Abstract
This paper addresses the problem of stochastic jointly scheduling of resumable jobs and preventive maintenance on a single machine, subject to random breakdowns, to minimise the earliness-tardiness cost. The main objective is to investigate using trending machine learning-based methods compared to stochastic optimisation approaches. We propose two different methods from both fields as we solve the same problem firstly with a stochastic dynamic programming model in an approximation way, then with an attention-based deep reinforcement learning model. We conduct a detailed experimental study according to solution quality, run time, and robustness to analyse their performances compared to those of an existing approach in the literature as a baseline. Both algorithms outperform the baseline. Moreover, the machine learning-based algorithm outperforms the stochastic dynamic programming-based heuristic as we report up to 30.5% saving in total cost, a reduction of computational time from 67 min to less than on big instances, and a better robustness. These facts highlight clearly its potential for solving such problems.
Data availability statement
The data that support the findings of this study are available from the corresponding author {Sabri A.}, upon reasonable request.
Disclosure statement
No potential conflict of interest was reported by the author(s).
Additional information
Funding
Notes on contributors
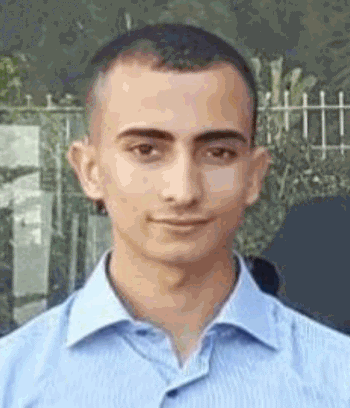
Abderrazzak Sabri
Abderrazzak Sabri is a machine learning engineer from the National Institute of Post and Telecommunications in Rabat, Morocco. He is currently enrolled in a joint Ph.D. program between the National Institute of Post and Telecommunications and the University of Artois. His research focuses on the integration of machine learning in industrial management and operations, specifically in the areas of production scheduling and maintenance planning. He is working on developing new algorithms and models to improve the efficiency and effectiveness of production processes and maintenance activities. He is actively engaged in the field and is committed to making significant contributions to the field of machine learning in industrial management and operations.
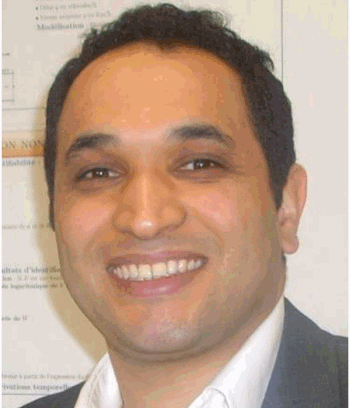
Hamid Allaoui
Hamid Allaoui is a full professor at the University of Artois in France and Director of LGI2A laboratory. After graduating with an engineering degree in electro-mechanical engineering in 2000, he joined ST-MicroElectronics Company as a Manufacturing Engineer. He received a Ph.D. in Computer Science in 2004. His current research covers design, management and optimisation of sustainable supply chains especially scheduling and planning of operations. He has published in several international journals and has been involved in several research and industrial projects.

Omar Souissi
Omar Souissi is an engineer of Supmeca PARIS and Polytechnic of Montréal in applied mathematics and Ph.D. doctorate in Operations research of University Polytechnic Haut de France. He is currently a qualified professor at the National Institute of Posts and Telecommunications (INPT) Rabat and leader of ‘DATA’ research team. His research field extend to the following areas: optimisation and machine learning applied for Industry, Healthcare and sharing economy. He is also engaged on scientific event organisation and he is the founder of ‘IWSIF’ the International Workshop of Services and Industry of the Future.