there has been a growing focus on developing forecasting approaches for intermittent demand from academic and practical perspectives. However, limited attention has been given to forecast combination methods, which have achieved competitive performance in forecasting fast-moving time series. The current study examines the empirical outcomes of some existing forecast combination methods and proposes a generalised feature-based framework for intermittent demand forecasting. The proposed framework has been shown to improve the accuracy of point and quantile forecasts based on two real data sets. Further, some analysis of features, forecasting pools and computational efficiency is also provided. The findings indicate the intelligibility and flexibility of the proposed approach in intermittent demand forecasting and offer insights regarding inventory decisions.
Acknowledgments
The authors are grateful to the editors and three anonymous reviewers for helpful comments that improved the contents of the paper.
Data availability statement
The RAF dataset has been used in previous literature (Teunter and Duncan Citation2009; Petropoulos and Kourentzes Citation2015; Kourentzes and Athanasopoulos Citation2021) and is available upon request. The M5 competition (Makridakis, Spiliotis, and Assimakopoulos Citation2021) data involves the unit sales of 3049 products between 2011-01-29 and 2016-06-19 (1969 days). The first 1941 observations for model training can be obtained from https://github.com/Mcompetitions/M5-methods; the final 28 observations are available upon request.
Disclosure statement
No potential conflict of interest was reported by the authors.
Additional information
Funding
Notes on contributors
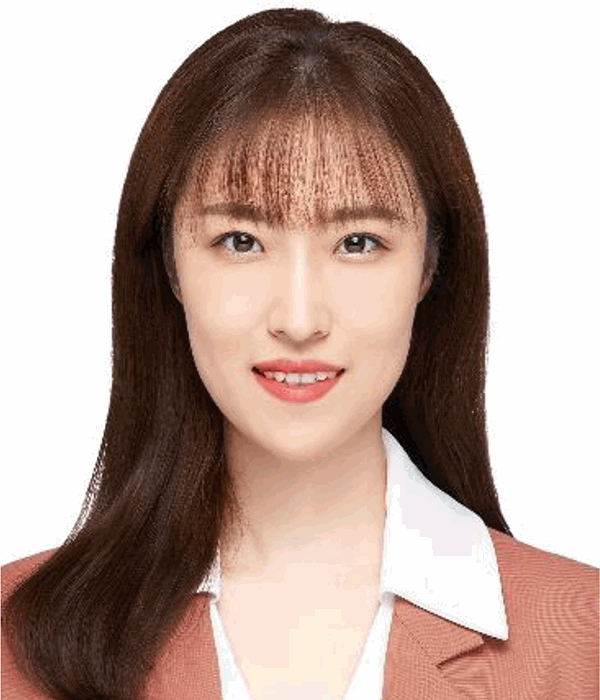
Li Li
Li Li is a Ph.D. student in statistics from the School of Economics and Management, Beihang University, China. She has published many academic papers in journals such as International Journal of Forecasting, Cognitive Computation. Her research interests include time series forecasting and statistical computing.

Yanfei Kang
Dr. Yanfei Kang is Associate Professor of Statistics at Beihang University in China. Prior to that, she was Senior R&D Engineer in Big Data Group of Baidu Inc. Yanfei obtained her Ph.D. degree at Monash University in 2014. She worked as a postdoctoral research fellow during 2014 and 2015 at Monash University. Her research interests include time series forecasting, time series visualisation, text mining and statistical computing.
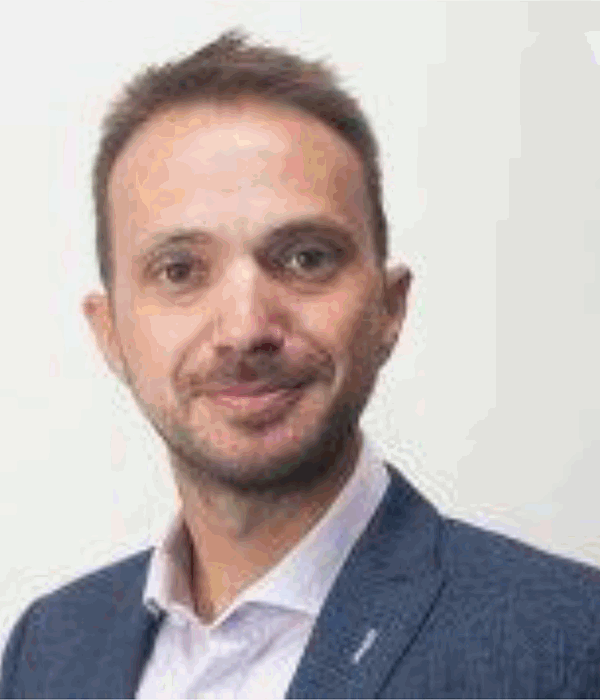
Fotios Petropoulos
Dr. Fotios Petropoulos is Professor of Management at University of Bath, UK. He is interested in research on time series forecasting, judgmental approaches for forecasting, statistical and judgmental model selection and integrated business forecasting processes. His research so far has focused on the improvement of forecasting processes.

Feng Li
Dr. Feng Li is an Associate Professor of Statistics in the School of Statistics and Mathematics at Central University of Finance and Economics in Beijing, China. He earned his Ph.D. degree in Statistics from Stockholm University, Sweden in 2013. Dr. Feng Li's research interests include Bayesian statistics, econometrics and forecasting and distributed learning. He develops highly scalable algorithms and software for solving real business problems.