Abstract
Recent advancement of sensor technology has made it possible to monitor high-dimensional data streams in various manufacturing systems for quality improvement. However, existing monitoring schemes commonly assume that all data streams have the same sampling interval. This assumption does not always hold in practice, which poses new and unique challenges for multivariate statistical process control. In this paper, we propose a generic nonparametric monitoring framework to online monitor high-dimensional asynchronous and heterogeneous data streams, where sampling intervals of data streams are different from each other, and measurements of each data stream follow arbitrary distributions. In particular, we first propose a quantile-based nonparametric framework to monitor each data stream locally for possible shifts in both location and scale. Then, for unsampled measurements due to different sampling intervals, a compensation strategy based on the Bayesian approach is introduced. Furthermore, we develop a global monitoring scheme using the sum of top- local statistics, which can quickly detect a wide range of possible shifts in all directions. Simulations and case studies are conducted to evaluate the performance and demonstrate the superiority of the proposed method.
Disclosure statement
No potential conflict of interest was reported by the author(s).
Data availability statement
The data that support the findings of this study are openly available in UCI Machine Learning Repository at http://archive.ics.uci.edu/ml/datasets/SECOM, and Harvard Dataverse at https://doi.org/10.7910/DVN/6C3JR1.
Additional information
Funding
Notes on contributors
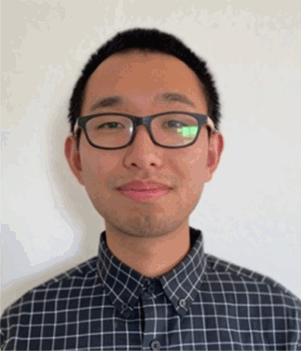
Honghan Ye
Honghan Ye received a BS degree in instrument science and opto-electronic engineering from Hefei University of Technology, Hefei, Anhui, China, in 2015, an MS degree in mechanical engineering from University of Kentucky, Lexington, KY, USA, in 2017, an MS degree in statistics and a PhD degree in industrial engineering from University of Wisconsin–Madison, Madison, WI, USA, in 2021. Currently, he is a data scientist with the Health Information System Division at 3M company to work on developing advanced language models.
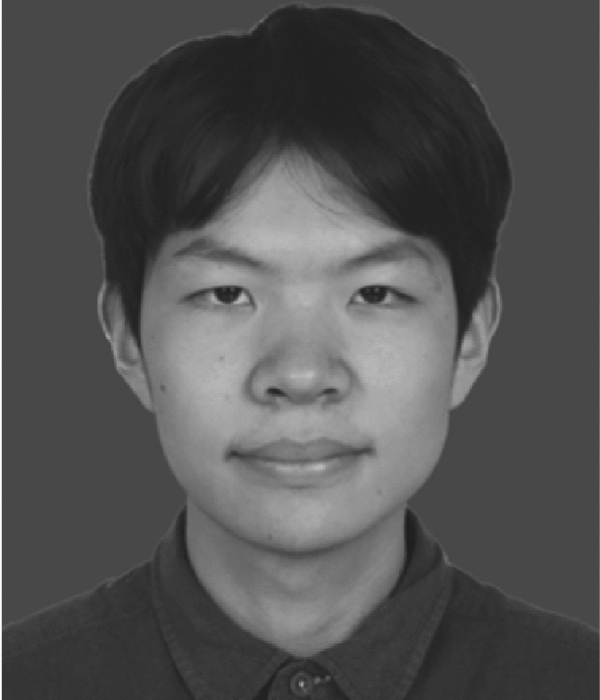
Ziqian Zheng
Ziqian Zheng received the B.E. degree in automation engineering from Xi’an Jiaotong University, Xi’an, China, in 2019. He is currently pursuing the Ph.D. degree with the Department of Industrial and Systems Engineering, University of Wisconsin–Madison, Madison, WI, USA. His research interests include data analytics for system prognosis and big data monitoring.
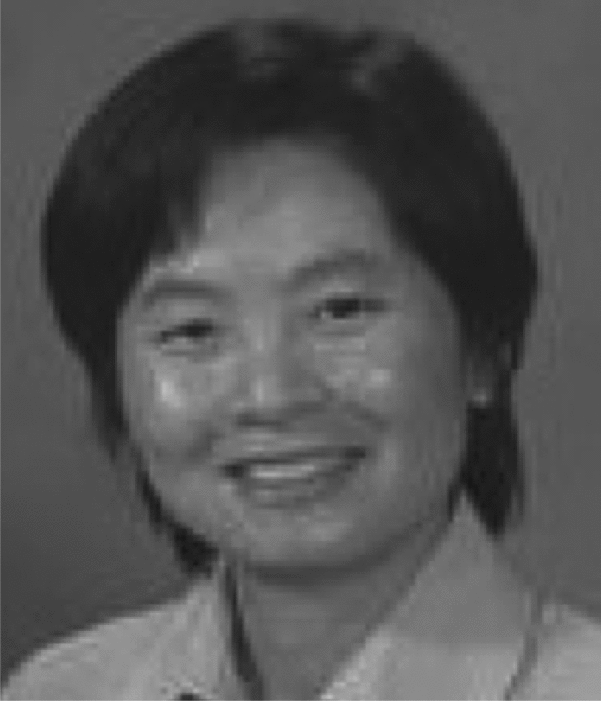
Jing-Ru C. Cheng
Jing-Ru C. Cheng received a PhD degree in computer science from Penn State University, University Park, PA, USA, in 2002. She has been a computer scientist with the U.S. Army Engineer Research and Development Center, Vicksburg, MS, USA, since 2002. Her current research interests include data analytics, object detection, parallel algorithm development, software tool development for scientific computing, and multiscale multiphysics code development.
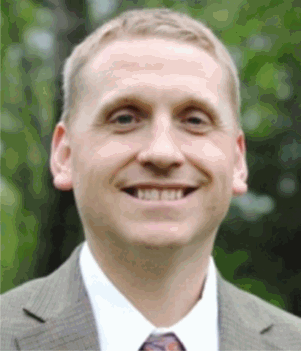
Brock Hable
Brock Hable received his MS degree in electrical engineering from University of Minnesota, Minneapolis, MN, USA, in 2014 and BS degree in electrical engineering and computer science from University of Wisconsin, Madison, WI, USA in 2004. He has been a Researcher and Research Manager with 3M, Maplewood, MN, USA since 2004. His current research interests include artificial intelligence and machine learning and edge solutions for industrial applications.
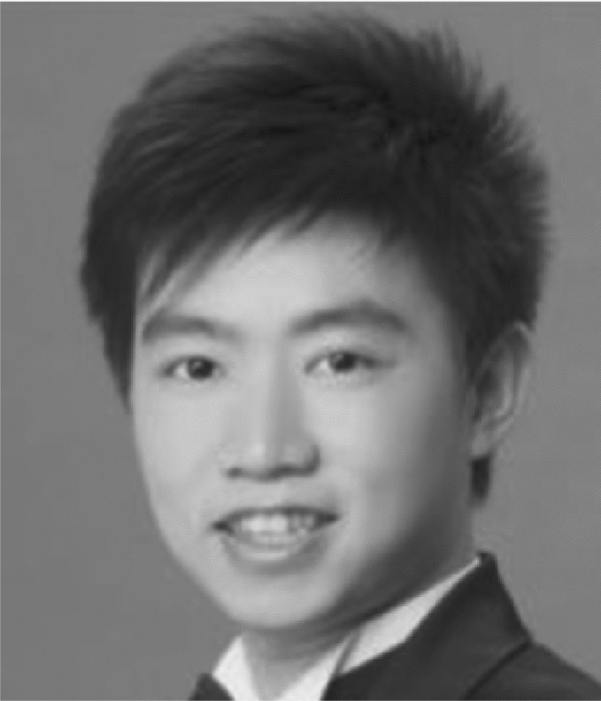
Kaibo Liu
Kaibo Liu received a BS degree in industrial engineering from the Hong Kong University of Science and Technology in 2009, an MS degree in statistics and a PhD degree in industrial engineering from the Georgia Institute of Technology, in 2011 and 2013, respectively. Currently, he is an associate professor with the Department of Industrial and Systems Engineering, University of Wisconsin–Madison, Madison, WI, USA. His research interests are data fusion for process modelling, monitoring, diagnosis, prognostics and decision making. Dr. Liu is a member of ASQ, SME, INFORMS, IEEE, and IISE.